Get amazing AI audio voiceovers made for long-form content such as podcasts, presentations and social media. (Get started for free)
Voice Cloning Technology Unlocking Audio Authentication in the Age of Digital Art Sales
Voice Cloning Technology Unlocking Audio Authentication in the Age of Digital Art Sales - Sound Signature Authentication Using Quantum Pattern Recognition
Quantum pattern recognition offers a fresh perspective on sound signature authentication, especially relevant to the rise of voice cloning technology. Traditional voice authentication techniques, relying on features like voice biometrics, are demonstrably susceptible to attacks like replaying recorded voices or real-time voice mimicry. The inherent weakness lies in the reliance on patterns readily reproducible by current technology. Quantum approaches propose a shift towards more complex, intricate analyses of audio signals, potentially making it far more difficult to create convincing fakes. This increased complexity aims to dramatically enhance the security of audio content.
Furthermore, integrating AI to detect and flag deepfakes, particularly within the realm of audiobook production or podcasting, highlights the urgent need for heightened security in audio. While voice cloning offers incredible potential in creative spaces, it also carries the risk of misuse. These emerging techniques, employing quantum algorithms and AI, can offer a more robust defence against malicious actors who seek to exploit cloned voices. The continuing development and refinement of these authentication methods will play a key role in ensuring the trustworthiness of audio content, vital for both creators and listeners.
Quantum pattern recognition offers a novel approach to sound signature authentication by utilizing quantum phenomena like superposition and entanglement to dissect intricate audio signals. This contrasts with traditional methods that primarily focus on frequency and amplitude, potentially allowing for a more nuanced understanding of the subtle variations in a person's voice that constitute their unique sound signature.
Instead of sequentially processing audio data, quantum computing can analyze multiple aspects simultaneously, leading to a significant reduction in error rates and a more robust pattern recognition capability. This translates to potentially identifying voice clones with a high degree of accuracy, even when compared against spontaneous speech patterns rather than the pre-recorded phrases common in current systems.
Quantum algorithms offer a dynamic approach to voice authentication by adapting their recognition processes in real-time based on the incoming audio. This responsiveness allows for authentication during live interactions, making it a promising tool for authenticating speakers in areas like podcasting and audio streaming.
The implications of this technology extend to the creative industries. Quantum signature authentication holds the potential to ensure with a high degree of confidence that the voiceovers in audiobooks and podcasts can be traced back to their original creators, even in an environment where voice cloning technologies are becoming increasingly sophisticated.
Quantum data encoding can enable the storage and analysis of a massive number of distinct voice signatures in a compact format. This efficiency can further accelerate retrieval times compared to conventional storage methods, presenting a significant advantage in authentication processes.
Interestingly, quantum pattern recognition techniques may be able to identify the emotional content conveyed in speech. This capability opens up possibilities for creating more complex verification measures that go beyond simply matching a voice to a stored sample.
As quantum technology matures rapidly, we anticipate the development of real-time applications for sound signature authentication. This would allow for verifying speakers' identities during live broadcasts or interactive sessions, setting a new standard for digital media security.
The human voice isn't just a matter of pitch and tone. It's a complex tapestry of individual speech characteristics including rate and phonetic pronunciation. Quantum pattern recognition can effectively analyze this multi-faceted nature of voice, posing a strong deterrent against standard voice replication techniques.
The potential for enhancing security and attribution in audio and voice-related media is immense with quantum techniques. However, it's crucial to acknowledge that these advancements come with the potential for both exciting benefits and new challenges, necessitating careful consideration as these technologies mature.
Voice Cloning Technology Unlocking Audio Authentication in the Age of Digital Art Sales - Audio Hashing Methods for Original Voice Protection
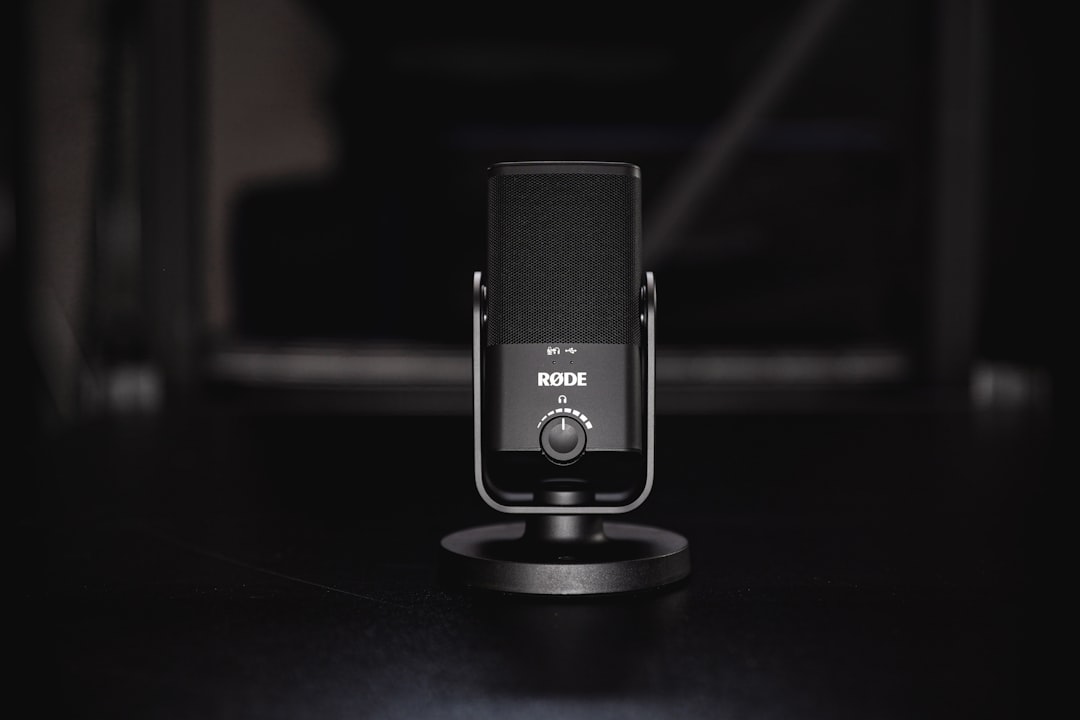
The rise of voice cloning technology necessitates robust methods to protect original voices and ensure audio authenticity. Audio hashing techniques offer a valuable approach to addressing this challenge. These methods create unique digital fingerprints for audio files, enabling the detection of unauthorized replication and fraudulent cloning attempts. The generation of these fingerprints serves as a crucial layer of protection in the authentication of audio content, particularly concerning the increasing prevalence of deepfake audio.
Furthermore, emerging methods like localized audio watermarking provide an additional layer of security by allowing for the detection of AI-generated speech within audio recordings. This targeted approach can help identify and isolate instances of voice cloning, further strengthening audio integrity.
The evolving landscape of audio production, including podcasts, audiobooks, and other voice-driven content, underscores the importance of these advancements. The development and implementation of these sophisticated audio authentication methods are critical to maintaining the trustworthiness of audio content. This is crucial for preserving the integrity of creators' work and ensuring a reliable experience for consumers amidst the ongoing evolution of voice cloning technologies.
Audio hashing methods offer a way to create unique fingerprints for each individual voice, essentially acting like a digital signature for sound. These fingerprints, or hashes, are generated by analyzing various aspects of the audio signal, including pitch, intonation, and even subtle variations in pronunciation. The idea is that even small changes in how someone speaks will result in a different hash, making it extremely difficult for someone to create a fake that matches the original.
Human speech is remarkably complex, extending beyond just pitch and tone. Aspects like rhythm, tempo, and even breathing patterns all contribute to the unique fingerprint of a speaker. Modern audio hashing algorithms are becoming increasingly sophisticated, attempting to capture these various elements, creating a much more robust and complete representation of an individual's voice.
To enhance the precision of these systems, audio hashing can be combined with voice activity detection (VAD). This essentially isolates the segments of audio that contain actual speech, filtering out background noise and other irrelevant sounds. This focusing on intentional speech makes the verification process more accurate, reducing the likelihood of false positives.
However, the effectiveness of hashing is often tied to the quality of the audio recording. Lower quality recordings, a common feature of many digital media formats, can significantly degrade the effectiveness of the hashing process, potentially compromising the reliability of the voice authentication.
Certain approaches, like perceptual hashing, focus on how humans perceive sound rather than the exact numerical representation. This makes the process more resilient to small variations in audio that might arise from compression or the presence of noise. If two audio files sound the same to a human listener, they might produce the same hash, even if their digital representations differ slightly.
Machine learning is also being incorporated into these systems to make them more adaptable to changes in a speaker's voice over time. The systems can essentially learn the natural variations in a person's speech, improving accuracy and ensuring that the voice verification continues to function even as a person's voice changes.
Beyond just security, audio hashing offers possibilities for creative insights. By comparing the voice hashes across different works—say, a series of audiobooks or podcasts—researchers and developers could potentially study how an artist's style and vocal patterns change over time, providing new avenues for artistic analysis.
While visual content can be easily manipulated with editing software, replicating a human voice convincingly requires a deep understanding of the speaker's unique sonic characteristics. Audio hashing stands as a critical tool for distinguishing between original voices and their cloned counterparts, helping to preserve the authenticity of audio-based media.
The increasing use of audio hashing raises questions about copyright and legal implications. As the world becomes ever more saturated with digital audio, establishing a reliable method for authenticating voice content and tracing it back to the original creator will become increasingly crucial for protecting creative ownership.
Finally, the combination of audio hashing and blockchain technology presents an interesting security enhancement. The immutability of blockchain can be used to store the audio hashes, making it extremely difficult to tamper with or falsify voice authentication records. This dual layer of security further strengthens the integrity of the authentication process, making it harder to forge or dispute the originality of a voice in any medium.
Voice Cloning Technology Unlocking Audio Authentication in the Age of Digital Art Sales - Machine Learning Tools Detect Artificial Voice Manipulation
The increasing sophistication of voice cloning technology has brought about a crucial need for tools that can differentiate between genuine and artificially manipulated voices. Machine learning plays a pivotal role in this development, with algorithms being trained to identify instances of deepfake audio. Deepfake voice conversions present ethical challenges like privacy breaches and the potential for malicious misrepresentation, making their detection a pressing concern. The ability to create incredibly realistic synthetic voices necessitates a constant evolution in detection methods, as fraudsters often find ways to circumvent existing safeguards.
Researchers are exploring various techniques, such as deep learning and advanced signal processing, to identify the telltale signs of artificial voices within audio recordings. These techniques aim to expose irregularities within the sound patterns, effectively differentiating them from natural human speech. Given the potential for misuse in creative fields like audio book productions or podcasting, the development of robust and reliable tools to detect manipulated audio is crucial for maintaining trust in digital content and protecting both content creators and audiences. The ongoing race between increasingly advanced voice cloning and detection technologies is an important one, with the future of authentic audio heavily reliant on the ingenuity of researchers in this field.
The human voice is a complex tapestry of over a hundred distinct characteristics, including cadence, pitch fluctuations, and even breathing patterns, all contributing to an individual's unique vocal fingerprint. Machine learning tools are becoming increasingly adept at analyzing these subtleties, allowing them to better identify instances where artificial voice manipulation has been employed. These systems can detect alterations that might escape a human listener's ear, particularly in cases of deepfake voice conversion which poses risks like privacy violations and the potential for misrepresentation.
Furthermore, the subtle temporal patterns within speech—the way we pause and the lengths of our silences—are proving to be another crucial aspect for detection. Machine learning algorithms can exploit these temporal nuances to differentiate between natural speech and AI-generated audio, bolstering their ability to expose manipulated voices. However, it's important to recognize that audio quality plays a critical role in the effectiveness of these detection tools. The heavy compression common in digital audio can obscure vital features, limiting the success of detection and authentication mechanisms.
Naturally, the human voice is not static. An individual's voice can vary due to mood, health, or simply the context of the conversation. Sophisticated detection systems need to incorporate this inherent variability into their algorithms, allowing them to better adapt and distinguish between genuine and cloned voices. We're seeing the emergence of machine learning models capable of performing real-time analysis of audio streams. This real-time authentication is essential for applications like live podcasts and online conferences, where immediate verification of a speaker's identity is necessary.
Some of the most advanced machine learning systems utilize a multi-layered approach to analysis, with different algorithms scrutinizing the same audio segment from various perspectives. Examining aspects like tone, pitch, and rhythm simultaneously creates a more resilient and effective framework for identifying altered voices. Within deep learning, unsupervised learning techniques are emerging as a powerful tool for detecting voice manipulation. These methods allow AI systems to learn and identify new and previously unseen forms of manipulation by analyzing unlabeled audio data.
Certain techniques, such as cross-spectral analysis, examine multiple frequency bands within an audio signal. This approach helps expose inconsistencies that might indicate voice manipulation, significantly improving the reliability of the detection process. These advancements aren't limited to the realm of creative media; the potential applications extend to fields like telecommunications and remote identification, where secure audio authentication is paramount.
There's also a growing interest in using machine learning to decipher emotional nuances conveyed through voice. These emotional recognition capabilities could be integrated into fraud detection systems, providing creators and listeners with warnings if a cloned voice attempts to imitate a specific tone or emotional state that isn't genuine.
The continued development of these AI-driven tools is a crucial area of research. As voice cloning technology becomes increasingly sophisticated, the tools used to combat it will need to keep pace, ensuring that we have the means to protect individuals and maintain the integrity of audio communication in a rapidly evolving digital landscape.
Voice Cloning Technology Unlocking Audio Authentication in the Age of Digital Art Sales - Audio Watermarking Standards for Voice Cloning Platforms
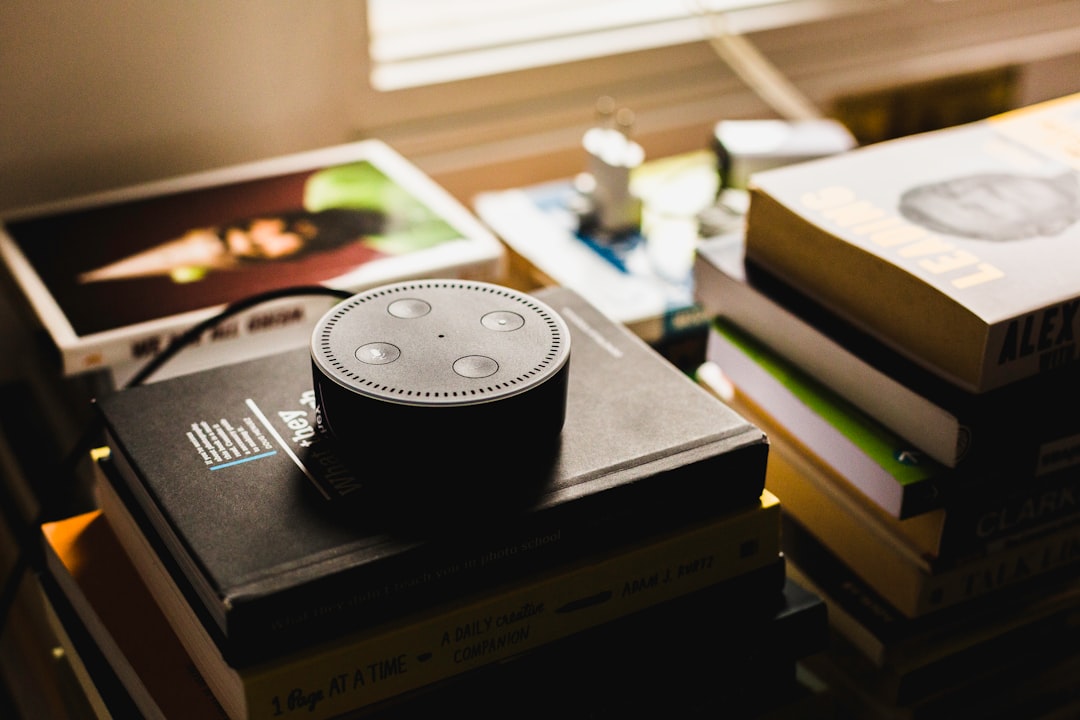
The development of specialized audio watermarking standards like AudioSeal signifies a crucial step forward in navigating the complexities of voice cloning. These standards are specifically designed to identify AI-generated speech, making them particularly relevant in the current landscape where voice cloning poses a growing threat to audio authenticity. AudioSeal is notable for its ability to embed watermarks in a way that's nearly undetectable while simultaneously being resistant to common audio manipulations. This is achieved by generating a unique watermark waveform that's then integrated into the original audio, producing a watermarked version. The watermarking process is optimized for both accurate detection and resistance to audio editing through a combination of techniques that address both perceptual and localization aspects of the audio. This allows watermarks to be decoded in precise, localized increments during detection, ensuring that the origin of any given audio segment can be verified. Importantly, this technology is significantly faster than previous methods, making it a practical solution for large-scale applications where rapid detection is crucial. The increasing need for robust audio authentication, especially in areas like audiobook creation and podcasting, underscores the importance of such standards. These advancements demonstrate a growing focus on ethical considerations within the field of AI-generated audio and can contribute to a more trustworthy environment for creators and consumers. While voice cloning offers incredible creative potential, it also presents risks, making these advanced watermarking techniques increasingly important for maintaining trust and protecting original works.
Audio watermarking, a technique for embedding imperceptible information within audio files, is emerging as a crucial method for ensuring authenticity in the realm of voice cloning. This approach involves subtly embedding a digital "fingerprint" within an audio file, allowing for its identification and authentication without affecting the listening experience. The goal is to maintain the integrity of the audio while creating a secure way to trace it back to its origin.
Modern watermarking algorithms are becoming increasingly sophisticated, adapting their embedding strategies based on the nuances of each audio file. This adaptivity allows the watermark to resist common audio manipulations like compression or re-encoding, a significant challenge in the digital world. By learning from the characteristics of a speaker's voice, systems can improve the robustness of their embedded information, creating watermarks that are more resistant to tampering or removal.
Utilizing multiple audio channels, like those found in stereo or surround sound recordings, enables a more resilient watermarking scheme. This approach makes it more difficult for unauthorized individuals to detect or remove the watermark without significantly degrading the audio. A critical aspect of this research is the development of watermarking techniques capable of withstanding the transformations introduced by voice cloning technologies. Current approaches are designed to ensure the original audio fingerprint survives even after manipulation by AI-driven voice cloning algorithms.
Furthermore, researchers are creating real-time watermark detection systems, making it possible to authenticate audio during live broadcasts. This is particularly important for ensuring the authenticity of podcasts and audiobooks where live speaker verification is desirable.
An important area of development is standardization across different platforms and devices, ensuring the watermarks can be detected and verified regardless of where or how the audio is played. This ensures that the integrity of digital content remains intact across various platforms. Watermarking techniques are incorporating psychoacoustic models to exploit how humans perceive sound. This approach embeds data in ways that are undetectable to a listener but readily revealed by specialized analyses, preserving the audio quality while fulfilling the need for secure identification.
The development of standards has led to important discussions about intellectual property and copyright. Establishing secure methods to link digital audio content to original creators is vital in today's digital ecosystem, particularly for those working with audio-based content.
Watermarking techniques offer a way to quantify the authenticity of audio, creating an index that can be readily verified by those consuming the content. This provides a degree of security for consumers of voice-based content, such as audiobooks or podcasts, as they can have a measure of assurance that the content is authentic.
In conclusion, while voice cloning technologies offer intriguing creative possibilities, they also raise significant concerns about authenticity and the integrity of audio content. Watermarking approaches provide a valuable path toward resolving these concerns, helping to establish a more secure environment for the production and consumption of voice-driven digital content in the rapidly evolving audio landscape.
Voice Cloning Technology Unlocking Audio Authentication in the Age of Digital Art Sales - Digital Rights Management in Voice Generated Content
The rise of voice cloning technology necessitates a renewed focus on Digital Rights Management (DRM) specifically tailored for voice-generated content. The ability to easily replicate voices creates a landscape where issues of authorship and ownership become complex. Without proper safeguards, the potential for misuse and unauthorized cloning becomes a significant threat to the rights of voice actors, podcasters, and audiobook narrators.
Protecting original voices and ensuring their proper attribution are now crucial. Solutions are emerging, like embedding unique audio fingerprints (hashes) or watermarks into recordings. These digital identifiers act as a form of authentication, making it easier to determine if audio has been cloned or manipulated. As the creation and sharing of audio content continues to grow, these DRM solutions will be critical to maintaining a level of trust. Creators and listeners alike will benefit from the reassurance that the voices they interact with are authentic and their rights are being respected in an environment increasingly shaped by artificial voice creation. The effectiveness and wide adoption of these measures will be key to maintaining the integrity and trustworthiness of audio content moving forward.
Digital rights management (DRM) within voice-generated content often combines methods like audio hashing and watermarking to create unique identifiers for each audio file, essentially a digital fingerprint. These identifiers are crucial for tracking the distribution and use of audio files, ensuring the protection of creative ownership without sacrificing audio quality.
The process of embedding watermarks into voice recordings can be so subtle that listeners are usually unaware of their presence, yet they remain effective against various audio alterations. This stealth is essential for preserving audio integrity while maintaining traceability to the original creator.
Modern audio hashing algorithms now take into account aspects such as emotional inflections and conversational dynamics, enhancing the ability to distinguish between cloned and original recordings. This intricate analysis leads to a better understanding of speech patterns that are otherwise hard to replicate accurately.
It's estimated that the human voice exhibits over 100 distinct physical and behavioral traits that make it unique, including pitch, tone, rhythm, and even breathing patterns. This wide range of features allows for more refined audio authentication systems capable of detecting forgeries or alterations with a high degree of precision.
Interestingly, many current watermarking techniques can enhance rather than degrade audio quality. By employing perceptual models, they ensure that even with embedded hidden data, the audio still sounds good to listeners, pushing the boundaries of how we perceive manipulated content.
The rapid evolution of machine learning tools has led to detection systems capable of working in real-time. This capability is particularly important for live events like podcasts or remote conferences, where immediate audio authentication is vital to counter the potential misuse of voice cloning in sensitive situations.
It's crucial to acknowledge that even high-quality audio recordings can introduce challenges for voice authentication methods. Factors such as background noise, recording equipment, and digital compression can all impact the accuracy of identification technologies.
The convergence of audio watermarking and blockchain technology represents a new frontier in DRM, offering immutable records that not only authenticate audio content but also provide a transparent chain of ownership. This ensures that even in a decentralized digital environment, creators can maintain their rights.
To improve the resilience of voice cloning detection, researchers are investigating "cross-spectral analysis," which analyzes multiple frequency bands within an audio signal. This multi-faceted approach allows detection systems to reveal even the slightest discrepancies indicative of voice manipulation.
A fascinating, often overlooked, aspect of voice cloning technologies is their potential to provide "voice signatures" for access control beyond simple audio content. These signatures could eventually be used for secure authentication in sensitive areas such as banking or personal data access, highlighting the vulnerabilities of traditional biometric methods.
Voice Cloning Technology Unlocking Audio Authentication in the Age of Digital Art Sales - Voice Token Verification Systems in Audio Production
Voice token verification systems are gaining prominence in audio production due to the rise of voice cloning. These systems employ advanced algorithms to generate unique digital tokens for each individual's voice, effectively creating a digital fingerprint for sound. This capability is particularly useful in creative applications such as audiobook production or podcast creation, where ensuring authenticity is paramount. By generating a distinctive token for a voice, these systems help combat the unauthorized cloning of voices and increase confidence in the originality of audio content. While these methods offer a powerful approach to audio verification in a world of increasingly sophisticated deepfake audio, they also present challenges. Maintaining the ability to accurately distinguish between genuine and synthetic voices, especially as cloning technology becomes more refined, is a crucial aspect that requires continuous research and development. Ultimately, the future of reliable audio production hinges on the successful development and adoption of robust voice token verification systems that adapt to a rapidly changing digital landscape.
The human voice, with its intricate tapestry of over 100 unique characteristics like pitch, tone, and cadence, presents both a challenge and an opportunity for voice token verification systems. These systems are becoming increasingly complex, needing to consider not just the audible sound but also the subtle nuances of speech dynamics—aspects a cloned voice might miss. For example, analyzing the subtle micro-expressions in a voice's tone and inflection, indicative of emotion, can potentially distinguish genuine emotional expression from its synthesized counterpart. This becomes particularly important in scenarios like audiobook narrations, where conveying emotion authentically is key.
Excitingly, we're seeing the rise of real-time voice token verification, enabling authentication during live broadcasts or podcasts. This technology utilizes machine learning algorithms that analyze audio streams in real-time, making it possible to authenticate speakers on the fly and prevent clones from infiltrating live conversations. However, the efficacy of these systems hinges on the quality of the audio recordings. Background noise or compression can obscure crucial audio features, potentially leading to a higher rate of incorrect authentication.
Researchers are experimenting with cross-spectral analysis, which examines the audio across various frequencies, to identify the telltale signs of voice cloning. This technique can identify manipulations that leave specific imprints on the audio signal, which traditional analysis methods might overlook. Another interesting area is the use of psychoacoustic models within watermarking. These models leverage how humans perceive sound, embedding data within audio imperceptibly while ensuring the audio quality remains pristine for listeners.
The constant evolution of machine learning is essential for voice verification systems. These systems need to learn and adapt to changes in a speaker's voice due to factors like aging, health, or emotional state. This continuous learning ability allows for greater accuracy in voice authentication over time. Furthermore, watermarking technologies like AudioSeal aim to integrate undetectable markers into audio while ensuring resilience against common manipulations like compression, providing a powerful tool for maintaining content authenticity.
Interestingly, audio hashing, traditionally used for security, can also serve as a valuable tool for artistic analysis. By comparing voice hashes across various works—audiobooks or podcasts, for instance—researchers can potentially track changes in an artist's vocal style and emotional delivery over time, providing novel insights. Finally, blockchain's integration with watermarking offers a compelling solution for authenticating voice content and managing ownership. This pairing helps create an immutable record, a digital chain of custody, that protects creators' rights and establishes a reliable lineage for voice content within the ever-evolving digital landscape.
This evolution in voice verification underscores the fascinating interplay between creative innovation and security. As voice cloning continues to advance, robust methods to authenticate and secure audio content will be vital for protecting the integrity of both creative works and communication in the digital age.
Get amazing AI audio voiceovers made for long-form content such as podcasts, presentations and social media. (Get started for free)
More Posts from clonemyvoice.io: