Get amazing AI audio voiceovers made for long-form content such as podcasts, presentations and social media. (Get started for free)
7 Open-Source TTS Engines with APIs A 2024 Performance Analysis
7 Open-Source TTS Engines with APIs A 2024 Performance Analysis - MaryTTS Voice Building Tool for Custom Audio Data
MaryTTS offers a unique feature for crafting custom voices using your own audio recordings, making it particularly useful in audio production scenarios. Imagine crafting unique voices for audiobooks, podcasts, or even experimenting with voice cloning. The modular nature of MaryTTS's design, combined with an intuitive graphical interface, simplifies the process of voice creation. This is advantageous as it doesn't require extensive technical knowledge, opening it up to a broader range of users. Beyond voice cloning, MaryTTS embraces multilingual support, which is a valuable asset in fostering diversity and inclusivity within speech synthesis.
Rooted in the principles of open-source software, MaryTTS enables users to freely access, modify, and redistribute its resources. This encourages a collaborative community dedicated to improving text-to-speech technologies. Recent updates to MaryTTS have emphasized enhancing language and voice support, suggesting a clear direction towards greater voice quality and variety. This, in turn, should benefit both developers and content creators seeking to leverage diverse and high-quality synthetic voices.
MaryTTS, a Java-based open-source platform originating from a collaboration between German research institutions, offers a unique capability for crafting custom voices. It's particularly intriguing for voice cloning, enabling the creation of new voice models with just a modest amount of audio – potentially significantly reducing the data demands compared to other methods. This opens doors for projects needing tailored voices, like audiobook production, without the need for extensive data collection.
The open-source nature empowers the community. Developers can readily tinker with and enhance its core functions, fostering an environment where researchers can push the boundaries of voice synthesis. This flexibility extends to the tool's multilingual abilities, catering to the growing needs of producing localized audio content, a key aspect in podcasting and international audiobook creation.
Interestingly, MaryTTS relies on concatenative synthesis – assembling speech segments to generate output. This approach, while perhaps less sophisticated than some newer models, can achieve natural-sounding voices and replicate finer aspects of speech like pitch and intonation. The built-in controls over speech prosody allow for crafting audio with specific emotional intent. This type of fine-tuning might be necessary for conveying subtle cues in audiobook narration or specific podcast styles.
Further, audio quality is a critical focus. The system's underlying signal processing mechanisms aim to clean up the generated audio, removing unwanted artifacts and ensuring a professional output. This attention to detail makes MaryTTS applicable in diverse situations, ranging from educational materials to interactive experiences needing bespoke voice outputs.
While MaryTTS isn't a new project, the ongoing development of tools and components aims to expand its capabilities, specifically for supporting new languages and making it easier to create custom voices. Its documentation and community support provide a robust resource base for anyone interested in mastering voice generation. Beyond basic voice generation, the tool also allows users to dive deep into phonetic features for a more precise control over pronunciation. This granular level of control can prove helpful for scenarios demanding consistent and distinct voices, as seen in voiceover projects or interactive systems where a specific vocal style needs to be maintained.
The real-time capabilities of MaryTTS are noteworthy. The architecture prioritizes swift output, making it potentially suitable for immediate audio generation in interactive or real-time applications like virtual assistant prototypes or voice dubbing, where quick, on-demand audio is needed. While not necessarily the most cutting-edge TTS engine, its combination of features and community-driven development makes it a worthwhile option to explore, especially for researchers and hobbyists keen on exploring TTS.
7 Open-Source TTS Engines with APIs A 2024 Performance Analysis - Tacotron 2 Deep Learning for Expressive Speech Synthesis
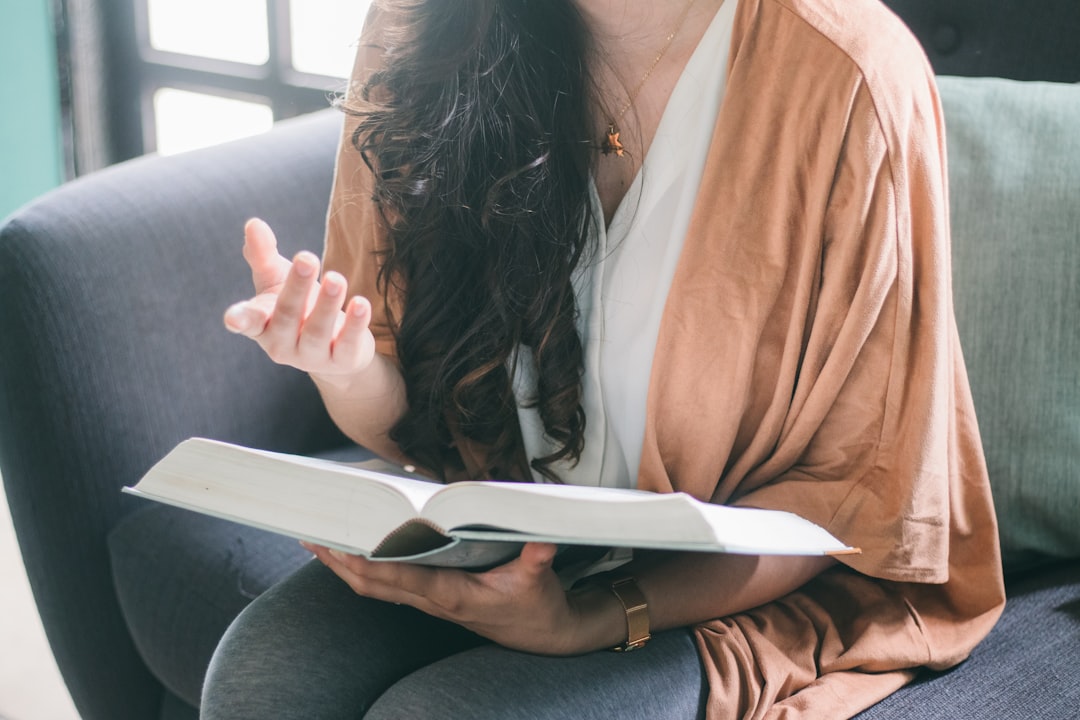
Tacotron 2 stands out as a notable advancement in the field of speech synthesis, employing deep learning to generate human-like speech from text input. It leverages a sequence-to-sequence model that transforms text into mel-spectrograms, a representation of sound that captures the essential features of speech. This approach simplifies the process of building synthetic voices by eliminating the need for intricate intermediate steps, ultimately contributing to more natural-sounding results. Further enhancing the output quality, Tacotron 2 integrates a modified version of the WaveNet model, a powerful neural network capable of synthesizing audio waveforms from the predicted spectrograms.
The resulting synthesized audio is remarkably lifelike, making Tacotron 2 particularly well-suited for applications that require high-fidelity speech. This includes audiobook creation, podcast production, and even voice cloning, where recreating a person's unique vocal characteristics is the goal. The availability of Tacotron 2 through open-source platforms, often accompanied by related models like WaveGlow, signifies the growing trend towards open and accessible speech synthesis tools. This trend, combined with ongoing research into neural network-based speech technologies, has led to considerable improvements in synthetic speech quality. However, it is important to acknowledge that while the technology continues to improve, it's still evolving, and there is always room for further advancements in achieving even greater realism and expressiveness in synthetic voices.
Tacotron 2 employs a blend of recurrent and convolutional neural networks to transform text into mel spectrograms, a process that effectively links linguistic data with sound waves, resulting in more natural-sounding synthetic speech. This approach allows for greater control over features like pitch, energy, and duration, opening possibilities for creating voices that reflect a range of emotions or speaking styles, a highly desired feature for captivating audiobook or podcast narrations.
The model's design allows for direct training from raw audio and corresponding text, meaning it can learn to generate high-quality speech from a comparatively smaller training dataset compared to traditional methods. The use of a WaveGlow vocoder in Tacotron 2 further improves the final sound output, resulting in clearer, richer audio. This aspect is especially valuable in voice cloning scenarios where detailed and personalized digital voices are the goal.
However, Tacotron 2 can be quite sensitive to changes in the input text. Minor variations can significantly alter pronunciation or intonation, posing a challenge for maintaining consistent voice quality across different scripts in projects like audiobook creation or podcast series.
The training process itself is resource-intensive, requiring large datasets of paired text-audio examples. This can make it less accessible for smaller projects or those focusing on niche voice types. It's a contrast to more traditional approaches where extensive manual tuning might be needed, as Tacotron 2 learns pronunciation based on context, which leads to a more natural flow of speech that aligns with the intended tone.
Using attention mechanisms, Tacotron 2 focuses on the most relevant portions of the input text when generating specific parts of the speech output, improving both efficiency and contextual awareness. This is especially helpful when dealing with longer audio segments like audiobooks, ensuring a smoother and more nuanced narrative.
Subjective listening tests have demonstrated that Tacotron 2 produces voices frequently indistinguishable from human speech, setting a high bar for the field. The audio quality is noteworthy and promising for applications in voice cloning or podcast production.
Despite these successes, Tacotron 2 struggles with certain phonetic challenges, like pronouncing proper nouns or complex linguistic constructions, suggesting a path for future development in fully capturing the intricate nuances of human speech. These aspects need further refinement to truly create perfect synthetic voices.
7 Open-Source TTS Engines with APIs A 2024 Performance Analysis - Deepgram's Aura API Real-Time Voice Synthesis
Deepgram's Aura API, introduced in March 2024, is a real-time voice synthesis tool designed to create natural and responsive speech. Its impressive speed, with latency under 250 milliseconds, positions it among the quickest TTS solutions currently available. Aura is geared towards diverse uses, including virtual assistants and large-scale media creation, and delivers high-quality voices that strive for authentic tone and emotional expression, features crucial for engaging interactions in things like podcasts or audiobook production. Deepgram promotes it as a cost-effective and scalable API, handling both real-time interactions and batch processing. While Aura looks appealing, particularly in situations where swift response and conversational nuance are essential, it's important to remember the TTS landscape is dynamic, and careful evaluation is key to deciding if Aura's features truly meet the specific needs of a project, be it voice cloning or interactive story-telling.
Deepgram's Aura API, launched in March 2024, focuses on real-time voice synthesis, aiming to create natural-sounding conversations. It's built on modern deep learning, utilizing techniques like recurrent neural networks and attention mechanisms to generate speech quickly and effectively. This "end-to-end" approach, where the entire process from text to speech happens within a single model, helps reduce delays. One of Aura's key strengths is its ability to adapt to different speakers with minimal training data. This makes it appealing for voice cloning or crafting specific vocal styles in podcasts or audiobooks. It supports numerous languages and dialects, which is a significant benefit when targeting global audiences.
Furthermore, Aura's controls offer the ability to alter emotional tone and speech patterns (prosody) within the synthesized voice. This opens up interesting possibilities for adding nuances to voiceovers or narration in audiobook productions. Achieving a low latency of under 250 milliseconds is impressive, making it suitable for interactive uses like voice assistants and even live event commentaries. One interesting aspect is its robustness to noise, which means it can potentially produce high-quality audio even in environments with background sounds, a useful feature for field recordings and interview applications.
Aura's developers focused on making it easy to integrate into other applications and services. It seems that they've built in a self-improving aspect, enabling the system to learn from user data over time. This continuous learning aspect should mean that the audio quality and adaptation features continue to refine over time. The API's design with good documentation and a streamlined integration process makes it accessible for various users, from those experimenting with prototypes to engineers developing more complex systems. While Aura and Deepgram position it as a comprehensive solution for speech AI within its platform, the future will show how it compares to other platforms in specific niche applications like voice cloning or high-quality audio book production. It's certainly an interesting option for those needing quick, high-quality, synthetic voices.
7 Open-Source TTS Engines with APIs A 2024 Performance Analysis - Mozilla TTS Tacotron 2 and WaveGlow Integration
Mozilla's TTS system, built upon Tacotron 2 and WaveGlow, presents a compelling approach to generating lifelike speech from written text. Tacotron 2, a neural network model, transforms text into mel-spectrograms, which represent the sound's essential characteristics. WaveGlow then takes these spectrograms and converts them into actual audio, resulting in high-quality synthesized speech. This method is particularly beneficial for endeavors such as audiobook production and podcasting where a sense of natural, emotional delivery is important. The strength of Tacotron 2 lies in its ability to infuse synthesized speech with subtle emotional nuances, which can greatly enhance the listening experience.
However, this technology comes with challenges. Tacotron 2, being sensitive to even minor changes in the input text, can sometimes struggle to maintain consistent vocal characteristics. Additionally, training the model, especially with customized datasets for voice cloning purposes, can be a computationally demanding process. Nonetheless, the open-source nature of Mozilla TTS provides a platform for collaboration and improvements, ensuring its continuous development. This evolving technology holds promise for future advancements in voice synthesis, but achieving truly indistinguishable synthetic voices requires continued research and refinement to overcome the current limitations in areas like pronunciation of complex phrases and maintaining absolute consistency.
Mozilla's text-to-speech system relies on Tacotron 2 and WaveGlow to generate audio from text. Tacotron 2, a deep learning model, converts text into mel-spectrograms, essentially a visual representation of sound. WaveGlow, a vocoder, then transforms these spectrograms into actual audio. This combination allows for generating high-quality, human-like speech.
One of Tacotron 2's key features is its ability to create expressive and nuanced speech, which is especially useful when trying to achieve natural-sounding results in voice cloning. It can even learn how to pronounce words based on the surrounding context, which makes it stand out from traditional methods that might sound robotic at times. The entire system is open-source, making it accessible for people to experiment with building their own text-to-speech applications.
It's important to realize that while Tacotron 2 can generate amazing results, its performance is not always easy to quantify. Simple metrics like error values don't fully represent the quality of the generated voice. While Tacotron 2 can be customized with specific datasets, it can occasionally sound robotic at the beginning of audio snippets. The preparation of text for the model involves converting it into a set of symbols, such as characters or phonemes.
There are lots of guides and online resources for setting up and training Tacotron 2, even examples on Google Colab. Researchers are also continuously developing new vocoders, like ParallelWaveGAN, which can potentially be integrated with Tacotron 2 for producing audio. These developments continually improve the quality and possibilities for voice cloning, especially in niche applications like audiobook or podcast production. While the current outputs are impressive, there is still space for improvement, especially in handling challenging pronunciation cases, which presents ongoing challenges for this technology. It's interesting how the deep learning approaches like Tacotron 2 and WaveGlow have changed how we approach voice cloning and potentially how audiobooks and podcasts could evolve as a result.
7 Open-Source TTS Engines with APIs A 2024 Performance Analysis - TTS Arena Community-Driven Model Comparison Platform
TTS Arena is a platform built by the community, aiming to let people compare different text-to-speech (TTS) models side-by-side. It's simple: you input some text, and the platform lets you hear how different TTS engines pronounce it. This direct comparison helps users quickly grasp each model's strengths and weaknesses. The community plays a crucial role, as user feedback in the form of votes shapes the model rankings displayed on a leaderboard. It's inspired by a similar initiative for large language models (LLMs). This approach encourages community involvement and lets people discover which models are most suitable for specific tasks, whether it's producing audiobooks, podcasts, or experimenting with voice cloning.
Several well-known, open-source TTS engines are included, such as MaryTTS, Mimic, and Flite, each with its own strengths and weaknesses. The platform fosters a collaborative environment where users can share their insights and drive the development of open-source TTS. A model's presence on the leaderboard is determined by accumulating a certain number of votes, underscoring the importance of community feedback in shaping the future of TTS. While still in its early stages, TTS Arena represents a promising avenue for bringing together the TTS community and accelerating innovation through shared evaluation. The platform's success will rely on continued community engagement and the ability to handle the growing number of available TTS engines.
TTS Arena is an interesting platform built by the community for comparing different text-to-speech (TTS) models. Users can input text and hear how various models generate speech, allowing for side-by-side comparisons. The platform then ranks these models based on user votes, creating a kind of leaderboard of the most popular and well-regarded engines. This idea is inspired by Chatbot Arena, which focused on large language models.
The platform highlights seven open-source TTS engines, including established options like MaryTTS, Mimic, and Flite. MaryTTS offers a flexible framework, even including tools to create new voices from recordings—which could be quite useful for voice cloning in audiobook or podcast production. On the other hand, Flite is designed for low-resource scenarios, making it a good option for mobile or embedded systems that need fast and efficient speech synthesis.
The beauty of open-source engines is that users can modify and share the software freely. A good example is ParlerTTS, a fully open-source project where everything from the training data to the model itself is made publicly available for others to use and improve upon.
TTS Arena relies on user feedback to drive model selection and addition. It needs around 700 unique votes for a model to be added to the leaderboard. This encourages community engagement and gives users a direct say in what models are highlighted. When evaluating TTS models, aspects like overall speech quality and how well they suit various applications are considered important factors.
The platform’s potential is immense, especially for anyone working with sound production. The user-driven feedback and model comparison features can help content creators like audiobook producers or podcasters pinpoint a model that best suits the style and characteristics of their voices, content, or target audience.
TTS Arena's platform itself is designed for interoperability, meaning it can integrate with other tools or workflows. Developers can also use the platform to gain valuable insights into user preferences and identify areas where engine capabilities could be improved. It's a compelling concept for fostering an active community and helping the evolution of TTS engines for varied applications. The platform's focus on multilingual support could also be a powerful tool for creating more diverse and inclusive synthetic voices for a global audience in the future. However, it will be interesting to see how effectively TTS Arena manages the constant churn of newer models and manages the community's feedback and engagement over time.
7 Open-Source TTS Engines with APIs A 2024 Performance Analysis - Mimic Open-Source Text-to-Speech Engine Overview
Mimic, a collaborative project between Mycroft AI and VocaliD, is a streamlined, open-source text-to-speech engine built on Carnegie Mellon University's Flite software. The newest iteration, Mimic 3, emphasizes speed and user privacy, making it suitable for less powerful hardware like the Raspberry Pi 4. This version can produce speech in a wide range of languages, with over 25 supported and access to more than 100 pre-trained voices.
Mimic 3 incorporates advanced techniques like VITS (Conditional Variational Autoencoder with Adversarial Learning) to produce high-quality speech. Both Mimic and Mimic 3 allow flexible deployment—as plugins, web servers, or command-line tools—and can operate offline or in the cloud. This flexibility enhances privacy and reliability, making it a solid choice for users who value data control.
It's worth noting that, like other open-source projects, Mimic's strengths and weaknesses are continually evolving. It holds up well when compared to engines like MaryTTS or ESpeak, showing strong potential. Though Mimic has seen improvements in speed, even surpassing real-time generation in some instances, certain aspects of speech, like natural intonation and expressing various emotions, still present challenges. This is an area of active development in the field of TTS, and Mimic, being open-source, can benefit from community input and further improvements. Despite these ongoing developments, Mimic is a strong contender for diverse applications, including podcast and audiobook creation, along with any project that may require voice cloning or manipulation.
Mimic, a product of Mycroft AI and VocaliD, is an open-source text-to-speech (TTS) engine built on Carnegie Mellon University's Flite software. Its latest iteration, Mimic 3, is a neural TTS engine that prioritizes speed and user privacy. It's notably efficient, capable of running on less powerful devices like the Raspberry Pi 4, making it a versatile option for a range of applications. Mimic 3 boasts a significant voice library—over 100 pretrained voices—and supports speech generation in over 25 languages. Under the hood, Mimic 3 employs VITS, a technique known as "Conditional Variational Autoencoder with Adversarial Learning," which attempts to capture more of the nuances in human speech.
Both versions of Mimic are quite flexible, functioning as plugins, web servers, or through the command line, and offer offline and cloud-based modes for increased control over privacy and reliability. The open-source nature of Mimic means it's freely available for modification and distribution. When compared to other TTS systems, like MaryTTS and ESpeak, Mimic appears to be a strong contender, delivering performance and a greater degree of adaptability across diverse scenarios. Users can even contribute to the project by donating their voices to VocaliD's voicebank, which is intended to support individuals with speech impairments. Mimic 3, in particular, has impressed researchers with its ability to generate speech faster than real-time, making it a solid choice for applications that rely on fast, responsive AI/ML-driven audio generation for things like audiobooks, podcasts, and even potentially in voice cloning projects.
While Mimic's versatility and performance are appealing, there are still some considerations. Its reliance on neural networks, while enabling more natural-sounding speech, also means it can be sensitive to input variations, which can sometimes lead to inconsistencies. Additionally, the actual voice quality can vary between voice models, and depending on your use case, some models may be better suited than others. The specific customization of voice characteristics and dialects in the Mimic platform requires more exploration and evaluation to fully understand what limitations it might have compared to other TTS engines. It will be interesting to see how the future development of Mimic influences and advances the world of speech synthesis, especially as it pertains to voice cloning and its use in other audio production pipelines.
7 Open-Source TTS Engines with APIs A 2024 Performance Analysis - Performance Analysis of Input Data Quality Impact
The performance of open-source text-to-speech (TTS) systems is significantly influenced by the quality of the input data used during training and generation. The diversity and quality of training datasets directly impact the clarity, expressiveness, and emotional nuances of the synthesized speech. For tasks demanding high-quality audio, like producing audiobooks or podcasts, using robust and contextually rich input data is essential for achieving natural-sounding voices. Engines like Tacotron 2, with its deep learning approach, or MaryTTS, with its custom voice building capabilities, rely on high-quality input to perform optimally.
Within the expanding field of open-source TTS, improving the quality and variety of input data will be crucial for unlocking the true potential of these tools. The ability to adapt and refine voice models through exposure to diverse training datasets is not just about enhancing their versatility but also shaping the future trajectory of TTS technologies, including more refined voice cloning and captivating audio content. The future of realistic and emotionally nuanced voice synthesis depends heavily on the quality and diversity of the data powering these systems.
When it comes to generating realistic and expressive synthetic voices, the quality of the input data plays a pivotal role in shaping the final output. For instance, the subtle nuances of pitch, tone, and emotional delivery in synthesized speech are heavily influenced by the quality of the audio recordings used to train the TTS models. This is particularly crucial for applications like audiobook production and podcast creation, where natural-sounding voices are essential to engage the listener.
It's quite fascinating that some newer TTS engines, like Tacotron 2, can generate high-fidelity speech from relatively small datasets. This is a departure from traditional methods that typically require massive amounts of data, showcasing a shift towards more data-efficient approaches in the field of speech synthesis.
Furthermore, it seems counterintuitive but the presence of background noise in the training data can sometimes enhance the robustness of a TTS model. We've seen this in engines like Deepgram's Aura API, which demonstrate resilience in noisy environments. This adaptability to varying input conditions can prove incredibly valuable in real-world applications such as field recordings where capturing clean audio might be difficult.
Another interesting point is the importance of phonetic variation. TTS models that effectively account for the nuances of phonetics across different languages and dialects tend to produce more accurate and natural-sounding voices. This is especially vital for projects aimed at a global audience, where language diversity is essential.
When we look at models designed for real-time processing, like those in Deepgram's Aura or Mimic, the quality of input data becomes even more crucial. These systems, aiming for low latency, are sensitive to variations in the input, meaning a small change can lead to a noticeable difference in the generated speech. It's essential to ensure the input data is consistently high quality to maintain the consistency and quality of the audio output.
The ability of synthetic speech to convey emotion is heavily reliant on the quality of the input data. Training data that captures subtle emotional variations provides the TTS engine with a foundation for generating audio that feels more emotionally authentic and relatable.
However, the process of creating these datasets can be a bit complex, especially when it comes to the annotation of phonetic features. Incorrectly annotated data can easily lead to errors like mispronunciations and unnatural-sounding speech patterns, showcasing the importance of careful data preparation.
The open-source nature of some TTS engines has fostered a collaborative community where users contribute their own data, often enriching the variety and quality of the available input. This collaborative spirit leads to a more diverse range of voices, which is beneficial for tasks like voice cloning and personalized voice synthesis.
There's always a trade-off to consider between speed and quality when it comes to TTS engines intended for interactive applications. Real-time systems, while focused on quick output, need to maintain high audio quality. Input data quality is a key factor in achieving this balance, as even minor imperfections can be amplified in fast-paced applications.
Looking towards the future, the development of TTS technology seems to be moving towards adaptive learning systems capable of responding to real-time input. Engines like Mimic may evolve to handle more diverse and heterogeneous input data without sacrificing voice quality. This adaptability will be crucial for enhanced audio production in various tasks, ranging from audiobook narration to podcast production, highlighting a potential for higher-quality audio productions in the years to come.
Get amazing AI audio voiceovers made for long-form content such as podcasts, presentations and social media. (Get started for free)
More Posts from clonemyvoice.io: