Get amazing AI audio voiceovers made for long-form content such as podcasts, presentations and social media. (Get started for free)
Leveraging Platform Engineering to Streamline Audio Production Workflows
Leveraging Platform Engineering to Streamline Audio Production Workflows - AI-driven Voice Cloning Accelerates Audiobook Production
The rise of AI-powered voice cloning is altering how audiobooks are produced, aiming for faster workflows and increased output. Efforts such as Audible's experimental program allow narrators to train AI on their voices, potentially speeding up audiobook recordings. This technology, while holding promise for greater audiobook availability and potentially lower production costs, introduces ethical concerns. Concerns arise about the potential for misuse of cloned voices without proper consent. As AI voice cloning matures, it could fundamentally change how listeners perceive audiobooks. Audiences may need to adapt to the possibility of AI-generated narration, which might challenge traditional notions of authenticity within the audiobook format. As the industry adapts, carefully managing the advantages of automation with the need for both ethical protocols and robust security measures will be vital.
The emergence of AI-driven voice cloning is revolutionizing how audiobooks are produced. Systems like the one being explored by Audible are allowing narrators to essentially create digital twins of their voices, significantly accelerating the process. It's fascinating how these models capture not just the basic sound of a voice but also aspects like pitch, inflection, and emotional nuances. This means audiobooks can be produced with remarkable authenticity, mirroring the author's or narrator's voice very closely.
Traditionally, audiobook production was a time-consuming process, potentially stretching out for weeks or even months. With AI voice cloning, the production timeline can be compressed drastically. An entire audiobook could theoretically be completed in a matter of hours, which has huge implications for getting new titles out to listeners faster. Research suggests that humans often find it surprisingly difficult to discern a cloned voice from a real one, particularly when the AI model has been trained extensively on a specific voice. This accuracy and the potential for wide-scale audio application are very interesting from a research perspective.
There's also a humanitarian aspect to consider. Authors facing physical limitations or difficulties with traditional narration now have a more accessible path to producing their audiobooks. The potential for authors to create customized versions of their own voices is another intriguing possibility. These custom AI voice models, trained on specific speaking patterns, can allow an author's voice to be consistently reproduced in a personalized way.
This is not just limited to one-off productions. AI allows for dynamic adaptation of content. Updates or corrections can be seamlessly incorporated without requiring a complete re-recording, which could lead to a very efficient workflow. The accessibility of these tools is also worth exploring. As they become easier to use, we could potentially see a surge in individuals creating audiobooks. However, a flood of new audiobooks raises questions about quality control and the overall listening experience.
The ability of AI to adapt the voice across multiple languages is a game-changer for global reach. This can potentially open up audiobooks to a massive, diverse audience without compromising the voice quality or the original speaker's characteristics. Additionally, features such as dynamic adjustment of speech rate and emotional tone offer further customization for individual listeners.
However, the exciting technological progress is accompanied by some very real ethical concerns. The ability to recreate someone's voice with such accuracy brings up important questions about consent and potential misuse. We are still in the early stages of understanding these issues, but it's vital that the development of AI voice cloning be coupled with strong safeguards and regulations to prevent unauthorized or malicious use. We need mechanisms to ensure responsible deployment and protect against potentially damaging consequences.
Leveraging Platform Engineering to Streamline Audio Production Workflows - Automated Noise Reduction Techniques in Podcast Recording
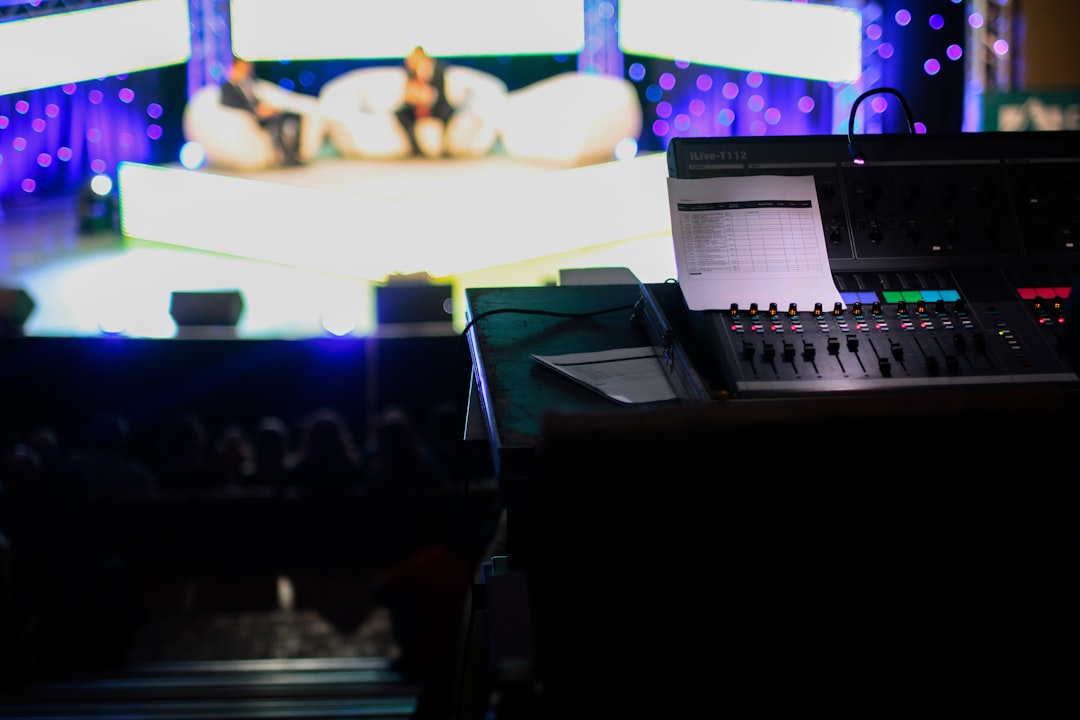
Automated noise reduction is becoming a vital part of creating high-quality podcast audio. These AI-powered tools are designed to identify and remove unwanted background sounds, like traffic noise or room echoes, enhancing the listening experience by ensuring the focus stays on the speaker's voice. The ability of these tools to automatically clean up audio can significantly speed up the post-production process.
However, relying solely on software isn't always the answer. Podcasters need to consider the limits of automated noise reduction and strike a balance between the tools' capabilities and the fundamentals of good sound. It's easy to get caught up in the promise of perfect audio with the push of a button, but achieving truly optimal results often involves a blend of skilled recording techniques and the intelligent use of these tools.
The field of automated audio production continues to develop rapidly. While it offers exciting possibilities for creators, it also demands a thoughtful approach to ensuring that the final audio product is not only free of noise but also maintains the integrity and natural quality of the recorded sound.
In the realm of audio production, particularly for podcasts and audiobooks, the human ear's ability to distinguish between natural and AI-generated speech is being challenged. Research shows that even as AI models refine their abilities, people often have difficulty spotting synthetic speech. This raises questions about what we consider authentic audio, especially in formats where the human voice plays a central role.
It's well-established that even minor background noise can drastically interfere with the clarity of spoken content. The good news is that automated noise reduction techniques are gaining traction, employing algorithms to separate the voice frequencies from disruptive sounds. These tools aim to preserve the overall audio quality while enhancing the listener's experience.
Many of these advanced systems employ a technique called adaptive filtering. This allows them to adjust in real-time as the surrounding sounds change. This adaptive nature is important for recordings made in unpredictable environments, like busy cafes or outdoor spaces.
Machine learning is another critical component in automated noise reduction. Algorithms are trained on large quantities of audio data, learning to recognize and suppress specific noise patterns, like traffic noise or the hum of air conditioning. These trained models are essentially becoming experts at separating unwanted sounds from the target audio.
Interestingly, a process called spectral subtraction is used in some of these systems. It involves analyzing the frequency profiles of the voice and the background noise and then effectively subtracting the noise components. This can be very effective in improving voice clarity without sacrificing the natural qualities of the voice.
Another fascinating development is the incorporation of perceptual models. These models aim to replicate how the human auditory system processes sound. This involves emphasizing or suppressing frequencies based on their significance for speech understanding. The idea is to make the sound output more natural and understandable by targeting the way the human ear perceives audio.
Our brains are quite good at filtering out unwanted noise when we are listening to conversations. This ability varies, of course, from one person to another. However, the goal of automated noise reduction systems is to replicate this human process. They seek to deliver a more consistent, clearer auditory experience, regardless of the listener's own noise filtering abilities.
One potential drawback with these systems is a phenomenon called latency. This refers to a slight delay introduced during the audio processing. Though the delay can be a challenge for live podcast recordings, researchers are constantly finding ways to minimize it.
Besides directly improving the quality of audio, automated noise reduction can be beneficial for AI voice cloning projects. The better the quality of the training audio, the more accurate and nuanced the generated voice replicas will be.
Finally, there's a constant need to consider trade-offs when using these tools. Overly aggressive noise reduction can produce undesirable sonic artifacts, causing the voice to sound artificial or even robotic. Finding the right balance is key to ensuring that the audio output is enhanced rather than distorted.
Leveraging Platform Engineering to Streamline Audio Production Workflows - Cloud-Based Collaboration Tools for Remote Audio Teams
Remote audio production, whether for audiobooks, podcasts, or voice cloning projects, increasingly relies on cloud-based collaboration tools to keep teams connected and workflows streamlined. Platforms like Google Workspace and Microsoft 365 offer a centralized hub for communication and file sharing, which is crucial for coordinating diverse aspects of audio production. These integrated suites help manage projects, share feedback, and facilitate seamless collaboration among team members who might be geographically dispersed.
Tools like Trello or ClickUp can be incredibly valuable for visualizing projects and keeping track of tasks, offering a clear picture of progress to everyone involved. This visual clarity is especially important in complex audio projects, where many different steps need to be coordinated. Additionally, platforms focusing on secure file storage, such as Box or LucidLink, become essential for managing large audio files, especially in voice cloning or audiobook production where maintaining the integrity of audio is vital. These platforms ensure that everyone on the team can access the necessary files safely and easily, regardless of location.
The ongoing development of these cloud-based solutions continues to reshape the audio production landscape, pushing the boundaries of both productivity and creative collaboration. While these tools can boost efficiency and coordination, it's crucial to recognize that they also raise new expectations for communication and creative exchange within remote teams.
Cloud-based tools are transforming how remote audio teams collaborate, especially within fields like podcasting, audiobook production, and voice cloning. Platforms like Google Workspace and Microsoft 365 offer familiar interfaces for communication and file sharing, streamlining the workflow for geographically dispersed teams. The real-time editing capabilities of these tools enable simultaneous collaboration on audio projects, significantly reducing the time spent on back-and-forth communication. This dynamic approach allows for a more fluid creative process compared to older methods of audio production.
One of the key benefits of cloud storage for audio is version control. Each revision of an audio file, which is crucial in voice cloning projects as AI models are fine-tuned, is readily available, easily tracked, and if necessary, the older versions can be accessed. This feature helps ensure that the most polished version of a cloned voice or any audio asset is always readily available.
Furthermore, some cloud platforms integrate advanced machine learning algorithms that automatically analyze and refine audio quality. These systems learn from audio editing best practices, constantly improving their ability to clean and enhance the final product. The continuous evolution of these AI-powered audio features means tools can tailor themselves to specific audio production needs, improving their effectiveness over time.
The global nature of audio production is also changing thanks to cloud-based tools. They break down geographical barriers, allowing teams to collaborate with specialists across the globe. Audio engineers in various time zones or voice actors with unique accents become more accessible, leading to diverse audio experiences and content. This distributed workforce model can be a boon to audio projects needing specific skills.
Cloud platforms can flexibly adjust processing power to handle diverse projects. For example, rendering complex audio projects, particularly those involving multiple AI voice clones, can be handled efficiently with cloud processing resources, freeing local workstations from overwhelming computational burdens.
Cloud tools also offer integrated analytical features, allowing teams to investigate audience engagement and listening patterns. The gathered insights can be used to tailor audio products to specific audiences, enhancing the overall listener experience and creating a feedback loop for ongoing refinement.
The move to the cloud also facilitates features like text-to-speech, opening up audio content to those who benefit from auditory learning or have visual impairments. It makes audio consumption and creation more accessible.
Within the cloud ecosystem, feedback loops can be rapidly established within a team. This allows for quick iterations and refinements, ensuring that the final product aligns with the project goals and artistic vision.
A crucial aspect, especially in voice cloning, is security. Many cloud platforms employ robust encryption and security measures to safeguard voice data and intellectual property during the collaborative production process. This security is vital, given the sensitivity of voice data and the potential misuse of AI-cloned voices.
Finally, the flexibility of the cloud allows audio tools to integrate with emerging technologies like augmented reality and virtual reality. This adaptability ensures that cloud-based audio workflows remain relevant and applicable across future technological innovations within the sound production field.
However, we need to remain cautious and consider the potential downsides. The overreliance on automated features might lead to the loss of certain aspects of human creativity and nuance in audio production. It’s critical to balance the advancements with the value of a human touch in the creative process. Finding that balance is a significant challenge that needs to be explored as the technologies advance.
Leveraging Platform Engineering to Streamline Audio Production Workflows - Machine Learning Algorithms Enhance Audio Quality Control
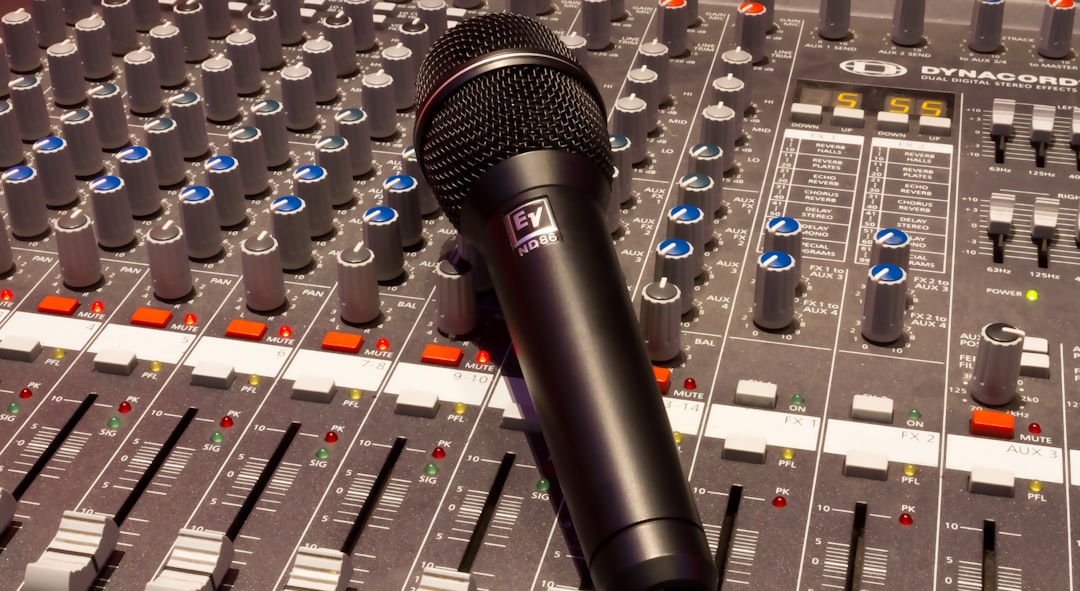
Machine learning algorithms are increasingly pivotal in refining audio quality during production, especially for projects like audiobooks and podcasts. These algorithms can analyze audio, identify and remove unwanted sounds like background noise or echoes, and apply intelligent adjustments to equalization and other parameters to create a balanced, clear sound. Essentially, they act as sophisticated audio engineers, performing tasks such as noise reduction and fine-tuning audio characteristics to a degree not previously possible. Additionally, these algorithms can learn to better predict how humans perceive sound quality based on specific audio traits, which in turn improves the overall listening experience. The potential is enormous, but it is vital to understand that excessive automation may lead to a loss of some of the authentic, subtle qualities that make recorded audio engaging. Striking a balance between the efficiency of these tools and the creativity and nuance of human input is key to shaping a future where machine learning and human artistry complement each other in creating truly compelling sound.
Machine learning algorithms are increasingly influencing how we manage and improve audio quality, especially in areas like voice cloning, audiobook production, and podcasting. Here's a look at some of the ways these algorithms are being applied:
Firstly, the ability to process audio in real-time is a significant step forward. Imagine recording a podcast in a noisy cafe—machine learning can now clean up the audio as you're recording, removing distracting background sounds without the need for extensive post-production. This real-time processing is valuable for a smooth recording workflow.
Secondly, there's a greater level of detail available when it comes to frequency separation. It's not just about noise removal, but about understanding the nuances of the different sounds in the audio recording. Algorithms can now dissect the audio into frequencies, allowing a more specific and accurate approach to cleaning up recordings. This meticulous approach to frequency analysis results in higher quality audio.
Third, these systems aren't static. They are designed to learn and improve over time. The more they are used in diverse acoustic environments, the better they get at recognizing and isolating specific noises. This adaptability is vital for applications like podcasts, which are often recorded in unpredictable spaces.
Furthermore, algorithms are now being used to optimize the dynamic range of audio. Audiobooks and podcasts, which often span many hours of audio, benefit from consistent volume and clarity. Machine learning can prevent sudden jumps in volume, resulting in a more comfortable listening experience.
Spectral analysis, where the audio is broken down into its component frequencies, allows for fine-grained adjustments. Engineers can identify and target problematic frequencies where noise resides, carefully tweaking them to improve the audio without sacrificing the integrity of the voice.
One interesting trend is the development of noise reduction algorithms that consider how humans perceive sound. The algorithms essentially try to mimic our auditory system, emphasizing or de-emphasizing certain frequencies. The goal is to make the sound feel more natural and easier to understand.
It's also worth acknowledging that these algorithms are constantly improving. They are now being designed to minimize unintended artifacts that sometimes arise during noise reduction, which can cause the audio to sound unnatural. Minimizing these artifacts leads to clearer and more authentic recordings.
Noise profiles are unique to every environment. Algorithms can now be trained on a variety of noise sources, like the hum of air conditioning or traffic noise, making them more versatile tools for different recording situations. This adaptability is a critical element for audio engineers working in diverse locations.
Some algorithms are moving towards a predictive model of editing. They learn common patterns in audio recordings, enabling them to suggest or even automatically perform edits, thereby potentially accelerating the post-production process for things like audiobooks or podcast episodes.
In the context of voice cloning, machine learning algorithms have advanced considerably. Not only are they analyzing the pitch and tone, but also things like subtle breathiness and emotional expression. This has led to incredibly realistic voice recreations, making it increasingly hard to discern a cloned voice from a human voice.
These advancements in machine learning are transforming the way we think about audio quality control, particularly within sound production workflows that are increasingly dependent on voice-based content. The future potential of machine learning algorithms in audio production appears to be substantial, promising to significantly enhance workflows and overall quality.
Leveraging Platform Engineering to Streamline Audio Production Workflows - Containerization Streamlines Audio Processing Workflows
Containerization is proving to be a powerful tool for simplifying audio processing workflows, especially in fields like audiobook creation, podcasting, and the increasingly prevalent area of voice cloning. By packaging applications and their dependencies into self-contained units (containers), developers can create consistent environments that make deploying and running audio processing tools much easier across different platforms. This simplifies the deployment process, making it quicker to get tools into production and reducing the chance of encountering platform-specific issues.
This consistent approach also has benefits for managing the intricate audio delivery infrastructure that many audio projects require. Teams no longer have to spend as much time dealing with operational headaches, freeing them to focus on the creative aspects of their work. The increasing adoption of open-source tools, like Klio, within audio processing workflows is a significant positive development. Klio specifically focuses on improving data pipeline management for large audio projects, enhancing efficiency and making it easier to manage and process a huge amount of data. This rapid iteration capability is crucial in a constantly evolving environment where audio projects often involve rapid prototyping and frequent adjustments.
The growing adoption of containerization within the audio industry signifies a broader trend – a deliberate shift towards more efficient, scalable, and streamlined production practices. It indicates a growing appreciation for the value of improved collaboration and a greater capacity for innovation within the world of audio production.
Containerization is reshaping how we approach audio processing workflows, particularly within the rapidly evolving world of AI-driven voice cloning and audiobook production. It offers a way to package up audio processing tools and their dependencies, creating portable and reproducible environments that can be deployed across various systems—from personal computers to cloud infrastructure. This means that audio processing tasks can be streamlined, making the overall production workflow more efficient.
Think about how platforms like Audio Weaver are being used to simplify the implementation of audio algorithms, whether they're custom-built or from external sources. This ability to easily incorporate new processing capabilities into existing workflows is a clear advantage of containerization, particularly when dealing with a dynamic field like voice cloning.
Tools like Portainer can make managing audio infrastructure much easier. By simplifying the management of containerized environments, it allows audio engineers to focus less on the complexities of infrastructure and more on their creative work. It’s becoming increasingly clear that the ability to quickly adapt to new technologies and workflow demands will be crucial for success in audio production.
Projects like Klio, an open-source framework based on Python and Apache Beam, show how containerization can improve the efficiency of working with large audio libraries. Imagine how that could be applied to audio book production, where there are massive collections of voice recordings. By efficiently processing this vast amount of data, containerization can make the entire production process smoother and faster.
The shift towards cloud-based and virtualized solutions in the audio industry offers both flexibility and scalability for various production workflows. For instance, think about the potential for remote audiobook recording, with a narrator in one location and the production team elsewhere, all working together seamlessly through the cloud. This shift to virtualized workflows challenges traditional methods of recording.
When it comes to voice cloning specifically, tools that enable real-time collaboration between artists and engineers are gaining traction. The use of AI-assisted software allows artists to express their ideas in a way that can be quickly translated into audio by engineers. The ability to easily iterate and refine these voice clones is another benefit of containerized workflows.
The DevOps movement has embraced containerization for its ability to speed up deployment processes while enhancing the reliability and availability of applications within development cycles. Similarly, in audio production, containerization allows for quicker testing and implementation of new audio processing features or techniques.
This concept of portability is becoming ever more important for audio processing. Containerization allows developers and engineers to move their audio processing tools between diverse environments—whether it's a local machine for testing, a development environment, or ultimately into a cloud deployment for production.
The growth of cloud processing for audio is quite interesting. It’s allowing for more robust and scalable audio production, paving the way for more innovative and efficient sound production.
Leveraging these cloud-based systems and frameworks, like Klio, teams can manage and process vast amounts of audio data more effectively. This is especially beneficial for large-scale audiobook projects or projects involving extensive voice cloning. The ability to process audio quickly and easily will allow audio production to become even more accessible.
There's no doubt that this field is evolving fast, and as it does, the ability to use containerization will be increasingly important. Whether it's the creation of voice clones, editing a podcast, or the production of audiobooks, these approaches will likely play a crucial role in how sound is created, managed and delivered in the future. The careful balance of the efficiency these tools offer with maintaining the artistry of sound is a ongoing challenge to watch in the years ahead.
Leveraging Platform Engineering to Streamline Audio Production Workflows - Real-Time Voice Synthesis Integration in Production Pipelines
Integrating real-time voice synthesis into audio production pipelines has the potential to dramatically improve efficiency, particularly for applications like audiobooks, podcasts, and the creation of voice clones. The automation of repetitive voiceover tasks can significantly speed up workflows, reducing the time and cost associated with traditional recording processes. Platform engineering can play a vital role in this transition by enabling better management and scaling of audio production systems, allowing for smoother integration of new tools like voice synthesis software. This blending of technology and creative workflows opens up new opportunities for accessibility and allows for more dynamic audio content. However, it's important to acknowledge the potential drawbacks. We must carefully consider the role of human creativity and ingenuity as we rely more on automated tools. Additionally, the ethical implications of voice cloning and synthesis need to be addressed proactively, with a focus on responsible development and deployment. As the technology evolves, navigating the interplay between automation, quality assurance, and ethical practices will be crucial in shaping a future where voice synthesis enhances, rather than diminishes, the art of audio production.
Real-time voice synthesis has the potential to drastically accelerate audio production by handling tasks like noise filtering, effect application, and pitch/modulation adjustments in the moment. It's like having a real-time audio engineer within the recording software, allowing for immediate feedback during sessions. This can be a massive time-saver for podcasters, audiobook producers, and anyone working with voice cloning.
The ability to generate audio in multiple languages with regional accents opens a whole new world of possibilities for expanding content reach. Think of creating an audiobook or podcast that naturally adapts to different dialects or languages—that's something real-time voice synthesis is making a reality. It's quite fascinating how the technology adapts to the intricacies of different spoken languages.
Beyond just words, modern voice synthesis is capable of mimicking the emotional context of speech. Narrators' subtle vocal cues, like happiness, sadness, or excitement, can be integrated into the cloned voice. It's remarkable how these systems capture those nuanced aspects of human communication. This capability can significantly increase listener engagement, making audio content feel more authentic and compelling.
We can manipulate aspects like the speed and pitch of synthesized speech in real-time. This means we can tailor the audio output to match specific content types or audience preferences. For instance, we could potentially create audio that automatically adjusts its pace for those who prefer faster or slower narration speeds. It's amazing that we can tweak audio delivery to fit specific needs in real-time.
It's quite challenging to record audio in unpredictable environments, and that's where real-time synthesis shows its strength. These systems are designed to adapt to changing sound environments by dynamically adjusting synthesis parameters. Whether it's a bustling street or a quiet studio, the system can try to adjust, ensuring audio quality isn't overly impacted by extraneous noises. The adaptability of these systems is pretty impressive.
Some platforms have incorporated user interfaces that allow for hands-on manipulation of vocal traits during recording. It's like having a soundboard specifically for manipulating the voice itself. This approach brings a human element to the process, giving audio artists a greater degree of control over their output. This interactive capability can enhance the artistic aspect of audio production.
The ability to analyze listener data and adapt voice outputs in real-time creates a fascinating feedback loop. This means the voice could potentially adapt to what listeners respond to most positively. The potential for dynamically adjusting the voice based on feedback and preferences is interesting. It also begs the question of how far this could go in the future.
Managing latency, or the delay between the input and output, is a major technical challenge. However, recent advancements in algorithms have improved latency management, leading to a smoother recording experience. Minimizing latency is critical for real-time applications, and it seems the technologies are improving at a rapid pace in this area.
We can also integrate voice synthesis and recognition systems to create a feedback loop. Essentially, the synthesized voice is constantly being compared to the original voice to make sure the cloned version is as accurate as possible. This constant comparison can help improve voice cloning accuracy, which is pretty useful for audio projects using this technology.
One of the most recent innovations in voice synthesis is the ability to mimic non-verbal cues. This includes things like breaths, pauses, and even laughter. This kind of intricate replication of natural speech patterns helps to bridge the divide between synthesized speech and natural human voice. As this technology progresses, we may see a point where it becomes incredibly difficult for humans to differentiate between synthesized and human speech. It's an interesting space to watch as the research continues in this direction.
Get amazing AI audio voiceovers made for long-form content such as podcasts, presentations and social media. (Get started for free)
More Posts from clonemyvoice.io: