Get amazing AI audio voiceovers made for long-form content such as podcasts, presentations and social media. (Get started for free)
The Neuroscience of Voice Cloning How AI Mimics Human Vocal Flexibility
The Neuroscience of Voice Cloning How AI Mimics Human Vocal Flexibility - Neural Networks Decoding Speech Patterns
Neural networks are increasingly adept at deciphering the intricate patterns of human speech, leveraging the power of deep learning to convert brain signals into understandable language. This capability holds immense potential for individuals struggling with speech disorders, as it offers a pathway to restoring vocal communication through brain-computer interfaces. Interestingly, the structure of these networks seems to echo the intricate processes of our auditory system, implying a reciprocal link between neural activity and the generated speech. However, obstacles persist in deciphering the complex codes representing speech and capturing the subtle nuances of human communication. As research progresses, the capacity to decode and synthesize speech directly from brain activity has the potential to revolutionize voice cloning and audio experiences, impacting fields ranging from clinical rehabilitation to artistic endeavors.
Neural networks are increasingly being used to decode the complex patterns embedded within human speech. This involves breaking down the raw audio into fundamental components, like phonemes and intonation, essentially dissecting the unique fingerprint of a speaker's voice. It's through this intricate analysis that highly realistic voice clones become possible, preserving the nuances of an individual's speaking style.
Researchers are finding remarkable similarities between the computational processes of neural networks designed for speech coding and the way our brains process audio signals directly from the brain. It's fascinating to see how these artificial networks are mirroring what happens in the human brain, albeit at a very simplified level. Some frameworks employ loop-based structures that analyze speech through the lens of vocal tract movements, but these methods often suffer from interpretability limitations and rely on high-dimensional data representations that can be complex to interpret.
Current methods for neural decoding are opening up avenues for translating brain activity into audible speech. While still in its early stages, the concept holds immense clinical promise, potentially offering new avenues for individuals who have lost their ability to speak. It's inspiring to see how technology is moving towards improving communication in such a fundamental way.
Interestingly, these neural decoders can convert electrocorticographic signals—electrical activity from the brain's surface—into comprehensible speech parameters. This information is then fed into a synthesizer that generates a spectrogram, creating the auditory equivalent of the brain's signal. This gives us insights into how neural activity might be related to spoken communication, but further research is needed to fully unravel this complex relationship.
Furthermore, studies have revealed a strong correlation between how neural networks represent speech and the patterns of neural activity observed in the auditory system. This ranges from the auditory nerve to the regions of the brain specialized for processing language. The consistency of these relationships provides evidence for the value of these models in understanding how the brain decodes sounds.
It's equally noteworthy that unsupervised models – ones that are not explicitly guided by labeled data – have achieved comparable results to their supervised counterparts. This suggests a latent capability in the networks to extract meaning directly from raw speech. This capability holds substantial potential for future developments in various speech processing applications.
Research on speech envelopes, which essentially represents the overall amplitude of speech, has helped us understand how intelligibility affects brain responses. We are learning, for example, that inferring direct causal links between neural activity and speech perception is challenging. Specifically, using noncausal weights in these models can lead to misinterpretations.
Our brains process language in a remarkably efficient manner by rapidly interpreting the different ways phonemes are realized in actual speech. However, neuroimaging studies have not yet consistently shown how our brains maintain sub-phonemic detail over time. The process by which speech is encoded at these granular levels remains a key question.
One possibility is that the cognitive load of the listening task itself can influence how much subphonemic detail is maintained. This points to a remarkable flexibility in the brain's ability to adjust its processing based on the needs of the situation. The process is clearly more complex than a simple one-to-one mapping, hinting that there are still significant complexities to unravel.
The Neuroscience of Voice Cloning How AI Mimics Human Vocal Flexibility - Acoustic Signature Analysis for Emotion Detection
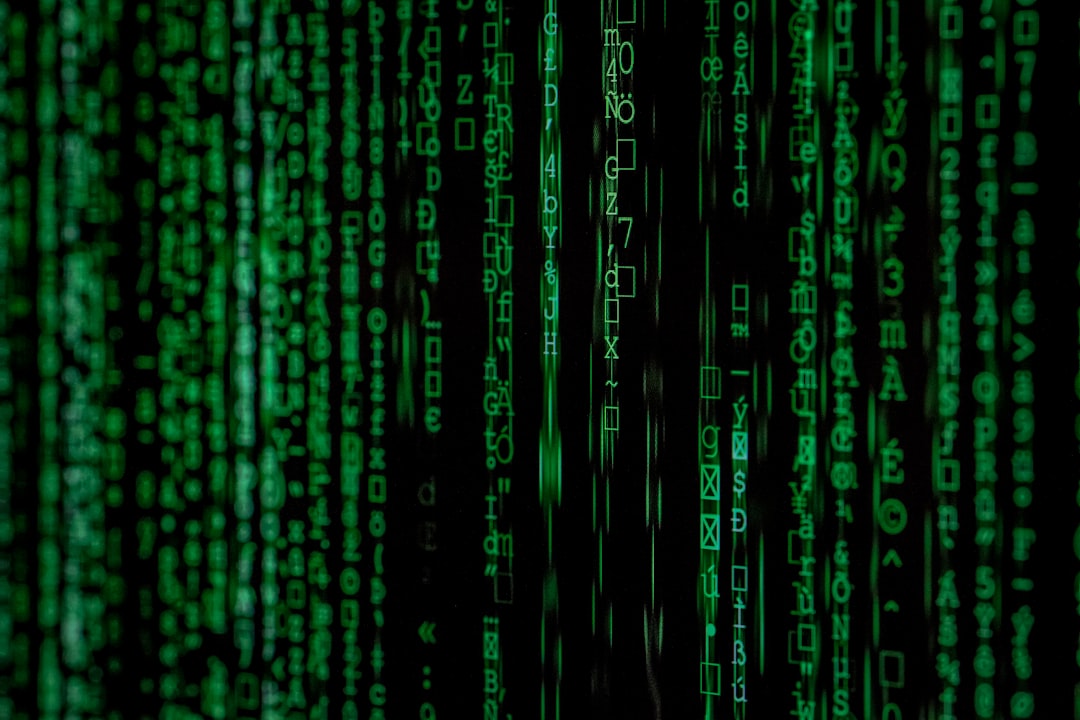
Acoustic Signature Analysis for Emotion Detection delves into how our voices reveal our emotional states. By analyzing sounds devoid of meaning, researchers can pinpoint the acoustic cues that signal emotions, such as variations in pitch, volume, and the rhythm of speech. Deep learning models, particularly deep neural networks, are being employed to decipher these patterns, achieving impressive accuracy in recognizing emotions—almost as well as humans. This process unveils the intricate connection between the physiological aspects of voice production and the expression of feelings. However, the inherent variability in how people communicate emotionally through their voices adds another layer of complexity. Every individual's vocal style is unique, making the task of universal emotion detection from voice alone quite challenging. Despite this complexity, the advancements in this field hold significant potential for shaping the future of human-computer interaction, enabling machines to better understand and respond to our emotional landscape.
The ability of humans to discern emotions from just the sound of a voice is quite remarkable, with accuracy often surpassing 80% in studies. This suggests that subtle shifts in acoustic features like pitch and tone can profoundly influence how listeners perceive the speaker's emotional state, highlighting the intricate nature of emotional communication through sound. Research has revealed connections between specific voice attributes and various emotions. For example, a higher pitch might be associated with fear or excitement, whereas a lower pitch could indicate sadness or calmness. These patterns demonstrate how the way we modulate our voice plays a key role in expressing our feelings.
Just like subtle facial movements can betray hidden feelings, fleeting alterations in the voice, known as "vocal micro-expressions", can convey emotional nuances that are sometimes difficult to consciously control. These incredibly brief changes, sometimes lasting only milliseconds, are particularly important for conveying complex emotions like sarcasm or anger, emphasizing the sophisticated interaction between acoustics and emotional signaling.
Studies using brain imaging have shown that the same neural pathways involved in processing emotions are closely related to those that process vocal features like intonation. For instance, the amygdala, a brain region associated with processing emotions, becomes more active when exposed to emotionally charged vocal stimuli. This highlights a biological basis for how sound can impact emotional cognition.
The field of psychoacoustics provides insights into how the brain interprets sound characteristics like frequency and loudness to understand emotional content. It appears that this process can happen extremely fast, often within just 100 milliseconds of a sound starting. This suggests that the human brain is effectively pre-wired to quickly make emotional assessments from auditory cues.
Interestingly, how we perceive emotion through voice can be influenced by cultural differences. For example, people from diverse cultures might assign different emotional meanings to the same acoustic cues, indicating the importance of context in interpreting emotional communication through sound.
Voice cloning technology is aiming to not only replicate a speaker's voice, but also the emotions they convey. Achieving this level of realism demands intricate algorithms that can accurately analyze and reproduce the nuances of emotional expression, a task that presents considerable challenges.
Researchers are exploring the potential of acoustic signature analysis in mental health settings to detect emotional states through vocal cues. Voice changes may provide early indicators of psychological distress, potentially enabling timely interventions.
Voice modulation and sound design techniques are already being utilized by professionals in audio production to create more impactful experiences in podcasts, audiobooks, and other media. Altering pitch, tempo, and reverberation can significantly impact a listener's emotional response, revealing the power of sound design to manipulate emotions.
The development of truly effective AI-driven voice cloning hinges on incorporating emotional data into the synthesis models. The goal is to create clones that not only replicate the speaker's voice, but also the emotional nuances they typically convey. This will hopefully improve the overall impact of cloned voices, enhancing the listener's emotional engagement with the content.
The Neuroscience of Voice Cloning How AI Mimics Human Vocal Flexibility - Data Collection and Synthetic Audio Generation
In the realm of voice cloning and synthetic audio generation, data collection is paramount. Deep learning models rely on extensive audio datasets to learn the intricate patterns of human speech and replicate them. These datasets can range from audiobooks and podcasts to conversational recordings, providing a diverse representation of the speaker's voice. While previously requiring hours of recordings, advancements have enabled high-quality voice cloning from surprisingly short audio snippets, which poses unique challenges related to potential for misuse and authenticity concerns.
Generating truly lifelike synthetic voices is further complicated by the fact that human vocal production is incredibly variable. Each individual's voice carries unique characteristics, and conveying emotions through sound introduces an additional layer of complexity. Developing AI models that can accurately capture and reproduce the nuances of emotional expression in a synthetic voice necessitates complex algorithms capable of deciphering subtle variations in acoustic features like pitch, tone, and rhythm.
As synthetic audio generation becomes increasingly sophisticated, the ethical considerations surrounding its use become more pronounced. Balancing the benefits of innovative voice technology with the need to prevent its malicious application, particularly in disseminating misinformation, is a crucial aspect of the field's future development. The creation of tools to identify synthetic voices is thus gaining momentum as the need to verify the authenticity of audio content becomes increasingly important.
Voice cloning, the art of synthesizing a voice that closely mirrors a specific person's, relies heavily on the intricacies of human sound production. Replicating the subtle nuances of a voice requires training AI models on extensive audio data, though recent breakthroughs enable realistic clones from surprisingly short audio clips. While AI has undeniably improved the realism of synthetic voices, there are still noticeable differences between them and real human voices. One crucial aspect is the dynamic nature of human speech. Tiny, rapid adjustments in vocal cord muscle movements create a constant flux in phonemes—the building blocks of spoken language—that impact meaning and expression. Current voice cloning systems often struggle to capture this level of detail, resulting in voices that, while sounding human-like, may lack the inherent subtlety of natural speech.
Furthermore, the human vocal tract acts as a complex acoustic filter, modifying frequencies as air passes through it. Replicating this filtering process precisely is a challenge for AI models. Another key hurdle is the diverse nature of training data. Effective cloning depends on access to a wide range of audio samples, encompassing different emotional states, speaking styles, and environments. Achieving this comprehensive dataset can be a significant roadblock. The role of fundamental frequency (F0), which dictates the perceived pitch of a voice, is also important. It heavily influences how we express intention and emotion in speech. Cloning systems that don't meticulously account for F0 variations often produce synthesized voices that sound rather flat and emotionally detached.
Moreover, each individual's voice possesses a unique acoustic signature sculpted by their vocal tract and larynx. This inherent complexity poses a major challenge for voice cloning, which aims to recreate these unique signatures without fully comprehending the underlying physiological factors. It's also important to consider that excessive digital manipulation can introduce artifacts into generated audio. These artifacts, noticeable to those accustomed to the sounds of natural speech, can reveal the artificial nature of the voice, highlighting a gap between artificial and human speech.
Interestingly, neuroscience research reveals specialized neural circuits in humans and some animals that are dedicated to vocal learning and mimicry. Gaining a deeper understanding of these mechanisms could inform the development of more sophisticated voice cloning systems, allowing for AI models to adapt and learn more effectively from human voices. We also need to be aware of how the techniques used by sound engineers to refine audio for podcasts and audiobooks differ from the output of most raw voice cloning methods. These techniques, like compression and equalization, carefully shape the audio spectrum to emphasize certain frequencies that enhance clarity and engagement, crucial aspects frequently missing in the output of some voice cloning approaches.
Finally, cultural differences can influence the way we perceive voice and emotion. Voice cloning systems might encounter difficulties in achieving universal appeal unless they are meticulously tuned to accommodate these varied acoustic expressions and emotional connotations—a potential challenge for broader applications of this technology. In the realm of AI, continuous research and advancements are required to improve the accuracy and authenticity of voice cloning, and with this, the challenges of detection are also increasing. The potential for misuse through impersonation and misinformation is also very real, so the field is navigating ethical considerations alongside technological advancements.
The Neuroscience of Voice Cloning How AI Mimics Human Vocal Flexibility - Voice Cloning Applications in Content Creation
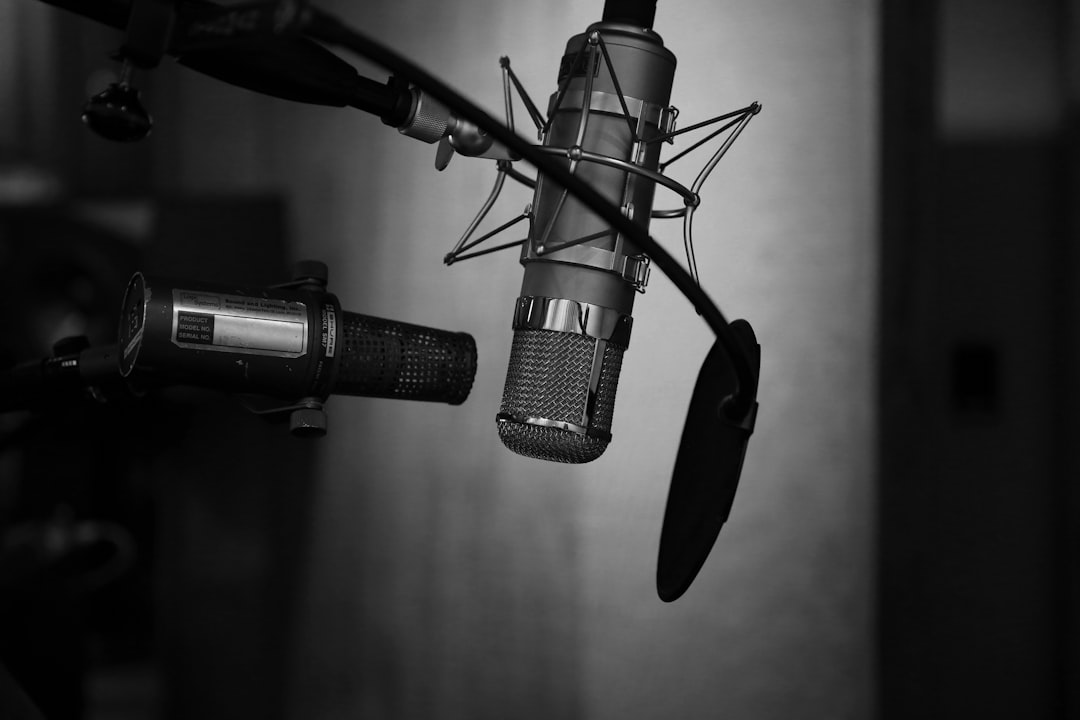
Voice cloning technology has revolutionized content creation, particularly in areas like audiobook production and podcasting. It allows creators to generate remarkably realistic digital voices, enabling them to craft compelling narratives that retain the emotional depth of the original speaker. This technology offers the potential to make audio content more accessible and engaging, whether through personalized narration or diverse character voices in a story. However, it also presents concerns related to the authenticity of the content and its potential for misuse. One of the key challenges for voice cloning is accurately capturing the complex variations in tone, rhythm, and other subtleties that define human speech. While AI-powered systems have made significant strides, synthetic voices often fall short of perfectly replicating the nuanced delivery of a real person, potentially hindering the creation of truly immersive audio experiences. Despite these obstacles, continuous advancements in the field hold promise for the future of voice cloning, potentially reshaping how we experience audio content in various forms.
Voice cloning technology has progressed significantly, enabling the generation of synthetic audio from remarkably short audio clips—sometimes just a 30-second sample. This advancement raises intriguing questions about authenticating and verifying the source of audio content. Beyond replicating the phonetic aspects of a voice, current systems are attempting to capture the nuances of emotion and expression. AI models now analyze parameters like intonation and rhythm to understand how humans convey feelings through their voices, a critical element for crafting effective audio experiences in audiobooks and podcasts.
However, the inherent dynamism of human vocal production remains a hurdle. Even minor adjustments in vocal cord tension can subtly alter pitch and emotional tone. Existing voice cloning approaches often struggle to replicate these rapid, often imperceptible, changes, leading to synthesized voices that can sound flat or lack the expressiveness of natural human speech.
Many voice cloning applications draw inspiration from psychoacoustics, recognizing the profound impact of specific sound frequencies and modulations on emotional communication. This understanding is essential for designing more engaging audio experiences.
Each individual's vocal tract creates a unique acoustic signature that voice cloning technologies strive to emulate. However, faithfully capturing and reproducing these personal identifiers, especially the subtleties of resonance and timbre, remains a considerable challenge for AI models.
Research indicates that voice perception is heavily influenced by context, including a listener's prior experiences and cultural background. This suggests that successful voice cloning needs to account for these variables to achieve wider acceptance.
Voice clones can enrich narrative storytelling in audiobooks and podcasts by allowing a single narrator to portray multiple character voices, increasing production efficiency while providing a novel avenue for creative storytelling.
The concept of vocal "micro-expressions"—brief, involuntary variations in tone—reveals how subtle changes can communicate complex emotions. Replicating these fleeting variations is key to achieving more realistic cloned voices, but currently represents a formidable challenge.
Some voice cloning applications are designed to adapt the synthesized speech dynamically based on the emotional context of the narrative, aiming to enhance listener engagement and bridge the gap between mechanical and human-like communication.
Finally, recent advances in voice cloning are enabling innovative applications in the field of digital mental health. Changes in a person's vocal patterns could potentially serve as early indicators of emotional distress, potentially leading to more proactive interventions and support systems. This highlights the potential of voice analysis as a tool for enhancing well-being.
The Neuroscience of Voice Cloning How AI Mimics Human Vocal Flexibility - Background Noise Adaptation in Voice Synthesis
Adapting to background noise is becoming increasingly important in voice synthesis. AI systems are now being developed to adjust how they produce sound in different environments, mirroring how our own auditory systems work. Our brains are remarkably good at understanding speech even when there's a lot of other noise around, but replicating this in synthetic voices is challenging. As voice cloning technology progresses, it's becoming clear that generating clear and emotionally expressive voices in diverse environments is a key issue. This is especially true for neural network-based systems, which often struggle with noisy audio.
There's a growing focus on adding more realistic emotional nuances and individual characteristics to synthetic voices. This work aims to improve the quality of audio experiences across a wide range of applications, from audiobooks and podcasts to interactive voice assistants. Despite these advances, creating truly natural-sounding voices in a variety of noisy conditions remains a hurdle. The complexities of human vocal production, especially in the presence of background sounds, continue to pose challenges, making this an area that warrants further research.
Background noise significantly impacts how we perceive and understand speech. Studies show that varying noise levels can affect how listeners interpret speech sounds, often leading to misunderstandings. Interestingly, the human auditory system shows remarkable adaptability, adjusting its neural responses based on the surrounding noise. This flexibility allows us to hone in on specific speech features, providing clues for how AI can replicate this behavior in synthetic voice creation.
Techniques like dynamic range compression in voice synthesis can mirror how human speakers naturally alter their volume and tone in different acoustic environments, leading to more realistic cloned voices. Our brains are adept at performing auditory scene analysis, which helps us separate sounds from different sources. Voice synthesis technologies are starting to implement similar algorithms to create synthesized speech that integrates more naturally with ambient sounds, like in podcast productions.
Different environments introduce unique reverberation patterns that can impact vocal clarity. Voice cloning technologies are tasked with recreating these effects for a natural sound, but simulating how a voice is perceived in various settings presents a complex hurdle. Humans possess remarkable perceptual adaptation abilities, getting used to consistent background noise and enhancing our focus on a primary speaker. Voice synthesis research is exploring how to integrate these features into systems to maintain clarity in challenging acoustic environments.
Real-time processing is crucial for voice synthesis systems that aim to rapidly adapt to changing background noise, mirroring the real-time nature of human speech production. This aspect highlights the computational demands of AI in striving for natural-sounding speech output. The precise timing of speech and background sounds greatly impacts how intelligible speech is. Modeling the subtle variations in timing in noisy settings is a complex undertaking for AI systems, which often struggle with intricate rhythmic patterns.
Background sounds don't simply mask speech; they filter it, altering its spectral properties. Effective voice cloning needs to incorporate ways to mimic these filtering effects to maintain the authenticity and unique character of a cloned voice. We also need to be mindful of cognitive load; background noise increases the cognitive effort needed to process speech. This is an important consideration for voice synthesis, as it can impact how synthesized voices are designed to enhance intelligibility in different acoustic settings. These challenges provide exciting avenues for researchers to explore and improve AI systems' capability to generate truly convincing and adaptable voices in various real-world settings.
The Neuroscience of Voice Cloning How AI Mimics Human Vocal Flexibility - Ethical Considerations and Identity Protection
The increasing sophistication of voice cloning technology necessitates a careful examination of ethical considerations, particularly concerning identity protection and the need for consent. The ability to replicate a person's voice with remarkable accuracy raises serious concerns about potential misuse, such as impersonation and the spread of false information. Individuals, especially those in the public eye, are increasingly vulnerable to having their voices cloned without their knowledge or consent. This highlights the need for regulatory frameworks that encourage responsible AI development and use.
Furthermore, the existing legal landscape may struggle to keep pace with the evolving technological advancements in this field. It's crucial that developers, policymakers, and users work together to establish clear guidelines and best practices to minimize the potential for harm. Open dialogues are needed to build a consensus on how to navigate the ethical complexities of voice cloning and foster a sense of trust in the authenticity of audio content. Failure to do so could lead to a decline in trust and a more fractured public discourse. Addressing these challenges will be instrumental in ensuring that voice cloning remains a tool for positive innovation while safeguarding individual identities and the integrity of communication.
The increasing sophistication of voice cloning presents a fascinating yet complex landscape of ethical considerations, particularly concerning the protection of individual identity and the potential for misuse. While the technology holds tremendous promise for fields like audiobook production and podcasting, the capacity to recreate someone's voice without their explicit consent raises concerns about privacy and the potential for impersonation. It's crucial to develop robust legal safeguards to ensure that individuals have control over their vocal identity in the digital age.
One area that warrants close attention is the impact of synthetic voices on human listeners. How easily we understand a voice clone, and the mental effort needed to decipher its meaning, depends on factors like the acoustic context and our familiarity with the cloned voice. If the listener experiences a high cognitive load due to the nature of the voice synthesis, it can potentially lead to misunderstandings. This suggests the need to develop voice cloning technologies that strive for clarity and produce not just accurate acoustic replicas but also communicatively rich and nuanced audio experiences.
Replicating the way human voices adapt dynamically to background noise represents a significant challenge for AI-powered voice synthesis. Our auditory systems are incredibly adept at understanding speech amidst a noisy environment, filtering out irrelevant sounds and focusing on the desired audio. Achieving this level of adaptability in AI models requires a deeper understanding of how sounds interact in various acoustic environments. This complexity is further compounded by the emotional nuances inherent in human speech, driven by principles of psychoacoustics. Even subtle changes in pitch or tone can alter the perceived emotional state of a voice, highlighting the need for AI systems to generate synthesized speech that conveys emotions in a way that aligns with human expression.
As voice cloning systems become increasingly indistinguishable from human speech, the need for effective detection mechanisms becomes more pressing. These systems need to effectively differentiate synthetic audio from authentic recordings, necessitating the development of tools that can analyze subtle acoustic markers indicative of AI-generated audio. The challenge lies in identifying those fine-grained features that distinguish synthetic voices from the natural variations in human speech.
Further complicating matters is the cultural aspect of voice communication. The way we perceive emotion and vocal nuances can vary dramatically across cultures. A synthetic voice designed for a specific culture might not necessarily resonate in the same way with people from a different background. This implies the need for voice cloning developers to incorporate cultural context into their models, ensuring that synthesized voices remain effective communication tools across diverse global communities.
Furthermore, the subtle shifts in tone known as vocal micro-expressions are critical to conveying complex emotional cues. While these rapid changes in speech can be nearly imperceptible, they play a key role in human interaction. However, accurately replicating them in AI-generated voices is a formidable challenge. Achieving realistic synthetic voices with a full spectrum of human-like emotional expression remains an ongoing area of research.
The use of cloned voices has the potential to impact listeners' psychological state, possibly reshaping their emotional responses to synthesized audio. This is especially true if the cloned voice belongs to a figure with whom the listener has a strong personal or social connection. In settings like therapy or when interacting with vulnerable populations, it's important to consider the potential psychological effects that synthetic voices might have and carefully weigh the benefits and risks before deploying these technologies.
The ultimate goal of many voice synthesis systems is real-time adaptation to diverse acoustic environments. Just like how we dynamically adjust our speech in different settings, AI-generated voices aspire to achieve similar adaptability. However, implementing these capabilities in AI systems requires substantial computational resources and complex algorithms. For example, in live applications like podcast recording or audiobook production, the ability to seamlessly adjust the synthesized voice to accommodate changes in background noise while maintaining clarity is essential. This ability adds another layer of complexity for developers striving to achieve truly lifelike synthetic speech.
Ultimately, every human voice is a unique expression of individuality, shaped by personal history, cultural influences, and inherent physical attributes. As we progress into an era where voice cloning becomes increasingly prevalent, we must remain mindful of preserving the rich tapestry of human vocal expression. Not only is it important to respect the individual's right to their vocal identity, but also recognizing that our voices represent a fundamental aspect of who we are. Maintaining the authenticity of voice and understanding its deeper implications for human identity is an integral part of developing voice cloning technologies responsibly.
Get amazing AI audio voiceovers made for long-form content such as podcasts, presentations and social media. (Get started for free)
More Posts from clonemyvoice.io: