Get amazing AI audio voiceovers made for long-form content such as podcasts, presentations and social media. (Get started for free)
Voice Cloning Accuracy New Study Reveals 7 Key Factors Influencing Synthetic Speech Quality
Voice Cloning Accuracy New Study Reveals 7 Key Factors Influencing Synthetic Speech Quality - Acoustic Model Refinement Boosts Naturalness
Refining the acoustic model is a key step towards creating more natural-sounding cloned voices. Improving the accuracy of voice cloning hinges on effectively integrating specific acoustic information into the cloning process. This involves employing advanced neural network designs that can better capture the subtle nuances of human speech. Researchers are actively working to overcome existing limitations in voice cloning frameworks, by developing new algorithms and techniques to reduce unwanted noise and artifacts in the synthesized audio. These efforts are paving the way for a new level of audio quality, particularly relevant for applications like producing audiobooks and podcasts where naturalness is crucial. However, achieving optimal results depends significantly on the quality of the training data. Choosing the right voice samples, especially when working with lower quality recordings, remains a vital aspect of producing realistic synthetic speech. The path forward for voice cloning suggests that we can anticipate synthetic speech that is increasingly indistinguishable from human speech, thereby transforming the way we interact with audio content.
Refining the acoustic models used in voice cloning has become a focal point for improving the naturalness of synthesized speech. These models, essentially the core of how the voice is constructed, are being meticulously tweaked to capture the subtle details of human speech – the way we vary our pitch, the rhythm and flow of our sentences, and the overall intonation that gives our voices personality. Researchers have shown that using advanced neural networks in this refinement process significantly boosts the similarity between the synthetic and original voice, to a point where the differences are increasingly difficult for listeners to discern.
This quest for naturalness is driving efforts to go beyond just creating a recognizable voice. The aim is to infuse synthetic voices with elements that make them sound more spontaneous, like the natural fluctuations in pace and pitch that we observe in everyday conversations. This focus on mimicking human speech variability enhances the listener's engagement and can greatly enhance the experience of applications like audio books or podcasts.
Another interesting angle is the growing ability to incorporate emotional nuance into synthetic voices. By fine-tuning the acoustic model, we can attempt to get the voice to convey the intended emotions of the text it is generating. This is important for a broader range of voice cloning applications.
While many tools are developed to improve speech quality, we can't ignore the role of the datasets used to train these systems. It's becoming apparent that leveraging more diverse and high-quality voice data allows these models to capture a wider range of accents, dialects, and speaking styles. Furthermore, transfer learning techniques, where the model learns from a limited dataset and generalizes it to produce high-fidelity cloned voices, offer promising directions in this domain.
It's worth noting that the acoustic environment plays a vital role in shaping the overall perceived quality of a synthetic voice. Things like the quality of microphones and the ambient sound characteristics of a recording environment can either elevate the realism of the generated voice or negatively impact it. As we refine these processes, the challenge remains to not just make cloned voices sound more natural, but also to make sure that the resulting sounds are not jarring or distracting in the contexts in which they are used.
Voice Cloning Accuracy New Study Reveals 7 Key Factors Influencing Synthetic Speech Quality - Prosody Transfer Techniques Enhance Expressiveness
The ability to transfer prosody—the rhythm, stress, and intonation of speech—is becoming increasingly important in voice cloning technology. This focus on prosody is crucial for creating synthetic voices that sound more natural and expressive. Researchers are exploring new methods, such as diffusion-based models like DiffProsody, which aim to improve the quality of the underlying prosody representation. By manipulating these representations, we can generate speech with a broader range of emotional and tonal variations, making the cloned voices sound less robotic and more engaging.
This enhancement of expressiveness holds particular promise for applications like audiobooks and podcast production, where conveying emotion and natural speech patterns is vital for audience connection. While considerable progress has been made, challenges remain in fully capturing the complex nuances of human prosody, which can vary significantly depending on context and individual speaker. The ability to synthesize speech that truly replicates the natural flow of conversation is still an area that requires further research. Despite these hurdles, the field is advancing at a rapid pace, suggesting a future where synthetic voices can be indistinguishable from human voices, particularly in terms of emotional expressiveness.
A recent study highlighted several factors crucial for achieving high-quality synthetic speech, emphasizing the importance of effectively transferring prosody – the rhythm, pitch, and intonation of speech – to enhance expressiveness and accuracy in voice cloning. Researchers evaluated synthetic speech quality using benchmark datasets, relying on mean opinion scores (MOS) collected from listener surveys to gauge the perceived quality.
One intriguing approach is DiffProsody, a diffusion-based model designed to refine the latent representations of prosody. The goal here is to improve the quality of the underlying ‘prosody vectors’, which, in turn, enhance the expressiveness of the generated speech. This is promising for improving emotional depth in areas like audiobook or podcast production, though the extent to which it achieves this remains to be seen in larger scale studies.
Another advancement, DaftExprt, focuses on developing multi-speaker acoustic models with enhanced cross-speaker prosody transfer capabilities. This is crucial for applications where a consistent speaker style is desired across different voices, such as potentially a series of audiobooks narrated by different cloned voices.
The current landscape of prosody transfer within speech synthesis is in a period of rapid evolution, with efforts concentrated on adapting pre-trained text-to-speech models for better performance. While cross-speaker style transfer techniques hold great promise for multi-style, expressive speech synthesis, current implementations still fall short of what is needed for real-world applications in terms of performance.
One fascinating aspect of these advancements is the capability to generate synthetic speech that deviates from the original utterance, simply by manipulating reference prosody embeddings. This flexibility allows for creating speech with various prosodic features. The challenge, however, lies in maintaining the integrity of the cloned voice while achieving desired variations.
To quantify the success of prosody transfer, researchers have been developing a range of metrics, employing both subjective and objective assessments. They've used models like Tacotron, both in single-speaker and multi-speaker setups, to analyze and improve the process. However, one inherent challenge in this domain is what researchers refer to as the 'one-to-many problem'. This problem arises from the fact that a single piece of text can have multiple corresponding prosody variations. This makes effectively transferring styles and nuances to synthetic voices quite complex.
Despite the hurdles, progress in end-to-end prosody transfer shows the potential for more realistic and emotionally rich speech synthesis. Ongoing research focuses on improving these techniques to create natural-sounding speech across a wider range of contexts. While the quest for perfection remains ongoing, the ongoing research into these techniques undoubtedly holds great promise for applications ranging from audiobook production to personalized voice assistants. However, one area that might benefit from further scrutiny is the extent to which the synthesized speech accurately mimics the emotional nuances desired, as this can vary considerably across listeners.
Voice Cloning Accuracy New Study Reveals 7 Key Factors Influencing Synthetic Speech Quality - Neural Vocoders Improve Audio Fidelity
Neural vocoders are revolutionizing audio quality, especially in the realm of voice cloning. These newer methods, exemplified by models like Vocos and HiFiNet, offer improvements in sound production through more efficient calculations and better waveform creation. This shift away from older source-filter vocoders allows for a more detailed representation of audio, which is especially beneficial for applications like audiobooks and podcasts that demand clear and expressive sound. The integration of noise reduction methods and Generative Adversarial Networks (GANs) has further elevated the fidelity of synthetic speech. This creates a strong foundation for more realistic and engaging audio experiences. The future of neural vocoders appears bright, with continued research likely to push the boundaries of synthetic speech to a point where it's nearly indistinguishable from human voices, transforming how we experience and interact with audio content. While there are still limitations, particularly in truly capturing the subtleties of human emotion, this technology is rapidly changing how we approach sound design.
Neural vocoders have revolutionized audio fidelity in areas like voice cloning, audiobook production, and podcast creation. These systems, often based on deep learning architectures like WaveNet or WaveRNN, have progressed significantly from earlier vocoder models that relied on simpler methods for synthesizing sound. Neural vocoders can now generate incredibly detailed raw audio waveforms, capturing the subtle nuances that make human speech sound so natural. This enhanced detail translates to a significant reduction in the artificial artifacts that plagued earlier synthetic speech.
One fascinating aspect of their development is the ability to minimize unwanted noise and distortions, leading to a significantly smoother and more pleasing listening experience for audiences of audiobooks or podcasts. While the quality of the output is heavily reliant on the dataset used for training, the ability of neural vocoders to operate in real-time opens up possibilities for interactive voice applications, such as virtual assistants, that require rapid response times.
Furthermore, researchers are constantly pushing the boundaries of what these systems can achieve. They are exploring ways to incorporate emotional expression into the synthesized audio, effectively allowing a cloned voice to express happiness, sadness, or any other emotion. This opens the door to more engaging and captivating narratives in audiobook productions or podcasts. In addition, the pursuit of unsupervised learning methods is promising, as it potentially allows vocoders to adapt to new voices more easily, without requiring the extensive labeled datasets that currently limit their capabilities.
Moreover, neural vocoders have gained the ability to manage multi-speaker environments, a critical feature when crafting audiobooks with multiple characters. This involves smoothly transitioning between different cloned voices seamlessly, which contributes to a more immersive and believable storytelling experience. We're also seeing progress in high-sample-rate audio generation, allowing for more detailed audio outputs that are comparable in quality to studio recordings, making them ideally suited for professional audio production workflows.
The potential for cross-lingual voice synthesis is also starting to be explored. Researchers are investigating the transfer of prosodic features across languages, aiming to eventually create systems that can accurately clone voices in different languages. The ability to effortlessly generate synthetic audio in diverse languages could have a profound impact on the global accessibility of educational materials, entertainment, and communication platforms. While challenges remain, the evolution of neural vocoders suggests that we're moving towards a future where synthetic speech becomes increasingly indistinguishable from human speech. This raises interesting questions about the future of audio content creation and the ways we interact with the world through sound.
Voice Cloning Accuracy New Study Reveals 7 Key Factors Influencing Synthetic Speech Quality - Data Augmentation Strategies Expand Voice Variety
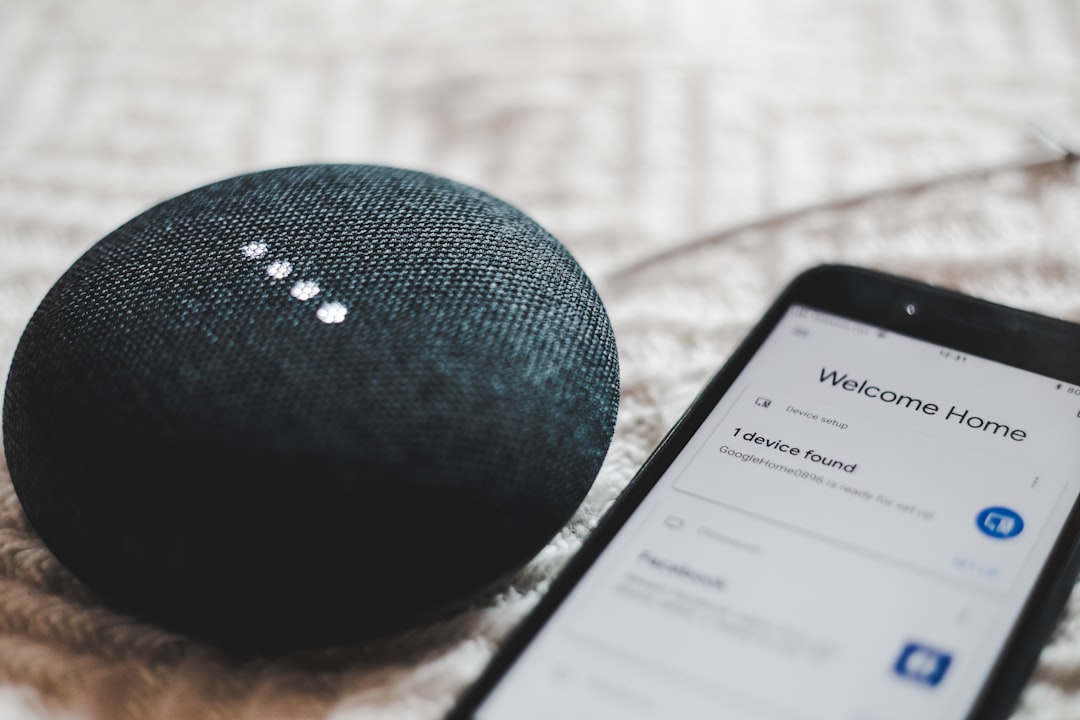
Expanding the range of voices produced through cloning relies heavily on data augmentation strategies. Techniques like CutMix, Mixup, and dynamic mixing are proving to be effective in broadening the adaptability of voice cloning models. This expanded versatility is crucial, as it helps synthetic voices capture a greater diversity of human speech patterns. For applications where naturalness is key, like audiobooks or podcasts, having a broader range of voice characteristics is essential to maintain audience engagement. Furthermore, these strategies help in developing voice conversion technologies that can achieve good results without needing a large dataset of the target voice. The effectiveness of these techniques is notable, suggesting that future advancements can bring synthetic voices even closer to the subtleties of human expression.
Data augmentation has emerged as a powerful tool to enhance the variety and quality of synthetic voices generated through voice cloning. It offers a compelling way to expand the scope of training data without needing to record vast amounts of new audio. By applying techniques like pitch shifting or adding noise, we can essentially create diverse variations of existing voice samples. This can lead to cloned voices that sound more natural and adaptable.
One fascinating aspect is the potential for data augmentation to create more realistic accents and dialects. By simulating diverse speech patterns found in different linguistic communities, we can train voice cloning models to generate audio that reflects these variations. This can help bridge gaps in communication by making synthetic speech more relatable and accessible for specific audiences.
Another interesting application of augmentation is the possibility of introducing emotional nuances into cloned voices. Manipulating speech delivery through prosodic changes, like alterations in tone or rhythm, enables us to make cloned voices sound happier, sadder, or express a wider array of emotions. This can dramatically improve the engagement of audiences consuming content like audiobooks or podcasts, allowing the synthetic voice to more convincingly portray emotions.
Furthermore, data augmentation has been explored for cross-language adaptation in voice cloning. By carefully manipulating phonetic representations, models can be trained to adapt voices to various languages. This could drastically expand the reach of voice cloning technologies by making it easier to create and translate audio content for global audiences.
Adding a layer of realism through augmentation also includes the ability to mimic human-like speech habits. By injecting typical elements like pauses, fillers like "um" or "uh", into the training data, voice cloning systems can generate speech that sounds less robotic and more spontaneous. This fosters a sense of natural interaction in conversational settings like chatbots and virtual assistants.
In educational settings, the availability of a broader range of synthetic voices opens interesting possibilities. Audiobooks and educational content could be presented in varied styles or intonations by manipulating the augmented data. This might potentially lead to improved memory and comprehension for listeners as they encounter the same information expressed in different ways.
However, the success of data augmentation heavily relies on the quality of the initial voice recordings. High-quality source material augmented with carefully chosen techniques tends to produce the most natural-sounding synthetic speech. This emphasizes the vital role of data selection in ensuring that the final cloned voice is not merely varied but also high-fidelity.
Advanced augmentation techniques employing variational autoencoders (VAEs) allow researchers to generate extensive collections of synthetic voice samples that still retain the core characteristics of the speaker. This approach permits a level of fine-grained control over variations within a cloned voice.
While augmentation significantly increases the training dataset, a careful balance between diversity and quality must be maintained. Overloading a model with low-quality data can lead to overfitting and unwanted artifacts in the synthetic speech. The challenge lies in finding an optimal balance to enhance a voice clone’s expressiveness and ensure its overall quality.
In conclusion, the use of data augmentation offers a promising avenue for advancing the field of voice cloning. It offers tools to significantly enhance the diversity and expressiveness of synthetic voices. As research progresses, we can expect to see further refinement of these techniques, enabling more natural and compelling synthetic voices across a broader range of applications.
Voice Cloning Accuracy New Study Reveals 7 Key Factors Influencing Synthetic Speech Quality - Emotional Speech Synthesis Advances Realism
The field of emotional speech synthesis is experiencing notable progress, making synthetic voices sound more human-like and benefitting applications like audiobooks and podcasts. Deep learning techniques have replaced traditional methods, resulting in modern text-to-speech (TTS) systems that can better represent the intricate nature of human emotions. These advancements allow for synthetic speech that blends multiple emotional expressions rather than simply mimicking a single emotion. Researchers are also developing specialized databases to facilitate ongoing research into creating even more natural-sounding emotional voices. However, obstacles still exist in areas like converting waveforms with precision in real-time and ensuring the synthesized emotions truly resonate with listeners. The journey toward creating synthetic voices that can authentically express a wide range of emotions is ongoing, with the potential to reshape how we create and interact with audio content.
The evolution of emotional speech synthesis, driven by the transition from traditional machine learning to deep learning, has significantly enhanced the realism of text-to-speech (TTS) systems. We now see TTS models capable of producing speech that closely mimics human vocalizations, serving a growing range of applications demanding realistic audio generation, including audiobooks and voice cloning.
The research landscape in this field is shifting towards creating synthetic speech that captures a blend of emotions, rather than simply replicating a singular emotional state. This requires a more sophisticated approach to understanding how emotions are conveyed in speech. Researchers have proposed a new framework for comparing speech across various emotional types, facilitating a more nuanced understanding of the relationship between the spoken word and emotional content.
While we have real-time voice cloning systems that employ several algorithms for synthesizing speech, accurate waveform conversion continues to present difficulties. These challenges highlight the intricacies of transforming text and vocal characteristics into audible outputs. There's a fascinating interplay between emotional speech synthesis and speech emotion recognition (SER) systems. Integrating emotional speech synthesis into SER could potentially boost their effectiveness, although the exact impact on SER's accuracy remains to be fully explored.
To support this line of inquiry, an emotional speech database (ESD) has been created as a resource for ongoing research in emotional voice conversion and synthesis. The development of neural voice cloning systems has made it possible to replicate a person's voice using just a limited set of audio samples. These systems leverage techniques like speaker adaptation and encoding, showcasing the potential for efficiently creating customized synthetic voices.
Controlling emotional expressiveness in synthetic speech involves meticulously examining emotional speech data and crafting systems capable of both extracting the emotional essence from the data and synthesizing speech that reflects these emotional elements. The emergence of deep learning techniques has been crucial in refining TTS systems, particularly in enhancing the emotional expressiveness of synthetic speech output. This advancement is crucial for improving engagement and immersion in applications like podcasts and audio storytelling.
However, there's a growing awareness of the importance of cultural context in the perception of emotions. For example, the way a particular emotion is expressed and perceived can differ widely across cultures. It is crucial that the future of this technology considers cultural sensitivity in its development and application. Otherwise, the generated synthetic voices might appear unnatural or even insensitive. The potential biases in training datasets also warrant attention as these biases can inadvertently perpetuate stereotypes within the generated audio, potentially limiting the intended scope of this exciting field.
The ongoing work in emotional speech synthesis, while impressive, still faces challenges. Further refinements in the control and expressiveness of emotional nuance in synthetic voices promise to continue transforming the field of audio production, bringing us closer to achieving audio that is nearly indistinguishable from real human voices.
Voice Cloning Accuracy New Study Reveals 7 Key Factors Influencing Synthetic Speech Quality - Language-Agnostic Architectures Enable Multilingual Cloning
Voice cloning technology is experiencing a significant shift with the development of language-agnostic architectures. These new systems can create synthetic speech in multiple languages without needing separate training for each language. This makes creating multilingual audio content much easier, which is beneficial for audiobooks, podcasts, and various other applications where conveying information across languages is important. The use of shared embedding spaces, which capture the core characteristics of a voice, helps to maintain the desired stylistic and emotional nuances during language transfer. While this new approach is promising for expanding the accessibility of audio content to wider audiences, ensuring that the quality of synthetic speech remains high across languages is still a challenge. Achieving consistent, natural-sounding speech across various languages will be crucial for improving the overall experience of synthetic audio for listeners. There is much potential in this field to expand the ways people interact with audio. But challenges remain regarding quality across a variety of languages.
Language-agnostic architectures are a new frontier in voice cloning, allowing models to learn from audio samples across different languages. This means a single model can replicate a speaker's voice no matter the language they're speaking, opening up possibilities for globally accessible audiobooks and other voice-based applications. Interestingly, researchers are finding that many languages share underlying acoustic features that can be leveraged to make the process of voice cloning more efficient, needing fewer language-specific training data.
A key development in this field is cross-language prosody transfer, which enables cloned voices to adopt the emotional intonation and speech rhythms specific to different languages and cultures. This creates a more immersive experience for listeners across diverse linguistic contexts, especially when considering content like audiobooks or podcasts designed for international audiences. The capacity to embed emotional nuances transcends language barriers, allowing these clones to convey feelings more universally. This could have significant implications for producing audiobooks and podcasts in a variety of languages, where emotional resonance is crucial.
Researchers have discovered commonalities in acoustic properties across different languages. By focusing on these shared elements, voice cloning systems can be designed to learn faster and with potentially smaller datasets than previously thought. Similarly, recognizing universal phonetic traits permits the development of models capable of transferring learned voice characteristics seamlessly between languages. This flexibility enables voice clones to adapt to a variety of linguistic contexts while maintaining a consistent vocal style.
Language-agnostic frameworks bring advantages in terms of dataset efficiency. Instead of needing enormous, separate datasets for each language, these models can be trained on smaller, higher-quality, multi-lingual datasets. This efficiency is key for scalability and broader application in voice cloning systems. These models also seem to improve the removal of unwanted noise and audio artifacts in synthetic speech, enhancing the audio clarity across various languages and dialects. This is particularly vital for applications like audiobook productions where audio fidelity greatly influences the listener's experience.
Researchers anticipate future voice cloning systems incorporating real-time language switching, a feature that would allow a cloned voice to shift smoothly between different languages while maintaining vocal consistency. This has the potential to redefine how content is created and delivered for audiences across the globe. The prospect of creating personalized vocal experiences that resonate with diverse cultural preferences, while retaining a consistent brand or author voice identity, is also gaining traction within this field. The pursuit of refining these processes could yield transformative results in the broader field of voice-based content creation. However, much more research and refinement are required before such sophisticated systems become truly usable.
Voice Cloning Accuracy New Study Reveals 7 Key Factors Influencing Synthetic Speech Quality - Real-Time Adaptation Methods Personalize Output
Real-time adaptation techniques are crucial for tailoring the output of voice cloning systems to individual preferences and specific applications. These methods utilize sophisticated algorithms to dynamically adjust the characteristics of synthetic voices based on various cues, enhancing the audio's quality and naturalness. This adaptability proves beneficial for scenarios like audiobook production or podcast creation, where a personalized and engaging auditory experience is essential. Through this dynamic adjustment, synthesized voices can not only mimic natural speech but also effectively capture the nuances and emotional expression inherent in human communication. The ongoing evolution of this technology promises even greater levels of personalization, creating more realistic and compelling interactions with synthesized audio. The challenge for developers, however, is to maintain a balance between flexible adaptation and the preservation of high audio quality to ensure consistently enjoyable listening experiences.
Real-time adaptation methods are increasingly vital in enhancing the quality and naturalness of synthetic speech, particularly in voice cloning. These techniques allow systems to dynamically adjust elements of the synthesized voice, such as pitch, intonation, and pace, in response to various factors. For instance, in interactive voice assistants, a system can adjust its response based on the user's previous interactions, leading to a more natural conversation flow.
One key aspect is the ability to capture multi-faceted speech characteristics. Voice cloning isn't just about mimicking a person's voice, it's also about capturing finer details like breathing patterns, pauses, and even subtle voice irregularities. These intricate aspects greatly influence the authenticity of synthetic speech, leading to a more natural and immersive listening experience. This is particularly relevant for applications like audiobook production where engaging listeners is paramount.
Furthermore, the emerging understanding of how emotional cues influence physiological responses has spurred further development of adaptive systems. Studies have demonstrated that the emotional expression conveyed through a synthetic voice can impact listeners' heart rate and other physiological indicators. This suggests that synthetic voices might have the potential to influence listener states, like mood, through skillful expression of emotional content in a podcast or story.
Moreover, adaptive systems can integrate contextual data to further personalize the audio experience. For instance, if the context involves text with a specific emotional undertone, the voice can adjust its delivery accordingly. This situational awareness allows for a more tailored approach to audio generation, ultimately increasing listener engagement.
The seamless blending of diverse emotional qualities within a single piece of audio is another area of advancement. Through sophisticated algorithms, synthetic voices can transition effortlessly between varying emotional expressions, which is a significant step towards generating more realistic and complex narratives. This flexibility holds immense promise for applications like audiobook creation, where the portrayal of diverse emotions is integral to engaging listeners.
In addition, understanding the influence of the surrounding environment on voice quality is becoming increasingly important. Adaptive techniques take into account factors like microphone placement and ambient noise, optimizing the resulting audio's clarity and richness. For audio-intensive applications like podcasts, this level of acoustic awareness is essential for creating a high-quality output.
Furthermore, intonation patterns play a vital role in conveying meaning and emotional intent within speech. Adaptive systems are being developed to dynamically adjust these patterns, which can significantly impact how listeners perceive emotions like sarcasm, excitement, or sadness. This is a crucial area of ongoing research, particularly for applications where authentic emotional expression is desired.
Neural networks have revolutionized this field, allowing systems to synthesize speech that replicates the natural variations and spontaneity observed in human speech. This ability to create authentic-sounding, unpredictable utterances is enhancing the experience of conversational interfaces.
Some researchers are also experimenting with feedback loop mechanisms. This allows the system to learn from audience interaction and adapt its speaking style over time. This dynamic learning capability further personalizes the experience and enhances future user engagement.
Finally, it's critical to emphasize the importance of cultural sensitivity in voice adaptation. Effective real-time adaptation requires an understanding of global linguistic patterns and how they impact speech tonality, pacing, and emotional expression. By incorporating these nuances, we can aim for a broader appeal and create synthetic voices that resonate more effectively with diverse audiences. The continued development of culturally sensitive voice adaptation is crucial for the responsible and equitable use of synthetic speech technologies.
The journey toward more human-like and adaptable synthetic voices continues, driven by a desire to achieve an immersive and personalized audio experience. These ongoing advancements in real-time adaptation promise to transform the landscape of audio production and further enhance human interactions with the audio world.
Get amazing AI audio voiceovers made for long-form content such as podcasts, presentations and social media. (Get started for free)
More Posts from clonemyvoice.io: