Get amazing AI audio voiceovers made for long-form content such as podcasts, presentations and social media. (Get started for free)
Exploring AI-Driven Audio Enhancement 7 Techniques for Clearer Podcast Production
Exploring AI-Driven Audio Enhancement 7 Techniques for Clearer Podcast Production - Noise Reduction Using Adaptive Algorithms
Adaptive algorithms are playing an increasingly important role in achieving pristine audio quality, especially in areas like podcasting, audiobook production, and voice cloning. These algorithms, often employing methods like adaptive noise cancellation (ANC), can effectively remove unwanted background noise without needing a detailed understanding of the noise itself. This is particularly useful in situations where the nature of the noise is unpredictable or constantly changing.
The performance of these noise reduction techniques hinges on the relationship between the noise and the speech. When noise and speech are largely unrelated (uncorrelated), filtering out the noise becomes significantly easier without negatively impacting the clarity of the spoken words. This is a key principle in many of these algorithms.
To further refine noise reduction, more sophisticated methods are being explored. Hybrid techniques that combine traditional adaptive filters with modern approaches like deep learning are proving very effective. These methods show great promise for real-time noise reduction, leading to a significant improvement in the overall quality of the audio experience. Combining the strengths of different algorithms allows for more robust and versatile noise reduction in diverse audio scenarios.
1. Adaptive algorithms are clever tools that learn to distinguish noise from the desired audio by using statistical modeling. This allows them to dynamically adjust to changing audio environments, a critical aspect for scenarios like live podcasting where background noise can be erratic.
2. Noise reduction often involves techniques like spectral subtraction, where the algorithm estimates the noise's spectral characteristics and subtracts it from the audio signal. This approach has demonstrated a noticeable improvement in voice clarity, particularly in scenarios dominated by constant background noise.
3. Deep learning has become a powerful force in modern noise reduction algorithms. These models can learn to discriminate between the desired audio components and noise by training on extensive datasets. This often leads to them outperforming traditional techniques, especially in complex audio environments.
4. Threshold-based noise gates are frequently used in audio production, allowing users to mute sounds below a certain decibel level. While effective in many instances, they can sometimes produce unintended audio dropouts, highlighting the need for cautious parameter adjustments.
5. Some adaptive algorithms can learn and improve their noise reduction capabilities based on user feedback. By analyzing user preferences, these systems can become more refined and tailored to the specific requirements of podcast creators and audiobook narrators.
6. Strategically inserting periods of silence or low-level noise between audio segments can sometimes enhance perceived clarity in voice recordings. Adaptive algorithms can manage these transitions intelligently, ensuring a smoother and more natural listening experience.
7. While adaptive noise reduction can significantly boost audio quality, it can occasionally produce artifacts, such as a muffled or 'squelchy' sound, especially when applied to highly compressed audio files. Audio engineers must consider these trade-offs when selecting noise reduction strategies.
8. Voice cloning techniques can see significant improvements when paired with noise reduction algorithms, as cleaner input audio leads to more accurate and high-fidelity outputs. This is crucial for audiobook production where the voice replication's authenticity is paramount.
9. Certain adaptive noise reduction systems are designed to be used at various stages of the audio production workflow, from initial recording to post-production editing. This flexibility facilitates consistent noise reduction without sacrificing audio quality at any stage.
10. It's interesting to observe that the effectiveness of adaptive noise reduction can vary significantly depending on the nature of the noise. For instance, continuous background noises like air conditioner hum are often easier to mitigate than transient sounds such as sudden claps or sirens, which can pose a greater challenge for the algorithms.
Exploring AI-Driven Audio Enhancement 7 Techniques for Clearer Podcast Production - Intelligent Vocal Enhancement and Clarity
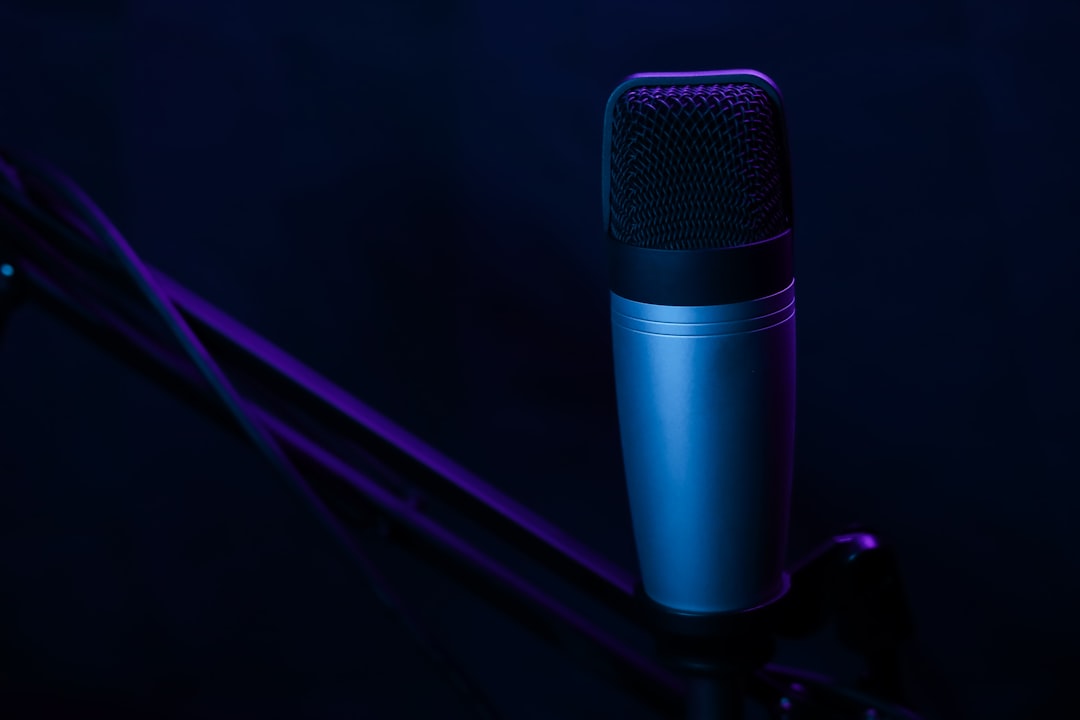
Intelligent vocal enhancement utilizes sophisticated AI techniques to refine and improve the clarity of voice recordings. These algorithms analyze audio signals, adeptly filtering out background noise and enhancing the prominence of the voice itself, making them vital for achieving crisp audio in podcasting, audiobook production, and even voice cloning. The methods employed can involve adjusting the audio's frequency spectrum to create a fuller, richer sound, ensuring the voice maintains its natural characteristics while becoming more prominent in the mix. This capability is particularly beneficial for creators working with recordings made in less-than-ideal acoustic spaces. Although these enhancements can yield remarkably better audio, it's essential to exercise caution when applying them, as overuse can result in unintended side effects such as audio artifacts or a loss of the natural nuances of the voice. While the pursuit of perfect sound is admirable, it shouldn't compromise the authentic qualities of the human voice.
Intelligent vocal enhancement and clarity are fascinating areas of audio engineering, particularly as we delve deeper into the nuances of human speech and how it interacts with the surrounding soundscape. The human voice has a characteristic frequency range, roughly from 85 Hz for the lower notes to around 1,100 Hz for higher harmonics. This knowledge is foundational for audio engineers who design algorithms to enhance voice while avoiding distortion. Successfully amplifying these frequencies is crucial for making voices stand out, particularly in environments with background noise.
When we consider voice cloning, maintaining a sense of naturalness and realism requires techniques like phase vocoding. This sophisticated process alters both the amplitude and phase of an audio signal, preserving the unique timbre of a voice while enabling incredibly precise replication. For example, in audiobook production, the ability to recreate a voice with such fidelity relies on these manipulations.
Formant interpolation provides another dimension in achieving clear voices, especially when dealing with diverse accents or emotional tones. This approach involves shifting the resonant frequencies of the vocal tract – in essence, fine-tuning the 'shape' of the voice – to align with a target voice. The results can be quite striking, improving clarity even in the face of a significant level of noise.
It's intriguing to consider the role of emotional tone in perceived clarity. Researchers are starting to uncover how our perception of a voice's clarity is affected by the emotional cues within the sound. This suggests that future AI-driven systems could adapt pitch and dynamics based on the emotional context of speech. Such adaptive systems could make a substantial difference in overall engagement and the perceived clarity of the audio experience.
Improvements in sample rate conversion play a significant role, particularly in voice cloning applications. Higher sample rates, which capture a more comprehensive snapshot of the voice's intricacies, are critical for high-quality voice cloning. This becomes particularly important in audiobook production where accurately reproducing subtle voice characteristics is vital for achieving authenticity.
Interestingly, a touch of reverb or echo can sometimes improve perceived vocal clarity by enriching the sound. However, this effect requires careful management. Excessive reverberation can mask subtle details of the voice, diminishing the overall quality. The goal is to enhance the perceived richness without obscuring the essence of the vocal performance.
Psychoacoustic models are a crucial guide in the design of vocal enhancement systems. These models help us understand how humans perceive sound, allowing engineers to tailor enhancement strategies to our auditory system's sensitivities. It's notable that frequencies around 2 kHz are particularly important for speech intelligibility and therefore should be a central focus in enhancement algorithms.
The ‘S' sound, or sibilance, is often a source of unwanted harshness in audio. Excessive sibilance can detract from clarity. Techniques like dynamic equalization can help tame this harshness in those frequencies, resulting in a smoother and more pleasant audio experience without sacrificing the voice's fundamental characteristics.
While we often associate vocal clarity with the benefits of audio compression, it's important to remember that this process can result in the loss of data. Balancing the benefits of compression against the potential for sound degradation is a constant challenge. Finding the optimal compression levels for podcast and audiobook productions is a crucial step towards achieving the best audio quality.
Finally, the algorithms that are used in real-time audio processing for live podcasting need to be exceptionally quick and adaptable. They must respond in near-instantaneous time to changes in the speaker's voice and environmental noise. Delays can significantly degrade the overall experience and introduce a sense of artificiality. The optimization of such real-time systems is critical to ensuring a seamless and natural listening experience for the audience.
Exploring AI-Driven Audio Enhancement 7 Techniques for Clearer Podcast Production - Automated Audio Leveling and Compression
Automated audio leveling and compression are vital tools for creating high-quality audio, especially for podcasts, audiobooks, and voice cloning. These techniques, often powered by AI, aim to achieve consistent volume levels throughout an audio recording. Imagine a podcast with varying speaker volumes – it can be jarring for the listener. Automated leveling helps address this by ensuring a smooth and uniform listening experience, adjusting the volume of different sections to match a desired output.
Compression, a related technique, helps control the dynamic range of audio, making quieter parts louder and tamping down overly loud sections. This leads to a more balanced sound that's easier to listen to, particularly in situations with unpredictable volume changes. This automation, particularly with tools designed for audio production, can be a real time saver and improves the audio's overall professional sound.
However, it's crucial to use these techniques judiciously. Over-compression can sacrifice the natural nuances of a voice, potentially creating an unnatural, processed sound. This becomes more important for voice cloning as it can affect how convincing the clone sounds. Finding the ideal balance between consistent volume and retaining audio fidelity is key to achieving high-quality results. Ultimately, these automated techniques provide a significant advantage in the audio production workflow, allowing creators to streamline their process and produce clearer, more professional-sounding output.
Automated audio leveling and compression techniques are designed to dynamically adjust the loudness of audio signals, often using a process called dynamic range compression. This aims for consistent volume, which can be beneficial for listeners who find fluctuating levels distracting, especially during podcast episodes. These systems often rely on principles of how humans perceive different frequencies and loudness, prioritizing key frequencies for improved clarity without excessive overall volume increase, creating a more enjoyable listening experience.
However, the configuration of automated compression can be quite complex, involving parameters like thresholds, ratios, attack, and release times. Incorrect settings can introduce unwanted artifacts like pumping or breathing sounds, particularly noticeable during quiet sections of audiobooks or podcasts. Furthermore, as cultural perceptions of loudness and clarity differ, audio levelers ideally account for cultural context during development. This is important when tailoring compression algorithms for international audiences.
Modern audio compression technologies often utilize machine learning to analyze audio streams. They identify patterns in speech and musical elements, applying specific compression algorithms for individual parts of the audio rather than a generalized approach.
Interestingly, automated audio leveling can sometimes conflict with the creator's artistic intent. While seeking clarity, these systems might alter the emotional impact of the audio by compressing dynamic ranges that were intentionally emphasized by the creator. Multi-band compression has emerged as a solution, allowing for separate compression of different frequency ranges. This permits tightening the bass without affecting higher-frequency clarity, making it valuable for enhancing voices within podcasts with music or background sounds.
Excessive compression can lead to listener fatigue as overly processed audio can sound unnatural. Balancing clarity with the preservation of the voice's inherent characteristics is essential for a comfortable listening experience. In voice cloning, automated leveling helps improve synthetic voices, ensuring stability in the cloned speech for a smoother, more authentic experience in audiobook productions. Some audio leveling systems even incorporate feedback mechanisms to learn from user interactions, allowing for adaptive adjustments based on individual listener preferences and enhancing the user experience. This can further refine the compression levels for different content, ensuring an optimal listening experience.
While these systems aim for consistent loudness and clarity, it's important to remain aware that audio artifacts might occur and there may be unintended alterations to the creator's original vision. Striking a balance between the desire for clean, clear audio and the integrity of the original source remains an important factor to consider when employing these automated techniques.
Exploring AI-Driven Audio Enhancement 7 Techniques for Clearer Podcast Production - AI-Powered Equalization for Optimal Frequency Balance
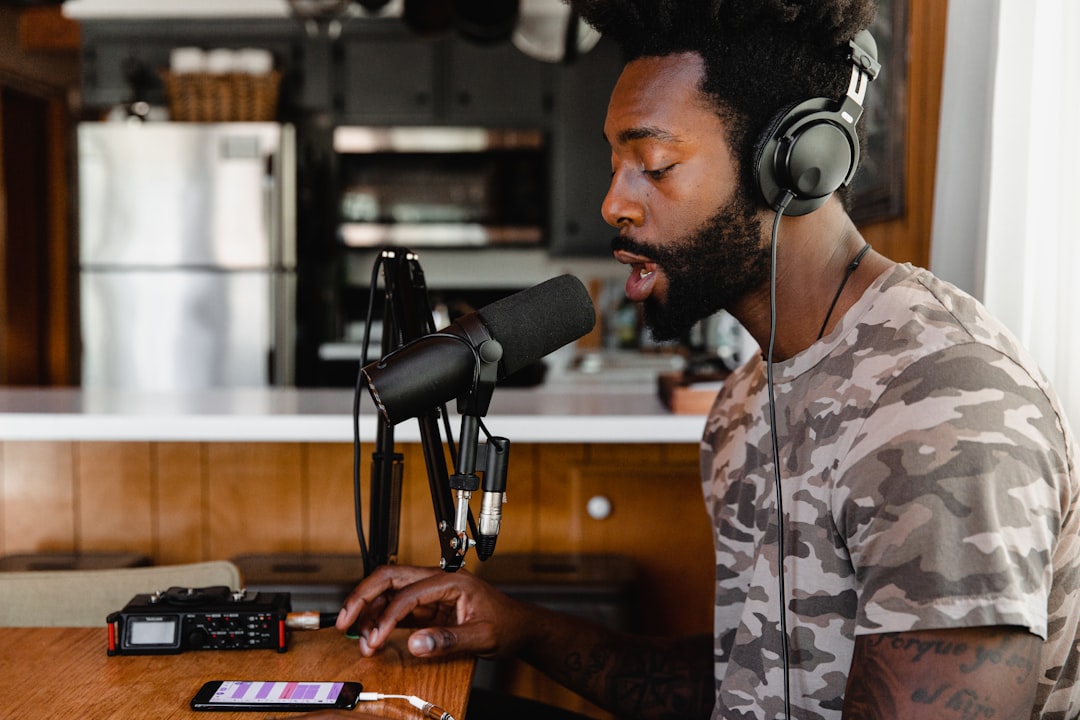
AI-powered equalization offers a compelling approach to refining audio, particularly in contexts like podcasting, audiobook production, and voice cloning. These systems use intelligent algorithms to analyze audio and automatically adjust frequencies, resulting in a balanced and optimized sound. This intelligent equalization process eliminates the need for extensive technical knowledge, making it more accessible to a broader range of creators. By pinpointing and managing specific frequencies, AI can effectively reduce unwanted noise and address acoustic imperfections, improving the overall clarity and quality of recordings. The enhancement of voice clarity is especially valuable for podcasters and audiobook narrators striving for a polished, professional sound.
However, while the promise of perfect audio is tempting, it's crucial to consider that over-reliance on these automated tools could compromise the natural qualities of human voices. The subtle nuances that lend authenticity and connection to spoken word should not be sacrificed for a technically flawless but potentially sterile sound. As AI continues its development, its potential to revolutionize audio production and make high-quality recordings accessible to a wider range of creators becomes more apparent. This represents a significant step forward in how we create and experience audio, yet the future of the technology depends on its responsible and balanced implementation.
AI-powered equalization is a fascinating development in audio production, particularly for applications like podcasting, audiobook creation, and voice cloning. These systems use artificial intelligence to automatically adjust the balance of different frequencies in a recording. For example, they can intelligently boost the frequencies around 2-4 kHz, a range where human speech is most prominent, making voices clearer even in noisy environments.
Beyond boosting certain frequencies, AI equalization also identifies and reduces undesirable ones. Often, frequencies below 250 Hz contribute to a muddy, unclear sound. By automatically reducing these, AI algorithms can refine the audio, making the voice more distinct. This is particularly relevant in voice cloning applications, where clean, well-balanced audio is essential for accurate replication of a person's voice. Creating a realistic-sounding audiobook requires this precision.
Interestingly, the environment where someone listens to audio can impact how they perceive its quality. Some AI equalization systems can adapt their frequency adjustments based on detected background noise. So, whether someone is in a quiet room or a bustling café, the audio experience can be optimized.
Furthermore, many AI equalization tools are designed to learn from user feedback. They adapt and refine their adjustments over time, developing a sound profile tailored to individual preferences. This is beneficial for podcast producers looking for a specific audio character.
The application of AI equalization isn't limited to a single approach. Different genres of audio require distinct frequency adjustments. Voice-focused content, like podcasts, benefits from enhanced mid-range frequencies, while music might need more emphasis on bass or treble, demonstrating the versatility of these systems.
Maintaining phase coherence during equalization is crucial to prevent degradation of audio quality. AI-powered equalizers are typically designed to avoid introducing phase shifts that can lead to comb filtering, which produces unwanted variations in the frequency response.
AI integration also enables the creation of presets tailored for different acoustic settings. For instance, a podcast recorded in a small room might benefit from a different equalization setting than one recorded in a larger space. This adaptive capability ensures that the audio is optimal for the environment.
However, it's important to remember that over-processing can negatively impact audio. AI-powered equalizers, unlike some traditional methods, typically employ subtle, context-aware adjustments to maintain the natural dynamics and character of the voice. This careful approach is vital for creating engaging podcasts and audiobooks that don't sound overly processed or artificial.
Remarkably, some AI equalizers are capable of working in real-time during live recordings. This is particularly useful for podcasts where multiple speakers with varying vocal characteristics might participate. Real-time equalization allows for continuous maintenance of optimal frequency balance, resulting in a consistent and engaging audio experience for the listener.
In conclusion, AI-powered equalization is a rapidly evolving field with the potential to significantly improve the quality of audio across a wide range of applications, from podcasts to audiobooks and voice cloning. As these technologies mature, we can anticipate even more innovative solutions for enhancing our audio experiences.
Exploring AI-Driven Audio Enhancement 7 Techniques for Clearer Podcast Production - Smart De-essing and Plosive Removal
Smart De-essing and Plosive Removal represent a significant step forward in audio enhancement, particularly valuable for podcasting, audiobooks, and voice cloning applications. This AI-powered approach offers a more nuanced solution compared to older techniques. Instead of just reducing volume when harsh sounds like "s" or plosives occur, these systems analyze the entire audio signal, understanding the specific characteristics of a voice or instrument. This "content-aware" processing adjusts the sound in a way that feels more natural, creating a smoother and more balanced audio experience.
A key aspect is the ability to dynamically identify and address these problematic sounds in real-time. The technology can essentially create a unique 'voiceprint' to understand how to best treat each voice, which is especially beneficial for maintaining authenticity when dealing with recordings or cloned voices. This innovation simplifies the audio editing workflow, freeing creators to focus on content rather than laborious manual corrections. The improvements in vocal clarity and overall sound quality offered by this method are likely to have a strong positive impact on listener experience and engagement. It's reasonable to expect that as AI-driven audio enhancement continues to develop, tools like Smart De-essing and Plosive Removal will become increasingly important for anyone striving for high-quality audio in their productions.
Smart de-essing and plosive removal are fascinating areas within AI-driven audio enhancement. These techniques are specifically designed to tackle issues like sibilance (the harsh 's' sounds) and plosive sounds (the bursts of air from 'p' and 'b' sounds) that can detract from the clarity of audio, especially in podcasting, voice cloning, and audiobook production.
The way these tools work is quite interesting. Rather than just lowering the volume of these harsh sounds, they utilize what's called spectral processing. This essentially means they shape the sounds themselves, offering a more nuanced approach to audio refinement. It's a bit like sculpting a sound rather than simply cutting off parts of it, leading to a more natural-sounding result.
One of the most interesting aspects of these AI-powered solutions is their adaptability. They can create a kind of "voiceprint" during playback, which allows them to tailor the processing to match the specific nuances of a particular voice. This ensures that the de-essing and plosive removal are optimized for the unique qualities of each speaker or voice clone.
It's also worth mentioning that some of these techniques can introduce issues if not carefully managed. For instance, phase interference can sometimes lead to unnatural shifts in the tone of the audio if the de-essing isn't carefully applied. Additionally, if too much sibilance is removed, it can actually make the speech less clear and harder to understand. Balancing these trade-offs requires a degree of finesse and careful consideration of how these tools affect the overall sound.
Furthermore, the techniques used for plosive removal are multifaceted, combining high-frequency filtering with adjustments to the audio's transient components. This targeted approach aims to reduce the impact of these burst sounds without completely removing their natural character.
It's interesting to note that while automated de-essing offers a streamlined solution, some audio engineers prefer to combine it with a more manual approach. This combination gives them the flexibility to address specific aspects of the audio where a more fine-grained control is needed. Also, the audio world is constantly evolving, but these smart de-essing methods seem to be compatible with older recording styles, offering a way to improve older recordings while still respecting their vintage qualities.
Another advantage of some of these modern solutions is that they can process audio in real-time, allowing for adjustments during live recordings and broadcasts. This is a huge benefit for podcasters or voice actors doing live performances.
In essence, the implementation of psychoacoustic principles within these algorithms is key. By understanding how humans perceive sound, these AI tools can carefully manage the frequencies in a way that is pleasant and natural to our ears.
As these AI tools continue to develop, they promise to become even more effective and accessible. This potential for more accessible audio clarity is exciting, but it's always essential to carefully consider how these tools impact the final audio product to ensure it doesn't lose its unique character in pursuit of technical perfection.
Exploring AI-Driven Audio Enhancement 7 Techniques for Clearer Podcast Production - Adaptive Room Acoustics Correction
Adaptive Room Acoustics Correction (ARAC) is a relatively new technique within AI-driven audio enhancement. It's proving particularly helpful in situations where recording environments aren't ideal, like podcasting in home studios or even voice cloning when the space isn't acoustically treated.
The way ARAC works is by using intelligent algorithms to analyze the room's shape, the speaker's position, and even listener placement within a room. Based on these details, the algorithms can adapt the audio in real time, compensating for issues caused by the room's specific acoustics. This can significantly help in situations where echoes, reverberations, and other undesirable effects are present. ARAC systems essentially tailor the audio experience to the specifics of the room, which is especially useful for podcasters and audiobook narrators.
However, this type of correction needs to be applied carefully. Too much manipulation can create an unnatural, overly processed sound. While the goal is to have crystal-clear audio, it's equally important to preserve the genuine qualities of the human voice. Striking a balance is crucial to ensure the audio sounds polished but not artificial. If not done carefully, these systems could unintentionally distort the natural timbre of a voice or, in the case of voice cloning, compromise the effectiveness of the replication.
Adaptive room acoustics correction is a fascinating area of audio enhancement, especially relevant for podcasting, audiobook production, and voice cloning. These systems dynamically adjust to the unique acoustic properties of a room, compensating for irregularities caused by factors like room shape, furniture placement, and surface materials. This adaptability is crucial for recording environments where sounds are unpredictable, like a live podcast setting.
Research indicates that the way we perceive audio is strongly influenced by the room's acoustics. For example, a well-corrected room can make voices sound more clear and authoritative, suggesting that background sound reflections can significantly change the overall perceived audio quality. These systems often focus on specific frequency ranges, like 300 Hz to 1 kHz, which are vital for ensuring speech intelligibility.
One major challenge these systems address is the issue of room modes. These are essentially standing waves that form at specific frequencies and can create significant peaks and dips in the audio frequency response. This problem is usually more pronounced in smaller, untreated rooms, making sophisticated algorithms essential for achieving balanced audio.
Interestingly, an adaptive correction system might treat audio differently depending on whether it's being recorded or played back. This distinction calls for adaptable processing strategies that maintain the desired audio quality for both situations.
Machine learning is increasingly being integrated into these systems to further refine their abilities. By analyzing large datasets from diverse acoustic environments, these algorithms are learning how to adapt and optimize for different scenarios. This can lead to more sophisticated and versatile room acoustic correction.
These systems can handle multiple audio channels efficiently, making them well-suited for environments with multiple speakers, like a multi-person podcast recording. They can also enhance the listener's sense of spatial audio, improving their perception of the sound's origin and leading to a more immersive and natural listening experience.
Some advanced systems include feedback mechanisms that monitor listener reactions in real time. By adapting their adjustments based on audience engagement, they can optimize the audio presentation for optimal listener satisfaction.
While traditional methods of acoustic treatment can be costly and involve significant physical modifications, adaptive room acoustics correction provides a more accessible alternative. Using software to correct recorded audio makes high-quality production more feasible for creators, especially independent podcasters and audiobook producers, without requiring significant investment in soundproofing or physical treatment.
The constant evolution of these technologies suggests that adaptive room acoustics correction will continue to play a growing role in enhancing our audio experience, making high-quality audio more readily available across a variety of audio production scenarios.
Exploring AI-Driven Audio Enhancement 7 Techniques for Clearer Podcast Production - Real-Time Audio Upscaling and Resolution Enhancement
Real-time audio upscaling and resolution enhancement offer a powerful way to improve audio quality, especially for podcasts and audiobooks. These techniques, driven by AI, effectively take lower-quality audio and enhance it on the fly, increasing the sampling rate and adding more detail to the sound. AI models specifically designed for this purpose can process audio signals with impressive precision, generating higher-fidelity results from originally lower-quality sources. This can bring new life to recordings that might have been made under less-than-ideal conditions. While this technology has the potential to create remarkably clean audio, it's important that the natural characteristics of the original sound, particularly the human voice, aren't lost in the process. Overly manipulated audio can often sound artificial and sterile, diminishing the authentic connection with the listener. As these AI-powered upscaling methods continue to mature, they'll likely become essential tools for anyone involved in creating audio content, particularly in demanding areas like voice cloning for audiobooks, where preserving a sense of realism is crucial.
Real-time audio upscaling and resolution enhancement are fascinating areas within AI-driven audio enhancement, offering a way to improve the clarity and quality of audio in various applications, including podcasting, audiobook production, and voice cloning. The core idea is that AI algorithms can analyze audio signals and intelligently add information to them, essentially creating higher resolution audio from lower resolution sources. This is accomplished by using techniques like super-resolution, which are adapted from the field of image processing.
For instance, some models, like AudioSR, are able to handle audio signals with very high sampling rates, allowing for remarkably detailed and accurate outputs across a range of genres. This ability to work with higher sampling rates allows for a more comprehensive capture of the intricacies of sound, especially important in capturing subtle aspects of voice and instrument performance. There are even open-source upscaling tools that are built on frameworks like IA Hispano, making this technology more accessible to researchers and audio engineers who want to experiment with its potential.
However, effectively upscaling audio in real-time demands adaptability. The algorithms used need to analyze the audio as it comes in and modify their parameters based on what they find. They need to react to changes in the source material and the audio environment almost instantly. This need for dynamic adjustments is especially critical in situations like live podcasts where the audio input can vary widely. It's also remarkable that many of these solutions are built to work as plug-ins, making them very easy to add to existing audio production workflows.
Testing has shown that AI models can lead to a genuine improvement in audio quality. Whether evaluated using standard objective measurements or through more subjective listening tests, the results often show that upscaling delivers a better audio experience. There are several neural networks designed for this specific purpose. One interesting example is NUWave, which takes low-bit, coarse audio and generates a high-resolution version.
Besides upscaling, many of these systems also include tools for real-time audio restoration. These tools can address common problems like audio damage, and also refine the soundstage, increasing the sense of width and depth for a more immersive listening experience. The development of new algorithms, specifically focused on dynamic audio, allows these applications to generate remarkably detailed audio across a wide variety of applications.
It's noteworthy that while upscaling promises greater fidelity and clarity, there's always the possibility of introducing artificial-sounding artifacts if the system isn't carefully designed and tuned. Furthermore, while these technologies show considerable promise, they continue to evolve, and the best methods are still being explored. The future of real-time audio upscaling will depend on how well engineers can address these nuances and design systems that enhance audio without losing its natural character.
Get amazing AI audio voiceovers made for long-form content such as podcasts, presentations and social media. (Get started for free)
More Posts from clonemyvoice.io: