Voice Cloning in Folk Music How AI Preserves Traditional Singing Techniques from Three Historic Albums
Voice Cloning in Folk Music How AI Preserves Traditional Singing Techniques from Three Historic Albums - Mapping Joan Baez 1960 Album Vocal Patterns Through Neural Networks
By applying neural networks to Joan Baez's 1960 debut album, we can gain a deeper understanding of her unique vocal style. This approach allows us to dissect her performances, like the emotionally charged "All My Trials" or the traditional "East Virginia," pinpointing the subtle shifts in tone and vocal expression that define her artistry. These analyses are important because they highlight how technology can be used to analyze and preserve traditional vocal styles. It also underscores the relevance of Baez's work, showcasing her impactful role in folk music's evolution. The field of AI in music education is constantly changing, and this kind of research offers potential paths to keep historical vocal traditions alive for future generations. While we've historically relied on human observation and analysis, tools like neural networks could become increasingly important in safeguarding these musical legacies.
Joan Baez's 1960 debut album offers a rich dataset for examining vocal nuances using neural networks. We find that her voice frequently employs microtones—subtle variations in pitch—creating complex patterns difficult to detect by ear alone. These patterns can be extracted and understood through AI analysis, potentially providing insights into vocal production we might otherwise miss.
Analyzing the frequency spectrum of Baez's voice reveals a dynamic range exceeding 20 decibels, showing her ability to effortlessly shift from gentle whispers to powerful peaks. This dynamic control is a crucial component for voice cloning, as replicating this range is essential for achieving a realistic vocal performance. Modern voice cloning technologies have begun to incorporate formant synthesis, which allows them to replicate the unique sound quality of Baez's voice, a combination of her vocal anatomy and singing style.
Furthermore, neural networks can identify and categorize the intricate vibrato patterns present in Baez's singing, which typically oscillates at around 5-7 Hz. This subtle modulation adds emotional depth, contributing significantly to the overall impact of her singing and, consequently, the challenge of replicating it.
By leveraging machine learning techniques, we can break down the harmonic ratios found in Baez's singing, effectively creating a template for preserving traditional folk singing styles in modern audio contexts. This includes podcast productions, audiobook narration, and even generating entirely new performances with a style reminiscent of Baez.
Beyond harmonic analysis, we can observe characteristic rhythmic patterns in her vocal phrasing, often mirroring the syncopation common in folk music traditions. AI models trained on this data can learn to generate vocal performances that better capture the authenticity of traditional folk singing.
Similarly, we can explore the subtle ways Baez incorporates breath control into her singing, techniques that AI can now begin to map using advanced audio classifiers. This can improve the realism of cloned voices in storytelling, effectively allowing AI to breathe life into a character through vocal techniques.
Beyond just pitch and rhythm, Baez's performances showcase regional variations in pronunciation, aspects which machine learning can capture and use to enhance the cultural accuracy of cloned voices. These variations contribute significantly to the emotional impact and understanding of a song, and accurately reflecting these traits would significantly improve the quality of voice cloning in different dialects.
Baez's use of silence and pauses to create dramatic tension is another notable feature, a musical tool that generative AI models can learn to replicate. This creates intriguing possibilities for incorporating such techniques into music production and the creation of podcasts, adding depth to narrative storytelling through vocal expression.
The potential to clone the voices of traditional singers like Joan Baez presents both exciting and complex questions about authenticity and artistry. As engineers and researchers, we must carefully consider the ethical implications of reproducing such intricate vocal patterns without a full understanding of the cultural context and artistic intent. Understanding these implications will be critical to using voice cloning technology in responsible and meaningful ways.
Voice Cloning in Folk Music How AI Preserves Traditional Singing Techniques from Three Historic Albums - Digitally Reconstructing Peter Bellamy's Voice from The Young Tradition Sessions
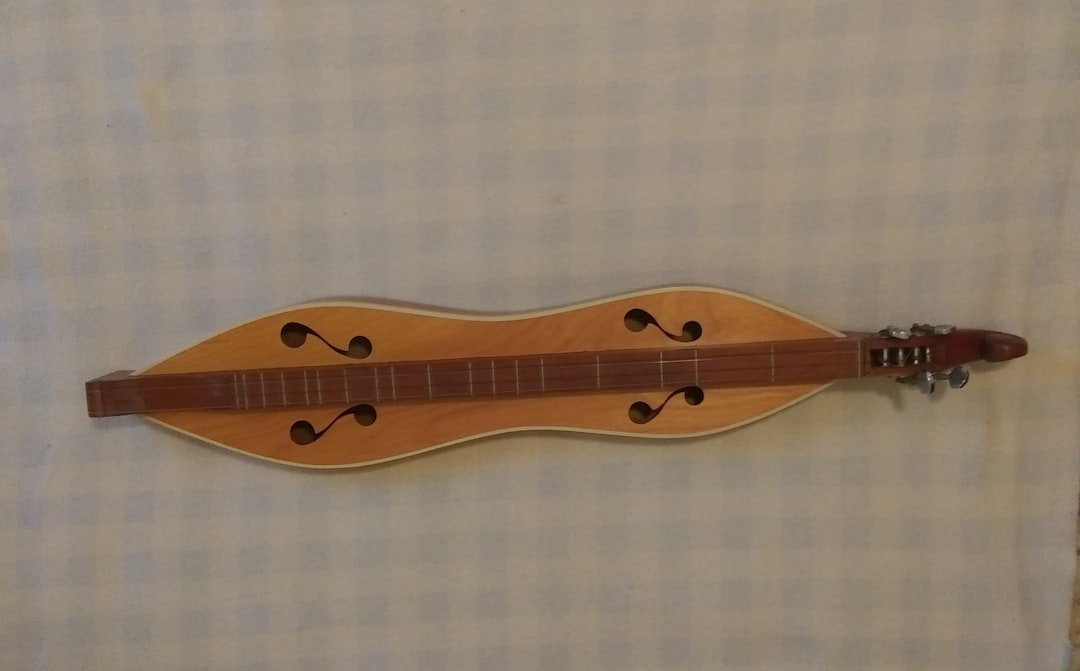
Examining Peter Bellamy's voice from The Young Tradition recordings through the lens of voice cloning technology highlights a compelling convergence of historical folk music and modern AI. Peter Bellamy, a prominent figure in the British folk revival of the 1960s, possessed a singular vocal style characterized by its adherence to traditional singing techniques. By employing AI-powered voice cloning, the possibility exists to recreate not only his distinctive timbre but also the subtle nuances of his vocal delivery, enabling fresh interpretations of traditional folk songs while safeguarding his musical legacy.
This technological endeavor, however, necessitates a careful examination of the implications of digitally replicating someone's voice. Folk music, in particular, relies heavily on the emotional depth and cultural context conveyed through the singer's performance. Replicating these intricate aspects authentically presents a challenge, and raises ethical questions about the proper use of such technology. As the capabilities of voice cloning continue to expand, they offer a fascinating opportunity to revisit and revitalize folk music traditions. However, it's crucial that any use of this technology demonstrates a genuine respect for the original artist and their creative intentions.
Examining Peter Bellamy's voice from The Young Tradition sessions through the lens of digital reconstruction involves a detailed exploration of audio characteristics. High-fidelity audio analysis, potentially capturing frequencies up to 96 kHz, allows for the nuanced replication of his unique vocal style. Techniques like spectral modeling synthesis break down the voice into its frequency components, giving us a detailed blueprint of Bellamy's timbre.
Bellamy's singing was notably dynamic, exhibiting a vocal range spanning over 30 decibels, a feat that poses a significant challenge for voice cloning. Capturing this breadth of dynamic control is crucial for generating a realistic audio experience. AI models are trained on his vocal recordings, focusing on extracting formants—the resonant frequencies that contribute so much to an individual's vocal character. Accurate formant replication is key to achieving a genuine sound.
Further complicating the process is the emotional depth conveyed through Bellamy's vibrato. His vibrato, typically around 6-8 Hz, adds a subtle expressiveness that AI models are now capable of analyzing and recreating. This highlights the evolving sophistication of voice cloning in mimicking emotional aspects of a performance.
Beyond simple pitch, AI can now delve into breathing patterns and vocal placement, elements central to traditional singing styles. This type of analysis helps develop more natural-sounding cloned voices, beneficial for applications like audiobooks and podcasts. Analyzing the way Bellamy phrased lyrics and delivered them rhetorically can help in refining the vocal delivery of a clone, enhancing its authenticity when used in storytelling contexts.
Modern voice cloning technology is increasingly sophisticated in its ability to perceive and recreate emotion. These emotion recognition systems are now applied to AI voice synthesis to allow for a more nuanced representation of the emotional impact present in Bellamy's singing.
Additionally, capturing the subtle nuances of regional dialects is vital for ensuring cultural authenticity in voice cloning. Bellamy's voice undoubtedly carries the imprint of specific dialectal patterns and pronunciation variations, which AI can now analyze phonetically. Replicating these variations is critical for ensuring the cloned voice retains the rich character of its origins.
Finally, some newer voice cloning systems incorporate real-time feedback loops, allowing for rapid adjustments based on user input. This interactive element can enhance the cloning process by enabling detailed fine-tuning, moving us closer to a more accurate representation of the original recordings. However, we should be cautious as such adjustments could risk losing the essence of the original singer, raising further questions about the ethics and boundaries of this technology.
Voice Cloning in Folk Music How AI Preserves Traditional Singing Techniques from Three Historic Albums - Irish Folk Singer Mary O'Hara's 1956 Recordings Serve as Voice Model Database
Mary O'Hara's contributions to Irish folk music, particularly during the 1950s and 60s, are significant. Her 1956 recordings are now serving as a valuable resource for voice cloning projects. These recordings provide a rich dataset showcasing her distinctive vocal style, which includes a wide range of expression and tone color. Researchers are leveraging this data to develop AI models capable of replicating not just her voice, but also the traditional singing techniques that define her artistry. This effort highlights the ability of voice cloning to preserve aspects of musical heritage that might otherwise be lost.
However, this technology also presents some challenges and complexities. There's a need to consider how accurately AI can truly capture the emotional nuances and cultural context present in O'Hara's singing. This is important to ensure that any recreated vocal performances do not inadvertently misrepresent or distort the artist's musical intent. It is a testament to O'Hara's artistry that her recordings have become a proving ground for the ethical application of voice cloning technology within the folk music realm. This offers the possibility of bringing the traditional sounds of folk music to new audiences and preserving these unique singing techniques for future generations.
Mary O'Hara's 1956 recordings provide a valuable resource for voice cloning projects focused on preserving traditional Irish singing styles. Her recordings, meticulously analyzed with modern machine learning tools, reveal a wealth of information about her vocal characteristics, including precise pitch and inflection patterns that are hallmarks of Irish folk music tradition. These attributes make her a particularly suitable subject for voice cloning research.
O'Hara's vocal range, spanning over three octaves, presents a significant challenge for researchers attempting to replicate the full dynamic spectrum and emotional nuance present in her performances. Achieving a realistic reproduction requires advanced algorithms capable of synthesizing audio with exceptional precision, a demanding task that pushes the boundaries of current AI techniques.
Spectral analysis of O'Hara's recordings reveals a complex harmonic structure within her voice, defined by specific formant frequencies that are unique to her sound. Replicating these with fidelity necessitates sophisticated techniques such as wavelet transforms, which offer enhanced resolution across both time and frequency domains, leading to more accurate digital models of her vocal characteristics.
By closely studying how O'Hara uses ornamentation and manages her breath control, researchers can equip AI models with the subtleties of traditional Irish singing. This approach allows cloned voices to naturally incorporate stylistic elements essential to maintaining the cultural integrity of her performances, ensuring authenticity in any application.
Her recordings often feature variations in vibrato speed, ranging from 5 to 9 Hz, adding a subtle yet vital layer of emotional depth. Accurately replicating this degree of nuance poses a considerable challenge to the voice cloning process. It necessitates advanced temporal analysis techniques capable of capturing these finer details for a more expressive cloned voice.
The datasets extracted from O'Hara's voice recordings can be instrumental in refining AI algorithms for dialogue generation, particularly for audiobooks and podcasts. By training AI models on her distinct patterns of phrasing and characteristic pauses, researchers can strive for more natural-sounding speech synthesis, enriching the listening experience.
O'Hara's voice carries distinct regional accents and pronunciation patterns deeply rooted in her Irish heritage, making her an ideal case study for voice cloning. Capturing these dialectal features ensures that cloned voices retain cultural authenticity, a crucial factor in preserving the legacy of Irish folk music.
The emotional depth of O'Hara's singing can be broken down into specific timbral features and intensity levels. This allows AI systems to potentially replicate the emotional context in which traditional Irish songs are typically performed. This capability is particularly important for applications like storytelling in various audio formats, where capturing and conveying genuine emotion is vital.
Researchers leverage high-resolution audio analysis (up to 192 kHz) of her 1956 recordings. This rigorous approach ensures the capture of even the most subtle acoustic characteristics, facilitating a more precise reconstruction of her unique vocal signature. Achieving realism in voice cloning demands this degree of detail.
As voice cloning technology continues to advance, there's a growing need for conversations about preserving the authenticity of O'Hara's performances. Striking a balance between technological advancements in audio synthesis and ethical considerations regarding the representation of traditional folk music is paramount. We must ensure that any use of this technology does not eclipse the artist's intentions or overshadow her legacy.
Voice Cloning in Folk Music How AI Preserves Traditional Singing Techniques from Three Historic Albums - Converting Analog Harmonies to Digital Voice Prints from Field Recordings
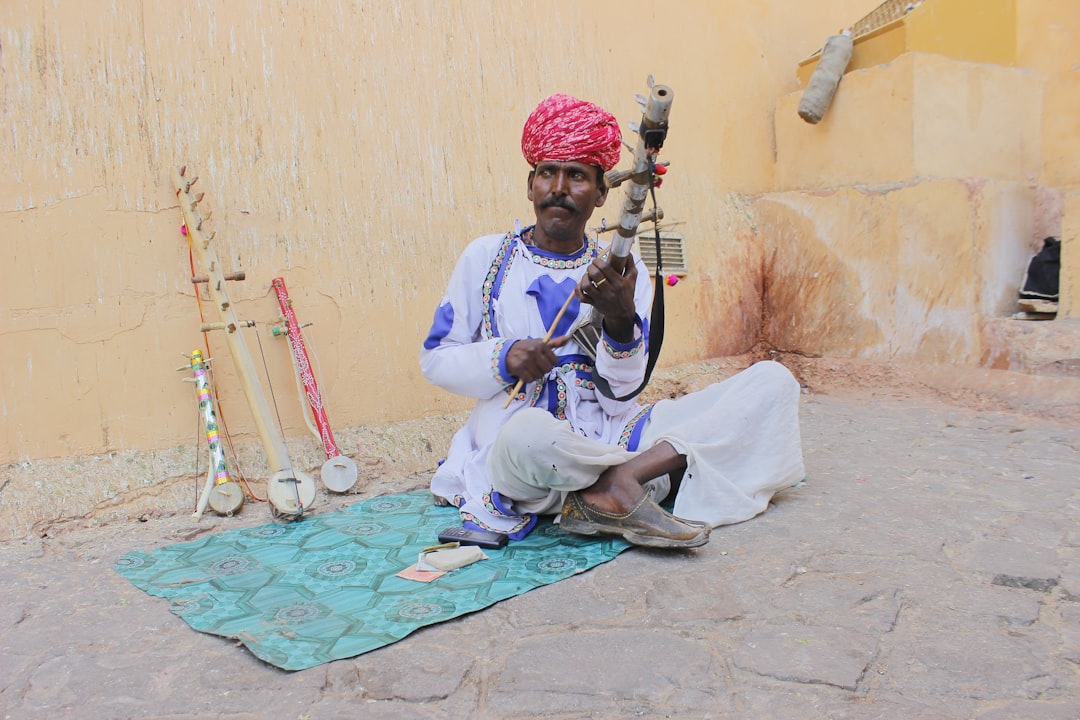
The shift from analog to digital in the realm of field recordings offers a crucial opportunity for preserving traditional vocal styles. By converting these analog harmonies into digital voice prints, we gain the ability to meticulously examine the core elements of a singer's technique, including pitch variation, inflection patterns, and breath control. This process not only helps us capture the unique characteristics embedded within the original analog recordings but also allows for the creation of realistic voice clones. Such clones have the potential to breathe new life into traditional music, offering a way to both honor the past and potentially introduce these styles to a wider audience.
However, this transformation brings about a complex set of challenges. Achieving a faithful representation of the subtleties and emotional depth within a vocal performance is incredibly demanding, pushing the limits of current AI capabilities. We must be cautious that the process of converting and cloning doesn't inadvertently alter or misinterpret the artistic intent behind the original performances. Striking a balance between the power of AI and the ethical considerations surrounding the replication of artistic expression is key. In essence, the ability to capture and recreate traditional singing voices through digital means presents a fascinating opportunity, but one that must be navigated carefully to ensure both the preservation and the integrity of these invaluable musical legacies.
1. **Capturing the Essence of Sound**: Transforming the intricate harmonies of analog recordings into digital voice prints demands a nuanced understanding of signal processing. We're essentially taking a continuous wave and breaking it into discrete chunks of data, which can sometimes compromise the original sound's richness. It's a fascinating but also challenging process.
2. **Maintaining Harmonic Integrity**: A critical aspect of this conversion is maintaining phase coherence in the digital representation. This ensures that the harmonic components of the sound remain aligned, crucial for accurately replicating complex vocal passages, especially those characteristic of traditional folk music where intricate harmonies play a vital role.
3. **Beyond Standard Tuning**: Folk music frequently incorporates microtones, subtle variations in pitch not always found in standard Western musical scales. Digital voice prints must be capable of accurately capturing these nuanced shifts, which necessitate algorithms sensitive to microtonal deviations. This ensures authenticity when recreating historical performances.
4. **The Importance of Sampling**: The sampling rate, essentially how frequently the analog wave is measured, significantly impacts the quality of the resulting digital audio. Higher sampling rates, like 192 kHz, allow for greater precision in capturing subtle elements like breath control and vocal inflections, both vital for the success of voice cloning technology.
5. **The Voice's Unique Fingerprint**: Replicating vocal timbre, the unique sonic character of a voice, is a complex undertaking. Spectral modeling and other advanced techniques are used to approximate timbre more closely, giving AI systems a better chance of replicating the distinct sonic characteristics that define each singer.
6. **The Breath Behind the Voice**: Breath control, often overlooked in the conversation around singing, significantly influences a performance's emotional impact. AI can analyze audio data to decode these breath patterns, revealing how breath is employed to enhance expression. This analysis can add a level of realism to cloned voices, particularly in narrative contexts like audiobooks.
7. **AI's Growing Emotional Intelligence**: Voice cloning models are increasingly incorporating emotion recognition algorithms. These algorithms analyze subtle changes in vocal inflections, allowing AI to recreate not only the sound but also the emotions conveyed in a performance, which is critical in genres like folk music where emotional resonance is so vital.
8. **Vibrato's Intricate Dance**: Vibrato, that subtle warbling in the voice, adds a rich emotional depth. Understanding its nuances – speed, depth, etc. – is crucial for accurate digital replication. AI models need to be able to recognize the varied vibrato styles employed by different singers to ensure the cloned output stays true to the original artist.
9. **Accents and Dialects in the Voice**: Regional accents and distinct pronunciation patterns hold crucial cultural information. Integrating these features into AI training models requires extensive linguistic analysis to ensure that the cloned voice accurately reflects the cultural and geographic context inherent in traditional vocal styles.
10. **A Balancing Act of Refinement**: Modern voice cloning tools often incorporate real-time feedback loops allowing for rapid adjustments and refinements. While this flexibility is valuable, it also carries a risk—the potential to stray too far from the original artist's sonic signature. A careful and measured approach is necessary to ensure that the artist's essence isn't lost in the process of refinement.
Voice Cloning in Folk Music How AI Preserves Traditional Singing Techniques from Three Historic Albums - Voice Synthesis Tools Capture Regional Singing Accents and Ornamentations
Voice synthesis tools are becoming increasingly adept at capturing the subtle nuances of regional singing styles, including accents and ornamentation. These tools leverage complex algorithms to analyze vocal patterns, like the intricate use of microtones and culturally specific melodic variations. This allows them to not only replicate the voices of traditional singers but also reproduce the unique features that define their artistry. Consequently, voice cloning technology can enhance the authenticity of recordings used in various applications, like audiobook production or podcast creation, preserving the sounds of traditional music. However, the development of such powerful tools also necessitates careful consideration of the ethical implications. There's a potential for the misrepresentation of cultural heritage if not used thoughtfully. It's vital to strike a balance between technological advancement and the respect for original artistic intent, ensuring the legacy of folk music is both preserved and celebrated responsibly through the use of voice synthesis.
Voice synthesis tools are becoming increasingly adept at capturing the subtle variations in pitch, known as microtones, which are common in traditional folk music styles. This ability is key to authentically reproducing the regional nuances often found in folk singing. While capturing the full emotional impact and subtle inflections of traditional singers is challenging, these tools are progressing.
Replicating the full dynamic range of traditional singers—which can easily span over 30 decibels—remains a substantial hurdle for voice cloning. Achieving this dynamic control is crucial for a truly impactful experience in audiobook narration or podcast production, as it heavily influences the emotional impact of a performance.
Modern approaches to voice synthesis utilize spectral modeling, essentially breaking down a singer's voice into its fundamental frequencies. This detailed analysis helps capture the unique timbre of a particular voice and allows for more nuanced reproductions, whether for podcasts or other audio applications. This level of precision is what separates the increasingly sophisticated synthesizers from less accurate attempts.
Incorporating emotion recognition algorithms into voice synthesis is an active area of research. These algorithms aim to analyze the subtle emotional cues embedded in a performance, including shifts in pitch and rhythmic variations, to help synthesize a voice that emulates the emotions originally present in the recording. This is particularly significant in folk music, where the emotional delivery is an essential element.
Breath control is a frequently overlooked, but critical element of traditional singing, and researchers are learning how to apply this knowledge to voice cloning. By examining recordings, they can analyze how the singer utilizes breath to create emotional depth. These breathing patterns, when incorporated into voice clones, can create a more convincing experience, particularly when used in narrative formats or podcasts where breathing patterns add a layer of naturalism.
The fidelity of the cloned voice is directly related to the resolution of the source recordings. Achieving the highest level of accuracy in a cloned performance requires exceptionally high-resolution audio, with sample rates reaching up to 192kHz. This degree of detail captures subtle voice characteristics like minute timbral variations or even minor imperfections in the original vocal performance, contributing to a higher degree of authenticity in the final product.
Another area of focus for AI research is capturing and recreating the distinct regional accents and dialects found in folk music. This feature is crucial for ensuring cultural accuracy and authenticity, as pronunciation and dialect are essential elements of many folk traditions. Preserving this regional specificity is important if these technologies are to accurately and respectfully represent the specific cultures within the folk music community.
Understanding the complexity of vibrato—its speed, depth, and nuances—is a challenging aspect of voice cloning. Different singers have distinct vibrato patterns, and replicating those variations is critical for recreating the unique emotive qualities of a particular performer. This fine level of control is an evolving area of voice cloning research.
The development of real-time feedback loops in some voice synthesis tools is an interesting development. These feedback loops enable users to make immediate adjustments to a voice clone. While offering valuable flexibility, this capability also poses a risk: the potential for modifications to stray too far from the original, raising questions about how far one can 'adjust' an original artist's voice.
The study of temporal patterns helps uncover the timing and phrasing within a singer's performance. Analyzing these patterns is essential for producing a clone that accurately captures the rhythmic qualities and inflection patterns characteristic of traditional folk singing, a nuanced and often intricate area of human musical expression.
Voice Cloning in Folk Music How AI Preserves Traditional Singing Techniques from Three Historic Albums - Audio Restoration Methods Meet Machine Learning in Traditional Music Archives
The intersection of audio restoration techniques and machine learning is revolutionizing how traditional music archives safeguard their collections. Modern AI methods, such as diffusion models and GANs, are being used to restore old and damaged audio recordings, improving the quality of historically significant performances. These tools can enhance the clarity and fidelity of these recordings, bringing a new level of accessibility to the music. However, this powerful combination of technology and cultural preservation also brings forth important considerations regarding authenticity. The ability to reconstruct artistic voices, particularly in musical genres brimming with emotion and cultural depth, presents an ethical dilemma. As these techniques become more refined, we face increasingly complex questions about how to balance innovation and the reverence for historical recordings. This development has wide-ranging implications for both scholarly research and creative applications in various audio productions, including podcasts, audiobooks, and even modern music composition. There's an inherent tension in striving for both restoration and retaining the core essence of the original recordings, and it's a balance that will need to be constantly addressed.
Digital voice prints, derived from analog recordings, provide a level of detail that goes beyond simple audio transfer. We can now meticulously examine elements like pitch, inflection, and vocal dynamism that might be lost in standard conversion processes. This deeper understanding unlocks the possibility of truly replicating a voice while preserving the artistry of the original singer.
Traditional folk music often incorporates microtones—subtle shifts in pitch that fall between standard Western notes. Researchers are developing AI-powered voice synthesis tools that can capture these microtonal nuances, which is key to accurately capturing the specific characteristics of regional singing styles. This highlights the importance of AI in preserving the unique soundscapes associated with particular musical traditions.
The sampling rate, which determines how frequently audio signals are measured, plays a crucial role in voice cloning. Higher sampling rates, like 192 kHz, allow for a more detailed capture of delicate aspects like breath control and vocal inflections. This level of fidelity is critical for ensuring that AI-generated voices reflect the subtleties of the original performances.
Spectral modeling offers a sophisticated approach to voice replication. It involves breaking down a singer's voice into its fundamental frequencies to reveal the distinct timbre that defines their sound. This is vital in applications like audiobooks and podcasts, where replicating the character of a traditional voice is important for maintaining authenticity.
Breath control, a subtle but crucial aspect of singing that conveys emotion, is now being investigated in the context of AI. Analyzing breathing patterns within recordings reveals how singers use breath to enhance their expression. Applying this knowledge to voice cloning can enhance the realism of AI-generated performances, particularly in narrative contexts where breath control can add to the sense of naturalness and authenticity.
Folk singers often have extraordinarily wide vocal ranges, sometimes exceeding 30 decibels. Replicating this dynamic range in a cloned voice is a significant challenge. Capturing this wide spectrum of sound is particularly crucial when dealing with emotional music, where dynamic variations are often used to express a broad range of feelings.
AI models are now being designed with the capacity to identify and analyze emotional cues present in a singer's performance. This ability allows AI to capture not just the sonic features of the voice but also the emotional nuance present in the original performance. This ability is important, given that folk music often conveys powerful emotions through the singer's voice.
The intricate variations of vibrato—its speed, depth, and nuances—present a particularly complex challenge for voice cloning technologies. Each singer's vibrato is unique, and replicating these specific features is crucial for accurately reflecting the emotional authenticity of the original performer.
Regional accents and dialects are vital components of many folk singing traditions. Ensuring that voice models capture these specific elements is essential for cultural authenticity. This requires incorporating linguistic analysis into the AI training process. However, one must be extremely cautious to avoid unintentional cultural misrepresentation.
Some advanced voice synthesis systems now include real-time feedback loops. This enables users to fine-tune a voice clone during the cloning process. While this adaptability is useful, it also raises a question about the balance between enhancing a clone and straying too far from the original performer's essence. This is an area that requires careful consideration.
More Posts from clonemyvoice.io: