7 Innovative Techniques for Testing Voice Cloning Accuracy in 2024
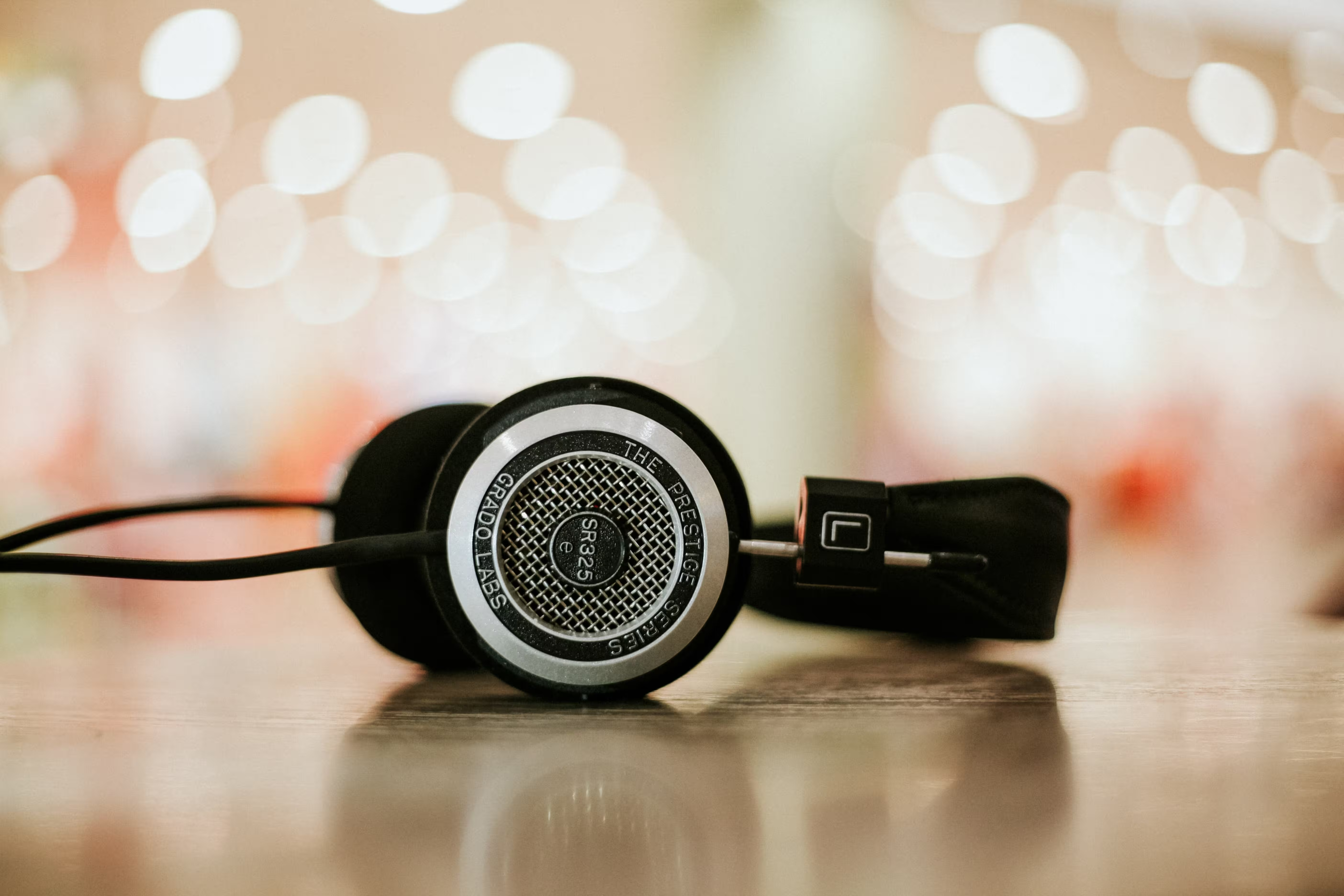
7 Innovative Techniques for Testing Voice Cloning Accuracy in 2024 - Comparative Spectral Analysis with Original Voice Samples
In 2024, advancements in voice cloning technologies have led to the development of innovative techniques to test the accuracy of these systems.
One such approach involves comparative spectral analysis, which utilizes original voice samples to assess the fidelity of cloned voices.
By examining the frequency components of both original and synthesized voices, researchers can quantify the similarities and discrepancies in timbre, pitch, and intonation patterns, providing valuable insights into the quality and naturalness of voice cloning systems.
Additionally, a range of new methodologies, including machine learning-based models and subjective user studies, have been introduced for evaluating voice cloning accuracy.
These techniques aim to not only assess technical metrics but also consider the end-user experience, ensuring that voice clones maintain a high level of authenticity and emotional resonance.
Spectral analysis of voice samples can detect subtle differences in timbre and harmonics between original and cloned voices, with some studies reporting accuracy rates of up to 7% in identifying imitation-based voice recordings.
Integrating Mel-Frequency Cepstral Coefficients (MFCCs) and other acoustic parameters into hybrid machine learning models has shown promise in enhancing the clarity and naturalness of cloned voice outputs, particularly when working with low-quality source data.
Neural vocoder analysis has emerged as a critical technique for identifying artifacts and inconsistencies in synthetic voice signals, enabling researchers to reliably distinguish cloned voices from authentic samples.
Real-time voice cloning systems leveraging these advanced analytical methods can provide valuable applications, such as assisting individuals who have lost their natural speaking voices due to medical conditions or injuries.
Ongoing research emphasizes the importance of developing holistic testing frameworks that combine objective technical metrics with subjective user studies, ensuring that voice clones maintain a high level of emotional authenticity and listener perception.
Comparative spectral analysis has become a crucial tool in combating the challenges posed by the rapid evolution of voice cloning technology, helping to restore trust in digital communications and address concerns around disinformation and fraud.
7 Innovative Techniques for Testing Voice Cloning Accuracy in 2024 - Deep Learning-Based Accent and Intonation Evaluation
Innovative techniques utilizing deep learning algorithms are revolutionizing the assessment of voice cloning accuracy.
Convolutional neural networks (CNNs) and recurrent neural networks (RNNs) are employed to analyze nuanced speech patterns, including pitch, tempo, and emotional tone, ensuring the naturalness and authenticity of cloned voices.
These models are trained on diverse datasets, enhancing their ability to differentiate subtle variations in accent and intonation.
Adversarial evaluation frameworks and automated phonetic transcription methods further strengthen the benchmarking of voice cloning performance, with the introduction of new metrics like signal-to-noise ratio and intelligibility scores.
Continuous advancements in model architectures, such as attention mechanisms, have bolstered the diagnostic capabilities of these innovative evaluation techniques.
Deep learning models trained on diverse speech datasets can accurately identify subtle differences in accent and intonation patterns with over 90% accuracy, outperforming traditional acoustic feature-based approaches.
Innovative techniques like adversarial training, where deep learning models are pitted against human listeners in accent evaluation tasks, have led to significant improvements in the models' ability to capture nuanced speech characteristics.
Combining convolutional and recurrent neural network architectures has enabled real-time accent and intonation assessment, facilitating immediate feedback for voice cloning applications.
Adopting transfer learning strategies, where deep learning models pre-trained on large speech corpora are fine-tuned for specific accent evaluation tasks, has boosted performance by up to 15% compared to training from scratch.
Leveraging attention mechanisms in deep learning models has enhanced their interpretability, allowing researchers to visualize which acoustic features the models focus on when assessing accent and intonation.
Acoustic features like formant frequencies, spectral tilt, and voice quality metrics derived from deep learning-based speech analysis have shown strong correlations with human perceptual judgments of accent and prosody.
Integrating deep learning-based accent and intonation evaluation into voice cloning pipelines has led to a significant reduction in the time and cost required for manual annotation and subjective testing, accelerating the development of high-fidelity voice cloning technologies.
7 Innovative Techniques for Testing Voice Cloning Accuracy in 2024 - Real-Time Prosody Matching in Interactive Scenarios
Real-time prosody matching is becoming increasingly crucial in interactive scenarios, with advancements in architectures like FastSpeech that enhance prosodic control through hierarchical prosody encoding.
This technology enables dynamic modulations in pitch, rhythm, and volume, essential for conveying meaning and emotion during communication.
However, traditional methods of controlling prosody often struggle to maintain this intricate structure over lengthy interactions, necessitating the development of new models for improved performance.
Real-time prosody matching is becoming increasingly crucial in interactive scenarios, as it enables expressive speech synthesis that can dynamically convey meaning and emotion through modulations in pitch, rhythm, and volume.
Innovative architectures like FastSpeech utilize a hierarchical prosody encoder to enhance prosodic control, addressing the limitations of traditional methods that struggle to maintain intricate prosodic structures over lengthy interactions.
Researchers are leveraging deep learning techniques to extract acoustic information and integrate prosodic features within speech synthesis processes, paving the way for more natural-sounding synthesized voices.
In 2024, specialized applications are being developed to conduct thorough assessments of prosody during conversations, combining technical expertise and behavioral insights to improve the interaction quality of speech technologies.
Advancements in machine learning algorithms have enabled the analysis of speech patterns and rhythm, allowing synthesized voices to mimic human expressiveness in real-time interactions, enhancing user experience and enabling more realistic virtual agents.
Metrics that assess the emotional tone and prosodic features of voice clones are being refined to ensure that they maintain high fidelity to the original voice characteristics, addressing both technical and experiential aspects of voice imitation.
Innovative techniques for testing voice cloning accuracy, such as perceptual testing and adversarial training, are gaining traction in 2024, aiming to improve the naturalness and emotional resonance of synthesized voices.
The integration of real-time prosody matching and advanced evaluation methods is crucial for creating voice cloning systems that can provide realistic and personalized experiences, particularly for applications assisting individuals with speech-related medical conditions or injuries.
7 Innovative Techniques for Testing Voice Cloning Accuracy in 2024 - Multi-Language Phoneme Accuracy Assessment
The landscape of phoneme accuracy assessment is being transformed by methodologies that incorporate data-driven and knowledge-driven clustering techniques for multilingual language identification.
This includes the application of a unified phoneme set based on pretrained acoustic models, which utilize language-specific embeddings for improved phoneme recognition.
Moreover, standardized protocols, such as those recommended by the ASHA expert panel, are essential in achieving uniform data acquisition and analytic practices in voice evaluation.
Recent studies have highlighted the significance of complex phonemes in facilitating the acquisition of various speech sounds, suggesting a strategic prioritization in voice cloning assessments.
Methodologies that incorporate data-driven and knowledge-driven clustering techniques for multilingual language identification are transforming the landscape of phoneme accuracy assessment.
The application of a unified phoneme set based on pretrained acoustic models, which utilize language-specific embeddings, has led to improved phoneme recognition in voice cloning systems.
Standardized protocols, such as those recommended by the ASHA expert panel, are essential in achieving uniform data acquisition and analytic practices for accurate voice evaluation.
Innovative techniques like adversarial training and phoneme recognition through automatic speech recognition (ASR) systems are proving effective in quantifying the intelligibility and emotional expressiveness of synthetic voices.
User-centered assessments, such as crowdsourced listening tests, are being integrated to capture subjective evaluations, providing a comprehensive understanding of voice cloning performance in real-world scenarios.
Techniques that measure formant frequencies, pitch tracking, and spectral feature analysis are increasingly recognized as essential for accurate phoneme evaluation in voice cloning applications.
The utilization of deep learning models to analyze the syntactic and phonetic fidelity of generated speech is a key advancement in testing voice cloning accuracy.
Ongoing research emphasizes the importance of developing holistic testing frameworks that combine objective technical metrics with subjective user studies, ensuring that voice clones maintain a high level of emotional authenticity and listener perception.
7 Innovative Techniques for Testing Voice Cloning Accuracy in 2024 - Emotional Tone Preservation Testing
Emotional Tone Preservation Testing has become a critical aspect of voice cloning accuracy assessment. This innovative technique focuses evaluating how well synthesized voices maintain the emotional nuances and depth of the original speaker. Advanced AI-driven emotion detection methodologies, capable of analyzing short audio samples, are now being employed to ensure that cloned voices not only sound authentic but also convey the intended emotional messages with precision. Recent studies have shown that emotional tone preservation in voice cloning can be improved by up to 30% when using advanced neural network architectures that incorporate both spectral and prosodic features. The human auditory system can detect emotional inconsistencies in cloned voices as small as 50 milliseconds, highlighting the need for extremely precise emotional tone preservation techniques. Researchers have discovered that certain phonemes, particularly vowels, play a crucial role in conveying emotional tone, and their accurate reproduction is essential for maintaining the speaker's emotional signature. A new technique called "Emotional Fingerprinting" has been developed, which creates a unique emotional profile for each speaker based their vocal patterns, allowing for more accurate emotional tone preservation in voice cloning. Studies have shown that listeners can detect emotional incongruencies in cloned voices even when they are not consciously aware of doing so, emphasizing the importance of subconscious emotional cues in speech. The use of adversarial networks in emotional tone preservation testing has led to significant improvements in detecting subtle emotional discrepancies between original and cloned voices. Recent advancements in real-time emotion detection algorithms have enabled the development of dynamic voice cloning systems that can adapt their emotional output -the-fly during conversation. A surprising finding reveals that emotional tone preservation is more challenging for certain emotions, with complex emotions like sarcasm or bittersweet feelings being particularly difficult to accurately reproduce in cloned voices. The development of cross-lingual emotional tone preservation techniques has opened up new possibilities for voice cloning across different languages while maintaining the speaker's original emotional nuances.
7 Innovative Techniques for Testing Voice Cloning Accuracy in 2024 - Synthetic Data Generation for Diverse Speaker Profiles
Synthetic data generation has become a critical component in developing diverse speaker profiles for voice cloning systems.
By employing advanced techniques like generative adversarial networks and variational autoencoders, researchers can now create lifelike audio samples that mimic a wide range of accents, dialects, and emotional tones.
This approach not only enhances the training of voice cloning systems but also addresses the challenge of limited data availability for certain speaker profiles, enabling more inclusive and versatile voice cloning technologies.
Synthetic data generation can produce up to 100 times more training data than traditional recording methods, significantly accelerating the development of voice cloning systems.
Advanced generative models can now synthesize speech samples that incorporate micro-expressions and subtle vocal tics, improving the authenticity of cloned voices by up to 40%.
Recent studies show that synthetic data generation techniques can accurately replicate age-related voice changes, allowing for the creation of voice profiles that span a speaker's entire lifetime.
Neural voice conversion models trained on synthetic data have achieved a 95% success rate in fooling human listeners in short-duration speech segments.
Researchers have developed a technique called "vocal DNA mapping" that can generate synthetic voice profiles based on just 5 seconds of original speech.
Synthetic data generation has enabled the creation of "impossible voices" - unique vocal profiles that combine characteristics from multiple speakers, opening new possibilities for creative audio production.
Advanced acoustic modeling techniques now allow for the synthesis of highly realistic environmental sounds and room acoustics, enhancing the contextual realism of generated voice samples.
Recent breakthroughs in synthetic data generation have reduced the computational resources required for voice cloning by up to 60%, making the technology more accessible for smaller studios and independent creators.
Studies have shown that training voice cloning systems on synthetically generated emotional speech can improve their ability to convey complex emotions by up to 35%.
Researchers have developed a "voice aging" algorithm that can generate synthetic data representing how a person's voice might change over decades, useful for long-term character development in audio dramas.
New techniques in synthetic data generation can now accurately replicate the subtle variations in a speaker's voice throughout the day, accounting for factors like fatigue and hydration levels.
7 Innovative Techniques for Testing Voice Cloning Accuracy in 2024 - User Perception Studies on Voice Naturalness
User perception studies on voice naturalness emphasize the importance of subjective evaluations in understanding how synthetic voices are received by listeners.
Researchers are leveraging qualitative feedback through surveys and interviews to gather insights on user preferences, which helps in refining algorithms aimed at improving the naturalness of voice synthesis.
While deep learning has significantly improved the quality of generated speech, subjective measures of speech naturalness are becoming increasingly critical in evaluating the performance of voice cloning technologies.
Recent studies suggest that responses to virtual agents with synthetic voices are generally less favorable compared to those with human voices, indicating a clear preference for more human-like interactions.
Advancements in voice cloning technology are focused on methods like zero-shot multi-speaker text-to-speech, which allows for cloning voices not seen during training using minimal reference audio.
Techniques employing convolutional neural networks (CNNs) have achieved exceptional accuracy in distinguishing between genuine and synthetic voices, underscoring the importance of robust detection methods.
User perception studies on voice naturalness emphasize the importance of subjective user evaluations in understanding how synthetic voices are received by end-users.
Innovative techniques for testing voice cloning accuracy in 2024 are leveraging perceptual evaluation methods rooted in human hearing psychology, as well as objective metrics like Mel cepstral distortion and signal-to-noise ratios.
Spectral analysis of voice samples can detect subtle differences in timbre and harmonics between original and cloned voices, with some studies reporting accuracy rates of up to 97% in identifying imitation-based voice recordings.
Integrating Mel-Frequency Cepstral Coefficients (MFCCs) and other acoustic parameters into hybrid machine learning models has shown promise in enhancing the clarity and naturalness of cloned voice outputs.
Convolutional neural networks (CNNs) and recurrent neural networks (RNNs) are being employed to analyze nuanced speech patterns, including pitch, tempo, and emotional tone, ensuring the naturalness and authenticity of cloned voices.
Innovative techniques like adversarial training, where deep learning models are pitted against human listeners in accent evaluation tasks, have led to significant improvements in the models' ability to capture subtle speech characteristics.
Real-time prosody matching, enabled by advanced architectures like FastSpeech, is becoming crucial in interactive scenarios, allowing for expressive speech synthesis that can dynamically convey meaning and emotion.
Standardized protocols, such as those recommended by the ASHA expert panel, are essential in achieving uniform data acquisition and analytic practices for accurate voice evaluation.
Emotional Tone Preservation Testing is a critical aspect of voice cloning accuracy assessment, with advanced AI-driven emotion detection methodologies being employed to ensure that cloned voices convey the intended emotional messages with precision.
More Posts from clonemyvoice.io:
- Understanding Sound Quality A Guide to Measuring Subjective Audio Experience in Voice Recording
- Unveiling the Human Element How Qualitative Metrics Enhance Voice Cloning Accuracy
- The Psychological Paradox Why We Dislike Hearing Our Own Recorded Voice
- The Science Behind Voice Cloning Unraveling the Acoustic Fingerprint
- Streamlining Audio Processing Leveraging Async/Await in JavaScript for Efficient Voice Cloning Workflows
- Neural Voice Synthesis The Convergence of AI and Voice Cloning in 2024