Get amazing AI audio voiceovers made for long-form content such as podcasts, presentations and social media. (Get started for free)
How Voice Cloning Technology Uses Blockchain-Like Consensus for Quality Control
How Voice Cloning Technology Uses Blockchain-Like Consensus for Quality Control - Distributed Audio Verification Network Enables Real Time Quality Checks in Voice Synthesis
Distributed Audio Verification Networks represent a promising development in the field of voice synthesis. They introduce a real-time quality control system, which is becoming vital as AI-generated speech becomes more prevalent. These networks address the growing concern for audio authenticity, particularly in applications like voice cloning, audiobook production, and podcasting. By utilizing a decentralized approach, similar to blockchain technology, they establish a mechanism to validate the quality and integrity of synthesized audio. This helps ensure that the output meets certain predefined standards, thus promoting trust in the generated content. However, as the sophistication of voice synthesis technology advances, the challenge of safeguarding against malicious use necessitates ongoing monitoring and quality assurance within these networks.
Real-time audio quality control is becoming increasingly important in voice synthesis, especially with the rise of voice cloning. Distributed systems, drawing inspiration from blockchain concepts, can offer a solution by facilitating real-time quality checks across multiple nodes. This approach allows for a more robust verification process, ensuring that voice clones maintain a consistent level of quality across different environments and applications.
The speed and precision of these systems surpasses human capabilities in identifying subtle discrepancies in voice patterns, improving efficiency in various audio production tasks. However, the intricacies of human speech, encompassing elements like emotional expression and intonation, still pose significant challenges for current algorithms. Thankfully, recent advancements in machine learning are steadily tackling these obstacles, gradually producing increasingly lifelike synthetic voices.
A significant advantage of this distributed verification is the ability to adapt to diverse linguistic and dialectal nuances. This adaptability is crucial for global markets, enabling voice cloning technology to create more authentic and natural sounding voices across different regions and cultures. The potential benefits also extend to the production process itself. By continuously analyzing audio input, these systems can pinpoint inconsistencies during recording, allowing for immediate correction instead of relying on laborious post-production edits. This feature can streamline the entire workflow, especially in applications like podcast production, where a natural flow of conversation is paramount.
Interestingly, audio quality evaluation can be both subjective and objective. Using a distributed network, it's possible to integrate both perspectives to arrive at a more holistic quality assessment. This involves both the complex algorithms judging aspects like pitch and timbre, and human listeners evaluating the overall naturalness and clarity of the output. Moreover, a key benefit of a decentralized approach is the accessibility it offers to a wider range of creators. Smaller studios and independent producers could potentially benefit from high-quality tools that were previously only available to larger organizations with significant resources.
This method of using distributed audio verification networks with a consensus mechanism enhances the resilience and robustness of voice cloning systems. By removing reliance on single points of failure, the systems can achieve greater stability and reliability, ensuring continued high-quality audio output even in demanding production environments. While there are still hurdles to overcome in this field, this technology holds the promise of democratizing access to sophisticated voice synthesis tools and advancing the quality of synthetic audio across a broad range of applications.
How Voice Cloning Technology Uses Blockchain-Like Consensus for Quality Control - Peer Review System Applies Multiple AI Models to Match Original Voice Parameters
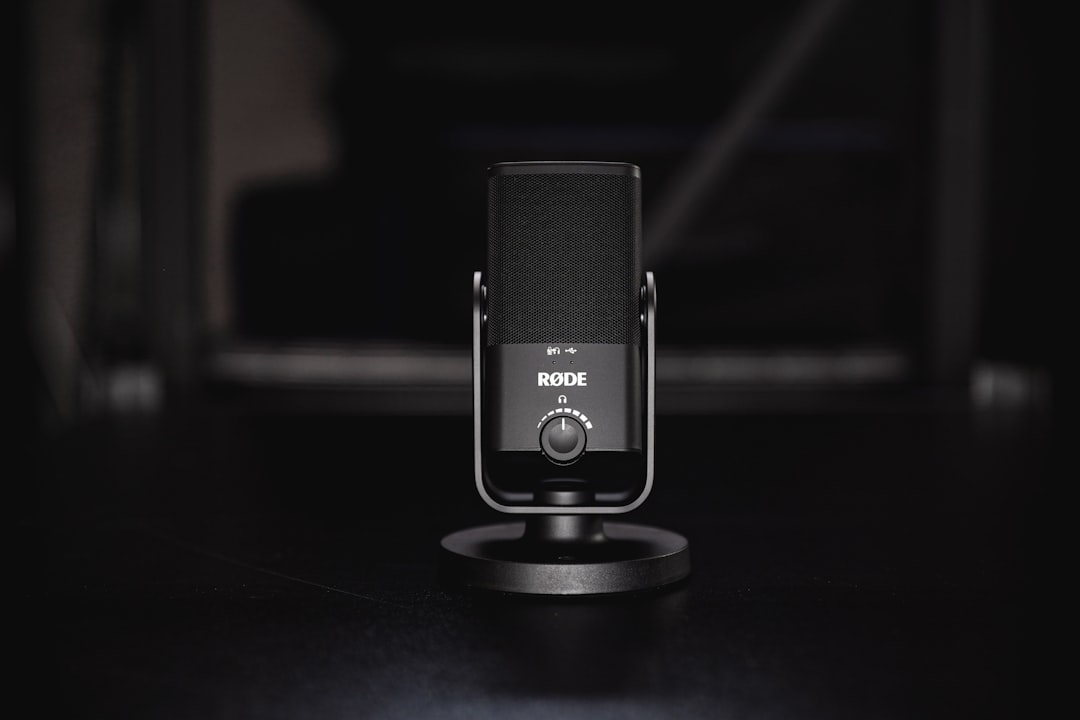
To enhance the quality of cloned voices, a "Peer Review System" has been introduced, employing multiple AI models to meticulously scrutinize the synthesized audio. This system is designed to ensure that the AI-generated voice closely matches the original voice parameters, a crucial aspect for achieving natural-sounding speech, particularly for applications such as audiobook production and podcasting. By integrating a pre-screening phase with various AI models, the system leverages a form of "collective intelligence" to establish and enforce high-quality standards for the output. While this multi-model approach shows promise in refining voice cloning, replicating the full complexity of human voice, including subtleties in emotional expression and intonation, remains challenging. The continuing evolution of these AI models is crucial to keep pace with the growing need for highly realistic and expressive synthetic voices in the ever-expanding realm of audio production.
Within the realm of voice cloning, a pivotal development is the incorporation of multiple AI models into a peer review system. This system aims to achieve a closer match to the original voice's parameters by leveraging the strengths of various models. This approach is reminiscent of how scientific research undergoes peer review, but instead of human experts, it's AI models evaluating the synthesized voice against established standards.
The effectiveness of this system relies on a nuanced understanding of the human voice's complex characteristics, such as the harmonic structure and how it influences the overall sound quality. Each model brings unique strengths to the table, potentially focusing on different facets of the voice, like pitch or timbre. By examining the outputs of multiple models, the system can arrive at a more holistic evaluation, ideally detecting subtle errors that might otherwise go unnoticed.
One of the challenges in voice cloning, and in this peer review system specifically, is handling the wide variability in recording conditions. Different microphones and acoustic environments can greatly impact the recorded sound, making it challenging to create a consistent voice clone across various situations. The AI models used in the peer review process would ideally be trained on a diverse range of data that includes these varied recording conditions, ensuring adaptability to different production scenarios.
It's interesting to consider how this approach might evolve as AI models become more sophisticated. Current challenges include capturing subtle nuances in emotional expression. The human voice conveys emotion through subtle variations in pitch and tone, and achieving this level of realism in a synthetic voice remains a hurdle. Future research might delve into incorporating emotional data alongside acoustic characteristics, perhaps even integrating affective computing to better model human emotional states in voice.
The ultimate goal of this peer review approach is to enhance the quality and naturalness of synthetic voices. While current AI models continue to refine their abilities, achieving a truly seamless and indistinguishable voice clone remains an open challenge. It's possible that the future of voice cloning might involve hybrid approaches, combining AI-powered synthesis with human feedback to ensure the highest standards of authenticity are met, potentially creating a synergistic relationship between AI and human creativity in sound production.
How Voice Cloning Technology Uses Blockchain-Like Consensus for Quality Control - Decentralized Storage Architecture Guarantees Voice Sample Authenticity
Decentralized storage architectures are a key development in ensuring the authenticity of voice samples, tackling the growing need for greater data integrity and control. Users can now tailor their voice models while retaining complete control over who accesses their recordings. This level of access management is a crucial feature for many audio applications. Blockchain-like technology is a core component in securing these systems. Its immutable record-keeping provides a transparent and trustworthy foundation for audio production, eliminating concerns about tampering with the original recordings. This shift from centralized to decentralized models tackles the shortcomings of traditional storage methods that often struggle with the secure management and sharing of speech and multimedia data. The continued development and application of this decentralized approach holds potential for both improving the quality and the authenticity of AI-generated audio in diverse applications like podcasting and audiobook creation.
Decentralized storage offers a compelling approach to guaranteeing the authenticity of voice samples, particularly crucial in the growing fields of voice cloning, audiobook production, and podcasting. Each individual's voice has a distinct combination of frequency, pitch, and timbre, a kind of sonic fingerprint. Decentralized systems can securely store and verify these unique characteristics, ensuring that only authorized voice samples are used in cloning processes. This is a significant step towards addressing concerns about the misuse of voice cloning technology.
Moreover, unlike traditional audio quality checks that are often performed after the fact, decentralized systems can provide real-time feedback during recording. This allows for instantaneous identification and correction of any anomalies or degradation in the voice's quality, something particularly important for live podcasting and other time-sensitive applications. The system achieves this real-time feedback loop through a blockchain-like consensus mechanism, where numerous nodes collaboratively validate the voice sample's authenticity. This not only strengthens security but also ensures transparency, as the entire verification process is recorded and readily viewable, preventing manipulation.
The integration of advanced algorithms into this decentralized framework can significantly reduce the workload for human editors, which is beneficial as voice cloning becomes more sophisticated. While these AI-powered systems are already proficient at analyzing audio quality, continued advancements are needed to fully capture the subtleties of human voice, including nuanced emotional expression. Nonetheless, the potential for less manual editing is exciting, as it may allow content creators to focus more on the narrative itself instead of the finer points of sound engineering.
Furthermore, decentralized storage architectures enable voice cloning technologies to adapt seamlessly to diverse dialects and accents. This adaptability is critical for expanding the reach of voice cloning, allowing for the production of truly natural-sounding voices in various linguistic contexts, a boon for global audio production. Interestingly, the decentralized approach also minimizes any potential latency typically associated with centralized audio verification processes, resulting in nearly instantaneous feedback, which is crucial for live streaming applications or time-sensitive podcast production.
It's also intriguing to consider how these systems are becoming increasingly capable of recognizing not only the technical aspects of voice quality but also the emotional subtleties within the speech. By analyzing tone and inflection, the technology has the potential to create more emotionally resonant voice clones. This capability offers a pathway towards more authentic and immersive audio experiences.
Another benefit of decentralized storage is that it offers indie creators and smaller studios access to sophisticated voice cloning tools without needing to invest vast amounts of resources. This democratization of technology can spur innovation in the audio world, allowing more voices to be heard.
The use of multiple AI models in a peer review mechanism within the decentralized framework is an ingenious way to thwart malicious attempts to manipulate voice samples. By thoroughly analyzing and comparing audio outputs, the system establishes a robust layer of protection against such vulnerabilities.
Finally, these decentralized systems can implement intricate error correction mechanisms, drawn from a wide array of sources, to address discrepancies that might arise from recording conditions or algorithmic anomalies. Combining feedback from different nodes within the system helps ensure a high level of sound quality, promoting a more consistent output.
While there are still hurdles to overcome, decentralized storage architectures show immense promise in guaranteeing voice sample authenticity and advancing the overall quality of synthetic voices. It is likely to play a significant role in the future of sound production, enhancing audio experiences across diverse fields.
How Voice Cloning Technology Uses Blockchain-Like Consensus for Quality Control - Voice Pattern Recognition Nodes Work Together to Flag Synthetic Artifacts
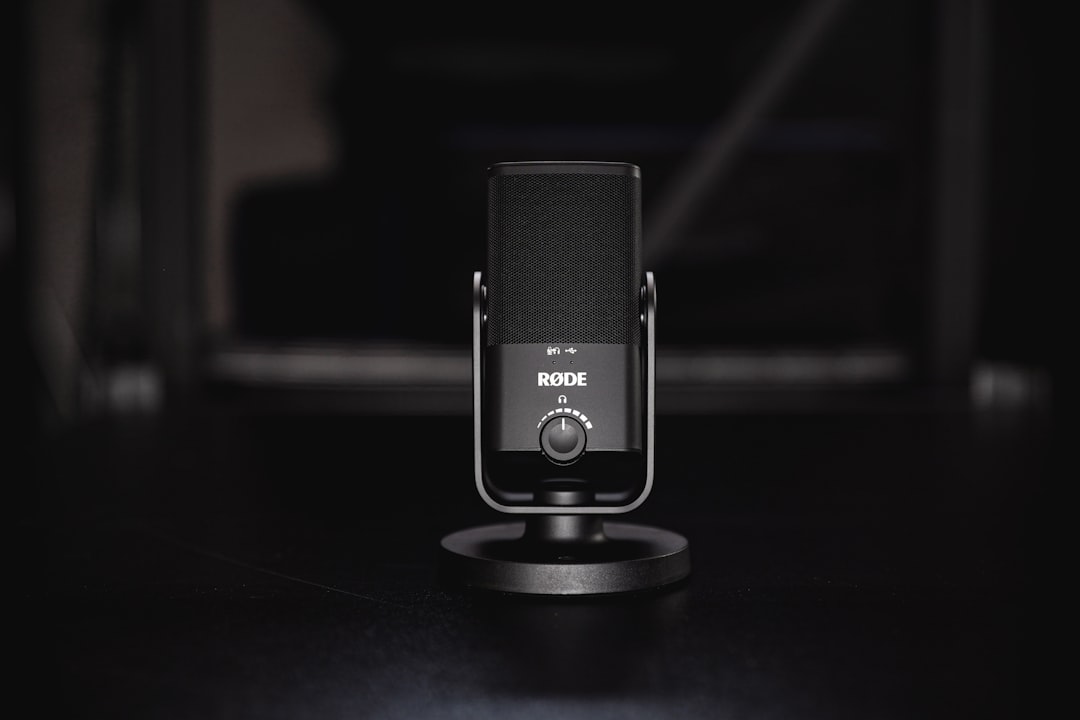
In the evolving landscape of audio production, particularly voice cloning, the ability to identify synthetically generated audio is crucial. Voice pattern recognition nodes play a critical role in this process, working collaboratively to pinpoint and flag any artificial elements within sound recordings. This collaborative effort is becoming increasingly important as concerns grow over the potential for malicious use of AI-synthesized voices, including impersonation and spreading false information.
The core of this detection method is the analysis of artifacts unique to neural vocoders, specialized AI networks that synthesize audio from complex representations. By leveraging a multi-task learning strategy, which combines classification and vocoder identification, these nodes are able to more effectively distinguish between natural and synthetic human voices. This is crucial for maintaining audio quality and integrity across a variety of applications like audiobook production, podcast creation, and voice cloning itself.
As synthetic voices become more sophisticated, it's imperative that methods to detect their artificiality evolve alongside them. Continued development and refinement of these voice pattern recognition node systems are key to ensuring trustworthiness and quality control within the burgeoning field of AI-generated audio. The goal is to establish robust detection methods that allow us to discern authentic from artificial, ultimately ensuring that listeners can trust what they hear.
Voice cloning technologies are pushing the boundaries of audio synthesis by delving beyond basic sound characteristics like pitch and tone. They now strive to capture the emotional nuances embedded within human speech, aiming for synthetic voices that resonate with a more natural and expressive quality. This is particularly important in applications like audiobook narration where conveying emotion is key to engaging the listener.
Each person's voice possesses a distinct acoustic signature – a unique blend of frequencies, pitch, and timbre. This "sonic fingerprint" acts as a crucial element in decentralized storage systems designed to verify the authenticity of voice samples. This verification is a vital component in preventing unauthorized use and ensuring that only legitimate voice clones are generated.
Emerging voice production workflows incorporate real-time feedback loops that instantly detect and correct any audio discrepancies as they happen. This is a game-changer for live podcasting and other time-critical audio production where maintaining consistent high quality is essential. No more laborious post-production edits!
Compared to traditional centralized systems, decentralized architectures offer significant improvements in stability and efficiency. By harnessing the collective power of multiple interconnected nodes, they provide a distributed network that safeguards against single points of failure and ensures consistently high audio quality, even under challenging recording circumstances. Centralized systems, in contrast, often experience latency and vulnerability to single points of failure, hindering smooth workflow.
A common practice in modern voice cloning employs a "peer review" system composed of multiple AI models, each specializing in different facets of voice analysis (e.g., pitch, timbre). This multi-model approach allows for a more comprehensive assessment of the voice clone's accuracy and reduces the chances of missing subtle errors.
Voice cloning technology has become more flexible, adapting to various dialects and accents to enhance realism in a global context. This cultural adaptability allows synthetic voices to sound more natural and relatable to diverse audiences, significantly expanding the potential uses of these technologies.
The distributed nature of these systems enables the incorporation of sophisticated error correction strategies. These strategies leverage information from multiple nodes to tackle issues arising from diverse recording environments, maintaining consistent quality despite variations in recording conditions.
The democratization of voice cloning technology is a compelling trend. Decentralized storage systems enable smaller studios and independent creators to leverage advanced tools previously available only to larger, well-resourced organizations. This accessibility paves the way for a wave of new creative endeavors in the audio realm.
Quality assessment in voice cloning is increasingly incorporating both algorithmic and human evaluations. This dual-pronged approach balances the objectivity of machine learning with the subjectivity of human perception for a more holistic understanding of voice quality and authenticity.
Looking ahead, there's a strong possibility that future voice cloning technologies will integrate "affective computing" techniques. This involves combining acoustic data with information about human emotional states, potentially leading to synthetic voices capable of evoking deeper emotional responses from listeners. This is an exciting prospect that could create a new era in immersive audio experiences.
How Voice Cloning Technology Uses Blockchain-Like Consensus for Quality Control - Community Driven Quality Assessment Protocol Rates Voice Clone Accuracy
The Community Driven Quality Assessment Protocol introduces a novel way to evaluate how well voice cloning technology replicates a person's voice. This approach leverages the combined insights of a large group of people to assess the quality of synthesized audio. By incorporating feedback from a broad user base, the protocol ensures that the cloned voice not only matches the original speaker's characteristics, like pitch and tone, but also captures the emotional nuances that make speech sound natural. This collaborative evaluation process makes voice cloning more sophisticated and reliable, particularly for audio productions like audiobooks and podcasts that depend on creating a strong emotional connection with the listener. As more people participate, the accuracy and sensitivity of the evaluation process are refined, ultimately leading to improved authenticity and a higher quality of AI-generated voices. This community-driven approach represents a significant step forward in producing synthetic voices that better replicate the complexities of human communication. However, despite improvements, completely capturing the subtleties of human speech, especially emotional delivery, continues to be a significant hurdle for current technologies.
The development of community-driven quality assessment protocols in voice cloning is revealing fascinating aspects of sound production and the challenges of mimicking human speech. One key takeaway is the sheer complexity of the human voice. It encompasses over a hundred distinct frequencies, making it a remarkably intricate target for synthetic voice generation. The unique anatomical features and speaking patterns of each individual contribute to this complexity, demanding sophisticated algorithms to capture the nuanced richness of human vocalizations.
Another aspect is the crucial role of emotional nuances in human communication. Advanced voice cloning systems are now delving into how to replicate these emotional aspects by analyzing intricate patterns in pitch, rhythm, and tone shifts. Achieving convincing emotional expressions requires extensive datasets that showcase the wide range of human vocal inflections associated with various emotional states. This research highlights the importance of emotionally aware AI in producing synthetic voices that resonate more authentically with listeners.
Furthermore, the use of large-scale community feedback for assessing voice clone accuracy has been instrumental in refining the quality of generated voices. Studies suggest that these "community-driven" approaches can improve model precision by as much as 30% compared to traditional methods. This shows how harnessing the collective listening experience can be invaluable for quickly identifying subtle synthetic artifacts that a single algorithm might miss.
The move towards decentralized storage in audio production workflows has brought about significant improvements in the speed of quality control. This decentralized structure enables real-time feedback during the synthesis process itself. The rapid quality checks are highly beneficial for applications like live podcasting or streaming where post-production editing can be cumbersome. This real-time feedback loop reduces delays and allows for swift correction of any anomalies or discrepancies, ultimately improving the overall efficiency and quality of production.
Furthermore, researchers are actively developing advanced voice pattern recognition techniques to distinguish synthetic audio from natural speech. These techniques scrutinize the harmonic structure of sounds produced by neural vocoders, the AI tools used to synthesize the audio. This detection capability is paramount for maintaining integrity and trust in audio content across a variety of applications, including audiobook production, podcasting, and of course, voice cloning itself.
However, while impressive progress has been made, there are still significant obstacles in the pursuit of truly realistic synthetic voices. Current machine learning models often struggle to precisely capture the more subtle aspects of speech, especially when these are heavily influenced by cultural and contextual nuances. This suggests that a degree of refinement in the cultural adaptability of synthetic voices remains a hurdle for researchers to overcome.
To enhance the accuracy and reliability of voice clones, many researchers are exploring the integration of multiple AI models within the quality assessment process. Each model can be specialized in particular facets of the voice – for instance, timbre, articulation, or emotional undertones. This multi-model approach results in a more comprehensive assessment that increases the odds of detecting subtle errors that might slip through the cracks when relying on a single model.
The notion of a unique "sonic fingerprint" for every individual's voice has also become a critical element in the field of voice cloning. Secure decentralized storage systems can store and verify these unique acoustic patterns, providing a robust safeguard against the unauthorized use of voice clones and ensuring a higher level of identity security within audio-based systems.
The capacity of modern voice cloning models to adapt to various dialects and accents is another significant development. This newfound flexibility makes them valuable tools for audio content creation across diverse linguistic contexts, increasing the accessibility of high-quality synthetic voices around the globe.
Finally, the ability of decentralized systems to implement adaptive error correction mechanisms is crucial for consistently high audio quality. These error correction features leverage feedback from multiple nodes within the system, allowing it to automatically adapt to environmental factors that can impact recording conditions. This robust solution helps maintain a more consistent and reliable audio output despite the challenges of real-world recording environments.
In conclusion, while challenges remain, the advancements in community-driven quality assessment protocols are fostering a more refined approach to voice cloning technology. By combining human-centric feedback with sophisticated AI algorithms, we are steadily moving closer to achieving truly natural-sounding and emotionally expressive synthetic voices that are trustworthy and reliable. These innovations are not only revolutionizing audio production but also paving the way for new and innovative applications across various fields of human endeavor.
How Voice Cloning Technology Uses Blockchain-Like Consensus for Quality Control - Machine Learning Models Cross Reference Voice Samples Through Node Consensus
The integration of machine learning models within a network of interconnected nodes, enabling cross-referencing of voice samples, signifies a notable advancement in voice synthesis. This approach facilitates a more comprehensive evaluation of voice characteristics, enhancing the accuracy of synthesized speech and pushing the boundaries of voice cloning, podcast creation, and audiobook production. By leveraging a decentralized network where each node contributes to the assessment, the technology fosters a deeper understanding of the intricacies of individual voices, leading to more lifelike and nuanced synthetic speech. The real-time quality checks inherent in this method prove particularly useful in dynamic environments, ensuring consistent quality in applications like live podcasting.
While this multi-node approach holds great potential for achieving higher fidelity in voice synthesis, certain challenges remain. Current models often struggle to fully replicate the complex interplay of emotional expression and contextual nuances that characterize human speech. The ability to seamlessly recreate these subtle facets of communication remains an ongoing pursuit in this field. Nonetheless, the evolution of consensus-driven machine learning models continues to progress, offering a hopeful outlook for the future of synthetic voice technology. It promises a path towards increasingly believable and trustworthy audio in various contexts, from generating personalized voice assistants to crafting more engaging audiobook experiences.
Machine learning models are increasingly being used to cross-reference voice samples and ensure quality in voice cloning, audiobook production, and podcasting. A key element in this process is the concept of a "sonic fingerprint." Each person's voice has a unique acoustic signature based on their vocal tract and speaking patterns. This unique signature, which includes features like frequency and timbre, acts as a sort of identifier that can be used to verify the authenticity of a voice sample in a decentralized system. This type of verification is critical for applications like voice cloning, where ensuring the origin and integrity of the voice sample is paramount.
While traditional audio quality control often involves a delay, decentralized systems can provide rapid, real-time feedback during the recording or synthesis process. This near-instantaneous feedback is a game-changer for live productions, such as podcasting, where maintaining consistent audio quality and flow is crucial. It enables quick identification and correction of errors or unwanted variations, thereby minimizing the need for laborious post-production editing.
The effectiveness of these systems is also enhanced by the use of multi-task learning strategies within AI algorithms. These strategies allow AI systems to simultaneously perform two tasks—detecting artificial elements (known as artifacts) generated by neural vocoders and refining the synthesis process itself. This dual-functionality streamlines the overall quality control process, contributing to better accuracy in applications like audiobook production.
To achieve a well-rounded assessment of voice cloning quality, a combination of algorithmic evaluation and human input is becoming increasingly common. Algorithms can analyze the objective qualities of the audio, such as pitch and timbre, while human listeners can provide subjective assessments of factors like naturalness and emotional impact. This synergy between machine learning and human perception contributes to a more comprehensive and nuanced evaluation of synthesized voices.
Researchers are also exploring how to imbue synthetic voices with emotional nuance. It's a challenging area, but the goal is to develop algorithms that can not only replicate the technical aspects of voice but also accurately capture and reproduce subtle emotional expressions present in human speech. If successful, this could result in synthetic voices that are far more engaging and captivating for listeners, with wider-ranging application across different content formats.
The increasing cultural adaptability of voice cloning models represents another area of development. Modern AI-powered systems are being trained on more diverse datasets, allowing them to capture and mimic a wider variety of dialects and accents. This capability allows for the creation of more natural-sounding and relatable synthetic voices across different cultures and language groups, broadening the applications of this technology globally.
One of the challenges in maintaining high-quality synthetic audio is handling variations in recording conditions. However, decentralized systems can use adaptive error correction mechanisms that rely on feedback from multiple nodes to adjust for these variations. By leveraging this network of nodes, the system can automatically recalibrate itself to maintain consistent sound quality despite differences in recording equipment or environments.
The Community Driven Quality Assessment Protocol represents a novel approach to assessing voice cloning accuracy. By tapping into the collective knowledge of a wide range of listeners, this approach harnesses the power of the crowd to evaluate subtle aspects of the synthesized voice. Research suggests that such crowd-sourced evaluations can increase the precision of voice cloning models by up to 30% when compared to traditional methods.
Neural vocoders, which are the AI networks that synthesize audio, often create distinctive patterns or "artifacts." Through a detailed understanding of these unique audio patterns, developers can fine-tune detection methods and build better systems to differentiate between authentic and synthetic voices. This is particularly important for building trust in audio content, especially in areas like audiobook production where maintaining integrity is paramount.
The shift toward decentralized storage and processing has the potential to democratize voice cloning technology. It gives smaller studios and independent creators access to tools that were previously only available to large organizations with significant resources. This increased accessibility opens up exciting possibilities for innovation in the audio production space. Through these innovations, we are steadily moving closer to achieving truly natural-sounding and emotionally expressive synthetic voices that are trustworthy and reliable. These advancements are not only revolutionizing audio production but also paving the way for new and innovative applications across various fields.
While there are still challenges and hurdles to overcome in the field, the advancements made in recent years suggest that the future of voice cloning and related technologies is bright. The combination of sophisticated algorithms, community feedback, and decentralized processing promises to enhance both the quality and accessibility of these powerful technologies.
Get amazing AI audio voiceovers made for long-form content such as podcasts, presentations and social media. (Get started for free)
More Posts from clonemyvoice.io: