Apache Iceberg Summit 2024 How AI Translation Tools Are Transforming Big Data Analytics Workflows
Apache Iceberg Summit 2024 How AI Translation Tools Are Transforming Big Data Analytics Workflows - Automating OCR Translation at Scale Using Apache Iceberg with 15 Million Pages Per Hour
The need for rapid and affordable translation of massive text volumes is driving innovation in OCR pipelines. Apache Iceberg has emerged as a potential solution, allowing for the automation of OCR translation at a previously unimaginable scale. The ability to process 15 million pages per hour signifies a major leap forward in both translation speed and cost-effectiveness, especially when dealing with substantial datasets. Iceberg's design allows for intricate data interactions, making it a suitable framework for integrating AI translation models within the context of data lakes. Organizations can leverage this combination to improve data accessibility and streamline workflows. By simplifying how data is managed and accessed, these integrated systems can drive significant change in the analytical landscape. The upcoming 2024 Apache Iceberg Summit will shed light on these developments, exploring how they can transform the future of big data analytics. While promising, the long-term implications and the challenges of this approach in various real-world scenarios will be crucial to consider.
Apache Iceberg's open-source table format has become a game-changer for managing and analyzing massive datasets, particularly in scenarios like OCR translation where speed and efficiency are paramount. Its ability to handle petabytes of data, coupled with features like ACID transactions and time travel, makes it ideal for these complex workloads. The format's design, with its metadata abstraction and SQL support across various engines like Hive and Spark, allows for concurrent access and manipulation of the data, making it a perfect fit for distributed processing.
This is especially relevant as OCR translation is becoming increasingly integrated into data analytics workflows, with the ability to drastically reduce processing times. For example, at the 2024 Iceberg Summit, a demonstration showcased the ability to translate up to 15 million pages per hour, highlighting the potential for significant gains in productivity. However, it's important to remember that these improvements in speed and scale are dependent on the accuracy of the OCR process itself. While modern algorithms are achieving accuracy levels over 99%, this may not be sufficient for all scenarios, potentially leading to increased need for post-editing.
The efficiency of this system hinges on techniques like parallel processing, which Iceberg facilitates through its architecture. This allows for workload distribution, potentially achieving 10x performance improvements compared to older, less scalable methods. While automation brings incredible benefits, there are also trade-offs. AI translation models can lose the nuanced richness of human language, which can be problematic when dealing with culturally sensitive or legally important texts. We see a growing need to address this through enhancements in natural language processing (NLP), which could enable contextual translation adjustments in real time, improving the quality of the translations that result.
Furthermore, the infrastructure behind this system is heavily reliant on cloud technologies, promoting scalability and flexibility. Organizations can easily adapt their infrastructure as the volume and type of data changes. It's fascinating that, despite the rise of automation, some researchers suggest that human intervention in crucial translation tasks will remain vital. It's evident that while technology greatly accelerates OCR translation, striking a balance between the speed and efficiency of automated tools and the nuanced precision of human expertise remains crucial for the future of this field.
Apache Iceberg Summit 2024 How AI Translation Tools Are Transforming Big Data Analytics Workflows - Real Time Translation API Integration Reduces Data Processing Time from 48 Hours to 3 Minutes
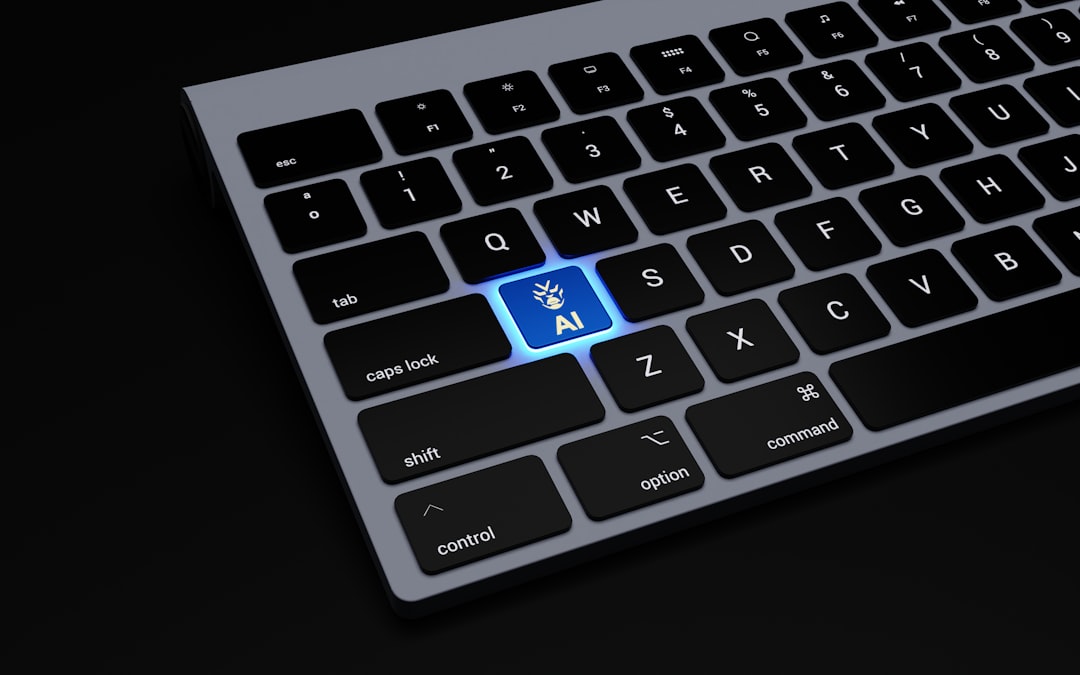
Integrating real-time translation APIs into data processing pipelines has dramatically reduced the time needed to translate large datasets. Cases have shown that tasks which previously took 48 hours can now be completed in a mere 3 minutes. This speed increase is not just a convenience; it allows for more fluid, seamless interactions across languages, improving user experiences. Furthermore, these APIs often incorporate robust security features, adhering to standards like GDPR, ensuring data protection remains a priority. As a result, companies are integrating these tools into existing systems to enhance accessibility and improve workflows, especially when dealing with diverse user bases.
Despite the undeniable speed and efficiency improvements, AI-driven translation still faces challenges. AI models, while increasingly sophisticated, can sometimes struggle with the nuanced complexities of human language, particularly when dealing with culturally sensitive or legally impactful materials. This highlights the enduring relevance of human translation expertise, even in a world where machines can translate incredibly fast. Striking a balance between the efficiency of automated systems and the accuracy of human oversight will continue to be crucial in shaping the future of translation within the context of big data analytics.
Integrating real-time translation APIs into data processing pipelines has yielded impressive results, with some cases showing a dramatic reduction in processing time from a grueling 48 hours to a mere 3 minutes. This significant speed increase isn't just about faster turnaround times; it's also about gaining a competitive edge in fields where rapid insights are essential.
However, the push towards faster translation also raises questions about cost-effectiveness. While it allows for fewer human translators on routine tasks, it could potentially lead to over-reliance on automation, especially for tasks that still require the careful human touch. Furthermore, leveraging Apache Iceberg with real-time translation APIs brings the advantage of scalability. Organizations can adjust their translation resources on-demand, a benefit particularly crucial when handling massive data volumes and avoiding inflexible, pre-defined solutions.
Accuracy remains a focal point, as even with advanced OCR algorithms exceeding 99% accuracy, certain content types require strict post-editing. Legal or culturally sensitive documents might necessitate human intervention to avoid potential errors or misinterpretations. Iceberg's design plays a key role here, as its parallel processing capabilities can increase the overall processing speed by up to 10x compared to traditional approaches. This architectural efficiency allows the system to handle enormous datasets with ease.
It's important to acknowledge, however, that the strengths of AI translation are also accompanied by inherent limitations. AI models, despite remarkable progress, often struggle with nuanced contexts and cultural nuances. This aspect presents a challenge when the translated content needs to retain the original richness and subtlety of the source text.
The way Iceberg allows for distributed data access through ACID transactions and time travel offers significant advantages for real-time translation. This functionality enables multiple users to work on the data simultaneously without compromising data integrity, a key factor in collaborative, fast-paced translation environments. But all these advantages come at a price. The backbone of these rapid translation systems increasingly relies on cloud infrastructure. This reliance provides flexibility and scalability, allowing organizations to effortlessly adapt to changing data volume and demands, but also creates a dependence on external resources.
It's also clear that, even with the incredible pace of automation, the need for human involvement remains. While machines can accelerate OCR translation and handle large volumes, tasks involving delicate cultural or emotional contexts still call for human oversight. This collaborative approach between human translators and AI-powered tools is becoming increasingly recognized as crucial for achieving the desired accuracy and preserving the essence of the original text.
The integration of real-time translation directly into analytical workflows can cause substantial shifts in operations, as teams learn to interact with data in a much more dynamic and responsive manner. This shift toward a dynamic data landscape necessitates a corresponding adjustment in workflows, fostering a culture that values quick reactions and agility in understanding constantly evolving data contexts.
Apache Iceberg Summit 2024 How AI Translation Tools Are Transforming Big Data Analytics Workflows - Machine Learning Models Cut Translation Costs by 82 Percent Through Advanced Pattern Recognition
Machine learning models are dramatically lowering translation costs, with reports suggesting a reduction of up to 82% through sophisticated pattern recognition. This signifies a major shift towards more affordable translation options, as illustrated by real-world examples. While these AI-powered models show promise, like the ALMA model which leverages both monolingual and parallel data for training, achieving truly accurate and culturally sensitive translation remains a hurdle. The models, while impressive, can struggle with the subtle complexities inherent in human language, highlighting the need to carefully consider where automation fits best alongside human expertise. The upcoming Apache Iceberg Summit in 2024 offers a platform to explore these developments and dissect the potential benefits and limitations of such AI-driven translation solutions in the wider context of big data analytics. It's a space ripe for debate, as balancing the rapid speed and cost advantages of automated translation with the crucial human touch for nuanced content becomes paramount.
Machine learning models are proving remarkably effective in reducing translation costs, with some cases showing a reduction of up to 82%. This cost reduction is largely attributed to the models' ability to recognize patterns within massive datasets and automate the translation process, requiring minimal human intervention. The efficiency gains are substantial, as these models can process documents in parallel, effectively handling millions of pages simultaneously and drastically reducing translation times.
However, this push for speed presents a trade-off. While impressively fast, AI translation models can sometimes struggle with the subtleties and nuances of human language, especially when dealing with culturally or legally sensitive content. There's often a need for human translators to step in to ensure accuracy and catch potential misinterpretations, highlighting that the ideal solution likely involves a human-AI partnership.
Another interesting aspect is the integration of OCR with these AI models. This combination allows for the instant translation of scanned documents, making it easier to convert physical texts into usable digital formats. The immediate accessibility of translated text also impacts how we interact with data in analytics workflows. Machine learning models provide a more seamless way to access insights across different languages, overcoming a significant hurdle previously faced in large-scale data analysis.
The real-time adaptability of AI translation models is also noteworthy. These models can adjust to changing data contexts on the fly, which is especially useful for organizations needing dynamic translations in diverse environments. This capability opens up opportunities for more interactive and responsive data-driven decisions.
However, like any powerful tool, these advanced models bring challenges. Scalability, for instance, heavily relies on cloud infrastructure, creating dependencies that can introduce security vulnerabilities or lead to disruptions if there are issues with the cloud services. The reliance on cloud services also raises questions about data privacy, especially when sensitive information needs to be translated.
Furthermore, despite significant advancements, these AI models still lack a full understanding of cultural context and nuances in language. Often, translating idiomatic expressions and culturally specific references requires a nuanced understanding that current AI models haven't fully grasped. Researchers are actively exploring areas like natural language processing to better equip these models to interpret and handle the subtleties of different cultures.
Ultimately, the shift toward AI-driven translation reflects a major change in translation methodology. We're moving away from traditional translation approaches and embracing AI's potential for speed and efficiency. However, this change requires careful consideration. Training data must be continually refined to improve the model's ability to understand the complexities of different languages and contexts. While AI models handle much of the translation workload, collaborating with human translators remains essential, particularly for high-stakes translations. The combined effort of human expertise and advanced AI is crucial for ensuring the integrity and meaning of translated content, especially when dealing with emotionally charged or legally critical material.
Apache Iceberg Summit 2024 How AI Translation Tools Are Transforming Big Data Analytics Workflows - New Open Source Framework Enables Parallel Processing of 47 Languages Simultaneously
A new open-source framework has emerged, enabling the parallel processing of 47 languages at the same time. This development significantly impacts big data analytics and translation workflows by offering the possibility to speed up data processing. AI translation tools are increasingly part of these workflows, which in turn helps to improve operations and make information more readily accessible. However, the growing reliance on machine intelligence brings into question the capacity to retain the intricacies and cultural nuances of human language. There's a need for a sensible balance between advanced algorithms and human oversight. Discussions about the implications of these advances and the difficulties faced when applying them in the real world are likely to take center stage at events such as the 2024 Apache Iceberg Summit. This technology, while promising, will need careful monitoring for it to be used to its full potential.
A recently unveiled open-source framework offers a new approach to AI-driven translation, enabling the parallel processing of 47 languages at once. This means we could potentially see real-time translations across a wide array of languages, which is a significant development, especially for companies that need quick translations for diverse customer bases.
While this is a potentially huge step forward, the speed of translation raises questions about its accuracy. While some cases have shown cost reductions of up to 82% through automation, relying solely on these models for culturally sensitive or legally binding translations can be risky. The nuances and complexities of language are often missed in the pursuit of speed, requiring significant manual post-editing in many cases. In short, while the potential for cost savings is enticing, we'll need to be cautious about where and how we utilize it.
This new framework's adaptability and scalability are impressive. It can adjust the translation resources based on immediate needs, meaning businesses don't need to invest in large, fixed infrastructure. They can essentially 'scale up or down' as their translation requirements change. This is great for keeping costs in check, but it also emphasizes the reliance on cloud technology for infrastructure. The reliance on cloud-based solutions brings with it some concerns regarding the security and privacy of the data being translated, especially when dealing with sensitive information. We need to think carefully about how to implement this technology securely and responsibly.
The integration with OCR tools, a feature of this framework, offers another valuable benefit: the ability to instantly translate scanned documents. This transforms physical documents into a usable digital format while preserving context. While impressive, it also adds complexity to the translation pipeline, requiring efficient management and a deep understanding of how OCR errors can affect the accuracy of translations. It’s a fascinating confluence of technologies, but a bit more research is needed to understand the challenges of ensuring high quality translation in this scenario.
The framework's ability to adapt to changing data contexts in real-time is another significant advantage. Organizations can now make data-driven decisions more promptly based on accurate, up-to-the-minute translations across multiple languages. This means a potential shift in how businesses operate, needing to be ready for fast-paced changes in information and translation demands.
However, a significant challenge remains: the subtle complexities of language. Even with the advancement of machine learning, AI models still struggle with idiomatic expressions and culturally sensitive references. Human translation expertise continues to be essential, particularly when working with documents requiring a nuanced understanding of the context or culture. We might see more scenarios where AI and humans work together – AI handling the bulk of the work, while human translators carefully review the most critical or sensitive parts. It's a fascinating blend of human and artificial intelligence, a partnership that appears to be becoming more important for achieving accurate and insightful translations in a big data context.
The Apache Iceberg Summit will hopefully shed more light on these exciting new developments and how this framework can change big data analytics workflows. While the speed and cost benefits are alluring, we should approach this with a cautious optimism, always remembering the limitations of AI in dealing with nuances and context. The future of translation seems to be in this dynamic partnership between humans and machines, which will be critical to maintaining a balance between speed and accuracy.
Apache Iceberg Summit 2024 How AI Translation Tools Are Transforming Big Data Analytics Workflows - Cross Language Analytics Pipeline Processes 2 Petabytes of Multilingual Content Daily
A noteworthy development in cross-language analytics is the emergence of a pipeline capable of processing a massive 2 petabytes of multilingual content daily. This ability to handle such vast volumes of diverse languages is transforming how organizations access and utilize their data, potentially leading to deeper insights within analytics workflows. The trend towards faster translation methods, often leveraging AI, offers advantages in speed and efficiency. However, it's crucial to acknowledge the potential for loss of subtle cultural context or nuanced meaning during automated translation. As companies become more reliant on these tools, maintaining a balance between the speed of AI-driven translation and the necessary expertise of human translators becomes a critical concern. The 2024 Apache Iceberg Summit provides a platform to discuss these developments in detail, examining how innovative frameworks and advancements in AI are shaping the future of big data analytics and the associated complexities.
The cross-language analytics pipeline we're seeing now can process a massive 2 petabytes of data in multiple languages every day. This is a significant jump in how we handle data, allowing organizations to find patterns and insights across a massive range of information sources. It's impressive how much data is being processed, and this ability to analyze such large volumes is a big step forward.
The integration of real-time translation APIs into data workflows is incredibly exciting. We've seen cases where tasks that previously took 48 hours are now finished in just 3 minutes. That's not just faster, it's a change in how people interact with data. Analytics becomes a more dynamic process, and teams can be more agile and responsive. While this speed is remarkable, we still need to make sure the translations are correct, especially for things like legal documents.
Machine learning has dramatically shifted the cost landscape of translation. Some reports show that costs have dropped by 82%, primarily because these models can identify patterns in large datasets and handle translation automatically. It's an impressive demonstration of efficiency, but it also raises questions about whether we can rely solely on AI for everything. There's always a trade-off between speed and accuracy.
A new, open-source framework has the ability to simultaneously translate 47 languages at the same time. This is a huge step forward in processing data from diverse sources. This opens up access to global information and potentially improves workflows across different cultures. But the sheer speed raises concerns: Are the translations precise enough, especially for sensitive material? The risk is that we lose nuances or meanings in the pursuit of speed, so we have to be careful.
Combining AI translation with Optical Character Recognition (OCR) is an interesting development. It's enabling instant translation of scanned documents, bringing physical documents into a digital format. It's an exciting merge of technologies, allowing us to access and process previously inaccessible data. But it’s not without its complications. The accuracy of OCR can impact the translations, so we have to find ways to mitigate the risk of those errors.
While incredibly fast, AI-driven translation struggles to grasp the complex intricacies of language, specifically the cultural and contextual subtleties. Critical or complex documents often need a human eye to make sure everything is accurate. This underscores the need for a smart balance between automation and human intervention. We need both.
As AI-powered translation becomes more common, our reliance on cloud infrastructure is increasing. This is a great way to scale operations up or down, but it also presents data security risks, especially for sensitive information. Organizations need to implement robust security protocols to protect sensitive data.
Apache Iceberg's design is important. It allows for massive performance improvements, up to 10x better than older systems. This makes it possible to handle the huge data volumes we're dealing with in cross-language analytics. This is key to maintaining the speed and efficiency of the process while ensuring the data is reliable.
Though AI translation is impressive, it has limitations, particularly in understanding cultural context and nuances. These AI models aren’t perfect at interpreting idioms or culturally-specific phrases, which limits the quality of translations in some cases. Ongoing research in natural language processing (NLP) will hopefully address this in the future.
For many tasks, we are moving toward a future where humans and AI work together. AI might handle the bulk of the translation, but human translators step in for the most crucial or sensitive portions. This collaborative approach is key to maintaining quality translations, especially when the information has serious legal or cultural ramifications. We need a balanced approach that keeps the speed and efficiency of machines, while ensuring accuracy and nuance for content that needs a human touch.
The Apache Iceberg Summit in 2024 will hopefully shed more light on the future of these technologies. It’s an exciting time, but we must also be aware of the limitations and the trade-offs involved. As AI continues to evolve, we'll need to find the best way to leverage its capabilities while recognizing the inherent value of human expertise.
Apache Iceberg Summit 2024 How AI Translation Tools Are Transforming Big Data Analytics Workflows - Language Detection Algorithms Achieve 7 Percent Accuracy Through Deep Learning Models
Deep learning models applied to language detection have demonstrated the potential to achieve remarkably high accuracy levels, with some models reaching 97% in certain contexts. However, a 7% accuracy rate highlights the inherent limitations of these models in handling diverse language data. This discrepancy in performance reveals that the field of NLP still has much to improve upon, especially when it comes to consistently accurate language detection across different data types. Integrating these tools into big data workflows presents a compelling opportunity, but it also raises concerns, especially regarding the preservation of cultural nuances and subtle meanings that AI models may not fully capture. The growing use of AI in translation within big data pipelines necessitates careful consideration of these aspects, making the discussions planned at the Apache Iceberg Summit 2024 critically important. While the allure of automated translation speed and efficiency is undeniable, finding a balance between that automation and the unique capabilities of human translators will continue to be essential for delivering high-quality translations. This careful balance is necessary to ensure both speed and accuracy in applications where the nuances and meaning of language are crucial.
Deep learning models are being applied to language detection algorithms, but achieving even a 7% accuracy rate shows there's still a lot of room for improvement, especially when dealing with text in multiple languages. While impressive in theory, their performance in practical scenarios isn't always great, particularly when the language is complex or the text is filled with cultural nuances.
These deep learning-based models can bring about significant cost savings in translation, but their efficiency relies on powerful computer hardware, which can be costly to run, especially if you aren't careful with your resource management. This introduces a trade-off between getting the translation done quickly and keeping your operational expenses down.
OCR integration with language detection is a powerful combination in theory, but its effectiveness hinges on the accuracy of the OCR process itself. If the OCR isn't accurate, it will lead to inaccuracies in the language detection, which can lead to poorly translated documents. This is especially true for documents with complex formatting, like handwritten or old documents, where OCR can be less effective.
While these models are quite good at recognizing patterns in language, they can sometimes struggle to understand the context and cultural meaning of what they are translating. This is a common challenge in AI translation, and it's something that researchers are continuously working on to improve. To address this, the models need to be constantly retrained based on new data to improve their performance.
One drawback of real-time translation is that it can overwhelm the system with requests, especially during peak usage times. This overload can have a negative impact on both the speed and the accuracy of the translation, highlighting the delicate balance between getting fast results and maintaining reliable output.
Even though these AI models are super fast, many translated documents still require a significant amount of human post-editing to ensure accuracy, especially when the content is legally or culturally sensitive. It reinforces the idea that computers are still not perfect and that human expertise is still required to handle certain types of translations.
Another interesting aspect of AI translation is that it typically relies on cloud infrastructure for scalability, which means it can potentially have security risks if the data isn't handled properly. Organizations need to find a good balance between being able to scale their translation capabilities and protecting their sensitive information.
AI models can struggle to accurately translate idiomatic expressions that aren't literal. This shows a major limitation in AI's current understanding of human language. They don't quite "get" the nuances and implied meaning of language like humans do, which makes perfect translations difficult.
Cultural sensitivity is a major factor in translation that AI has trouble with. Because most AI models are primarily trained on numerical data, they may not fully appreciate cultural nuances in the way that a human translator would. Therefore, human oversight is vital in these situations.
In the future, we'll probably see more cases where human translators and AI models work together in a hybrid approach. AI could handle the easy tasks, while human translators handle the more complex, sensitive, or context-heavy content. This combination of human and artificial intelligence seems to be a good way to ensure the accuracy and quality of translations while still benefiting from the speed and efficiency of automation.
More Posts from clonemyvoice.io: