Leveraging Voice AI A Strategic Guide to Upskilling Audio Production Teams in 2024
Leveraging Voice AI A Strategic Guide to Upskilling Audio Production Teams in 2024 - Voice Dataset Collection Methods for Training AI Models in Audio Production
The increasing use of AI in audio production necessitates robust methods for gathering voice data to train AI models effectively. Outsourcing or crowdsourcing data collection can be a valuable strategy, enabling teams to acquire a wider range of audio samples that better suit their project requirements. Preparing this raw audio through techniques like streamlined data management libraries is critical for ensuring the dataset's quality and suitability for training. The manner in which we structure the collected audio data is equally important. Converting audio annotations into standardized formats like JSON or XML is vital for training AI systems effectively, especially when aiming to combat potential biases that can arise from a skewed representation of voices. It's essential to prioritize data quality and diversity when collecting audio data for AI. Adhering to best practices ensures audio production teams can fully realize the potential of voice AI and successfully integrate it into their workflows. This approach will prove crucial for the future of audio production, enabling improvements in voice cloning, podcast creation, and audiobook production, amongst other applications.
The field of AI in audio production, particularly voice cloning, is experiencing rapid growth, fuelled by the ever-increasing demand for natural-sounding, versatile AI voices. This demand highlights the critical role of high-quality voice datasets for training these models effectively. While outsourcing or crowdsourcing can expand the scope of data collection, it’s essential to acknowledge that the resulting data might not always be uniform in quality or represent the full diversity of human speech. Tools like the Datasets library can help standardize and preprocess audio data, making it more suitable for AI training pipelines.
Datasets such as ESC50 provide valuable resources for understanding the nuances of environmental sounds, which are often present in recordings, and datasets like the Million Song Dataset offer a rich source of audio features for music-related applications. However, the composition of these datasets can introduce bias. For example, studies have shown that a disproportionate amount of data for certain AI applications originates from a small number of locations, which might limit a model's ability to generalize across different populations and dialects.
Ensuring data quality is paramount. Converting audio annotations into structured formats like JSON or XML is crucial for compatibility with AI platforms and for efficient training. We've seen the rise of repositories like Hugging Face which host a multitude of audio classification models. While useful for identifying existing solutions, it is important to understand the underlying data which powers these models and select models appropriate for the task at hand.
The need for diverse datasets is undeniable. While voice cloning is becoming increasingly refined, there are challenges in creating models that can accurately mimic various accents and dialects due to limitations in the datasets. Furthermore, capturing the richness of human speech, including subtle cues like emotions and hesitations, requires careful design of the data collection process. As we delve deeper into applications like clinical speech AI, the importance of data diversity becomes critical. Skewed datasets can lead to inaccurate or biased model outputs, impacting their ability to provide accurate assessments across diverse populations.
The development of synthetic data generation techniques represents an exciting opportunity to produce substantial amounts of voice data without the need for extensive real-world recording. Methods such as waveform manipulation allow us to create diverse voice samples from existing recordings, potentially addressing issues of dataset size and diversity. However, it's important to acknowledge the potential limitations of synthetic data, such as a potential lack of naturalness or diversity compared to authentic recordings.
Furthermore, data collection methods that leverage existing technology, such as voice assistants, can offer access to vast quantities of real-world interaction data. However, careful consideration of ethical and privacy concerns associated with such methods is crucial. The potential for misuse and the public's increasing demand for transparency regarding how their data is used must be carefully addressed. Finally, the dynamic nature of language necessitates ongoing updates to the datasets used for training voice AI models, ensuring that models can adapt to shifts in language and speaking patterns. By acknowledging the limitations of existing datasets and actively pursuing methods for creating more comprehensive and representative resources, we can contribute to the development of more robust, equitable, and versatile voice AI systems.
Leveraging Voice AI A Strategic Guide to Upskilling Audio Production Teams in 2024 - Workflow Integration of Voice AI Tools with Traditional DAW Software
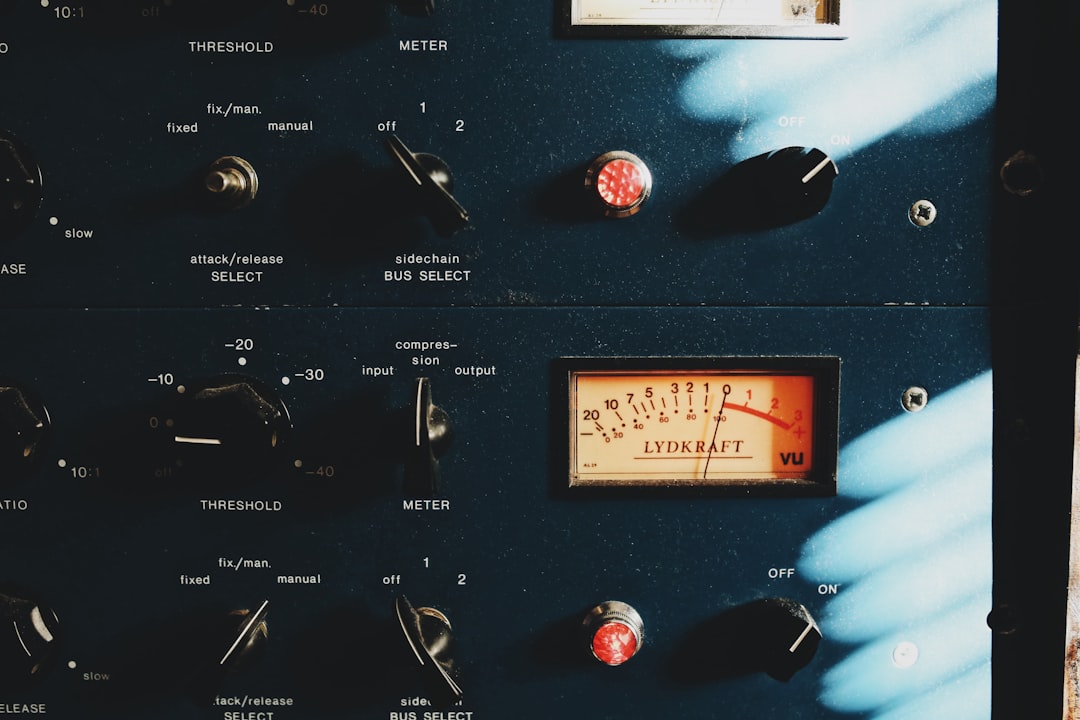
The convergence of voice AI tools and traditional Digital Audio Workstations (DAWs) is reshaping how audio is produced, impacting fields like music, podcasting, and audiobook creation. This integration allows audio professionals to streamline processes like vocal editing and audio enhancement within their existing workflows. AI-powered plugins can significantly improve vocal tuning, offering greater control over sound quality and consistency. Moreover, the use of voice cloning tools within DAWs opens up new possibilities for creative manipulation of vocal tracks, fostering greater flexibility in experimentation and sound design.
While these advancements offer clear advantages, it is important to acknowledge potential drawbacks. As AI becomes increasingly integrated into the production process, there's a risk that the human touch and emotional depth often present in audio could be compromised. It’s important that these tools augment human creativity rather than replace it entirely. Moving forward in 2024, upskilling audio production teams to understand the capabilities and limitations of AI-powered DAW plugins will be essential. Adapting to these advancements while maintaining a focus on the artistic value of sound will be key for teams seeking to remain at the forefront of audio production.
Voice AI tools are starting to change how we use traditional Digital Audio Workstations (DAWs). They can handle tedious tasks like recording and basic edits, freeing up audio producers to concentrate on the creative side of things instead of getting bogged down in technical details.
Bringing voice AI into the DAW workflow has the potential to really speed up the editing process. I've seen research that suggests AI-powered tasks like noise reduction and vocal alignment can cut post-production time by as much as 50%. It's intriguing to think of the impact that could have on project timelines.
These voice AI systems are capable of learning how we work within DAWs, and based on our actions, they can offer customized voice commands for our workflows. This could lead to more streamlined workflows and happier producers.
I see a lot of potential for using voice AI in podcast production. It might allow for real-time suggestions during recording, so the host gets feedback on things like delivery and pacing instantly. It could be like having a coach right there in the headphones.
Voice cloning technology integrated into DAWs opens up new creative possibilities. It means that you could reproduce voice performances without having the original talent present in the studio. This level of flexibility would be great for various projects, especially when you need to recreate a specific vocal style or address talent availability issues.
Improvements in voice recognition algorithms mean that voice AI can now accurately transcribe spoken words, which can be a game-changer for creating scripts from recordings for things like audiobooks or podcasts. It could really streamline that initial stage of the process.
The idea of AI working together with DAW software for generating musical scores that adapt to the tone of a recorded voice is quite interesting. Imagine a music composition that shifts based on the emotions expressed in the voice recording. It could generate a cohesive and dynamically responsive auditory experience.
The recent progress in voice synthesis means that we can now produce completely new character voices for animated productions right inside the DAW. This could really streamline the initial phases of animated content creation, as it removes the need for extensive voice actor collaboration at the very beginning of a project.
In audio mixing, it's promising to see that voice AI can automate the process of balancing tracks using verbal instructions. The system can understand the context of your instructions, like if you want to emphasize a specific vocal track over background effects.
Of course, there are hurdles to overcome when it comes to merging voice AI tools into traditional DAWs. One of the biggest is compatibility across different platforms and file formats. If we don't think about this early in the development process, it could really create complications down the road. It's an interesting technical challenge that needs to be addressed before these integrations become widespread.
Leveraging Voice AI A Strategic Guide to Upskilling Audio Production Teams in 2024 - Quality Control Protocols for AI Generated Voice Synthesis
As AI-generated voice synthesis becomes more prevalent in audio production, from podcasts to audiobooks and voice cloning, establishing robust quality control protocols is essential. These protocols need to ensure the synthesized voices are not only technically accurate but also emotionally nuanced and natural-sounding. This requires continuous evaluation against established quality benchmarks to identify any deviations from the desired output. Producers should be particularly cautious of potential biases that might creep in, affecting accents, emotional expression, and vocal tone. Unrefined AI models can lead to artificial or inaccurate representations of dialects or emotional nuances.
With AI tools seamlessly integrating into traditional production workflows, audio teams need to establish guidelines for assessing both the technical and artistic aspects of the AI-generated output. It's important to ensure that AI enhances the artistic narrative of audio, not diminishes it. In the fast-paced world of audio production, consistently applying rigorous quality control is crucial for unlocking the full potential of AI voice synthesis while simultaneously preserving the artistry inherent in creating sound. This continuous process of refinement is vital to achieving greater authenticity and expanding the possibilities of what AI can achieve within the audio production landscape.
AI-generated voice synthesis has made remarkable strides in creating realistic voice replicas with control over emotions and language translation capabilities. However, replicating the nuanced aspects of human speech, like intonation, stress, and emotional delivery, still poses challenges for these systems. This complexity necessitates the development of rigorous quality control protocols to ensure that synthetic voices closely mimic the subtleties of natural speech, particularly crucial in applications like audiobooks where emotional connection is paramount.
The evaluation of AI-generated voice quality often extends beyond simply analyzing the audio output. A more comprehensive approach integrates feedback from various sensory inputs. Combining auditory and visual feedback offers a more accurate assessment of voice synthesis, proving particularly beneficial in applications like podcasts or animated productions where the overall experience is a combination of audio and visuals.
One of the hurdles in refining the quality of AI voice synthesis lies in mastering the prosodic elements of speech. Research has shown that even minor inconsistencies in the rhythm and flow of synthetic speech can render it unnatural or robotic. Therefore, rigorous testing against human benchmarks during production is crucial to address this challenge.
The evolution of voice synthesis systems has allowed for real-time feedback mechanisms during the recording process. This allows producers to address errors and inconsistencies instantly, preventing issues from impacting the final output. This real-time quality control can be very helpful in dynamic recording environments like live podcasts.
Unfortunately, biases can sneak into voice synthesis, potentially favoring certain accents or genders. Consequently, it's vital to incorporate dedicated quality control measures designed to identify and mitigate these biases. This type of controlled evaluation can highlight any systematic tendencies in voice synthesis, impacting how diverse content is perceived by listeners.
Interestingly, listener preferences often lean towards authentic human voices over synthetic ones, especially in narrative formats like audiobooks. Understanding these preferences through listener studies is crucial for improving voice synthesis techniques and managing listener expectations. This could guide developers in refining AI systems to bridge the gap between synthetic and authentic voices.
Fortunately, advancements in technology allow for the implementation of automated error detection systems. These tools can quickly identify unnatural pauses, pitch inconsistencies, and other discrepancies in the voice synthesis output. This automated approach to quality control is especially helpful in settings where production volumes are high, such as podcast networks or audiobook publishers.
It's essential to consider how post-production techniques can affect the quality of AI-generated voices. Manipulating pitch, adding effects, or blending with other tracks can alter the overall quality and clarity of the synthesized audio. Thus, post-processing quality control checks are also necessary.
The increasing adoption of AI voice synthesis also presents ethical considerations. Questions regarding consent from original voice sources and concerns about potential misuse in deceptive contexts need careful consideration. It's crucial that quality control doesn't just focus on technical performance, but also aligns with ethical standards to ensure trustworthiness.
Voice cloning, while a powerful technology, still has limitations. Current models might struggle to spontaneously adapt to changes in context or express a wide range of emotional states. Understanding these restrictions is essential to set realistic expectations, especially for content involving storytelling and character development. By being mindful of these limitations and incorporating them into quality control processes, producers can ensure the technology is used responsibly and effectively.
Leveraging Voice AI A Strategic Guide to Upskilling Audio Production Teams in 2024 - Team Training Strategies for Voice Cloning Technology Adoption
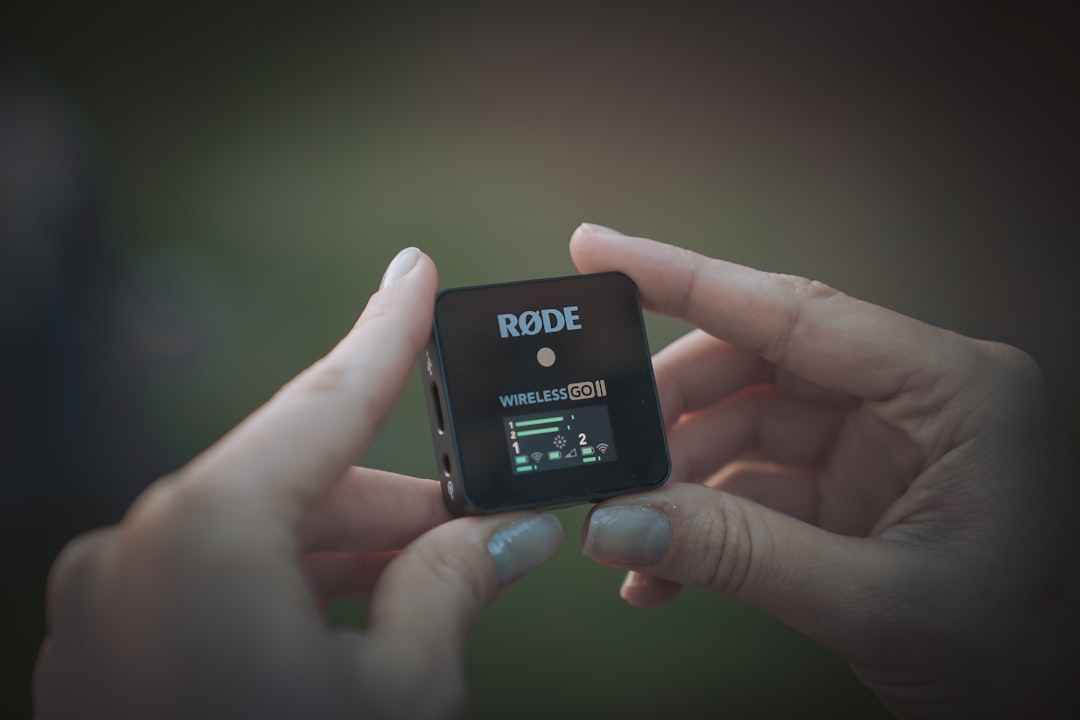
The rapid advancements in voice cloning technology demand that audio production teams adapt through well-structured training. Instead of comprehensive programs, a more effective approach involves breaking down learning into focused modules. This allows team members to progressively acquire a thorough understanding of voice cloning concepts without being overwhelmed by a large volume of information at once. Incorporating real-time feedback mechanisms into these training modules can greatly aid comprehension and allow for immediate application of the learned skills across various audio production tasks like podcasting and audiobook creation. It is crucial to approach this integration critically, carefully assessing the ethical considerations and potential for biases that voice cloning can introduce. The goal should be to ensure that this innovative technology enhances, not detracts from, the emotional richness and genuine character that good audio production often conveys. Encouraging a mindset of continuous learning and adaptation will be vital for navigating the ever-evolving landscape of voice AI in 2024 and beyond.
Voice cloning technology is becoming increasingly sophisticated, utilizing unsupervised learning methods to analyze vast amounts of audio data. This allows the creation of synthesized voices that accurately capture unique phonetic traits, accents, and even individual emotional nuances. The result is a new level of personalization that could greatly enhance the listener experience in mediums like audiobooks and podcasts.
Research suggests that in controlled experiments, listeners are unable to differentiate between human and AI-generated voices more than 80% of the time. This growing indistinguishability has intriguing implications for the future of audio production, particularly in commercial voiceover work. It raises the question of whether we may eventually see a shift towards AI-generated narration without compromising quality.
We're learning that even minor alterations in vocal pitch can profoundly impact the emotional response in listeners when it comes to AI-generated voices. This emphasizes the crucial role of precise emotional expressiveness in voice synthesis. For audio producers aiming to create compelling narratives, understanding this sensitivity is essential for keeping audiences engaged.
It's been shown that combining voice cloning with real-time data analytics can potentially enhance listener engagement metrics. These systems can adjust vocal styles in response to audience reactions during live recordings, creating dynamic feedback loops. This is a fascinating concept that could change how producers interact with their audience, particularly in podcasting.
The gaming industry is seeing a growing trend in the use of AI voice synthesis. Dynamic voice generation within games can create immersive character interactions on-the-fly. This provides a more personalized gaming experience and allows narratives to adapt based on player choices, marking a new chapter in interactive storytelling.
Traditionally, post-production in audio production has been a very time-consuming process. However, AI advancements have the potential to revolutionize this aspect of the workflow. Automated systems can analyze recordings for inconsistencies and propose solutions, potentially reducing editing time by as much as 70%. This would free up audio engineers to focus on more creative aspects of their work rather than being bogged down in tedious edits.
There has been exciting development in voice cloning models capable of synthesizing multilingual speech using a single model. This recent advancement is breaking down linguistic barriers in audio production. It's particularly advantageous for creating audiobooks and conducting global marketing campaigns, as it allows for a seamless adaptation of content across diverse linguistic audiences.
Another remarkable development is the ability of some AI models to clone "micro-expressions," like laughter and sighs, and incorporate them into synthesized voices. This can add a greater sense of authenticity to character portrayals in audiobooks and animated projects. This addresses the previous limitations of AI voices, making them more relatable and dynamic.
Ethical considerations related to voice cloning are paramount. Developing protocols to ensure that individuals whose voices are used for modeling provide consent is crucial. This has sparked the creation of governance frameworks demanding transparency about how synthesized voices are utilized, influencing industry standards across audio and multimedia production.
The integration of AI into the audio production workflow necessitates specialized training for audio engineers and producers. A deep understanding of machine learning models, their capabilities, and limitations is vital for teams aiming to effectively use this technology while preserving their artistic vision.
Leveraging Voice AI A Strategic Guide to Upskilling Audio Production Teams in 2024 - Collaborative Projects Using Voice AI in Audiobook Production
Voice AI is revolutionizing audiobook production, creating opportunities for collaboration and enhancing both speed and artistic expression. Utilizing AI-generated voices accelerates production cycles, making audiobooks more accessible to authors and publishers, especially those facing budget constraints associated with hiring human narrators. This collaborative approach often involves AI handling tasks such as voice modulation and editing, while human narrators contribute the emotional nuance and artistic flair that are crucial for compelling storytelling. The trend suggests that audiobook creation is becoming more accessible to a wider range of creators, potentially shifting the landscape away from a small group of established producers. However, challenges still exist. Producers need to be mindful of inherent biases within AI models and ensure that the resulting audiobooks retain the emotional depth that captivates audiences. The future of this process is tied to how effectively these technological advancements are balanced with the human element of storytelling.
Collaborative projects using voice AI in audiobook production are revealing a new landscape of possibilities. It's fascinating how AI can now generate distinct character voices, allowing audiobook producers to craft unique vocal personalities for different roles without the need for a large ensemble of human voice actors. The potential for weaving emotional nuances into these AI-generated voices, creating more dynamic and engaging listening experiences, is intriguing.
Moreover, the integration of sophisticated Natural Language Processing (NLP) into voice AI is allowing for a deeper level of contextual awareness within audiobook narratives. AI-powered voices can now respond in real-time to evolving plot points and character interactions, leading to a more immersive experience for listeners. The ability to tweak vocal characteristics in real-time based on emotional cues in the script is remarkable, producing a more fluid and nuanced narrative flow.
One of the most promising uses of voice AI within audiobook production is the creation of dyslexia-friendly audio. By adjusting the speed of narration and modifying the vocal characteristics, we can produce audiobooks that are easier for people with dyslexia to follow and comprehend. It's a compelling example of how AI can contribute to greater accessibility in the audio production realm.
We are also seeing substantial progress in using voice AI to create multilingual audiobooks. This could revolutionize the production process, especially for international audiences. Imagine an audiobook where the narrator seamlessly switches between languages, effortlessly bridging cultural divides for listeners around the globe.
The ability to gather data on how listeners interact with AI-narrated audiobooks and using this information to shape future productions opens up a new avenue for personalization. Imagine real-time audience feedback guiding the refinement of vocal styles and delivery for a more tailored and immersive listening experience.
Voice AI is also becoming more adept at identifying and reflecting the subtleties of human speech, particularly stress and emotional tone. This could be invaluable in generating believable audiobook characterizations and fostering deeper emotional connections with listeners. However, the field is not without its caveats. The potential for subtle biases to creep into voice AI models, unintentionally favoring certain genders or ethnicities, remains a concern. It’s critical for developers to carefully monitor outputs and address these issues to ensure a more equitable and representative representation of voices in audiobook productions.
It's noteworthy that the growing sophistication of voice cloning opens up intriguing new avenues for the audiobook industry. For instance, it might be possible to “restore” the voices of historical figures for audiobooks about historical events, effectively bringing voices from the past into a modern storytelling context. This could be an incredibly powerful way to blend educational and narrative elements within audiobook content.
There's also a fascinating focus on creating more reliable metrics to assess the quality of AI-narrated audiobooks. It's not enough to merely measure technical aspects like clarity and pitch. Developers are recognizing that the listener experience is a crucial factor, and we need new ways to evaluate the perceived authenticity and enjoyment of audiobooks produced with AI voices. This will drive innovation and improve the overall quality of audio production using these systems.
These are just a few examples of how voice AI is reshaping the collaborative landscape of audiobook production. While the field is still in its early stages of development, these advancements are pushing the boundaries of what is possible in audio storytelling. The future of audiobooks undoubtedly looks more diverse, engaging, and accessible thanks to these ongoing developments.
Leveraging Voice AI A Strategic Guide to Upskilling Audio Production Teams in 2024 - Ethical Guidelines for Voice Recreation and Authenticity Verification
With the rise of voice AI and cloning technologies in audio production, like podcasting and audiobook creation, establishing clear ethical guidelines for voice recreation and authenticity verification has become crucial. Protecting individuals whose voices are being synthesized is paramount, necessitating informed consent and transparency around how their voice data is used. Maintaining the human element in audio production, especially the emotional depth and authenticity that are integral to compelling narratives, is vital even as AI tools become increasingly integrated into workflows. It's a balancing act that requires careful consideration of the technology's capabilities alongside the need to preserve the artistry and storytelling aspects of audio.
The guidelines need to evolve alongside the technology. Including diverse voices in the conversation around ethical standards is crucial for responsible development. It's easy to get caught up in the technological advancements and overlook the ethical implications. As the landscape shifts rapidly, it's important to avoid the potential pitfalls of biased or misused voice AI models, and ensure that the emotional essence of audio production is not sacrificed in the pursuit of technological progress. Navigating this path responsibly is a collective effort, one that requires a nuanced approach that blends technical expertise with ethical awareness.
Exploring the ethical landscape of voice recreation and authenticity verification reveals some intriguing aspects we need to consider as we integrate voice AI into audio production. For instance, it's not just enough to obtain consent for using someone's voice in training an AI model; ethical guidelines advocate for obtaining consent for any future uses of that voice, even ones we might not anticipate today, such as in political campaigns or advertising.
Furthermore, researchers are finding that these voice cloning systems can inadvertently perpetuate biases that exist in our society. This means that the AI might associate certain accents or vocal tones with specific genders or ethnicities, which isn't ideal. Ethical guidelines are crucial in pushing for equitable representation within these systems.
The ability of AI voices to mirror human emotion is an exciting advancement. But, it also necessitates careful evaluation. These emotional inflections need to be accurate and contextually appropriate, considering cultural nuances to avoid unintentional misinterpretations.
The debate on whether AI-generated voices can truly replicate the authenticity of a human voice is an ongoing one. Ethical guidelines are suggesting that transparency is key here. Producers should inform listeners when an AI voice is used, letting the listener decide whether that impacts their experience.
When we consider voice cloning, it's essential to acknowledge the cultural context of the voices being replicated. Guidelines stress the importance of understanding the socio-linguistic aspects of a voice to avoid misrepresenting cultures or perpetuating stereotypes. This is particularly important in a world with increasing globalization.
Live interactions with voice AI, like in interactive podcasts, necessitate transparency in real-time. Listeners should know when they're interacting with an AI-generated voice versus a human speaker.
We are also facing questions regarding who owns the rights to content created by AI. The discussion surrounding ownership and royalty payments is still in its early stages, but there are growing calls for clear frameworks to ensure fairness for all parties involved.
Furthermore, we need to acknowledge the potential impact these AI voices can have on our psychology. The emotional responses that AI-generated voices elicit can have a significant influence, especially when we're dealing with sensitive topics like mental health support.
Interestingly, research shows that while AI voices are technically impressive, they sometimes create a dissonance between emotional delivery and content. This "authenticity mismatch" highlights the need for ensuring that the emotional narrative is congruent with the actual content.
Lastly, as these technologies continue to rapidly evolve, the need for ethical guidelines is becoming even more important for shaping the future of legislation surrounding voice AI. It's a dynamic area with discussions regarding legal frameworks that protect both those creating the AI and the individuals whose voices are being used.
These points highlight the complexities we face as voice AI progresses, emphasizing the importance of a robust ethical framework to guide its development and application responsibly.
More Posts from clonemyvoice.io: