Neural Voice Synthesis The Convergence of AI and Voice Cloning in 2024
Neural Voice Synthesis The Convergence of AI and Voice Cloning in 2024 - DDSP Models Revolutionize Voice Synthesis Accuracy
The emergence of Differentiable Digital Signal Processing (DDSP) models represents a significant leap forward in the realm of voice synthesis. By integrating established signal processing methods with the power of deep learning, DDSP allows for a new level of control and accuracy in audio generation. Unlike older methods, DDSP can faithfully capture the intricate details of pitch and dynamics during voice transformations, offering a much richer and more expressive auditory experience. This ability is particularly relevant for applications like voice cloning and audiobook production, where nuanced vocal performances are crucial. The development of DDSP has shifted the focus towards creating more lifelike synthetic voices. We are seeing a strong push towards greater accuracy and realism, driving the exciting intersection of AI and voice cloning seen throughout 2024. As research continues to uncover the vast capabilities of DDSP, we can expect a wave of innovation across numerous areas of audio production, including podcast creation and other forms of sound design, where the pursuit of authentic and captivating audio remains paramount.
DDSP, short for Differentiable Digital Signal Processing, has emerged as a game-changer in the realm of voice synthesis by blending traditional signal processing techniques with the power of neural networks. This approach allows for a more nuanced control over the synthesized audio compared to earlier methods that relied solely on samples. Essentially, it gives us the ability to fine-tune the timbre and pitch in ways that were previously impossible.
A fascinating aspect of DDSP is its capacity to inject expressiveness into synthesized speech. We can now manipulate elements like emotional tone and even accents, leading to a more natural and lifelike audio experience. Details like breathiness and vibrato, often lost in traditional methods, can be meticulously reproduced with DDSP, creating a bridge between machine-generated and human speech.
What's intriguing is that DDSP models can achieve high-quality audio generation even with relatively limited datasets. This presents a more accessible approach to voice synthesis, potentially opening the door for smaller research teams and individual creators to experiment with this technology without the need for extensive voice libraries.
The applications of DDSP are incredibly diverse. Imagine crafting realistic voiceovers for audiobooks, designing distinct character voices for animated productions, or creating engaging podcasts with custom soundscapes. It even surpasses conventional text-to-speech systems that often fall short with their robotic-sounding output. DDSP opens doors for more individualized voice experiences with emotional depth, perfect for story-driven scenarios.
However, as with many advancements, there are some practical hurdles. DDSP's strength lies in real-time audio processing, but this requires significant computational power. It's a limitation for devices with lower processing capabilities.
When applied to voice cloning, DDSP offers the remarkable ability to rapidly adapt to new voices or stylistic shifts. By tweaking specific parameters, we can generate bespoke voiceovers in a fraction of the time compared to traditional techniques.
While the accuracy of DDSP-based voice cloning is impressive, it inevitably raises critical questions. Its potential for creating remarkably realistic deepfakes or unauthorized impersonations is undeniable. This technology's ability to manipulate voice has sparked heated discussions within the industry and broader society regarding the ethical implications of its use. It emphasizes the need for careful considerations and possibly even regulations moving forward.
Neural Voice Synthesis The Convergence of AI and Voice Cloning in 2024 - Few-Shot Learning Enables Rapid Voice Cloning
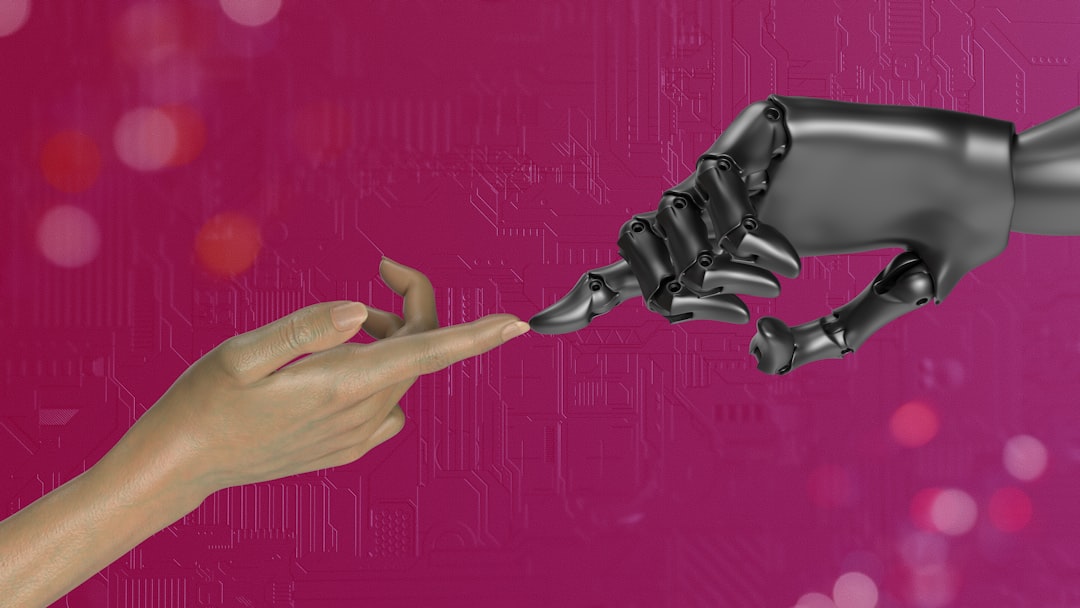
The ability to clone a voice with just a small number of audio samples, known as few-shot learning, has become a significant development in voice synthesis. This advancement drastically reduces the amount of data needed to adapt to new speakers, making voice cloning a much faster and more accessible process. Furthermore, it's opening the door to creating multilingual voices, which is a huge plus for fields like audiobook production and podcasting where diverse language options are increasingly important.
Current techniques leverage concepts like meta-learning and multimodal learning to enhance efficiency and accuracy in voice cloning. However, challenges persist. Maintaining the natural rhythm and intonation (prosody) of a speaker while also ensuring high-quality voice output remains a hurdle.
Despite these hurdles, few-shot learning is driving advancements in personalized audio experiences, particularly within the emerging landscape of AI-driven voice synthesis. The future holds exciting possibilities for how we create and interact with synthetic voices, spanning applications from interactive storytelling to personalized audio experiences. While progress is being made, the ethical implications of increasingly realistic voice cloning should continue to be a topic of discussion.
Few-shot learning has emerged as a powerful technique in voice cloning, enabling the creation of personalized voices using just a handful of audio samples. This drastically reduces the time and computational resources needed for training, allowing creators to more quickly develop unique voice profiles for diverse applications such as audiobooks and podcasts. It's quite remarkable how rapidly a model can learn the essence of a voice with limited data.
Intriguingly, these few-shot learning systems can sometimes capture subtle stylistic nuances, like emotional tone or speech patterns, after processing only a few audio examples. This opens up exciting possibilities for voice actors and audiobook producers to craft characters with distinct vocal personalities in a short amount of time. Imagine the potential for rapidly developing a whole cast of characters with varied voices for a complex narrative.
The democratization of access to high-quality voice technology is another exciting facet of few-shot learning. Smaller creative teams and individual creators can now explore advanced voice synthesis capabilities without needing substantial resources typically associated with traditional methods. This increased accessibility could foster a new wave of creativity in audio production, allowing for more innovative approaches to storytelling and sonic design.
Researchers are actively exploring how few-shot learning can be used to tailor voice clones to specific demographics or target audiences. This capability could enable audiobook and podcast producers to tailor content that resonates more deeply with listeners, making the experience feel more personalized and engaging. It's a promising area of exploration that could have a profound impact on audience connection.
One of the interesting breakthroughs is the ability to generate voice variations, such as uncommon speech patterns or dialects, with minimal training data. This level of versatility can add a new dimension to storytelling in audiobooks and make characters more relatable across different contexts and audience segments. It's fascinating how much variety can be teased out from limited training data.
However, few-shot learning also presents its own set of challenges, especially when it comes to synthesizing a wide range of emotional expressions. Achieving truly natural-sounding emotional variations often requires more comprehensive training data, which might complicate rapid deployment in some scenarios. Balancing the speed of training with achieving nuanced emotional output is an ongoing area of research.
The integration of few-shot learning within existing voice frameworks opens up the potential for more interactive voice applications. This could lead to a future where users can provide real-time feedback that impacts the performance of a voice clone, making it more responsive in conversational scenarios, particularly for podcasts and virtual assistant applications. Interactive voice responses could usher in new forms of engagement and creative possibilities.
Few-shot learning models can sometimes make the process of acoustic adaptation more efficient, enabling voice clones to adapt to the acoustic characteristics of different environments, such as varying noise levels during recordings. This leads to cleaner and more polished audio output across various recording conditions, improving the overall quality of the audio. It's a practical improvement that enhances the usability of the technology.
Beyond voice cloning, the application of few-shot learning is even beginning to find its way into music production. In electronic music, for example, producers can now synthesize unique vocal elements without relying on vast libraries of pre-recorded vocals. This could be a game-changer in terms of the creative flexibility available to musicians. There's a clear potential to unlock new artistic avenues with this technology.
As the technology matures, combining few-shot learning algorithms with advancements like DDSP could usher in a paradigm shift in the creation of virtual assistants. Synthesizing a user-specific voice could potentially become as simple as uploading a few spoken sentences. This personalized user experience could be a catalyst for significant improvements in user interaction. Imagine an assistant with your own voice—it's a compelling glimpse into future possibilities.
Neural Voice Synthesis The Convergence of AI and Voice Cloning in 2024 - Cross-Language Voice Synthesis Breaks Barriers
The ability to synthesize voices across different languages is pushing the boundaries of audio production and offering new ways to connect people globally. Techniques like the VALLE X model demonstrate the potential of neural codecs to generate high-quality speech in various languages while retaining the individual's unique voice characteristics, including emotional nuances. While still facing challenges in achieving the same level of naturalness as monolingual voice synthesis, particularly in preserving speaker identity, cross-language voice cloning is steadily advancing. This development is particularly beneficial for industries like audiobook production and podcast creation where accessing diverse language options is increasingly important.
The advancements in semantic token use and audio encoding methods highlight the continuous evolution of these technologies toward a more seamless and authentic replication of human vocal interactions. This is transforming how we create and experience audio, leading to new possibilities in sound design and audio storytelling. Although some obstacles remain in achieving completely fluent cross-language voice synthesis, the potential for breaking down language barriers and fostering wider accessibility in audio content is undeniable. This burgeoning field continues to alter how we conceive of language and creative applications in audio production.
Cross-language voice synthesis is a fascinating area of research, pushing the boundaries of what's possible with artificial intelligence and voice technology. It's quite remarkable how these systems can now translate a speaker's voice from one language to another, often preserving the original speaker's unique characteristics.
One of the most interesting developments is the emergence of neural codecs that can synthesize high-quality speech in a target language using just a single spoken prompt in the source language. While it's still a developing area, these models are increasingly capable of capturing a speaker's emotional tone and acoustic features, making it possible to create audiobooks or podcasts with a voice that feels consistent regardless of the language it's speaking.
A significant hurdle for this field is that traditional voice cloning methods often require massive amounts of training data from a specific speaker. It can be a significant bottleneck for expanding into multiple languages. Researchers are experimenting with novel prompt-based approaches to synthesize speech, often combining source and target language prompts to achieve the desired output. However, the quality of the synthesized speech in cross-lingual applications isn't quite on par with monolingual text-to-speech systems, particularly in maintaining a consistent speaker identity.
The overarching goal is to move beyond basic text-to-speech and develop systems that directly imitate and replicate the user's voice for multiple languages. This direction aligns with the growing trend of AI-powered voice cloning technologies. We're seeing innovations that let users effectively "speak" foreign languages using their own voice. It's a remarkable feat of engineering.
Leveraging pre-trained models is accelerating the progress in this field. It can significantly cut down on the time it takes for a voice synthesis system to adapt to new languages. We're also seeing advancements in synthesizing voices from relatively few audio samples, making voice cloning more accessible and versatile.
There's also a focus on improving the overall quality and naturalness of the generated voices. It involves using sophisticated encoding techniques like Meta's audio codebooks to better manage the nuanced aspects of cross-lingual sound production. By incorporating more sophisticated semantic information, systems can more accurately capture the intended meaning, enhancing the overall quality and intelligibility of the output.
However, it's crucial to acknowledge that there's still much to improve. Cross-language speech synthesis often faces challenges in maintaining the fidelity and nuances of the source voice. This area of study is constantly evolving. Researchers continue exploring the use of semantic tokens and advanced audio codebooks to improve the naturalness of cross-lingual voice synthesis. It's a complex and multifaceted challenge.
As this field progresses, the potential for impactful applications will only grow. Imagine audiobooks and podcasts that effortlessly switch between languages, or characters that can seamlessly transition between dialects or accents within a story. The intersection of AI, voice cloning, and multilingual capabilities is undeniably exciting. It presents numerous opportunities for creating richer and more immersive experiences across various creative applications.
Neural Voice Synthesis The Convergence of AI and Voice Cloning in 2024 - Fine-Grained Control Over Synthesized Speech Emerges
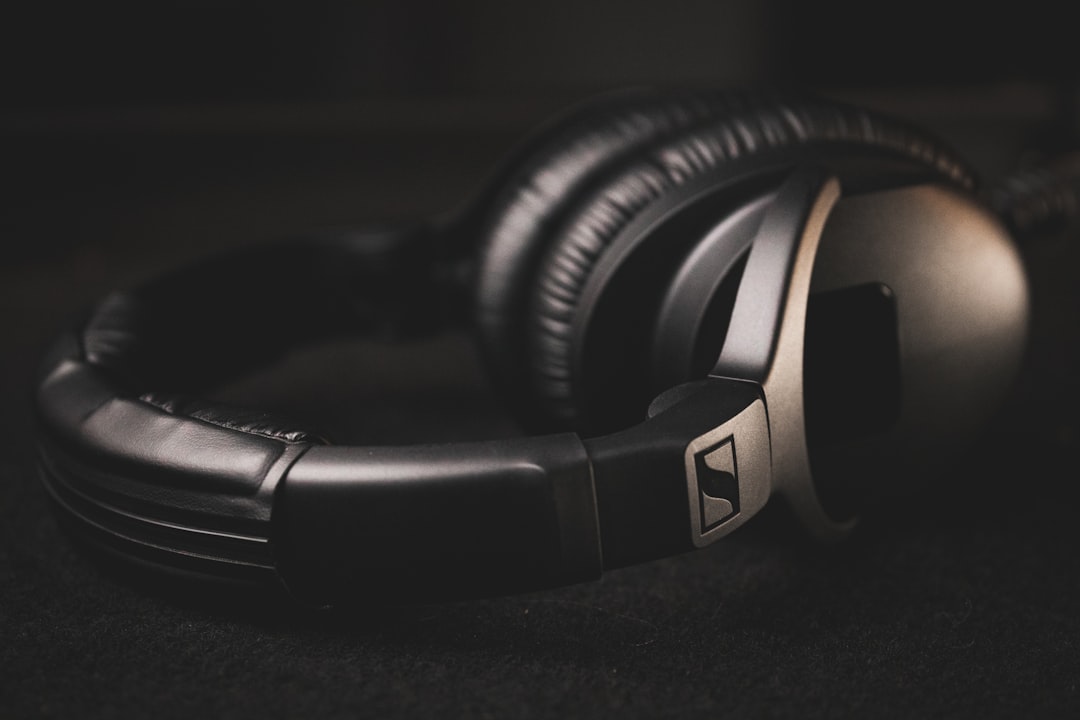
The field of neural voice synthesis is witnessing a remarkable evolution, marked by the ability to exert increasingly fine-grained control over the synthesized speech output. This newfound control empowers creators to manipulate various aspects of the synthetic voice, going beyond simple voice cloning. Now, it's possible to condition the voice synthesis models on elements like speaker characteristics, pitch patterns, and even stylistic details, leading to much more expressive and nuanced synthetic voices. This fine-tuning of voice qualities is a game changer for applications requiring emotional depth, like audiobook narratives and engaging podcast productions, where the realism and emotional impact of the voice are essential for captivating listeners.
The PiCoVITS model serves as an example, demonstrating the capability to precisely control the emotional tone of a synthetic voice by using pitch contours. This suggests we're moving towards the ability to craft vocal performances, rather than merely replicating a voice. While these advancements offer remarkable possibilities, they also highlight the growing need for discussions around the implications of this technology. Concerns about the authenticity of synthetic voices and the ethical boundaries surrounding their use in media and audio production become increasingly relevant. The future of voice synthesis hinges on navigating the balance between creative potential and responsible innovation in this rapidly evolving field.
The field of synthesized speech is experiencing a surge in capabilities, offering a level of precision previously unimaginable. We're moving beyond simply generating speech to achieving fine-grained control over its emotional nuances. Instead of a binary 'happy' or 'sad' setting, we can now craft subtle shifts in vocal tone to convey a vast array of emotions, enriching the narrative depth of audiobooks and podcasts.
Moreover, we're seeing techniques that can convincingly replicate regional accents or dialects in synthesized voices. This is a game-changer for projects seeking authentic representation of linguistic diversity. Imagine audiobooks where characters speak with a range of accents, truly bringing the narrative to life.
Real-time customization of synthesized voices is becoming a reality, opening new avenues for live audio interactions. This means that voice properties could be adjusted on the fly, potentially allowing audience interaction to directly impact the characteristics of a voice during a live podcast or interactive storytelling experience. It's a fascinating glimpse into a future where the audience is part of the audio design process.
DDSP models are evolving to incorporate ambient soundscapes into the synthesized audio. This means we can create more immersive listening experiences in audiobooks by contextualizing a character's voice within a specific environment – be it a quiet library or a bustling street corner. This creates a more robust audio landscape that enhances listener engagement.
Furthermore, synthesized voices are becoming more adaptable for interactive media, such as video games. Voice clones can be integrated to create unique, dynamic characters whose voices evolve and adapt throughout gameplay, pushing the boundaries of immersive audio experiences.
The ability to preserve a speaker's unique characteristics while translating their voice into different languages is a remarkable achievement. This ensures that a voice maintains its distinct personality across language barriers, a boon for the creation of globally accessible audiobooks.
Recent advancements also allow for a detailed replication of speech dynamics, capturing the subtleties of timing and emphasis in spoken language (prosody). This attention to detail gives synthetic voices a more conversational, natural feel, making them increasingly difficult to distinguish from human voices in everyday contexts.
Smaller datasets are now sufficient for creating effective voice clones. This increased accessibility is democratizing high-quality voice production, allowing individual creators and small teams to produce their own distinct voice profiles without the need for vast, pre-existing voice libraries.
The dream of personalized voice assistants tailored to an individual's unique voice is edging closer to reality. The ability to generate a custom voice from just a few spoken sentences could be a significant leap forward in user interaction with technology. Imagine interacting with your own virtual assistant, utilizing your very own voice.
The ability to seamlessly transition between languages within a single sentence while maintaining vocal characteristics is a substantial step forward. This technology could revolutionize language learning applications and multilingual podcasts, connecting a broader audience to diverse content through a familiar, personalized voice.
The continuous evolution of synthesized speech technology is leading to a future where the possibilities are boundless, offering unprecedented levels of realism and expressiveness. However, with these exciting advancements, come equally important discussions regarding ethical considerations and responsible use. We must ensure that the immense potential of these technologies is harnessed in a manner that benefits all of humanity.
Neural Voice Synthesis The Convergence of AI and Voice Cloning in 2024 - ECoG Framework Interprets Neural Signals for Speech
The ECoG framework offers a novel approach to speech synthesis by directly interpreting brain signals. Utilizing deep learning, it decodes electrocorticographic (ECoG) signals from the brain's cortex and converts them into parameters that can be used to generate speech. This system effectively bridges the gap between neural activity and spoken language, enabling the reconstruction of sentences from brain signals alone. The framework leverages a combination of ECoG decoders and a speech synthesizer to achieve this goal. This technology is particularly promising for those with neurological conditions who may struggle with traditional communication methods, offering a potential avenue to restore or improve speech abilities. Furthermore, this research contributes to the broader field of voice synthesis by revealing how neural activity directly relates to speech production and how this relationship can be leveraged for more sophisticated audio generation. While still in its early stages, the ECoG framework shows potential for fostering new types of brain-computer interfaces and enriching the potential of AI-powered voice cloning and synthesis in a variety of sound production applications, such as audiobook creation and podcasting. Continued research and advancements could lead to more intuitive and personalized communication methods in the future. However, it's important to acknowledge the invasive nature of ECoG and the inherent challenges related to reliably interpreting complex neural signals for speech.
Electrocorticography (ECoG) offers a unique window into brain activity, providing a more detailed look at neural signals compared to methods that don't involve direct contact with the brain. This high-fidelity view of brain activity is crucial for tasks like voice cloning, where subtle variations in vocal patterns are important.
ECoG frameworks can decode the neural signals linked to speech production in real time. This allows for swift feedback to voice synthesis systems, a crucial feature for creating dynamic experiences in scenarios like live podcasting or interactive audiobook formats.
ECoG has a spatial resolution that surpasses EEG (electroencephalography) in detail but doesn't achieve the level of functional magnetic resonance imaging (fMRI). This means it's especially adept at identifying specific brain regions involved in speech, allowing researchers to personalize voice synthesis by focusing on neural patterns linked to individual vocal characteristics.
Integrating ECoG data with audio processing can greatly improve the accuracy of voice cloning. By combining neural responses with audio output, systems can better align synthetic speech with a speaker's specific pronunciation style and emotional expression.
Using ECoG, researchers are creating predictive models that can guess a speaker's next sound (phoneme) based on prior brain activity. This could lead to incredibly smooth and natural-sounding synthesized speech, making it closer to human conversation.
Patients with speech difficulties could potentially benefit greatly from future ECoG-based technologies. These systems might not only mimic their original voice but also provide a means of communication that feels authentic and improves their overall quality of life.
However, the accuracy of ECoG raises ethical concerns. If these technologies become capable of generating speech from solely neural signals, questions about consent and the ownership of synthetic voices will likely arise. This is particularly relevant in fields like healthcare where sensitive information is involved.
ECoG-derived insights into emotional tone and speech pacing could revolutionize audiobook production. Imagine incredibly immersive storytelling that fully captures the emotional content of a narrative.
As ECoG research continues, the tools built upon it might become more accessible for smaller teams, leading to more democratized high-quality voice synthesis, a similar shift to what's happened with few-shot learning in voice cloning.
It's likely that ECoG will be integrated with more sophisticated AI models in the future. This might allow systems to create voices with incredibly nuanced emotional expressions based on real-time monitoring of brain activity, pushing personalized audio experiences to a whole new level.
Neural Voice Synthesis The Convergence of AI and Voice Cloning in 2024 - Ethical Challenges of Voice Cloning Technology
The rapid advancement of voice cloning technology brings forth a set of complex ethical dilemmas. The ability to replicate human voices with remarkable accuracy carries the potential for misuse, leading to concerns about privacy, authenticity, and the spread of misinformation. Creating realistic deepfakes, for example, could lead to identity theft, defamation, or the manipulation of public opinion through fabricated audio content. These possibilities raise questions about how to ensure consent and prevent unauthorized use of individuals' voices, particularly within contexts like audiobook production and podcasting. Maintaining trust in media and communication becomes increasingly challenging as the line between genuine and synthetic audio blurs. Moving forward, establishing guidelines and potentially regulatory measures will be crucial to balancing the exciting potential of voice cloning with the need to protect individuals and uphold ethical standards across diverse areas of audio production. The future of voice cloning hinges on fostering responsible development and utilization, ensuring the technology benefits society while safeguarding individual rights.
The remarkable advancements in voice cloning technology, particularly with the rise of DDSP models, present a fascinating intersection of possibility and ethical concern. The ability to generate incredibly realistic synthetic voices has led to a surge in applications, including audiobook production and podcast creation, where the pursuit of immersive audio experiences is paramount. However, this level of realism also raises questions about the authenticity of audio content in the digital landscape.
The ease with which deepfake audio can now be produced challenges our traditional notions of trust in media. We face the daunting task of discerning between genuine and manipulated audio, and this is particularly crucial in domains where trust is paramount, like news dissemination and public discourse. While regulations have historically struggled to keep pace with the rapid evolution of technology, the growing potential for misuse – including unauthorized impersonations and the spread of misinformation – urgently calls for a comprehensive reevaluation of legal frameworks specifically designed to address voice cloning.
The ethical implications extend beyond the simple replication of a voice. The capacity to synthesize nuanced emotional expressions, such as sarcasm or irony, in synthetic voices presents a new frontier in audio production. We face a dilemma around transparency in creative uses of this technology. Should creators be obligated to disclose the use of AI-generated emotional expression in their work? It seems the lines between what is considered 'authentic' in storytelling are increasingly blurry.
Further, the potential to imbue synthetic voices with cultural accents and dialects necessitates careful consideration of cultural sensitivity. There's a risk of amplifying existing stereotypes or misrepresenting marginalized communities if this technology is not used thoughtfully. It underscores the critical need to promote the responsible use of these tools and acknowledge the impact they have on how voices and cultures are represented in the digital realm.
The issue of user consent and data privacy looms large as well. When a person's voice is captured and used to generate a synthetic voice, who owns that voice? What protections should be in place to prevent the unauthorized use of a person's unique vocal traits? These are critical questions for the development of clear and ethically sound practices within the audiobook and podcast industries.
The potential for identity theft also demands our attention. The frightening prospect of using voice cloning for malicious purposes—such as impersonating someone in fraudulent transactions—is a genuine concern. The need for robust countermeasures to prevent such activities is increasingly important as this technology becomes more readily accessible.
While readily available stock voices might simplify the production of audiobooks and podcasts, we need to consider the implications of this homogenization of voice. It could lead to a less diverse and more generic soundscape in audio production, potentially diminishing the value placed on unique creative voices. The accessibility of this technology also raises concerns about equity. How can we ensure that all creators have fair access to voice cloning capabilities, thus preventing the creation of additional barriers to entry within the field of audio production?
The convergence of voice cloning with deepfake video adds another layer of complexity to the ethical concerns. A synthetic voice paired with manipulated visual content can dramatically increase the persuasiveness of misinformation. This highlights the importance of a coordinated approach to addressing these technologies, and this will require input from a wide range of researchers and regulatory bodies.
Finally, we must consider the impact on voice actors. As synthetic voices continue to evolve in quality and adaptability, questions about the future of human talent in voice work are emerging. It is crucial to have open conversations about the ethical and societal impact of these developments and to consider ways that voice actors can navigate the changing landscape of audio production.
In conclusion, while voice cloning technology holds incredible promise, it is imperative that we address these emerging ethical challenges proactively. The development of transparent and ethically sound practices, as well as carefully considered regulatory frameworks, will be critical for ensuring that this technology serves humanity and not further exacerbate existing societal inequalities. The future of audio production, much like the broader AI landscape, will depend on a commitment to harnessing the benefits of innovation while minimizing the risks associated with its misuse.
More Posts from clonemyvoice.io: