Voice Cloning AI Integration How Knowledge Management Systems Shape Enterprise Audio Production
Voice Cloning AI Integration How Knowledge Management Systems Shape Enterprise Audio Production - Seamless Text to Speech Workflows Transform Audio Book Narration at Penguin Random House
Penguin Random House is pioneering a new era in audiobook production by seamlessly integrating text-to-speech technologies, including AI voice cloning. This shift allows for quicker creation of high-quality audiobooks, revolutionizing the narration process. Services like ElevenLabs and Speechki generate remarkably human-like voices in various languages, significantly speeding up production. However, even with these advancements, AI voices often struggle to replicate the intricate emotional range that human narrators naturally bring to a story. This gap underscores the continuing need for human artistry in audiobook creation. Meanwhile, managing the growing volume of content requires robust knowledge management systems to keep production organized and efficient. The future of audiobooks likely hinges on finding the balance between the efficiency of AI and the emotional depth that only humans can convey.
It's fascinating how Penguin Random House is leveraging AI to reshape audiobook production. By streamlining the process with text-to-speech workflows, they've managed to cut down narration time from weeks to a matter of hours. This is a game-changer in a highly competitive market, allowing for quicker turnaround and potentially higher output.
The ability to clone a narrator's voice is also intriguing. This technology enables them to maintain consistency, even if the original narrator isn't available, ensuring that a specific voice remains associated with a particular series or author. The AI analyzes the nuances of a text, attempting to capture the subtle emotional tones that humans bring to reading aloud. Though this is still an evolving area, it shows promise for delivering a more nuanced reading experience.
The whole production pipeline has become more automated, from the initial text-to-speech conversion to post-production editing. These advancements in audio editing tools help maintain high audio quality while simultaneously removing the need for extensive manual clean-up.
Furthermore, using the same voice model for multiple languages opens doors to accessibility on a global scale, avoiding the need for multiple narrators. AI's ability to learn and replicate various accents and phonetic details, based on large datasets of recorded speech, further enhances the realism of the generated voices.
However, a note of caution is warranted. While technology can replicate vocal patterns, it's still debatable whether AI can capture the full range of human emotional expressiveness. For instance, capturing the subtle inflections that a human reader might use to emphasize a particular passage is still a challenge. The integration of cloud-based tools also facilitates remote collaboration, making the audio production process more flexible and responsive to changes in workflow or feedback. Ultimately, effective knowledge management systems are essential for large-scale projects. Having organized audio libraries and easily searchable information resources is crucial for quick access to past work, leading to faster turnaround times in future projects.
Voice Cloning AI Integration How Knowledge Management Systems Shape Enterprise Audio Production - Automatic Voice Synthesis Models Reshape Podcast Post Production Methods
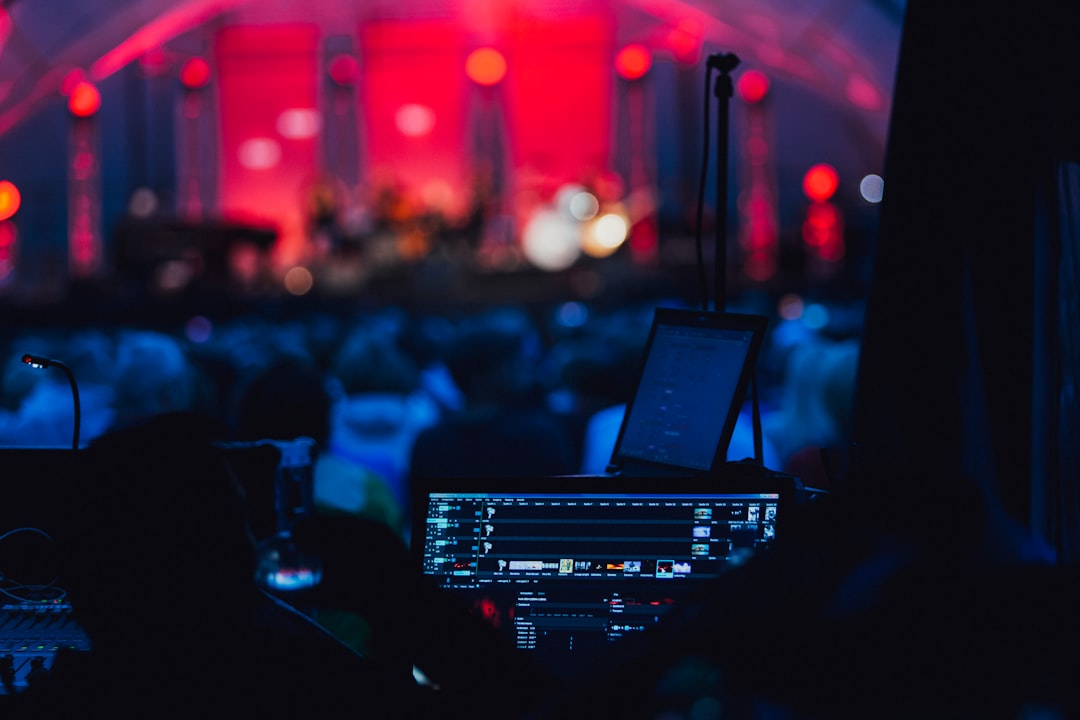
The emergence of automated voice synthesis models is dramatically reshaping how podcasts are produced after initial recording. These tools empower podcasters to create high-quality audio more efficiently, reducing the need for extensive manual editing. AI voice cloning, in particular, provides a compelling solution for maintaining consistent audio branding even if the original voice talent is unavailable. This can be beneficial for keeping a unique podcast feel. But, while technology improves, accurately replicating the subtle emotional variations of human speech remains a significant obstacle. These models struggle to fully capture the nuances that make human narration so compelling. The challenge for the future of audio production will likely be in finding the right mix between automated efficiency and the uniquely human quality of emotional storytelling in a voice.
The field of automatic voice synthesis is rapidly altering how podcasts are produced, especially in the post-production phase. We're seeing models that go beyond simply generating speech; they're becoming adept at mimicking the intricate syntax of multiple languages, which is a boon for podcasts targeting global audiences. This means audio can be tailored to specific linguistic nuances, making the synthesized voices sound more natural and less robotic.
Intriguingly, these models are also starting to incorporate a sense of emotional context. While still not perfect, they can analyze a script's tone and attempt to replicate matching emotions in the synthesized voice. This adds a new dimension to podcasting, potentially creating more immersive listening experiences where the audio mirrors the story's emotional landscape.
Furthermore, these AI systems are enabling real-time adjustments during the podcast recording process. Producers can now tweak the voice, changing its pitch, tone, or even accent on the fly. This opens up exciting possibilities for dynamic content, as producers adapt to feedback from their audience during recording.
The use of deep learning techniques, including Generative Adversarial Networks (GANs), is a driving force behind the increasing realism of these voices. We're at a point where distinguishing between human and AI-generated speech can be challenging in many scenarios. As these models continue to advance, the line between the two will likely blur further.
Another interesting application is the concept of voice preservation. Voice cloning can capture and retain a person's unique vocal characteristics for future use. This can be helpful for podcasts with a consistent host or narrator who, due to health reasons or other circumstances, might be unable to record new episodes. The AI can step in to maintain the show's distinctive voice, keeping the listener's experience consistent.
The speed and scalability of these systems are transforming content production. Companies can produce a large volume of audio without a corresponding growth in human resources, allowing for broader reach and consistent quality across diverse markets. It’s also opening avenues for creators to establish distinct brand identities through custom voices tailored to a specific brand or thematic focus.
We're seeing experimentation with augmented reality (AR) platforms, which may enhance podcast storytelling. The idea of integrating voice synthesis into AR experiences, creating more spatial and realistic auditory environments, is still in its early stages but has the potential to greatly enhance listener engagement.
Perhaps the most advanced models operate at a phoneme level, allowing them to replicate incredibly specific accents and dialects. This has broad implications for content creation, opening up new storytelling possibilities through a wider range of narrative voices.
However, the growing prevalence of voice cloning presents both exciting and complex ethical questions. Specifically, it raises concerns regarding intellectual property ownership and the protection of artists' unique vocal characteristics. These developments are likely to lead to shifts in copyright law as creators navigate the legal landscape of AI-generated audio. The balance between creativity and protection of individual work is a critical area for continued discussion.
Voice Cloning AI Integration How Knowledge Management Systems Shape Enterprise Audio Production - Voice Data Collection Standards Within Knowledge Management Databases
The development of voice cloning technology hinges on robust voice data collection standards, but a standardized approach is currently missing, hindering progress in voice AI research. As the use of voice technologies expands across various applications, like audiobook and podcast production, consistent methods for collecting and managing voice data are essential. Choosing the right audio data collection services is key to capturing a broad range of languages and dialects, ensuring that AI models can accurately represent the subtle variations found in human speech. The evolving nature of voice AI underscores the importance of establishing ethical guidelines and maintaining public trust, especially when voice data is used in sensitive contexts like healthcare. Overcoming these challenges will be vital for fully harnessing the potential of voice AI within knowledge management systems, fostering responsible and effective integration.
The current state of voice data collection lacks a universal standard for managing acoustic information, which hinders the broader use of these datasets for voice AI research. This is especially relevant in the growing fields of audiobook production and podcasting, where the need for high-quality, diverse, and emotionally nuanced synthetic voices is paramount. Finding an appropriate audio data collection service is vital, especially when considering the specific language and dialect needs of target audiences for applications like speech recognition and natural language processing in various content forms.
As voice-driven technologies become more prevalent, the way we collect audio data is changing. It is essential to have well-defined methods to ensure that we can foster innovation. The drive to improve voice recognition hinges on the effective collection and management of audio datasets. However, achieving that is challenging, with questions around ensuring high audio quality and replicating natural variations in human speech.
Integrating AI into knowledge management systems can enhance how businesses function and share information. This includes the development of voice cloning technology. Yet, as these technologies become more prevalent, particularly in sensitive fields like healthcare, concerns about ethical considerations and user trust rise. The use of voice AI as a non-invasive health indicator is gaining recognition, as it utilizes readily available tools like smartphones to collect voice data. This is creating new avenues for research, yet highlights the variability in data collection methods across different researchers and clinicians, demanding more standardization.
Given the increasing adoption of voice-enabled technologies, refining how we manage voice data is essential for driving future advancements in AI. As demand for these applications continues to grow, the realm of voice data collection is expanding, bringing about new challenges that need to be thoughtfully considered. It's important to understand these aspects, including questions of how we can incorporate emotion and cultural context into voice models, while also addressing issues like copyright and protecting unique vocal characteristics. Without this, the full potential of AI in audio production might not be realized.
For instance, the use of MFCCs offers a way to objectively assess the quality of a synthesized voice, crucial for ensuring that audiobook and podcast listeners perceive the audio as natural. Similarly, diverse datasets containing variations in dialect and speech patterns are important for creating voice models that resonate with wider audiences and improve accessibility. While we've seen progress in implementing emotional intelligence into voice synthesis, accurately capturing the nuances of human expression in speech remains a challenge, suggesting that achieving truly human-like communication through AI may take some time.
In addition, there is a need to ensure data privacy. Strong standards are required to guard against the unauthorized use of personal voice data as voice cloning technology develops. Feedback loops integrated into these systems allow for real-time adjustments based on listener reactions, offering the opportunity to fine-tune the audio experience. The advancement of phoneme-level processing enables AI models to analyze and reproduce individual speech sounds, potentially allowing for a level of detail that can create remarkably realistic synthesized voices.
Moreover, knowledge management systems are beginning to incorporate cultural factors into voice data collection. This aspect is crucial in creating voices that can resonate across different communities and languages, enhancing the reach and impact of AI in audio content. Voice preservation technologies rely on standardized data collection practices to preserve individual vocal qualities, ensuring that unique voices can be maintained for future content even if the original voice talent is unavailable. The rise of voice cloning is leading to intense conversations surrounding intellectual property and copyright, which will need to be addressed as this field advances.
Voice Cloning AI Integration How Knowledge Management Systems Shape Enterprise Audio Production - Neural Network Speech Models Enhance Audiobook Character Performance
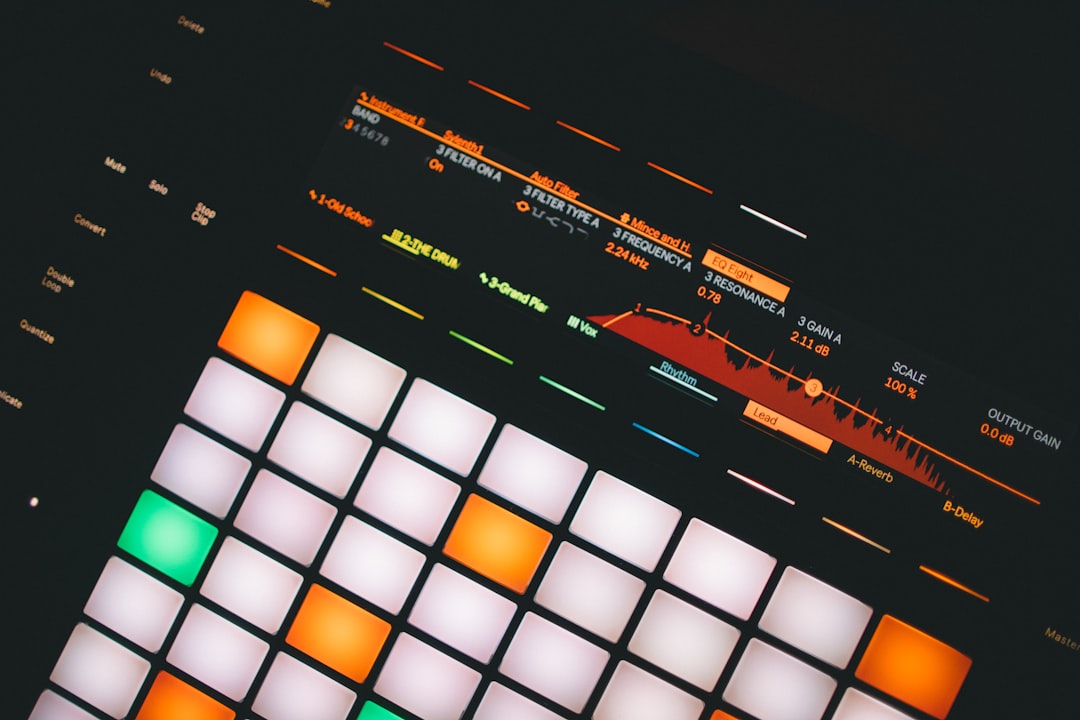
Neural networks are revolutionizing how characters sound in audiobooks by creating very realistic and expressive voice clones. AI models, like those based on WaveNet or Transformer architectures, are able to generate speech with a high level of fidelity, mimicking the subtle nuances of human voices. This means a narrator's unique style can be maintained even if they are not available for a project. The technology also allows audiobook producers to use consistent voices across multiple languages. However, there's a current limitation: accurately capturing the full range of human emotion, especially in crucial parts of a story, remains difficult. These models are still learning how to perfectly reflect the emotional depth that a human narrator brings to a performance. The challenge moving forward is to develop these neural network models further, refining their ability to accurately portray emotion in synthesized speech, ensuring audiobooks maintain a high level of engagement and emotional impact for listeners. The development of these voice cloning tools is not only altering the presentation of stories in audiobook formats but is pushing the overall creative possibilities in digital audio production as a whole.
Neural network models are becoming increasingly sophisticated in their ability to analyze vocal patterns and generate speech that captures the unique characteristics of different characters in audiobooks. This represents a significant shift from traditional methods, offering a potential path toward more engaging listening experiences. For instance, these models can now learn to mimic the subtle vocal nuances that define a character, creating a more immersive experience for the listener.
Research in neuroimaging suggests that different AI-generated voices can stimulate distinct regions of the listener's brain, similar to how human voices evoke emotional responses. This finding implies that AI may be achieving a level of sophistication in mimicking human vocal performances and could be effectively employed to enhance the emotional connection between the listener and the story being narrated.
Interestingly, these systems are also starting to incorporate machine learning to replicate the natural aging of a character's voice throughout a series of audiobooks. This helps maintain character consistency across multiple installments, which could contribute to a more cohesive narrative and improve the overall quality of the audiobook experience. The challenge with this, of course, is capturing the specific complexities of the natural human voice throughout aging.
AI voice models are increasingly incorporating dialectal variations into their synthetic speech, allowing them to accurately portray different regional accents. This is especially important for creating audiobooks that resonate with wider audiences, particularly for stories set in specific locations or when a character's identity is intrinsically tied to a specific regional dialect. This application of AI opens up new possibilities for increasing representation in audiobooks.
A fascinating trend emerging is that some listeners, particularly in genres like science fiction or fantasy, have expressed a preference for AI narrations over human ones. This suggests that the quality of AI-generated voices has advanced to the point where they can be a viable replacement for a human narrator in particular types of stories. Particularly, if characters have unusual vocal patterns or characteristics, an AI model can replicate them more easily and with less chance of error.
Current AI voice models are striving to incorporate emotional intelligence into their synthetic speech. By analyzing contextual cues within the written text, they can adjust their vocal intonations, pitch, and pace to match the emotional tone of the story. While still a developing area, this could significantly enhance the ability of AI to mimic human emotional expression in a narrative, creating a more nuanced listening experience.
Early studies show that AI-generated voices can maintain consistent vocal quality even in high-stress, emotionally intense scenarios, such as audiobooks requiring a narrator to convey excitement or urgency. This suggests that the models can potentially outperform human narrators in maintaining vocal consistency in challenging narrative environments. But, the quality of emotion and the resulting emotional effect on the audience is still up for debate.
A technique known as "voice morphing" allows AI voice models to seamlessly transition between multiple characters within a narrative. This effect is similar to the ability of a skilled actor to change their voice to play different roles in a theatrical production. It creates a layered storytelling experience with added depth and character differentiation within the audiobook. This presents significant technical hurdles, and the naturalness of the effect can be easily spoiled by simple inaccuracies.
Ongoing improvements in signal processing techniques have made AI-generated voices more pleasing to the human ear. These improvements ensure that the voices produced adhere to the psychoacoustic principles that influence how we perceive and process audio. This effort moves AI-generated speech closer to a natural, relatable human experience in its acoustic properties.
The ethical aspects surrounding voice AI are becoming increasingly complex. Recent studies have highlighted the potential for cloned voices to be used to spread misinformation or misrepresent individuals if not properly controlled. This has prompted calls for better regulatory frameworks for the use of this technology in audio production. This is a topic with deep roots in society, as we've seen with deep fakes for videos and photos.
Voice Cloning AI Integration How Knowledge Management Systems Shape Enterprise Audio Production - Real Time Voice Generation Tools Power Live Audio Productions
**Real-Time Voice Generation Tools Power Live Audio Productions**
The ability to generate voices in real-time is changing the landscape of live audio productions, bringing a new level of control and adaptability. AI-powered tools can instantly change or create a voice, allowing producers to maintain a consistent vocal style even during live events. This helps maintain a brand or character's specific sonic identity in situations where voice actors might not be readily available. These advancements make it easier to adjust and refine the audio output in response to audience reactions or changes in the production flow. Despite the exciting improvements, the technology's ability to fully replicate the subtle complexities of human emotions in speech remains a hurdle. AI-generated voices can sometimes sound artificial or fail to convey the desired emotional weight, especially in nuanced storytelling or emotional performances. It's a persistent issue that needs continuous research and innovative approaches, so that the field continues to develop and provides a truly enriching experience for the listener. The future of this technology hinges on finding the sweet spot where the benefits of automation are balanced by the irreplaceable human capacity for emotional expression in storytelling.
Real-time voice generation tools are transforming live audio production, particularly in areas like voice cloning, sound design, and podcasting, in ways we're only beginning to understand.
One fascinating aspect is how some newer models utilize spectral analysis to identify emotional cues within speech. By analyzing pitch patterns and frequency changes, they try to replicate the emotional delivery of human speakers, making synthesized audio more resonant and engaging. However, this quest for realism throws up new challenges, especially the issue of latency. In complex audio setups with multiple tracks or remote participants, achieving real-time voice generation without noticeable delays can be tricky. Balancing quality with speed is crucial for keeping listeners engaged.
Beyond audio production, the applications of AI-generated voices are expanding. We're seeing experiments where synthetic voices are being integrated into humanoid robots, not just to replicate human speech, but to capture subtle vocal patterns like hesitations or stutters, making these robotic interactions feel more natural and relatable. Furthermore, there's a growing interest in what's called vocal emotion transfer. Researchers are working on methods to transfer the emotional nuance of a human speaker's voice to synthesized speech. This could be a breakthrough in making AI voices feel more personal and less mechanical, potentially enhancing the listener experience.
It's interesting that real-time voice generation can even be integrated with live audience feedback. Imagine a system where the voice model adjusts its output based on the reactions of the audience, whether it's excited cheers or quiet moments. This ability to dynamically shift performance could open up exciting new possibilities for live interactions. Another interesting development is in how these models can now handle complex phonetic situations. Advanced systems can now generate smoothly overlapping sounds and coarticulations, mimicking how humans actually talk. This enhances the believability of these synthetic voices.
It's also worth noting the growing integration of real-time voice generation with 3D audio technology. Imagine listening to a podcast where the sounds seem to emanate from different points in space, creating a more immersive auditory environment. And there's the fascinating concept of voice fingerprinting – giving every cloned voice a unique acoustic signature. This technology could help us distinguish between authentic and synthetic voices in a world where cloning is increasingly common.
From a neuroscientific perspective, recent studies suggest that our brains can distinguish between AI and human-generated speech on a subtle level. Specific frequency patterns and modulations in AI-generated voices trigger different responses than naturally human voices. While the specifics of these responses are still under investigation, they underscore that the journey of perfecting AI-generated voices is a complex one.
As voice generation tools become increasingly commonplace, legal questions about cloning are coming into sharper focus. Issues like copyright, intellectual property, and even the rights associated with a public persona are raising complex questions that require serious debate between creators and lawmakers. This is a critical aspect for developing responsible and ethical standards in this field.
These insights reveal the wide range of applications and unresolved challenges surrounding real-time voice generation tools in live audio productions. This technology is undeniably powerful, yet it still has limitations and potentially far-reaching implications. It will be fascinating to see how these technologies continue to evolve and impact the future of audio creation.
Voice Cloning AI Integration How Knowledge Management Systems Shape Enterprise Audio Production - Voice Sample Processing Methods Improve Studio Recording Quality
The methods used to process voice samples have greatly improved the quality of recordings made in studios. We're now able to capture audio with much higher fidelity thanks to things like the widespread adoption of 48 kHz sampling rates and 24-bit depth. It's noteworthy that AI techniques, specifically deep learning algorithms and advanced noise reduction models, are able to refine even lower-quality audio to a standard that's comparable to professional studio recordings. This enhanced processing makes voice cloning more accessible and efficient. AI can now replicate a person's voice very realistically using relatively small amounts of audio, which accelerates the workflow. These advancements are making a noticeable difference not only in audiobook and podcast creation but also raise fascinating questions regarding the extent to which AI-generated voices can accurately convey the subtleties of human emotion and the authenticity of a speaker's voice. While these tools hold promise for making audio production faster, we still need to think about the trade-offs in terms of how natural the voice sounds and whether it captures the genuine emotional expression humans bring to storytelling.
The field of voice synthesis is making significant strides in replicating the nuances of human emotion through advanced spectral analysis. By scrutinizing the frequency and pitch variations within speech, AI systems are now able to create synthesized voices that convey a range of emotions, like joy or sorrow, moving beyond the traditionally robotic sound. While this is encouraging, applying these methods in real-time, particularly in live audio productions with multiple audio feeds, introduces the challenge of managing latency. Even minor delays can be disruptive, highlighting the ongoing need for balance between speed and accuracy in the generation process.
Interestingly, there's a growing emphasis on security within this evolving field. Voice fingerprinting is emerging as a powerful tool to differentiate human and AI-generated voices by assigning a unique acoustic fingerprint to each cloned voice. This is becoming increasingly relevant in a world where voice cloning is increasingly prevalent, offering a layer of authentication and trust in the authenticity of audio.
Moreover, recent neuroscience research is shedding light on how our brains process AI-generated versus human speech. Studies have found that specific acoustic characteristics of AI voices trigger different neural responses compared to natural human voices, though the exact mechanisms are still under investigation. This insight holds promise for a deeper understanding of how we perceive and emotionally engage with synthesized audio.
We are also witnessing the rise of dynamic audio experiences, where AI voice models can respond in real time to the reactions of a live audience. This presents an exciting prospect for creating interactive audio experiences, with the synthesized voice adapting and evolving during a performance based on listener responses.
Furthermore, improvements in AI models are allowing for a more natural replication of speech, with systems now capable of generating smoothly blended sounds and complex coarticulations—the way our mouths and vocal cords naturally transition between sounds when speaking. This enhances the realism of AI-generated voices and makes them sound less artificial.
The capacity of AI to smoothly shift between different character voices, known as voice morphing, is becoming increasingly sophisticated. It allows for a greater depth of characterization in audiobooks without the need for multiple voice actors. This technique mimics the natural talent of a human performer seamlessly adapting to various roles.
Research hints that well-crafted AI voices can trigger similar emotional responses in listeners as human voices do. This suggests that AI models are beginning to tap into the cognitive mechanisms behind human emotional responses to audio, hinting at a future where truly engaging and meaningful connections can be fostered through AI-narrated stories.
Another intriguing application is the ability to simulate aging within a character's voice over a series of audiobooks. AI models can replicate the gradual changes in a voice associated with growing older, creating a consistent and nuanced experience for the listener across multiple installments of a narrative. This, however, presents a challenge in truly capturing the complexities of aging in human speech.
Unexpectedly, certain listener demographics, particularly those who favor genres like science fiction or fantasy, have shown a preference for AI-generated narration over human performers. This fascinating trend raises questions about the evolving roles of both human and AI narrators in the future of storytelling and suggests that there are particular niches where AI-generated voices excel.
While the technological advancements in voice synthesis are exciting, they also raise complex questions regarding ethics and the role of human artists. As AI-generated voices become increasingly sophisticated, we need to continue to investigate how these technologies impact the human experience and the creative landscape of audio.
More Posts from clonemyvoice.io: