Irish AI Governance Standards 7 Key Voice Technology Implementation Guidelines for Content Creators
Irish AI Governance Standards 7 Key Voice Technology Implementation Guidelines for Content Creators - Mandatory Voice Sample Documentation Requirements Under Dublin Audio AI Framework
The Dublin Audio AI Framework's introduction of mandatory voice sample documentation marks a crucial step towards establishing ethical guidelines in the rapidly evolving world of voice technology. This framework, specifically targeting areas like audiobook production, podcasting, and the burgeoning field of voice cloning, aims to bring much-needed transparency and accountability to AI-driven audio content creation. By requiring thorough documentation of all voice samples used in AI systems, the framework seeks to ensure compliance with privacy and data protection regulations. This is especially relevant as these technologies become more accessible and creators increasingly integrate AI tools into their workflows.
However, the question remains whether these requirements effectively navigate the ethical complexities that voice technology introduces. While promoting responsible content creation, it's vital that the framework itself doesn't stifle innovation or become overly burdensome. The implementation of these guidelines will be a crucial test in the ongoing effort to balance the advancement of AI with the preservation of individual rights and societal values in the realm of audio creation.
The Dublin Audio AI Framework imposes a set of detailed specifications for voice samples used in AI voice applications. These specifications, including minimum recording duration and a range of emotional expressions, are designed to ensure the generated voice models achieve a certain level of accuracy. It's crucial for creators to be aware of these technical prerequisites before starting any voice data collection process.
Maintaining a controlled acoustic environment during voice sample recordings is another core element of the Framework. By minimizing background noise, the quality of the recordings improves significantly, which is critical for meeting regulatory requirements. It's not just about the audio, either. The documentation process mandates detailed metadata about the recording environment, such as temperature and humidity. This, it seems, has a direct link to the subtleties of voice cloning technology. I find that rather intriguing.
A noteworthy divergence from common practice is the explicit consent requirement. The Framework explicitly mandates that all individuals providing voice samples grant informed consent, ensuring adherence to ethical principles and safeguarding individual rights within the realm of voice cloning.
The Framework's objective isn't simply to create generic voice models. It actively promotes the use of voice samples from diverse dialectal and accentual backgrounds. This initiative aims to build voice models that encapsulate the rich tapestry of cultural and linguistic variety, reflecting a broader view of audio production.
Interestingly, the Dublin guidelines mandate regular audits of voice sample databases. This ensures the long-term quality of the voice data. It also helps prevent the use of outdated or subpar recordings in AI models. It appears to be a proactive measure to maintain a consistent standard of quality.
Integrating blockchain technology enhances the enforcement of these guidelines. Through transparent record-keeping, it facilitates the tracking of consent and usage of registered voice samples, strengthening accountability within voice cloning practices. This adds a layer of trust and clarity to the whole process.
By adhering to the Framework, content creators not only gain legitimacy for their work but also contribute to building greater trust among audiences who consume AI-generated audio. This trust is vital for the ongoing growth and appeal of areas like podcasting and audiobook creation.
A crucial element that's not widely emphasized is the requirement for content creators to participate in educational programs regarding the ethical implications of voice cloning. This aspect is aimed at fostering responsible development and deployment of voice AI and addressing potential concerns related to misuse.
The Dublin Framework resonates with broader European regulatory trends surrounding AI, although its emphasis on the social consequences alongside technological advancements sets it apart. This 'holistic' approach attempts to bridge the gap between innovation and the public's concerns related to the use of voice technology. It's a compelling attempt to balance a rapidly evolving field with societal responsibility.
Irish AI Governance Standards 7 Key Voice Technology Implementation Guidelines for Content Creators - Real Time Consent Tracking for Voice Data Collection and Storage
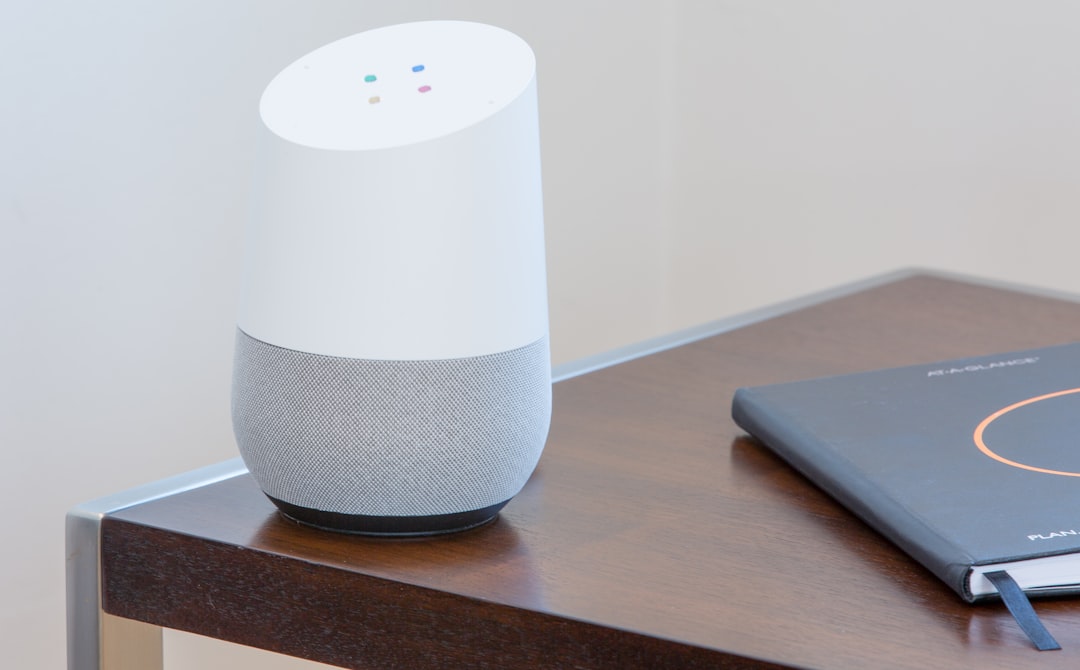
In the burgeoning fields of audiobook production, podcasting, and voice cloning, real-time consent tracking for voice data is emerging as a crucial practice for ethical data handling. It's about giving individuals ongoing control over how their voices are used, especially within AI-powered systems. This approach acknowledges the potential benefits of voice technology while emphasizing the need to protect individual rights within a framework of transparency and accountability.
As voice AI becomes more prevalent, implementing a system for real-time consent tracking becomes increasingly important for managing the ethical and legal complexities involved. It's a way of bridging the gap between the innovation these technologies offer and the crucial need to ensure user privacy. This kind of tracking is becoming increasingly significant as public awareness and concern over ethical data usage grow.
Furthermore, embracing real-time consent is a way for creators to foster trust and confidence in their work, particularly given the potential for misuse within the sphere of voice technology. This is especially crucial within the Irish context, given the emphasis on data protection regulations. Moreover, the implementation of such systems can also help to ensure diversity and inclusivity within voice data sets, avoiding biases that might emerge from less careful data collection practices. This is an important aspect that content creators need to consider to ensure the ethical development of their content. However, it's important that implementing such systems doesn't become overly burdensome or stifle innovation in the field.
Real-time consent tracking is becoming increasingly important in the responsible collection and storage of voice data, particularly within the context of AI applications in audio production, such as audiobook creation, podcasting, and voice cloning. This approach uses sophisticated audio processing to not only confirm initial consent but also to continuously monitor it throughout the data collection process. This continuous monitoring offers a more robust confirmation that individuals are aware of how their voice data is being used.
Furthermore, each voice sample could be uniquely identified through cryptographic techniques, forming an immutable record of its origins. This "data provenance" significantly enhances accountability by establishing a clear history of the consent agreement linked to each sample. It helps us trace the history of data and provides confidence about where it originated and how it was acquired.
However, questions arise regarding the effect of environmental conditions on voice clarity and the implications this may have on the integrity of consent. The sensitivity and placement of microphones can significantly impact the quality of voice data. It's interesting to ponder whether such factors influence a person's willingness to provide voice samples or whether adjustments need to be made to capture clearer recordings. Additionally, it's been observed that AI systems can discern differences in voices based on the expressed emotion. Real-time consent systems might be able to assist with managing these subtle shifts in emotion during a recording, leading to a richer and more nuanced dataset. It's a fascinating question whether we can achieve that and ensure a balance.
Background noise, too, is a noteworthy aspect. The presence of noise in a recording environment might alter the participant's decision to give consent in the first place. Advanced noise reduction algorithms embedded within real-time consent systems could improve the clarity of the samples and provide a better basis for obtaining truly informed consent.
We must also consider the ethical dimensions of auditing the collected voice data. Audits aren't just about confirming compliance, they can also identify potential biases within the datasets. This is especially crucial in voice cloning, where skewed datasets could reinforce existing societal stereotypes. It's a critical responsibility to avoid perpetuating bias, a responsibility that requires us to critically evaluate our practices and tools.
Perhaps we could explore more dynamic consent models, ones that allow individuals to adjust their preferences as new information arises or their understanding of how their data is being used changes. This could lead to a more responsible application of voice data, respecting individuals' evolving choices.
But there's a trade-off here. Asking individuals to simultaneously manage consent while providing voice samples could increase cognitive load. This might lead to less optimal data. It requires us to design systems that simplify the consent process, avoiding overwhelming participants and ensuring the highest quality voice data possible.
Perhaps we should consider a multimodal approach to consent that includes audio, visuals, and user interfaces. This could enhance communication and potentially suit a wider range of user preferences, promoting broader participation and acceptance.
Finally, there are the legal implications, especially in regions like Ireland, with its robust data protection laws. These laws mandate meticulously documented consent, and real-time consent systems could facilitate compliance, offering protection to creators from potential legal challenges.
While voice technology has immense potential for creative and productive applications, it's evident that maintaining ethical and transparent practices is of paramount importance. These are some of the aspects that researchers and engineers will need to further explore as the field continues to grow and develop.
Irish AI Governance Standards 7 Key Voice Technology Implementation Guidelines for Content Creators - Audio Quality Standards for Commercial Voice Cloning Projects
The quality of audio is a crucial aspect of any commercial voice cloning project, especially those involved in fields like creating audiobooks and podcasts. For successful voice cloning, high-quality audio samples are necessary, ideally encompassing at least 30 minutes of recording, although 3 hours is preferable for achieving optimal results. The reason for this is simple: clear and detailed audio allows the AI algorithms used in voice cloning to effectively replicate the unique characteristics of a person's voice.
However, obtaining good quality audio isn't just about the length of the recording. The environment in which the recording takes place can significantly impact the clarity of the audio. Things like ambient noise, the temperature, or even the humidity can affect how well a voice is captured. These factors, sometimes overlooked, are important for the accuracy of the voice cloning process. This becomes even more important as the ethical and legal considerations around using someone's voice with AI technologies become more complex. Creators need to not only meet technical requirements for audio quality but also navigate the emerging standards that emphasize transparency and ethical considerations.
The pursuit of high-quality audio standards in voice cloning is not simply a technical requirement; it's a crucial step toward promoting trust and legitimacy in AI-generated audio. It's an area where technical quality and ethical responsibility intersect. When audio quality is consistently high, it boosts the reliability of AI-produced content, whether it's an audiobook or a podcast, contributing to the wider acceptance of these emerging audio technologies.
In the realm of voice cloning, achieving high-fidelity results hinges on the quality of the audio samples used to train the AI models. A minimum sample rate of 44.1 kHz, akin to CD quality, is often considered the baseline for capturing the intricacies of human speech. This ensures that the nuances of a voice, those subtle inflections and tonal shifts, are preserved, crucial for generating synthetic speech that sounds natural.
Beyond the sample rate, bit depth plays a significant role in preserving dynamic range. Using a 24-bit depth allows for a wider capture of sound levels, capturing quieter sections without distortion. This is particularly relevant in voice cloning, as it's essential to retain the emotional subtleties conveyed through changes in vocal intensity.
Minimizing the noise floor is another crucial aspect of audio quality in voice cloning projects. Maintaining a low ambient noise level, ideally below -60 dB relative to the voice signal, significantly enhances the clarity of the recordings. This underscores the need for meticulously controlled recording environments, a detail that often gets overlooked in simpler audio production settings.
Research has shown that human voices convey a broad range of emotions through subtle modulation. However, capturing this emotional range in AI voice samples requires a more intentional approach. The cloning process heavily relies on how well the emotional spectrum of the voice is represented in the training data. A model trained on a restricted range of emotions might not generate the diverse and nuanced responses desired in a voice cloning project.
The push for ethical data practices in voice cloning projects goes beyond obtaining consent. It necessitates meticulous documentation of how voice samples are utilized over time. This has implications for the long-term management of voice data in a way that's far beyond what's typical in other audio production domains. These practices are necessary but can place increased pressures on those utilizing these technologies.
The way microphones are placed during recording has a direct impact on the quality of the audio. Placing the microphone at an optimal distance, usually within 6-12 inches from the speaker's mouth, helps enhance audio fidelity and minimize unwanted 'plosive' sounds created when consonants are pronounced. This is important to preserve the integrity of the original voice when creating an AI model.
Voice cloning workflows now involve a range of advanced audio post-processing techniques, like equalization (EQ) and compression. These techniques are used to refine and 'polish' audio samples, creating a more professional sound for applications like podcasts or audiobooks. The methods involved aren't just straightforward audio edits. There's a greater degree of intent behind the choices that are made when implementing these techniques.
Increasing the temporal resolution of voice recordings, effectively capturing audio more frequently, is another technique that helps improve the accuracy of voice cloning. Higher temporal resolution helps the AI more precisely model individual sounds called 'phonemes' in a language. This can reduce glitches or noticeable breaks when the synthetic voice speaks, creating smoother transitions between words.
A lesser-discussed aspect of voice cloning involves the potential impact of vocal fatigue. Prolonged speaking can subtly change a voice, leading to variation in tone and timbre. Recording sessions should be planned and structured to minimize the effects of fatigue on the voice samples. This can have a noticeable impact on the overall quality of the resulting AI voice.
Finally, creating authentic AI voices necessitates attention to linguistic and cultural nuances in pronunciation. Research shows that human listeners are acutely sensitive to these subtle variations in speech. For AI voice cloning to be successful, it's vital to acknowledge and incorporate these nuances in the voice model. This makes for more relatable and culturally appropriate use cases for the voice cloning technology itself.
These details, often overlooked in more conventional audio production, become paramount in voice cloning due to the specific nature of AI training. As voice cloning continues to evolve, it's apparent that these technical aspects play a critical role in achieving the desired level of realism and authenticity. It's a field where human speech becomes a raw material for AI, placing a greater focus on its various sonic characteristics.
Irish AI Governance Standards 7 Key Voice Technology Implementation Guidelines for Content Creators - Voice Actor Rights Protection in AI Generated Audiobooks
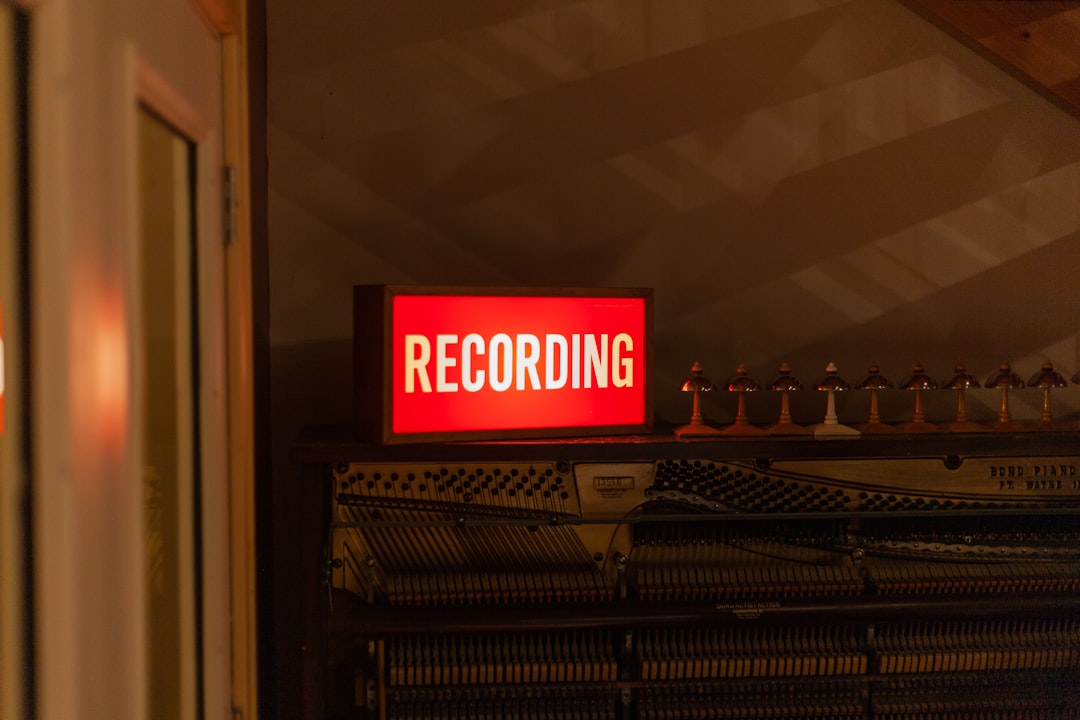
The burgeoning field of AI-generated audiobooks presents a complex challenge to the rights of voice actors. Existing legal frameworks, such as the proposed NO FAKES Act, are not designed to deal with the specific issue of voice cloning and modification, leading to a concerning gap in protection for voice actors. While AI offers potential benefits to audiobook production through efficiency and accessibility, the creation of synthetic voices derived from real voice actors raises significant ethical concerns, particularly when done without consent. The lack of established legal protections, specifically a clear right of publicity for voice actors, leaves them vulnerable to exploitation in this emerging landscape. This highlights a pressing need for new legal structures that address the use of AI-generated voices, particularly in relation to audiobook production. As AI technology advances, creating legally robust definitions of "voice rights" becomes vital, ensuring that innovation in this field is balanced with the ethical protection of individual creators and artists. It's a critical issue that requires ongoing attention to ensure fairness and prevent potential harm to voice actors in the context of AI-powered audiobook production.
The evolving landscape of AI-generated audiobooks and related audio content raises a complex set of legal and ethical questions regarding voice actor rights. While some legal precedents suggest that voice can be considered a form of personal property, providing some level of protection, current legislation often falls short of adequately addressing the specific challenges posed by AI voice cloning. For instance, existing laws in the UK don't explicitly recognize a standalone right of publicity for voice actors, leaving a legal grey area for AI applications that replicate vocal traits. Additionally, the NO FAKES Act, in its current draft, doesn't specifically protect voice actors if their voices are altered to create entirely new synthetic voices. This lack of clear legal frameworks highlights the crucial need for future legislation to define "voice rights" more specifically.
Intriguingly, AI's ability to replicate emotional nuance in synthesized voices hinges on the variety of emotional expression captured in the training data. Research shows that a wider spectrum of emotional tones in voice samples leads to more engaging and natural-sounding audiobooks. This underscores the need for diverse datasets, not just technically but also ethically and legally. On the flip side, there's the ongoing debate about the cognitive load placed on individuals during consent processes. Studies suggest that navigating complex consent protocols can tax participants, potentially impacting the quality of the voice samples captured. It's a delicate balance between ensuring informed consent and optimizing the quality of data for training purposes.
Another factor that directly affects the fidelity of AI-generated voices is vocal fatigue. Sustained vocalization during recording sessions can result in subtle changes to vocal tone and resonance, potentially impacting the accuracy of the AI model. This highlights the need to consider recording structures that include regular breaks to minimize the effects of vocal fatigue. The recording environment also plays a crucial role in voice sample quality. Even seemingly minor background noise can significantly impact the acoustic characteristics of a voice, ultimately influencing the AI training process. Thankfully, newer noise reduction technologies could potentially improve both recording quality and clarify consent processes. It is also important to note that the duration of a voice recording is key for good voice cloning. For optimal results, AI algorithms often require around 3 hours of diverse speech, a timeframe that allows for accurate modeling of subtle vocal characteristics.
Researchers also found that the accuracy of voice cloning is directly tied to how well the system captures the dynamic range of the human voice. AI models seem to perform best when they are trained on a broad range of emotional and tonal contexts, creating more natural-sounding AI voices. Moreover, microphone placement significantly impacts audio quality. Proper microphone positioning can reduce "plosive" sounds and enhance overall clarity, ultimately impacting the quality of the voice samples used for training AI. It is equally vital that AI models incorporate cultural nuances in pronunciation. Research reveals that human listeners are extremely sensitive to these variations in speech, directly influencing the success of AI voice cloning.
In conclusion, the use of AI in fields like audiobook creation and voice cloning raises a range of issues around voice rights, ethics, and legal protections. As this technology continues to evolve, the need for transparent, responsible, and ethically sound data collection practices becomes increasingly vital. There is a significant need for a thoughtful legal framework that balances innovation with the rights and interests of both voice actors and the broader public. Continued research, particularly into the human element of voice and emotion, will undoubtedly play a vital role in shaping a future where AI voice technology is both impactful and ethical.
Irish AI Governance Standards 7 Key Voice Technology Implementation Guidelines for Content Creators - Automated Voice Recognition Testing Requirements Before Public Release
Prior to making automated voice recognition systems publicly available, rigorous testing is vital to ensure they meet both ethical and technical benchmarks. This involves guaranteeing accurate transcriptions and consistent performance across a variety of dialects and accents. This is particularly important when we consider the growing use of these technologies in applications like audiobooks or podcast production, which should be accessible to all. Achieving this requires careful consideration of diverse speech patterns and promoting inclusivity.
Creating high-quality recordings within a carefully controlled environment is essential. These controlled environments help minimise background noise and other factors that can interfere with the sound quality. Ensuring this is paramount for producing trustworthy and realistic AI-generated voices.
The safeguarding of individual rights is paramount, which means implementing mechanisms for obtaining and continuously monitoring real-time consent regarding how voice data is collected and used. It's essential that individuals understand how their voices are being employed by AI systems, and these processes should ensure transparency and accountability.
The purpose of these testing requirements is to navigate the delicate balance between promoting innovation in the field of voice technology and addressing valid societal concerns about privacy and the fair representation of diverse voices. Meeting these benchmarks facilitates responsible development and contributes to the creation of voice technology that benefits everyone.
The effectiveness of AI voice cloning hinges on the quality and diversity of voice samples used in training the AI models. Researchers have found that extending the voice sample duration to a minimum of three hours, preferably more, leads to more accurate capture of those subtle, nuanced features that make human voices unique. This is crucial for generating truly natural-sounding AI voices, especially for applications like audiobooks, podcasts, and other audio content.
Interestingly, the emotional range captured in the voice samples directly influences the quality of the resulting AI-generated voice. When AI systems are trained on diverse emotional expressions, they produce more captivating and engaging audio outputs. This becomes increasingly important as we aim to create AI voices that are not just technically accurate but also emotionally resonant, particularly within fields like audiobook production where audience engagement is vital.
However, achieving high-quality voice samples isn't simply a matter of duration and emotional range. Studies indicate that environmental factors like noise, humidity, and even temperature can subtly alter the acoustic properties of voice recordings. This raises an interesting question: how do these environmental influences impact the way AI systems interpret and recreate human voices? There's a possibility that the very environment in which a voice is captured could affect the AI model's ability to clone it accurately.
Another intriguing aspect is the interplay between the consent process and the cognitive load it places on individuals providing voice samples. It's been observed that overly complex consent procedures can lead to increased cognitive load for participants, potentially affecting the quality and naturalness of the recorded voices. It's a balancing act between ensuring individuals are fully informed about how their voices will be used and capturing optimal voice data for AI training.
Furthermore, prolonged periods of speech recording can cause vocal fatigue. This subtle vocal change might have implications for the AI model's accuracy. Designing recording sessions with breaks to minimize fatigue could be a way to maintain the integrity and consistency of the voice samples used in training AI models.
Another critical area is ensuring a wide dynamic range in the recordings. This allows AI models to replicate a full range of vocal nuances, including both soft and loud speech. It contributes to greater realism in the synthesized voices.
Microphone placement plays a significant role in capturing high-quality audio for AI voice cloning. An optimal microphone distance, usually between 6 and 12 inches from the speaker's mouth, helps to minimize unwanted sounds and maximize clarity, improving the overall quality of the voice samples and their use in AI training.
It's also crucial to recognize the impact of cultural and linguistic nuances on pronunciation. Research suggests that human listeners are extremely sensitive to these subtle variations in speech. AI models that incorporate these variations are likely to be perceived as more authentic and relatable by a broader range of listeners.
Thankfully, improvements in noise reduction technologies offer a promising solution to improve audio quality and potentially make the consent process more user-friendly. This is a vital development, as the clarity of recorded voice samples directly impacts the quality of the AI models that are built from them.
Currently, the legal frameworks concerning the rights of voice actors in the realm of AI-generated audio are not robust enough. The absence of established 'voice rights' leaves voice actors potentially vulnerable. This raises a critical concern regarding the protection of intellectual property in the age of AI voice cloning. There's a growing need for legislation that clarifies these rights, ensuring fairness for both voice actors and the broader public.
These are just some of the areas where researchers and engineers need to direct their focus. It's evident that as AI voice technologies continue to evolve, it's crucial to maintain ethical and transparent data collection and usage practices. We need to strike a careful balance between innovation and the responsible implementation of these technologies to ensure that they benefit society as a whole.
Irish AI Governance Standards 7 Key Voice Technology Implementation Guidelines for Content Creators - Data Privacy Controls for Voice Sample Management Systems
Within the sphere of voice technology, particularly in applications like audiobook production and podcasting, the management of voice sample databases necessitates robust data privacy controls. As these systems gather substantial amounts of personal voice data, ensuring the ethical use of this information and protecting individuals' rights is paramount. Content creators now operate within a landscape where frameworks like the Dublin Audio AI Framework are driving the need for comprehensive data privacy standards. This necessitates informed consent procedures, transparent data handling, and meticulous attention to how voice samples are stored and applied.
The growing use of voice technology in content creation presents a balancing act. While innovation promises exciting advancements in personalization and audio production, the ethical and legal ramifications of collecting and processing this sensitive data cannot be overlooked. This careful consideration of data privacy within voice sample management will play a key role in shaping the future of content creation, especially within areas where personal voice and expression are central, such as podcasts and audiobooks. There's a growing tension between technological innovation and maintaining ethical and legal compliance that needs constant attention.
Within the sphere of voice sample management systems, particularly those used for audio book creation, podcasting, and voice cloning, several key considerations arise regarding data privacy and the responsible use of voice data. The way we position microphones during recording, for example, can affect not only the audio's quality but also the emotional nuance captured within the sample. Getting too close might lead to unwanted pops, while positioning the microphone too far away might remove the subtle intonations that make a voice unique. This is a challenge as we aim to create AI-generated voices that sound authentic and natural.
Environmental aspects also play a role, with temperature and humidity subtly altering how a voice sounds when recorded. Research suggests even minor variations in these factors can impact an AI's capacity to accurately clone a voice. This raises questions about the level of environmental control needed to ensure the data used to train AI voice cloning models are truly representative.
Consent processes are also of great interest. We've seen that overly complicated consent protocols can be tiring for those involved, potentially affecting the naturalness of the recordings. This highlights the struggle to ensure both data quality and ethical compliance. Striking the right balance is key here, so we don't sacrifice quality for the sake of compliance, nor the other way around.
Furthermore, extended recording sessions can lead to vocal fatigue. As a person's voice tires, subtle changes occur in its resonance and tone. Studies show that structuring recording sessions with breaks can help minimize this fatigue and keep the voice data more consistent.
It's also important to ensure a wide dynamic range in voice recordings, allowing AI systems to capture a broad spectrum of vocal nuances, like soft and loud speech. This, in turn, contributes to a more realistic sounding output in synthesized voice applications.
Another crucial factor is the emotional range within voice samples used to train an AI. It appears that a larger emotional palette in the training data directly contributes to more engaging and natural-sounding voices. For instance, in the realm of audiobook production, it’s essential to evoke a spectrum of emotions to keep the listener engaged.
AI models that are trained on voice samples that reflect diverse linguistic and cultural characteristics, tend to perform better. Human listeners are acutely sensitive to the subtleties of pronunciation, and models that account for these variations often result in more relatable and authentic cloned voices.
The use of cryptographic techniques for tracking the origin of voice data strengthens accountability and promotes trust. By knowing where each voice sample comes from, we improve the overall ethics of how this data is handled.
Routine audits of the voice sample databases also help to maintain a consistent level of quality. It helps to prevent outdated or inadequate samples from negatively affecting the performance of AI voice models, assuring that the data used is consistently excellent.
The advancement of real-time consent tracking methods holds promise for dynamically managing the use of voice data in AI applications. This increased control, combined with transparency, provides individuals with a stronger understanding of how their voice data is being used and, in turn, allows them to direct how it’s employed.
As the fields of audiobook production, podcasting, and voice cloning continue to evolve, it's clear that the ethical handling of voice data is paramount. We are at the very beginning of understanding how to leverage this new technology in ways that are ethical and beneficial. While the promise of AI voice technology is enticing, a nuanced understanding of its implications and a constant critical eye towards ethical practices are needed to ensure its positive development and integration into society.
Irish AI Governance Standards 7 Key Voice Technology Implementation Guidelines for Content Creators - Technical Guidelines for Voice Model Training and Testing
Technical guidelines for voice model training and testing are crucial for ensuring the quality and ethical use of voice technology, especially in applications like audiobook production and voice cloning. These guidelines emphasize the need for high-quality audio samples, suggesting a minimum recording duration of three hours to capture the nuanced characteristics of human speech. Maintaining a controlled environment during recordings is also vital to minimize external noise and other factors that could affect the clarity of the voice sample. Proper microphone placement and techniques to ensure clear audio are also essential.
Furthermore, the guidelines highlight the importance of real-time consent tracking to ensure individuals are aware of how their voice data is being used and to promote transparency. They stress that the training datasets should include a diverse range of emotional expressions to generate synthetic voices that are both accurate and emotionally resonant. Regular audits of voice sample databases are also recommended to ensure data quality and prevent the use of outdated or unsuitable recordings.
Ultimately, the guidelines aim to establish a balance between the potential benefits of voice technology and the ethical considerations that must guide its development. These technical standards are crucial in building trust and confidence in AI-generated audio content, helping to ensure that this technology is developed and used responsibly within society. While there are potential benefits of voice cloning and its application in audio book production and podcasts, it is crucial to acknowledge that without strict technical and ethical standards the use of such technologies can be fraught with potential ethical issues.
Within the realm of voice technology, particularly for tasks like audiobook production, podcasting, and the increasingly prevalent field of voice cloning, the quality and nature of the voice data used to train AI models are paramount. A number of technical details, often overlooked in other audio production contexts, are crucial for ensuring both the technical fidelity and ethical use of this data.
Firstly, the acoustic environment in which recordings are made plays a substantial role in determining the quality of the final audio. Minimizing ambient noise and echoes, through careful room design and equipment choices, enhances the clarity of voice samples, which directly benefits the accuracy of AI models designed to replicate human speech.
Furthermore, the duration of a recording significantly affects the capability of an AI model to accurately clone a voice. Typically, at least three hours of recordings are required for creating robust voice models. This extended duration allows the AI algorithm to capture the subtleties and idiosyncrasies of a person's voice that might be missed in shorter recordings.
Another key aspect is the dynamic range of recordings – the difference between the softest and loudest parts of an audio signal. AI systems relying on a wider dynamic range during training are capable of generating voices that exhibit a more comprehensive array of human emotions, leading to more engaging and natural-sounding synthetic speech. This is especially significant in fields like audiobook creation, where emotional expression is fundamental to the listening experience.
Proper microphone placement is another factor that can have a substantial impact on voice recording quality. Keeping a consistent microphone distance, typically within the range of 6 to 12 inches from the speaker's mouth, reduces unwanted sounds and enhances the quality of the sample. It also ensures that the nuances and specific qualities of a voice are captured without being affected by unwanted "plosive" sounds common in certain consonant pronunciations.
We also need to consider environmental factors that can inadvertently affect voice characteristics. Subtle changes in temperature and humidity within the recording environment can affect the acoustic nature of a person's voice. These variables can slightly alter voice pitch and resonance, which, in turn, could lead to a slight skewing of how accurately AI models can clone that voice.
Furthermore, the training data must encompass a diverse array of emotional expression. AI voice cloning models that learn from datasets rich in different emotional states generate more engaging and relatable synthetic voices. In turn, this characteristic contributes to improved audience engagement when those voices are used in applications such as audiobooks or podcasts.
Maintaining consistency in vocal quality is a concern during prolonged recording sessions. Extended sessions can lead to vocal fatigue, which subtly changes the characteristics of a voice. Implementing structured breaks to minimize vocal strain is crucial to ensuring that voice samples are consistent and of high quality.
Human listeners are acutely aware of cultural variations and accents in spoken language. Therefore, AI models trained on a wider range of language and cultural variations are more capable of producing natural and relatable synthetic voices that will resonate more authentically with a broad audience.
Innovation in consent methods, such as real-time consent tracking systems, represent an opportunity to ethically manage the use of voice data. Such systems allow for greater transparency and offer ongoing control to individuals over how their voice data is employed. This also creates a foundation of trust between individuals and the AI systems that utilize their voices.
Maintaining the quality and relevance of voice data used to train AI models requires regular audits of the sample databases. These audits ensure that data is current, of high quality, and doesn't introduce biases from outdated recordings. This step is essential for preventing inaccuracies or unintended biases from entering the training process.
These technical guidelines are just some of the aspects that researchers and engineers will need to explore further as AI voice technologies continue to evolve. The ongoing development of these technologies necessitates striking a balance between innovation and responsible data handling practices. There is a need to establish robust, clear technical frameworks that acknowledge the intricate nature of voice, its relationship to cultural and emotional contexts, and the ethical implications of its use in AI applications. This is vital to ensuring that these technologies benefit society in an ethical, respectful, and beneficial way.
More Posts from clonemyvoice.io: