Get amazing AI audio voiceovers made for long-form content such as podcasts, presentations and social media. (Get started for free)
Analyzing Audio Dynamics A Deep Dive into Indoor Pets' Live Sound Production
Analyzing Audio Dynamics A Deep Dive into Indoor Pets' Live Sound Production - Neural Networks for Animal Sound Classification
Neural networks are increasingly being used to classify animal sounds. They leverage deep convolutional networks, processing raw spectrograms or extracting features like MFCCs. These approaches have proven effective in classifying not just call types, but also in identifying specific animals. The success of these systems hinges on large datasets and realistic data collection. While challenges remain in classifying sounds across varying acoustic environments, advancements in audio signal augmentation are improving model performance. This progress in sound classification is poised to reshape how we study animal communication and behavior by automating the laborious process of labeling acoustic events.
Neural networks have emerged as a powerful tool for classifying animal sounds, offering insights into the intricacies of animal communication. By analyzing audio features such as pitch, frequency, and duration, these networks can differentiate between different vocalizations with remarkable accuracy, sometimes exceeding 90%.
One area where neural networks excel is in capturing the spatial relationships within audio waveforms. Convolutional Neural Networks (CNNs) are particularly well-suited for this task, mirroring their success in image processing. This ability to identify patterns in sound allows for the classification of complex vocalizations, even amidst environmental noise.
However, the effectiveness of these networks hinges on the quality and quantity of training data. Different animal species produce sounds in distinctive frequency ranges. A dog's bark, for example, falls between 100 Hz and 1 kHz, while a cat's meow can reach up to 4 kHz. This highlights the need for specialized training data, tailored to specific animal sounds, to achieve accurate classification.
Recent advances in transfer learning have alleviated some of the data burden. Researchers can now leverage pre-trained models, trained on general sound data, to accelerate the development of specialized animal sound classifiers. This approach significantly reduces the amount of labeled pet sound data required, making it more feasible to develop robust models.
Feature extraction techniques, like Mel-frequency cepstral coefficients (MFCCs), play a crucial role in isolating relevant characteristics of animal sounds. By focusing on specific aspects of the audio signal, these techniques enhance the neural network's ability to discriminate subtle variations in vocalizations, improving the precision of classification.
It is important to recognize that environmental noise can significantly impact the performance of sound classification models. Training neural networks with diverse background sounds is essential to enhance their robustness and ensure accurate classification in real-world scenarios.
While the potential of neural networks in understanding animal communication is undeniable, challenges remain. The meticulous task of annotating animal sound datasets is often time-consuming and requires expert knowledge in animal behavior to ensure accurate labeling. This presents a significant challenge in developing high-quality training sets for these models.
Despite these challenges, the future of animal sound classification is promising. The potential applications extend beyond basic understanding, holding promise for assisting in veterinary diagnostics and improving pet care by analyzing sound to detect behavioral issues.
Analyzing Audio Dynamics A Deep Dive into Indoor Pets' Live Sound Production - WASIS Software Revolutionizing Species Identification
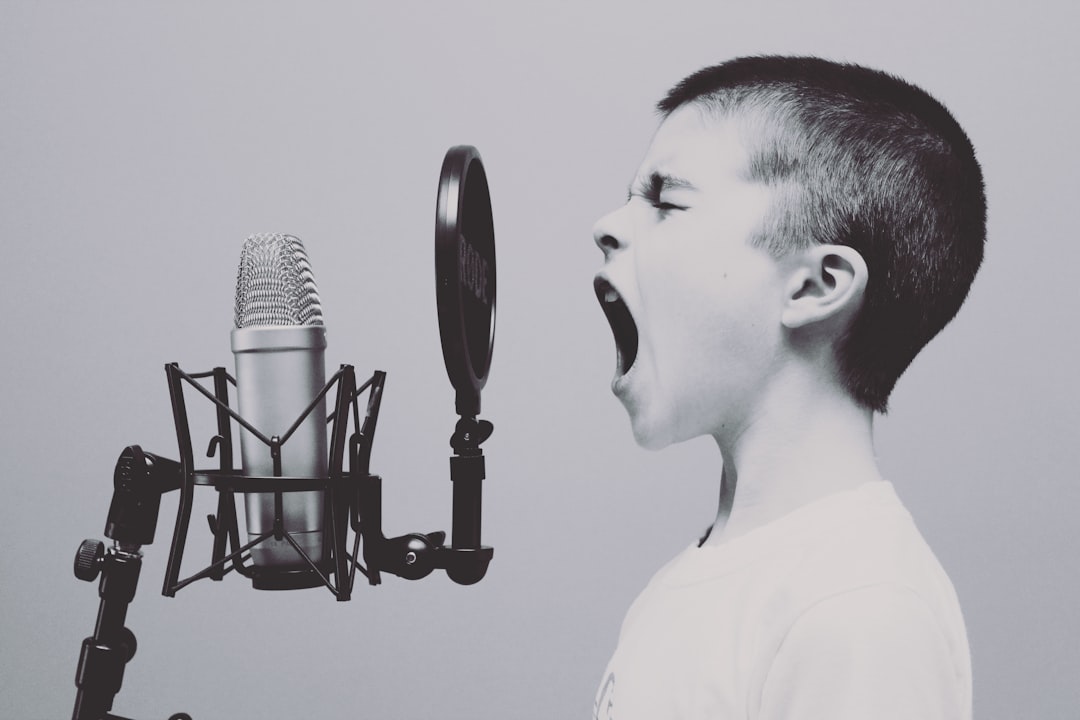
WASIS, short for Wildlife Animal Sound Identification System, is a software program that utilizes audio analysis to identify different animal species. It was created through a collaboration between researchers in Brazil, and is publicly available for use. WASIS employs machine learning algorithms to process audio recordings, extracting relevant features and comparing them to a database of known animal vocalizations. This enables the software to accurately identify the species producing the sound.
While WASIS offers a powerful tool for researchers and conservationists, its reliance on accurate data and sophisticated algorithms raises concerns about potential biases. The effectiveness of WASIS relies on the comprehensiveness of the database and the quality of the audio recordings, both of which can be challenging to manage. Despite these challenges, WASIS demonstrates the growing importance of utilizing technology in understanding animal communication and fostering biodiversity research and conservation efforts.
WASIS (Wildlife Animal Sound Identification System) represents a significant step forward in acoustic monitoring, much like the use of neural networks in classifying animal sounds. It leverages advanced audio fingerprinting, drawing parallels with music identification apps that match unique sound profiles against vast databases. This approach not only accelerates identification but also enhances accuracy, particularly when considering species that share similar frequency ranges.
WASIS goes beyond basic frequency analysis by considering temporal characteristics, like the attack and decay times of sounds. This adds a dimension to the identification process, helping to differentiate between species with overlapping frequency patterns. It also introduces the potential for real-time identification, which could prove invaluable in veterinary settings or shelters by providing immediate insights into animal behavior or welfare.
The software's multi-modal capabilities allow for integration of both audio and visual data, potentially including video feeds. This combined approach could offer a richer understanding of an animal's communication, taking into account both vocalizations and body language.
Crowdsourcing audio submissions from pet owners presents an exciting opportunity for data enhancement, creating a more diverse database and fostering greater engagement in species conservation and sound recognition. The software's potential for longitudinal studies is also promising, allowing researchers to track changes in sound production over time and analyze the impact of environment and upbringing on vocalizations. This could lead to significant advancements in ethology.
Beyond basic identification, WASIS incorporates semantic analysis, enabling categorization of sounds into specific contexts. This allows for deeper insights into the intentions behind animal communication, identifying, for instance, mating calls versus distress signals.
The software's commitment to algorithmic transparency is crucial for trust and accountability, particularly in applications like veterinary diagnostics, where understanding the basis of classifications is critical.
WASIS also allows for customization, enabling users to fine-tune parameters for specific species or breeds, adapting the software to the unique vocal traits of animals within their environment. This personalized approach can ensure targeted reactions and feedback.
The software's interactive feedback loop, which generates real-time feedback for pet owners on their animal's sounds, is particularly noteworthy. This could contribute to behavioral tracking and potentially identify stress or anxiety signals, promoting better pet care practices.
While these advancements are promising, it's crucial to remain aware of the challenges inherent in data collection and annotation. The need for expert knowledge in animal behavior and the time-consuming nature of these tasks remain significant obstacles. However, WASIS and similar technologies represent a remarkable evolution in the study of animal communication, opening doors to new avenues of research and applications in various fields.
Analyzing Audio Dynamics A Deep Dive into Indoor Pets' Live Sound Production - Convolutional Neural Networks in Noisy Environments
Convolutional Neural Networks (CNNs) are increasingly becoming the go-to tool for analyzing audio in noisy environments. Their strength lies in their ability to decipher the complex patterns within sound waves, allowing them to effectively classify various vocalizations even when surrounded by background noise. Techniques like data augmentation and the integration of Long Short-Term Memory (LSTM) units within CNNs have been developed to enhance their resilience against the interfering effects of environmental noise. Research is ongoing to further refine these models, focusing on the ability to distinguish between structured and unstructured sounds, a crucial skill for applications in fields like automated audio surveillance and the study of animal communication. As we delve deeper into the nuances of audio dynamics, the development and refinement of CNNs for noisy environments will be essential for the advancement of technologies like voice cloning and other audio production tools.
Convolutional Neural Networks (CNNs) are emerging as a crucial tool in analyzing audio dynamics, particularly in noisy environments. Their ability to pick out specific frequency patterns helps them to classify sounds even when there's a lot of background noise.
One approach to improve CNN performance in noisy settings is through "data augmentation," which involves introducing synthetic noise into training audio. This makes the models more resilient to real-world noise variations. CNNs also employ "pooling layers" that act like filters, focusing on the most important parts of an audio signal and minimizing the impact of irrelevant noise.
It's intriguing to see how CNNs can leverage their image recognition capabilities to analyze audio through spectrograms, transforming sound into two-dimensional images that highlight its spatial and temporal characteristics. This representation allows CNNs to identify patterns that might otherwise be missed.
Furthermore, multi-channel audio input, using multiple microphones, has been proven to enhance CNN performance, especially in complex acoustic environments. This approach helps to separate animal vocalizations from overlapping sounds, offering more precise identification.
The choice of activation function in CNN architectures can also play a key role in handling noise. Variations of ReLU (Rectified Linear Unit) have shown promise in accelerating convergence and identifying relevant features even in noisy conditions.
CNNs are adept at adapting to changing sound patterns through "online training," which enables them to continuously update their models based on new audio data. This is particularly important when vocalizations change due to environmental variations.
The combination of convolutional and recurrent layers, known as CNN-RNN architectures, is showing great potential in analyzing audio dynamics in variable noise environments. These hybrid architectures can recognize patterns over time, making them more effective in situations with changing noise levels.
Despite their strengths, CNNs face challenges in environments with masked sounds, where overlapping frequencies can obscure distinct vocalizations. However, ongoing research in feature extraction holds promise in addressing this limitation by enhancing signal clarity during training.
The future of voice cloning and podcast production is likely to be shaped by developments in CNNs. Their ability to learn and replicate distinct audio features could lead to more natural-sounding voice synthesis, even in noisy real-world scenarios. This would ultimately improve the listener experience, making these technologies more accessible and immersive.
Analyzing Audio Dynamics A Deep Dive into Indoor Pets' Live Sound Production - Marmoset Monkey Vocalizations Dataset Analysis
The study of marmoset monkey vocalizations provides a unique glimpse into the complex world of sound production, offering valuable insights applicable to fields like voice cloning and audio analysis for pet sounds. Marmosets demonstrate remarkable flexibility in their vocalizations and possess a neural system that suggests sophisticated communication capabilities, echoing those seen in humans. The intriguing developmental patterns of their vocalization highlight the profound influence of social interaction and feedback on their vocal skills, which might hold potential for improving audio processing technologies. The rich dataset of marmoset vocalizations enables researchers to explore the intricate relationship between biology and technology, opening avenues for advancements in automated sound classification, podcasting, and even virtual assistant applications. By delving into the intricacies of marmoset vocalization, we gain a deeper understanding of the evolution of vocal communication and its implications for the development of artificial intelligence within audio environments.
Marmoset monkeys possess a sophisticated vocal system, employing over 30 distinct calls for various social purposes, such as warning about predators or coordinating group activities. Their vocalizations, however, are not static, evolving dynamically over time. This suggests that they learn and adapt their calls based on social interactions, highlighting their advanced communication capabilities.
Marmosets show a remarkable ability to mimic the calls of their companions, resembling "vocal turn-taking" observed in human conversations. This indicates a sophisticated social awareness and communication strategy. Interestingly, context influences their vocal patterns, demonstrating that their calls are not purely instinctual but rather context-sensitive and deeply intertwined with their social environment.
Acoustic analysis reveals distinct frequency modulations in their calls, varying significantly across different call types. This makes marmoset vocalizations a valuable resource for research, potentially informing sound classification methodologies employed in voice cloning technologies. Dominant marmosets, it seems, utilize lower frequency calls, perhaps to exert control or enhance communication within their social groups.
Neurophysiological studies have uncovered highly developed sound processing regions in marmoset brains, reminiscent of human brain structures. This suggests potential evolutionary pathways for voice and sound production. Marmoset calls can be exceptionally loud, reaching up to 100 decibels, comparable to chainsaw noise. This presents intriguing challenges for sound classification algorithms, especially in noisy environments.
The vocalizations of marmosets provide a model for understanding basic sound communication principles. This has profound implications for improving audio analysis techniques across diverse applications, ranging from animal behavior studies to podcast sound design. Machine learning algorithms trained on marmoset vocalizations demonstrate promise for advancing real-time sound recognition systems, which could be integrated into vet clinics to analyze and interpret animal sounds for better health monitoring.
Analyzing Audio Dynamics A Deep Dive into Indoor Pets' Live Sound Production - Mobile AI Applications for Animal Sound Recognition
Mobile AI applications for animal sound recognition are changing how we understand animal communication. These apps use machine learning to classify animal sounds right on smartphones, making research easier than ever. We have so much more animal sound data now than before, making automatic detection and analysis possible. This includes systems that use deep learning to analyze complex sounds like bird songs. There are still challenges, though. We need good training datasets and ways to filter out noise in different environments. As we improve deep learning and transfer learning, these mobile apps will give us better insights into animal behavior and other aspects of audio, including voice cloning and podcast production.
The development of AI applications for animal sound recognition is being driven by the increasing complexity of vocalizations found in certain species, like marmoset monkeys. These animals have over 30 distinct calls, each serving specific social functions. This vocal diversity presents exciting possibilities for developing more nuanced and advanced AI systems capable of recognizing sounds.
However, one challenge in analyzing animal vocalizations is their often high decibel levels. Some calls can reach over 100 decibels, similar to the noise of a chainsaw. This creates a difficult environment for algorithms that need to distinguish between the desired sound and potentially overwhelming background noise.
Another factor that is fueling research is the temporal dynamics of animal calls. Vocalizations are often context-sensitive, meaning their patterns change based on the social interactions taking place. Understanding these dynamic changes could lead to more effective feature extraction approaches in AI models designed for sound recognition.
Intriguingly, neurophysiological studies on animals like marmosets have revealed brain structures related to sound processing that bear remarkable similarities to those found in humans. This sheds light on the potential evolutionary pathways of sound and voice production, and can potentially inform the development of AI systems that mimic these biological processes for improved audio analysis.
Furthermore, marmoset monkeys exhibit a behavior known as "vocal turn-taking," where they alternate calls in a way that resembles human conversation. This behavior suggests that AI models capable of processing audio in a more human-like, interactive fashion might be achievable.
There's ongoing research focused on customizing neural network parameters for specific species in sound classification applications. This tailoring can significantly enhance accuracy, ensuring that the unique vocal patterns of different animals are recognized more effectively.
Researchers are exploring the potential for advanced audio recognition software to provide real-time feedback to dog owners. This feedback could offer valuable insights into the emotional states or stress levels of their pets based on vocalizations. This capability could have direct implications for behavioral analysis and potentially even lead to early detection of health issues.
Data augmentation techniques are increasingly employed to improve the robustness of neural networks in noisy environments. Researchers are introducing synthetic background noise into training datasets, making the models more resilient to real-world variations.
The development of AI for animal sound recognition is not limited to its direct applications. The techniques being developed for animal sound recognition have the potential to cross over into other fields. For example, they could be used to improve voice cloning technologies and enhance audio quality in podcast production by enabling a better grasp of how to filter and analyze diverse vocal inputs.
While there are many challenges to overcome, research in animal sound recognition holds enormous promise for advancing our understanding of communication across species and for developing new tools for human interaction with animals and each other.
Get amazing AI audio voiceovers made for long-form content such as podcasts, presentations and social media. (Get started for free)
More Posts from clonemyvoice.io: