Get amazing AI audio voiceovers made for long-form content such as podcasts, presentations and social media. (Get started for free)
The Evolution of Voice Recognition Technology in Podcast Production
The Evolution of Voice Recognition Technology in Podcast Production - From Cold War Monitoring to Podcast Production Automation
The journey of voice recognition technology began in the shadows of the Cold War, its initial purpose rooted in surveillance and intelligence gathering. Early systems, like IBM's Shoebox, focused on the rudimentary task of recognizing spoken digits, a far cry from the sophisticated capabilities we see today. However, these early efforts established the foundational principles that have paved the way for significant advancements. Modern voice recognition, fueled by deep learning and AI, has become a critical component of podcast production. Podcasters now benefit from automated processes that previously demanded significant manual effort, leading to improved audio quality and a more efficient workflow. This shift has democratized podcasting, enabling a wider range of creators to participate in the medium. While the evolution of voice recognition presents tremendous opportunities, it also calls for a thoughtful examination of its potential impact, prompting conversations about privacy, responsibility, and the ethical implications of its increasing presence in creative fields.
The initial applications of voice recognition were primarily focused on national security, stemming from the Cold War era's need for intelligence gathering. Early systems, while rudimentary, laid the foundation for future advancements. Imagine the technology used for monitoring phone calls – that's where it all began.
Early systems primarily focused on recognizing simple commands like numbers, exemplified by IBM's Shoebox in the 1960s. While seemingly basic, these early efforts demonstrated the potential for machines to interpret human speech.
The field significantly evolved with the introduction of deep learning, which allows algorithms to learn intricate patterns in audio data. This includes leveraging neural networks like RNN-Transducer and encoder-decoder models, a dramatic departure from older, more simplistic pattern-recognition approaches.
Podcast consumption has exploded, evolving from a niche hobby to a major medium. While podcasting was barely a concept in 2004, search queries related to it skyrocketed to over 19 billion by 2021, highlighting the rapid growth of the space.
This surge in podcasting has fueled a demand for efficient production techniques. AI, in particular, has revolutionized post-production aspects. Automation tools have streamlined editing, reducing the workload on creators and opening up new avenues for creative expression.
Today, voice recognition is a ubiquitous technology extending far beyond the podcast world. Voice assistants, smart home interfaces, and in-car systems are all powered by voice recognition technologies, showing its widespread influence on human-computer interaction.
AI has brought significant changes to the podcast creation pipeline. It has enabled smoother content production, offers improved insight into audience engagement through advanced analytics, and leads to higher overall production quality, influencing industry standards.
As voice recognition technology expands, it also brings up concerns, particularly about privacy. The discussion around ethical development and responsible AI use has gained prominence, often taking the shape of collaboration between governments and private tech developers.
The increasing interest in voice analytics and the wider integration of AI in both business and consumer areas has significantly impacted voice recognition technology. The field is influenced by the growing use cases for understanding and manipulating speech.
The future holds exciting possibilities for voice recognition, fueled by ongoing advancements in deep learning and artificial intelligence. We can anticipate more intuitive user experiences in various applications, along with solutions to emerging challenges, creating a more interactive and accessible landscape for voice-based technologies.
The Evolution of Voice Recognition Technology in Podcast Production - Dragon Dictate's $9,000 Price Tag in 1990 vs Today's Accessible Tools
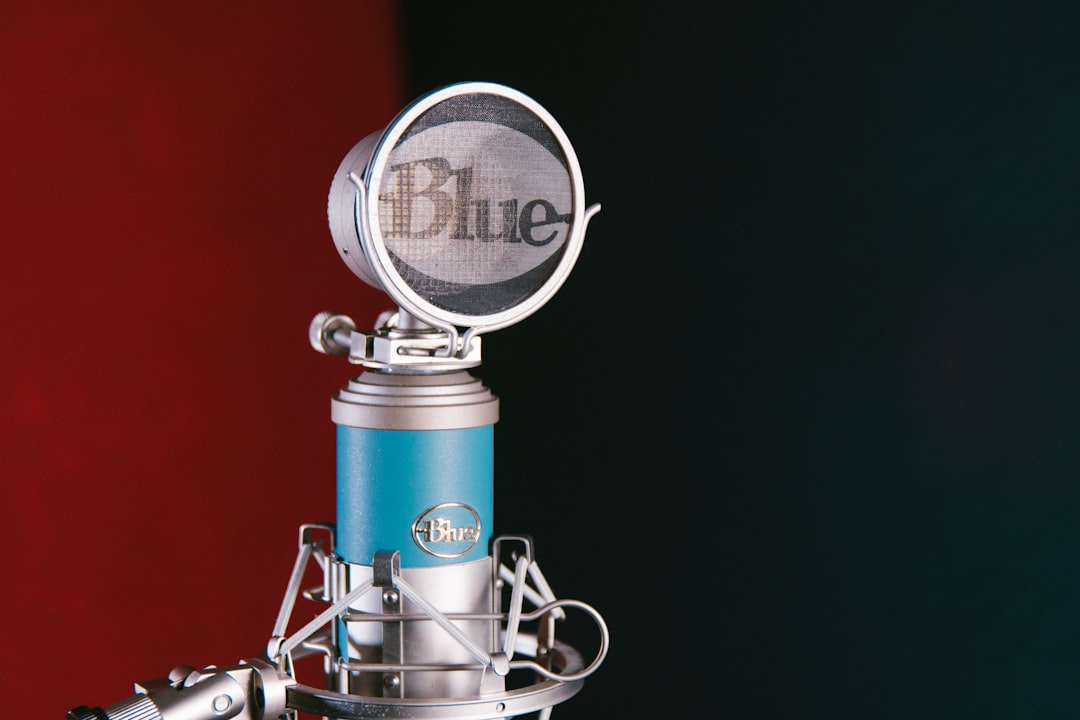
Back in 1990, accessing voice recognition technology like Dragon Dictate was a luxury reserved for a select few. Its hefty $9,000 price tag effectively limited its use to specialized professionals, despite the technology's groundbreaking ability to understand around 100 words per minute – quite impressive for the time. Today, the picture is vastly different. Modern tools, such as Dragon Professional v16, provide significantly enhanced capabilities, including speed and accuracy, at a significantly more approachable price point of roughly $699. This accessibility has democratized the use of voice recognition across various creative fields, particularly podcasting. Podcasters can now employ advanced speech-to-text features to streamline production processes and boost audio quality, leading to more polished productions. Modern systems are not only capable of understanding complex voice commands, but they also integrate seamlessly with diverse platforms, enabling hands-free operation and streamlining workflows. The stark contrast between the past and present demonstrates how far this technology has advanced. Sophisticated AI within modern voice recognition software empowers podcast creators to produce content that's not only faster to create but also richer in quality, fundamentally altering the podcasting landscape.
Back in 1990, Dragon Dictate represented a pioneering step in consumer-level speech recognition, but at a hefty price of $9,000. It relied on specialized hardware and a large vocabulary, catering primarily to professionals needing dictation and transcription capabilities. Its accuracy was limited, recognizing around 100 words per minute, which was considered quite an achievement at the time.
Fast forward to today, and we find that voice recognition has become incredibly accessible. Modern tools like Dragon Professional v16, while still favored by some professionals due to their specialized features, can be purchased for around $699. Moreover, smartphone apps can now achieve accuracy levels of up to 95% for common phrases, highlighting the remarkable progress made in voice recognition technology. This progress is primarily due to advancements in processing power and sophisticated algorithms like machine learning and neural networks.
Early systems typically required users to train the software with their unique voice patterns using predefined prompts. Modern systems have embraced continuous learning, adapting to a user's voice over time through machine learning, making them much more user-friendly and versatile. These enhancements are evident in the incorporation of natural language processing (NLP) within current voice recognition software. NLP allows them to understand context and nuance, resulting in more coherent transcription outputs, a crucial feature for podcasters needing accurate representations of dialogue.
Beyond simple commands, today's speech recognition systems handle complex conversations, making them ideal for interactive podcast interfaces or virtual assistants. Furthermore, modern algorithms effectively filter out background noise and distinguish between multiple speakers, which was a major hurdle for earlier systems.
The shift from dedicated hardware, like the computers required by the original Dragon Dictate, to everyday devices is testament to the improvements in both algorithm efficiency and hardware capabilities. The initial software also heavily relied on proprietary vocabulary sets, limiting its adaptability. Today, AI-driven tools draw upon extensive datasets and can adapt to specialized vocabularies, including various accents and slang.
The move from hardware-bound solutions to cloud-based applications makes voice recognition even more accessible. Podcasters can now edit content on-the-go, streamlining the creative process. This enhanced accessibility has truly democratized audio production, opening up podcasting and audiobook creation to a much wider range of creators. While earlier systems demanded specialized skills, many individuals can now easily utilize simple voice commands to produce high-quality audio content. This shift underscores how far we've come from the expensive and somewhat niche technology of Dragon Dictate in 1990.
The Evolution of Voice Recognition Technology in Podcast Production - Deep Learning's Impact on Speech Recognition Accuracy Since 2012
Since 2012, deep learning has significantly boosted the accuracy of speech recognition systems, pushing the boundaries of what was once thought possible. This has been a game-changer for podcast production, smoothing out the editing process and leading to higher-quality audio. Modern systems are better at understanding various speech patterns, including accents and the subtle emotional nuances in a speaker's voice, making them incredibly useful for creators. This surge in capability not only simplifies the podcasting workflow but also allows for more nuanced storytelling, enriching the podcasting experience as a whole. However, the rapid evolution of this technology raises important considerations about ethical implementation, user privacy, and the potential biases that might be inherent within AI-powered speech recognition systems.
The field of speech recognition has experienced a remarkable transformation since 2012, largely due to deep learning. Improvements in modeling techniques and access to vast datasets have pushed the accuracy of Automatic Speech Recognition (ASR) systems from around 80% to over 95% in many situations. This leap is largely attributable to the introduction of neural networks, specifically Long Short-Term Memory (LSTM) networks, which are adept at capturing the context within longer audio sequences, a crucial feature for understanding conversational speech prevalent in podcasts and audiobooks.
Furthermore, modern speech recognition systems are able to focus on relevant sections of audio inputs through a technique called attention mechanisms. This focus on context leads to more accurate and understandable transcriptions, vital for correctly interpreting the nuanced dialogues frequently found in podcast content. The capabilities of deep learning have enabled speech recognition systems to adapt to a wider array of languages and dialects, making podcast content accessible to a broader global audience.
One of the challenges traditionally faced by speech recognition systems has been the presence of noise. Deep learning has contributed significantly in designing algorithms that can better differentiate between desired speech and unwanted background sounds, utilizing techniques like spectral masking and noise robust models. This advancement is particularly important in podcast production where various environments and audio equipment might produce variable quality.
The same advancements in deep learning that have improved ASR have also yielded significant improvements in voice cloning technologies, such as Neural Text-to-Speech (TTS). These AI-powered systems can now generate incredibly realistic voice clones, opening new avenues for podcasters who want to personalize their audio content or perhaps create new synthetic voices. These innovations impact how we perceive and interact with audio.
The marriage of increasing computational power and deep learning has enabled the development of real-time speech recognition, making it suitable for live podcasting or other interactive applications. The technology can now respond instantaneously to spoken prompts and this has major implications for listeners engaging with podcasters in a more fluid way.
This enhanced functionality is also supported by the creation of incredibly varied training datasets that encompass a wide range of cultural contexts and accents. This inclusivity fosters better adaptation to a wider spectrum of speech patterns, which makes voice recognition technologies more readily available to more people.
The integration of speech recognition tools into podcast workflows has greatly streamlined the editing process. Tasks such as generating automated show notes or extracting highlight reels are now much faster. This has the potential to improve a podcaster's overall efficiency and allows creators to spend more time on the creative aspects of their production.
As voice technology, in general, continues to be integrated into more aspects of our lives, it also becomes important to reflect on its ethical implications. Podcast producers, and the industry as a whole, are facing discussions about how to ethically manage data, especially in regards to consent and privacy concerns. These debates are essential as podcasting continues to evolve and rely on analyzing the data contained within audio recordings.
The integration of deep learning has transformed speech recognition, impacting the way podcast producers, audiobook creators, and voice cloning engineers approach sound design. The evolution of this technology demonstrates the profound impact of AI, from enhanced accuracy and contextual understanding to multilingual capabilities and voice cloning. As we continue to explore this technology, it's vital to understand the ethical considerations it brings along, fostering a balance between innovation and responsible deployment for the benefit of podcasting and beyond.
The Evolution of Voice Recognition Technology in Podcast Production - Neural Network ASR Models Revolutionizing Podcast Transcription
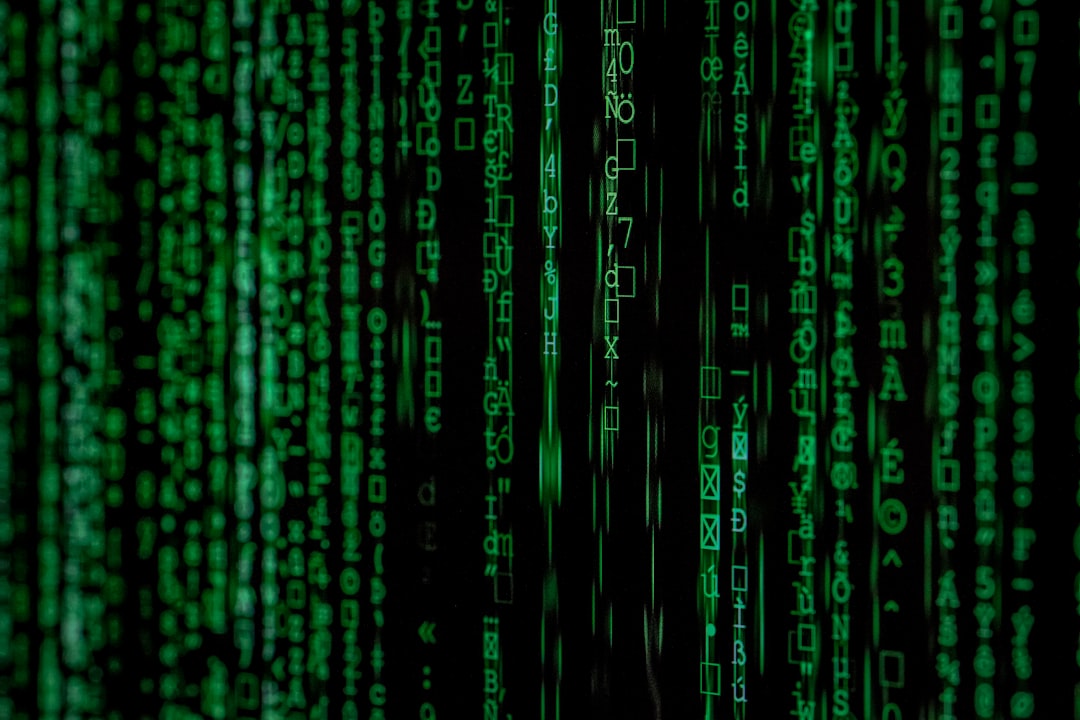
Neural network-based Automatic Speech Recognition (ASR) models are revolutionizing podcast transcription, offering significant improvements in accuracy, speed, and ease of use. The move towards more sophisticated end-to-end models, powered by deep learning methods like transformers, has dramatically refined the process of converting spoken words into written text. This progress allows for more reliable transcriptions, even when dealing with different accents or noisy environments, a challenge that previously hindered audio production. As these advanced models become integrated into podcast production pipelines, they are streamlining tasks like automated note generation and dialogue extraction, freeing up podcasters to focus on the creative aspects of their work. This advancement in the technology does, however, introduce ethical concerns around data privacy and responsible AI practices, issues that require thoughtful consideration as the field continues to evolve.
Neural network-based Automatic Speech Recognition (ASR) models are fundamentally altering the podcast landscape. These models have moved beyond simply recognizing individual words and are now capable of understanding the broader context within spoken dialogue. This allows podcasters to preserve the emotional nuances and narrative flow of their content, ultimately enhancing the listening experience.
Furthermore, these models have become increasingly multilingual, thanks to extensive training datasets encompassing a wide range of languages and dialects. This global reach enables podcasters to connect with a more diverse listener base, offering content in multiple languages without sacrificing accuracy. Addressing a historical challenge, advanced algorithms effectively filter out unwanted background noise, crucial for podcasters recording in various environments. This noise robustness is made possible by techniques like spectral masking and noise-resistant model designs.
The integration of powerful computing with deep learning has also enabled real-time speech recognition, a game-changer for live podcasting. This allows for immediate audience interaction, creating a more dynamic and responsive experience. The models are becoming increasingly personalized, adapting to individual speaking patterns and unique vocabularies. This personalization enhances accuracy and allows podcasters to use specific jargon or slang, creating a more genuine connection with their audience.
These advancements have led to transcription accuracy rates exceeding 95% in many cases. This level of precision is vital for efficient editing, generating show notes, and ensuring that written content accurately reflects the spoken word. It's not just transcriptions; the improvements in ASR have also driven innovation in voice cloning technologies. Neural Text-to-Speech (TTS) systems can now produce incredibly realistic synthetic voices, creating opportunities for podcasters to personalize their content or even introduce diverse character voices.
The incorporation of ASR tools into the podcast production workflow has streamlined various tasks, such as creating summaries or extracting specific audio segments. This automation not only improves efficiency but frees up creative resources for podcasters to focus on storytelling. The design of these models often incorporates attention mechanisms, enabling the models to hone in on essential parts of the audio input. This capability is crucial for understanding complex dialogues, especially in podcasts with multiple speakers or intricate conversations.
While incredibly promising, the evolution of these technologies necessitates thoughtful consideration of their ethical implications. As podcasters increasingly rely on AI, questions of data privacy and ethical data handling become more critical. Ensuring transparency and obtaining informed consent are vital aspects of responsible podcast production in this era of AI-driven tools. The rapid evolution of neural network ASR models has undeniably reshaped the podcasting landscape, bringing improved accuracy, global reach, and new creative opportunities. However, as we move forward, it's essential to continually engage with the ethical questions that arise with these powerful technologies, ensuring their use benefits podcast creators and listeners alike.
The Evolution of Voice Recognition Technology in Podcast Production - AI-Driven Editing and Mixing Tools Streamlining Production Costs
AI-powered editing and mixing tools are transforming how podcasts are produced, making the post-production stage much more efficient. These tools use complex algorithms to automate tasks that used to require a lot of manual work, like removing unwanted background noise or adjusting audio levels. Tools employing these techniques, like Cleanvoice, help podcasters achieve high-quality audio without needing to be experts in sound engineering. This automation not only speeds up the workflow but also often results in better-sounding audio overall, freeing creators to focus on crafting the content itself. It's crucial to remember, though, that while these technologies are exciting, there's a need to keep human judgment involved, ensuring the finer points of sound production are considered carefully. As these new technologies become more common, discussing their potential effects on the industry is vital to make sure they are used responsibly in the evolving podcast landscape.
The integration of AI into audio editing and mixing has significantly altered the podcast production landscape, introducing a new era of efficiency and accessibility. AI-powered tools are now adept at identifying and separating multiple speakers within a recording, previously a painstakingly manual task. Imagine an algorithm that can automatically isolate each person's voice and create individual audio tracks – this level of automation drastically reduces editing time. Further, some AI systems are even beginning to analyze the emotional nuances within a voice, potentially helping creators understand how their delivery affects listener engagement. While this is a fascinating development, it raises questions about the biases inherent in such algorithms and their impact on human expression in audio.
Additionally, AI-driven noise reduction has become increasingly sophisticated, employing spectral analysis to differentiate between desired speech and background noise. This is especially useful for podcasters who often record in less-than-ideal environments. However, we need to consider the possibility of AI systems inadvertently removing subtle cues, like environmental sounds that could contribute to the podcast's ambiance or authenticity. The ability of these tools to adapt and learn based on user interactions also continues to improve. It's fascinating to observe how AI can evolve over time to become more attuned to a particular podcaster's style and preferences.
Voice cloning has also advanced, allowing for the creation of multiple voice variations based on a single input text. This is particularly intriguing for storytelling and creating unique character voices within a podcast. However, ethical considerations around the use of voice cloning are paramount – particularly in cases where voices are used for impersonation or without consent.
Beyond basic editing, AI is starting to grasp the broader context of the audio, potentially suggesting edits that improve narrative flow and maintain a coherent structure. This contextual understanding could help podcasters to maintain a consistent and compelling story throughout their content. The ability to tailor these AI tools to industry-specific jargons or technical vocabularies is another significant improvement. This ensures that niche podcasts and audiobook productions remain accessible to their intended audiences, a crucial aspect in preserving the diversity of content. Furthermore, AI is becoming increasingly proficient at automatically generating materials like show notes and episode summaries, freeing up creators to focus on the artistic elements of their work. The sheer speed at which these AI features are developing raises new challenges and opportunities for creators, urging us to explore the ethical and creative considerations associated with this ongoing technological advancement.
The evolution of AI-driven audio tools is transforming the creative process in podcast production, and beyond. While the advancements are significant, it's important to acknowledge the potential challenges related to bias, the unintentional removal of nuanced cues, privacy concerns, and the responsible use of voice cloning technologies. Moving forward, we will need to carefully navigate the balance between the efficiency and automation provided by AI and the preservation of human creativity and ethical considerations within the medium. The continued development of these technologies will undoubtedly lead to new methods of content creation and delivery. We can anticipate even more sophisticated AI-driven tools emerging, pushing the boundaries of what's possible in the realms of podcasting, voice cloning, audiobook production, and audio creation as a whole.
The Evolution of Voice Recognition Technology in Podcast Production - Natural Language Understanding in Modern Voice Recognition Systems
Natural Language Understanding (NLU) plays a crucial role in modern voice recognition systems, particularly within podcast production. It allows computers to comprehend not just individual words, but also the underlying context, purpose, and subtle emotional cues embedded in spoken language. This capability bridges the gap between humans and machines, creating more natural and engaging interactions between podcasters and listeners. NLU doesn't just enhance the accuracy of transcriptions; it also empowers podcasters with sophisticated tools. These tools can generate summaries of episodes, edit audio automatically, and streamline the production process for those without extensive audio expertise. However, as NLU advances rapidly, it also raises important ethical concerns, such as data privacy and the potential for bias in AI systems. It's crucial to develop and deploy these powerful technologies responsibly. The increasing demand for high-quality audio content signifies that the connection between voice recognition and NLU will continue to mold the future of audio creation and influence how podcasts are developed.
The intersection of Natural Language Understanding (NLU) and modern voice recognition systems is yielding fascinating results, especially in the context of podcast production. It's becoming increasingly clear that these systems are capable of far more than just transcribing spoken words. For example, advanced NLU algorithms are getting better at deciphering the intent and context of spoken language. This means they can differentiate between similar commands depending on the surrounding conversation, which significantly boosts the accuracy of automated transcriptions, particularly during complex podcast discussions.
Some NLU systems are even starting to delve into the realm of emotion, analyzing and interpreting the subtle emotional shifts in a person's voice. This offers podcasters a new dimension of audience analysis, allowing them to gauge listener engagement based on how emotion influences reception, shaping their content creation and engagement strategies. It's a bit like giving podcasters a real-time emotional barometer for their listeners.
Furthermore, NLU is increasingly being integrated with other forms of data input, like visual cues or other sensory feedback, pushing beyond simple audio processing. This "multimodal" approach can make voice-driven environments far richer, resulting in more engaging podcast applications and enhanced voice-based storytelling. Imagine a podcast where the system can adapt based on the visuals or mood of the story being told – it's a really exciting concept.
NLU can also offer real-time feedback to podcasters while they are recording. This could mean that creators receive immediate insight into how their delivery is being received or even suggestions based on AI-generated analysis. Essentially, NLU becomes a co-creator, transforming the way podcasters craft and refine content. It's a very powerful concept that blurs the lines between the role of the creator and the tool.
Another interesting aspect of these evolving systems is their ability to adapt their vocabulary dynamically. This means NLU systems can quickly learn the specialized jargon or language shifts prevalent within niche podcasting communities. This adaptability creates smoother interactions between hosts and their particular audiences, tailoring the system to meet their needs. It's sort of like having an AI that becomes a true partner in understanding the intricacies of a podcast's subject matter.
NLU is also breaking down language barriers. These advanced systems are now becoming increasingly adept at handling multiple languages and dialects. This opens up tremendous potential for podcasters who want to reach global audiences without sacrificing the quality of the content, or the listener's ability to understand. The idea of a seamless global podcast experience for both creators and listeners is a captivating prospect.
It's also worth noting that modern NLU can differentiate between multiple speakers within a recording. This means cleaner, more organized transcripts, but it also simplifies the editing process by allowing podcasters to organize audio clips based on speaker. This is huge for podcasts with multiple hosts, as it drastically cuts down on the time needed for editing.
Another benefit is the ability to better manage noise in recordings. Systems with robust audio processing can filter out a lot of unwanted background noise, improving audio quality, even in challenging recording environments. Podcasters could record from anywhere, with less worry about needing professional-grade sound isolation.
Finally, over time, NLU systems can learn an individual's voice patterns and unique style. This results in improved transcription accuracy, and personalized editing suggestions tailored to each podcaster. It's like having a system that learns and adapts to your style as a creator, leading to a more efficient workflow.
However, the advancement of NLU also raises some cautionary flags. Because these systems learn from the data they are trained on, they can inadvertently inherit biases that exist within those datasets. This has implications for how voice recognition interprets accents or colloquialisms, highlighting the need to continually monitor and evaluate these systems to ensure they are treating all speech patterns fairly and equitably. The ethical and societal implications of AI systems need careful consideration.
In conclusion, NLU's impact on contemporary voice recognition technologies is profound, particularly in the world of podcast production. These systems are shifting from simple transcription tools to sophisticated companions, capable of understanding nuanced communication, offering insights into audience engagement, and creating more accessible, global content. But as the technology advances, it's critical to remain aware of the potential biases these systems may inherit and actively work to mitigate them, ensuring that these tools benefit all communities, creating a more inclusive and accessible space for audio content creators.
Get amazing AI audio voiceovers made for long-form content such as podcasts, presentations and social media. (Get started for free)
More Posts from clonemyvoice.io: