Voice Pattern Recognition What Birds and AI Can Teach Us About Sound Processing
Voice Pattern Recognition What Birds and AI Can Teach Us About Sound Processing - Songbird Vocal Learning Mirrors Human Speech Development Through Neural Networks
The study of songbirds, particularly species like the zebra finch, offers a unique window into how complex sound production and processing can be. These birds, much like human infants, refine their vocalizations through a process of learning and auditory feedback. Their neural pathways demonstrate a structured sequence from hearing to vocalizing, echoing the developmental stages of human speech.
Scientists have identified how quickly these birds can adjust their vocalizations based on what they hear, which is analogous to how humans adjust their speech based on various cues. Their neural networks demonstrate a fascinating interplay of sensory input and motor output. This parallels the complex mechanisms of human speech production and provides a valuable model system for understanding the fundamental aspects of vocal learning.
Interestingly, the study of songbirds has relevance beyond basic biology. By understanding how their brains learn and refine sounds, researchers are gaining a greater understanding of neural networks and feedback systems involved in sound processing, which could influence advancements in voice technologies like audio book creation or podcasting, even voice cloning, perhaps leading to more sophisticated systems that better mimic and manipulate human voices.
The remarkable ability of certain songbirds to learn and produce complex vocalizations offers a compelling model for understanding human speech development. Species like the zebra finch, a common subject in research, demonstrate a learning process strikingly similar to human infants, where they acquire vocalizations by mimicking adults. This parallel extends to the neural networks involved, with brain regions dedicated to song learning in birds sharing functional similarities to those responsible for human speech production.
Interestingly, songbirds, like humans, have a sensitive period for vocal learning, a crucial window during which they readily acquire new vocalizations. This parallels the critical period for language acquisition in humans, underscoring the importance of early exposure for developing complex vocal abilities.
Further studies have shown that specific brain areas in songbirds, like the human auditory cortex, exhibit heightened activity during both vocal production and auditory processing. The complexity of a bird’s song appears to be linked to the size and interconnectivity of these brain regions. It's fascinating to consider the correlation between enhanced vocal repertoire and cognitive abilities.
While the ability to learn vocalizations is widespread in the animal kingdom, it's a rather rare trait, suggesting that the complex neural mechanisms that enable it are evolutionarily advantageous. This observation leaves us wondering about the selective pressures that led to this remarkable adaptation.
In a more practical realm, our understanding of songbird vocalizations has already influenced technological advancements. Tools for analyzing bird songs have led to improved machine learning algorithms and contribute to enhancing the efficacy of voice recognition software, including technologies useful for audiobook production.
Beyond communication itself, bird song also sheds light on social dynamics. Just as human speech reveals our emotions and relationships, specific bird calls can convey social information. We can gain insights into how communication functions as a vital tool for creating social bonds in diverse species.
Moreover, some bird species exhibit a unique ability to develop new vocalizations throughout their lives. This flexibility in sound production provides valuable lessons for artificial intelligence engineers seeking to improve the adaptability and sophistication of synthesized voices.
In recent years, there has been significant interest in applying the principles of avian vocal learning to AI. The potential for creating realistic voice clones capable of mirroring the subtle nuances of human intonation is increasingly being explored through neural network models based on bird song learning. The quest for accurate and expressive synthetic voices is one that can potentially benefit greatly from mimicking the processes of nature.
Voice Pattern Recognition What Birds and AI Can Teach Us About Sound Processing - Audio Pattern Recognition From Nature Applied to Digital Voice Clones
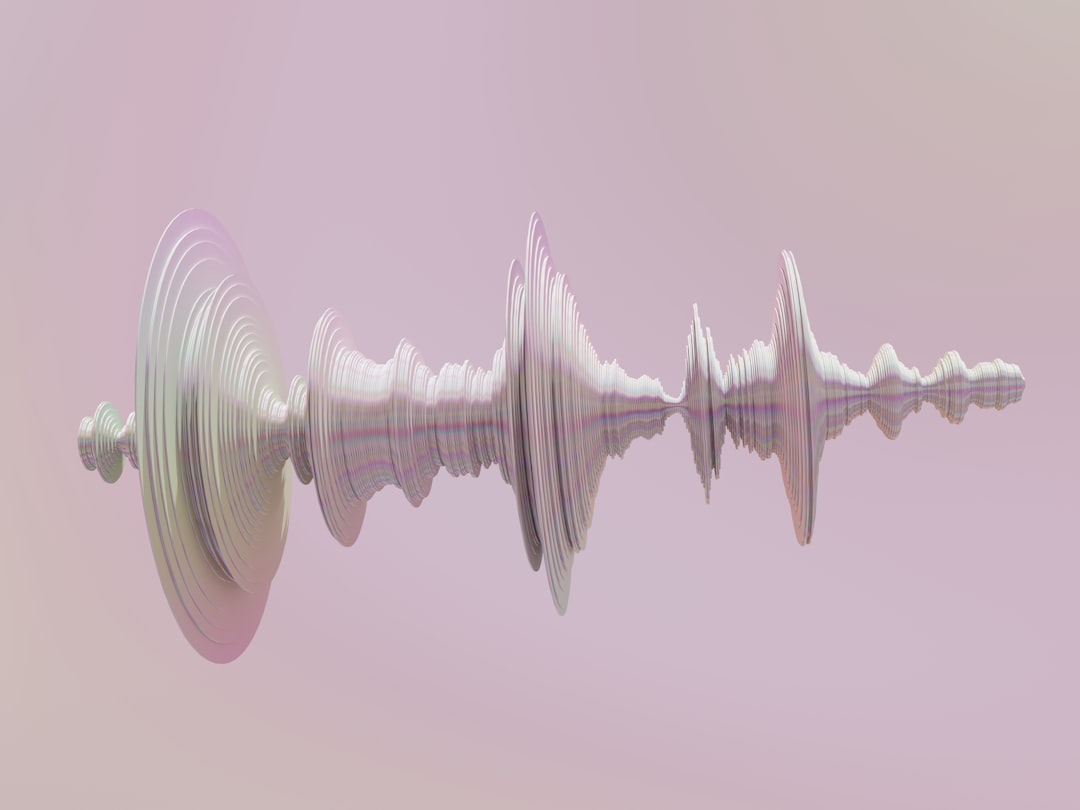
The field of audio pattern recognition is drawing inspiration from nature, particularly the intricate vocalizations of birds, to refine digital voice cloning and other audio technologies. Researchers are leveraging machine learning methods like Convolutional Neural Networks to analyze and classify sound patterns, mimicking how birds learn and replicate their calls. This biological insight has the potential to greatly enhance the capabilities of voice synthesis systems, enabling the creation of more expressive and nuanced synthetic voices. However, the complex nature of bird calls, including issues such as overlapping sounds and background noise, present significant challenges for current technologies, driving the need for further innovations. This exploration of sound production in nature has the potential to advance not only the creation of voice clones for applications like audiobooks and podcasts, but also to deepen our overall understanding of acoustic communication and its role in the natural world. The ability to more accurately mimic and control synthesized voices could have implications across several fields, including the enhancement of voice user interfaces and potentially even new forms of artistic expression. There's still much work to be done, but by studying the ways animals produce and interpret sounds, it’s possible that we can unlock the next generation of sound processing capabilities.
The way birds utilize distinct sound characteristics within their songs to convey different messages offers intriguing parallels to human speech. Just as we use variations in pitch and tone to express emotions, birds seem to employ similar acoustic cues. Understanding these subtle auditory features in bird songs could lead to the development of more expressive synthetic voices, capable of imbuing emotional nuances into their output.
Researchers have successfully translated certain songbird learning strategies into neural network architectures, particularly in relation to pattern recognition and sound mimicry. This exciting development has direct ramifications for voice cloning technologies, potentially enabling the creation of even more realistic and nuanced cloned voices.
The process of song learning in certain bird species appears to rely on a 'template matching' mechanism, where the bird constantly compares its vocal output to the songs it hears. This concept holds promise for creating adaptive algorithms in voice synthesis, paving the way for more natural-sounding outputs, and reducing some of the current limitations of robotic-sounding AI voices.
Some birds, like the ubiquitous mockingbird, have a remarkable ability to imitate a broad array of sounds, including human-made ones. This inherent versatility suggests that we could design voice cloning systems with a broader range of sonic capabilities, potentially allowing them to adapt more readily to user-specific voices and dialects.
Learning and memorizing songs in birds often involve a period of intense practice and continuous auditory feedback, similar to how humans refine their speech. This suggests that incorporating robust feedback loops within voice synthesis algorithms could significantly enhance their learning efficacy and perhaps make the process of generating cloned voices more efficient and less resource intensive.
The study of song learning also highlights the significance of social interaction among birds in facilitating the development of complex song structures. This underlines the potential value of incorporating collaborative learning mechanisms into AI voice models, which currently are limited in this aspect of the complex field of AI voice technology.
The neural pathways that underpin song production in birds undergo noticeable changes as the birds learn new songs, a phenomenon known as neuroplasticity. This could provide a valuable model for exploring how AI systems might one day be able to adapt and evolve their own vocal repertoires over time, leading to a potential paradigm shift in the field of vocal artificial intelligence.
Birds often adapt their calls based on their surrounding environment, showing an impressive ability to adjust their acoustic output based on context. This flexibility suggests a future where we might see dynamic voice cloning systems that are able to modify their output based on a given context or situation, leading to more believable and nuanced voice productions.
Intriguingly, certain bird species can learn and reproduce sounds even from distant, unrelated species. This demonstrates a potential for cross-species imitation, a finding that could inspire hybrid approaches to voice synthesis, effectively leading to a more diverse array of generated vocal outputs.
The development of regional dialects in species like the song sparrow showcases how localized cultural trends can influence sound production. This provides a potential pathway towards creating voice cloning technologies that can naturally replicate regional accents and slang, leading to a more realistic and authentic sound in synthetic voices. It is still uncertain, though, how much of these dialects are acquired behavior vs. genetic factors influencing sound production.
Voice Pattern Recognition What Birds and AI Can Teach Us About Sound Processing - Bird Syrinx Architecture Shapes Modern Voice Processing Models
The bird syrinx, a unique vocal organ unlike the human larynx, offers a compelling model for understanding and improving modern voice processing models. Its dual-sound source capability gives birds a level of control over sound production that's highly relevant to the goals of advanced voice technologies. The wide variety of syrinx structures across different bird species illustrates the value of adaptability and diversity in vocal design, which can inform the creation of more flexible and robust AI models for voice cloning and synthesis. Studying the evolutionary journey of this organ and its role in producing complex bird songs helps researchers identify innovative approaches to enhance the realism and expressiveness of synthetic voices, bridging the gap between natural and artificial sound production. The principles behind the syrinx's functioning can potentially inspire the development of more sophisticated voice processing systems that can improve the quality of audiobooks, podcasts, and voice cloning applications. While the challenges of replicating the complexity of biological sound production remain substantial, the syrinx provides valuable clues on how we might move toward more natural-sounding and versatile AI-driven voices.
The syrinx, a bird's unique vocal organ located at the base of the trachea, differs significantly from the larynx found in mammals. It's a fascinating structure that allows birds to produce incredibly complex and nuanced sounds, unlike any other animal. Interestingly, the oldest known syrinx dates back about 67 million years, coinciding with the emergence of modern bird groups, indicating a significant evolutionary leap. It seems birds evolved this new vocal organ rather than modifying their existing larynx, which is quite remarkable.
The syrinx shows incredible anatomical diversity across different bird species. Many aspects of its functionality and evolutionary trajectory remain a bit mysterious. However, researchers believe the common ancestor of modern birds likely possessed a syrinx with two sound sources, which could explain the capacity for complex vocalizations in today's birds. Studying the syrinx's development across various species, by examining gene expression in embryos, has shed some light on the evolutionary connections between birds and their ancient relatives.
Birds, thanks to the syrinx, can produce sounds in a fundamentally different way than mammals. They can even manipulate the length of their necks as resonators, enhancing the sounds produced by the syrinx, particularly in long-necked birds. Understanding the syrinx in living birds could potentially offer valuable clues about the vocalizations of extinct dinosaurs, given the close evolutionary relationship. The syrinx's evolution appears to be tightly linked with other major developments in birds, such as the evolution of flight and the complexity of their songs, underscoring its importance.
The ability of the syrinx to create simultaneous sounds on both sides, much like producing chords in music, provides a blueprint for advanced voice synthesis technologies. Imagine synthetic voices that can layer sounds in real-time to create a richer and more dynamic experience. The complex musculature surrounding the syrinx enables rapid modulation of pitch and volume, highlighting the possibility of designing more responsive and expressive synthetic speech.
Birds can generate a wide array of sounds with their syrinx, from harmonic tones to percussive noises, which expands the possibilities for bio-inspired voice synthesis technologies. Furthermore, each bird species' syrinx has a unique design, leading to different acoustic patterns used for communication. Understanding these differences could pave the way for creating AI models that generate synthetic voices with emotional nuances and intentions, making the voices more expressive and natural.
The ability of some bird species to imitate complex sounds, even from other species, highlights the potential for adaptive machine learning algorithms in voice cloning. These algorithms could allow digital voices to learn and adapt in response to various inputs. The syrinx's structure allows birds to adjust airflow and pressure based on their surroundings, which inspires the concept of voice cloning technologies that modify vocal output based on the situation, like a virtual voice actor that automatically changes its tone to match a scenario.
Unlike humans, who have a single source for sound production, birds can control the left and right sides of their syrinx independently. This bilateral control could potentially lead to the creation of more versatile and complex synthetic voices, capable of exploring a broader range of acoustic possibilities. Some birds even exhibit unexpected sound productions that are not typical of their species, pushing the boundary of their vocal abilities. Perhaps understanding these instances of 'vocal creativity' in birds can inform the design of AI systems that introduce a degree of creativity and adaptation into voice cloning techniques.
Research has shown that certain bird species can accurately replicate human-made sounds, like machinery and other animal calls. This ability could inspire the development of more versatile synthetic voices, especially in areas like podcasting, where capturing the essence of diverse sounds is critical. The process of song learning, through vocal play in young birds, reveals opportunities to incorporate experiential learning into AI models. This could lead to voice models that refine themselves based on user feedback and evolve to adapt to specific audiences, becoming a continuously self-improving tool.
While there is a lot we don't fully understand about the syrinx and the complex sounds it creates, the study of bird vocalization continues to be a rich source of inspiration for researchers working in voice technology. It demonstrates that nature often provides brilliant models for complex challenges in technology. The potential for future voice technologies, with their enhanced capabilities for nuance, expressiveness, and adaptability, is immense. It's a fascinating field with exciting possibilities on the horizon.
Voice Pattern Recognition What Birds and AI Can Teach Us About Sound Processing - Spectrogram Analysis Tools Bridge Animal and Digital Communication
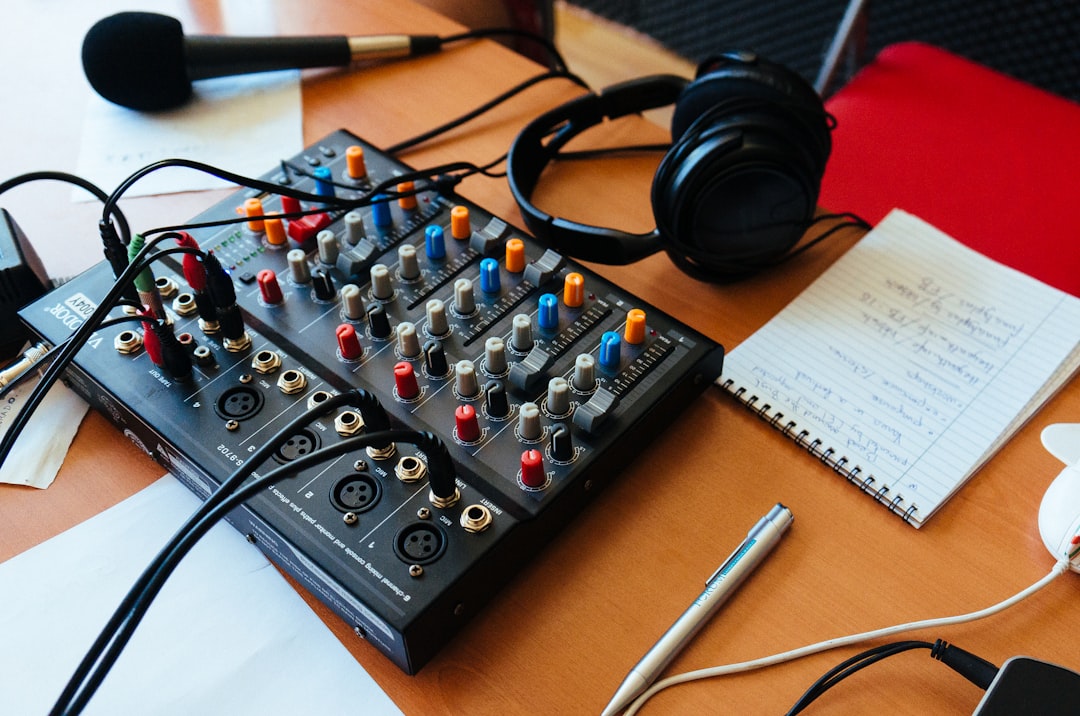
Spectrogram analysis, a tool that translates audio into visual representations, is fostering a new understanding of how animals communicate and how that knowledge can inform digital sound processing. By visually representing the complex sounds produced by creatures like birds, researchers can dissect the unique patterns of their vocalizations. This approach not only helps with species identification but also provides valuable data for developing better voice recognition technology. This could have a big impact on areas such as voice cloning, where creating more realistic and nuanced synthetic voices is a constant goal.
While spectrogram analysis offers a powerful tool for studying animal sounds, its application to digital voice synthesis still faces hurdles. The complexity of natural environments, with overlapping sounds and background noise, makes it challenging to isolate and analyze individual vocal patterns. This complexity necessitates ongoing development of more robust AI systems to further enhance the ability of machines to understand the subtle details of sound production. By overcoming these challenges, the potential exists to improve digital voice technology, from creating more realistic voice clones to designing more sophisticated audio production tools.
Spectrogram analysis, a technique for visualizing sound, has become a valuable bridge between animal communication and digital audio processing, particularly in understanding bird vocalizations. This approach allows researchers to identify and analyze intricate sound patterns in a way analogous to reading musical sheet music, with the horizontal axis representing the duration of sounds and the vertical axis representing pitch. While spectrogram analysis is a powerful tool, accurately classifying bird calls remains challenging. Overlapping sounds, background noise, and changes in sound intensity due to varying distances all create hurdles for both humans and algorithms.
Deep learning models, like Convolutional Neural Networks (CNNs), have shown promise in identifying bird species based on the unique patterns found in their vocalization spectrograms. However, these methods face challenges with imbalanced datasets. Innovative approaches are being employed to tackle this issue, improving the accuracy of automatic bird species identification through AI. Tools like OpenSoundscape utilize a blend of AI and traditional methods like time-of-arrival analysis from multiple microphones to enhance sound recognition in complex environments.
Furthermore, we find parallels between avian vocal learning and human speech development. Birds, like humans, have sensitive periods for learning vocalizations, and their neural pathways related to sound production undergo changes as they learn new calls. This neuroplasticity in birds suggests that AI voice models could adapt and develop their vocal repertoire over time, leading to more versatile and sophisticated synthetic voices.
Interestingly, some bird species, such as mockingbirds, demonstrate impressive cross-species mimicry, suggesting that AI systems could be designed to replicate a wider range of sounds. This ability is linked to the syrinx, a unique avian vocal organ that allows for complex sound production. The syrinx can produce two simultaneous sounds, similar to a musical chord, indicating that future AI voice models could potentially create a more rich and layered sound output.
The intricate details of songbird vocalization offer a biological model for improving the nuanced expressiveness of synthesized voices. Much like humans utilize pitch and tone to convey emotions, birds also use sound variations to signal intent and emotion, presenting an opportunity for AI developers to design synthetic voices capable of imbuing emotional contexts into their output. Moreover, the song learning process in birds heavily relies on feedback loops, much like humans fine-tune their speech based on auditory feedback. Applying this principle to AI-generated voices could lead to more naturally-sounding synthetic voices.
Further insights come from the observation of vocal play in young birds, which resembles the trial-and-error learning that's part of human language acquisition. This suggests that AI voice systems could be designed to undergo a process of continuous improvement based on user interactions, potentially leading to more adaptive and sophisticated voices. Additionally, the emergence of regional dialects in certain bird species indicates that it might be possible to create AI voice models capable of replicating authentic regional accents and speech patterns, adding a layer of naturalism and relatability to synthesized voices.
The remarkable adaptability of birds' calls to their environment suggests that future voice cloning applications could utilize adaptive algorithms to modify synthetic voices based on context. This could result in voice clones that shift their tone and mannerisms according to the situation, leading to a more sophisticated and natural-sounding experience. This includes creating a wider range of sounds, mimicking everything from harmonic tones to percussive sounds or even imitating human-made noises, thereby mirroring the versatility of natural vocal production. The syrinx's evolutionary journey, dating back over 67 million years, represents a rich potential source of bio-inspiration for refining how we think about sound processing and design the next generation of synthetic voice systems. The remarkable complexity of the syrinx may contain clues to unlocking new possibilities in the creation of artificial voices. While still a frontier, this interdisciplinary approach could bridge the gap between natural and artificial sound production, leading to more expressive and nuanced synthetic voices for applications such as audiobooks, podcasts, and even more realistic voice cloning.
Voice Pattern Recognition What Birds and AI Can Teach Us About Sound Processing - Neural Network Memory Banks Draw Insight From Avian Sound Libraries
Neural networks are increasingly leveraging the vast libraries of bird sounds to gain a deeper understanding of how sound patterns are processed. By studying the intricate vocalizations of birds, particularly songbirds, researchers are able to develop and refine AI systems that can more accurately recognize and categorize audio data. Projects like BirdNET utilize deep learning models to analyze spectrograms of bird calls, much like the techniques used in human speech recognition. This biological approach offers a new lens for developing more sophisticated voice cloning technologies and enhancing existing voice synthesis systems used in applications like audiobook creation or podcast production.
The fascinating parallels between how birds learn to sing and how humans learn to speak provide a rich source of inspiration for AI developers. The neural pathways involved in song learning share remarkable similarities with the brain regions responsible for human language acquisition. Studying the nuanced differences in bird song based on regions and species can lead to creating synthetic voices with richer expressive qualities, helping us generate more natural-sounding voices. The application of these insights holds the promise of creating AI-driven voice systems that are more adaptable and able to mimic the subtleties of human communication. However, the complexities of natural sounds, with their inherent background noise and overlapping sounds, present ongoing challenges for AI researchers. Despite these difficulties, the potential for advancement in voice technology through this bio-inspired approach is immense.
The intricate world of avian vocalizations offers a treasure trove of insights for improving digital voice technologies. Bird songs often display complex patterns, exceeding the complexity of many human musical compositions. Analyzing the harmonics and rhythms within these songs could reveal underlying structures with potential to guide improvements in digital sound synthesis. We see how different bird species adjust their calls to match the acoustics of their surroundings, demonstrating remarkable adaptability. This suggests a path towards designing more context-aware voice cloning systems that adapt their outputs based on the environment or situation, like a voice actor tailoring their performance to the scene.
The neural networks within songbirds show considerable plasticity as they learn new songs, their pathways constantly reshaping in response to experience. This characteristic has striking parallels to how future AI voice technologies could potentially evolve and improve their vocal abilities over time. The avian syrinx, a unique vocal organ, demonstrates the capability to produce sounds simultaneously from both sides, much like layering different musical notes or chords. Leveraging this principle could guide the development of new voice synthesis technologies that generate richer, more layered and dynamic audio outputs.
Birds often learn their songs through social interaction, much like humans acquire language skills through interaction and collaboration. This hints that integrating social learning mechanisms into AI voice models might unlock a new level of natural-sounding speech with a wider range of emotional expressiveness. We know that young birds perfect their vocalizations through iterative attempts and feedback, a process reminiscent of human speech refinement. Incorporating such feedback loops into voice synthesis algorithms could help create more realistic, adaptable, and naturally sounding synthetic voices.
Further, some birds, including the familiar mockingbird, exhibit a remarkable ability to mimic a vast array of sounds, even those created by human-made machines. This incredible versatility suggests that future voice cloning technology could broaden its repertoire to encompass a broader range of human-like and environmental sounds, including the more subtle details.
Certain bird species have developed regional variations in their songs, akin to human dialects and accents. This observation offers a fascinating possibility that AI-based voice cloning technologies could be enhanced to capture authentic regional accents and speech patterns, making the resulting voices more realistic and relatable. Similarly, the ability of some birds to mimic sounds of species unrelated to their own suggests a possible path for future AI voice cloning models to blend different vocal characteristics, resulting in a wider variety of synthetic voices.
Parrot species, for example, have been observed using a template-matching mechanism to learn new sounds, comparing their own vocalizations against those they hear. This provides a model for future voice synthesis algorithms. By incorporating such methods, we may be able to create more adaptive, context-aware, and naturally sounding voice clones. While there's a long road ahead, studying the intricacies of avian sound production and vocal learning provides fertile ground for exploring new approaches that could revolutionize the field of digital voice technology.
Voice Pattern Recognition What Birds and AI Can Teach Us About Sound Processing - Deep Learning Models Extract Audio Features Common to Birds and Humans
Deep learning has emerged as a powerful tool for analyzing audio, revealing shared characteristics in the sound production of both birds and humans. Models like ResNet50 and InceptionV3 have proven particularly adept at extracting and identifying these features, surpassing traditional methods in their ability to handle complex sound patterns. These models demonstrate a clear advantage when trained on extensive datasets, allowing them to accurately distinguish between different bird calls. This capability provides intriguing insights into the underlying mechanisms of human voice production as well.
This newfound understanding of shared audio features has applications beyond scientific curiosity. It can support environmental monitoring and conservation efforts by facilitating the automatic detection and identification of bird species. Furthermore, the ability of deep learning models to effectively process complex bird vocalizations has direct implications for voice technologies like voice cloning and podcasting. By mimicking the way birds learn and refine their vocalizations, developers could potentially build more lifelike and expressive synthetic voices, further narrowing the distinction between natural and artificial sound production. However, these deep learning models can sometimes require a lot of data to perform well, highlighting a limitation in their practical applications.
Deep learning models, particularly those based on architectures like ResNet50 and EfficientNet, have proven adept at extracting and recognizing audio signals from a variety of bird species. This approach is increasingly replacing traditional audio processing methods, automating the process of feature extraction and data preparation without the need for extensive manual intervention. Techniques like the Fourier Transform and Mel-Frequency Cepstral Coefficients (MFCCs), which were previously staples in bird sound analysis, are now often integrated into these deep learning pipelines.
While deep learning offers substantial advantages over older methods like linear regression in deciphering complex, unstructured audio data, they rely heavily on a large volume of unique audio samples to achieve accurate results. This creates a significant hurdle for research focused on endangered species, where large datasets are often unavailable. It’s a trade-off—gaining accuracy often comes at the cost of needing massive quantities of unique sounds.
The ability to automatically detect bird species based on their vocalizations holds immense promise for ecological studies and conservation efforts. Researchers can leverage this technology to monitor populations and assess the health of ecosystems in real-time across large geographical areas, allowing them to quickly and efficiently track changes in bird populations. Similar principles are already being employed in many virtual assistants like Alexa and Google Home, where deep learning algorithms analyze audio data for tasks like automatic speech recognition and audio classification.
Interestingly, deep learning has also proven effective at identifying and classifying bird calls, an asset to conservation efforts, especially when it comes to threatened species. But, the availability of sufficient, varied audio samples remains a considerable challenge, especially for endangered or geographically isolated populations. This scarcity of data can hinder model performance and limit the effectiveness of the technology.
As deep learning continues to evolve, its applications within audio analysis are expanding. It’s quite likely that future innovations will lead to refined methods for tasks like audio tagging and potentially even audio generation. We’re still early in the exploration of these applications, but there’s a strong indication that this research may produce important tools for understanding and influencing the sonic world. The ability to create realistic and nuanced synthetic voices holds promise for improvements in audio book production, podcasting, and potentially even voice cloning, but those developments are still some time away. The work in bird vocalizations is giving us many insights into how to more effectively develop some of these technologies. We are at the foothills of this exploration, but the potential impact on both ecological research and human-centered applications of voice technology could be significant.
More Posts from clonemyvoice.io: