Vocaloids vs AI Voice Synthesis Key Technical Differences in Digital Voice Production
Vocaloids vs AI Voice Synthesis Key Technical Differences in Digital Voice Production - Core Synthesis Methods From Concatenation to Neural Networks
The core of how we generate synthetic voices has seen a dramatic shift, moving from simpler methods to complex neural networks. Early approaches involved stitching together pre-recorded snippets of speech, a technique known as concatenative synthesis. These methods, which often relied on techniques like HMMs and unit selection, laid the groundwork for more advanced systems. However, the emergence of deep learning has fundamentally changed the landscape of voice synthesis. Neural networks, with their ability to learn intricate patterns in audio data, now enable the production of remarkably natural-sounding speech with less dependence on extensive pre-recorded libraries. This change has impacted not just the general creation of speech from text but also the realm of creating artificial singing voices, highlighting the increasing role of AI in producing high-quality, expressive audio. As this technology matures, there's an increasing emphasis on making these systems more responsive to user needs, facilitating finer control over the generated sound.
Fundamental approaches to synthesizing speech involve techniques like concatenative synthesis, which essentially stitches together pre-recorded snippets of sound like individual phonemes, and parametric synthesis, which takes a more algorithmic approach, generating sound from scratch using mathematical models. We've seen a shift from these older techniques towards neural networks, particularly recurrent neural networks and their variations like LSTMs. These neural networks are powerful tools for generating naturally-sounding speech based on large amounts of training data.
One important method is vector quantization, which significantly reduces the storage space needed for voice samples by representing them with fewer bits without noticeable loss in quality. The move away from concatenative techniques and towards neural synthesis has brought about a decline in the frequency of voice artifacts, those distracting imperfections that were noticeable in older synthetic voices, leading to a smoother, more lifelike experience.
Significant advancements in TTS have been driven by models like Tacotron and WaveNet, which combine features like linguistic and prosodic elements into the generated speech, giving it a sense of emotion and emphasis. This is especially beneficial for audiobooks and other narrative applications where the expression of voice plays a key role. Voice cloning has gone beyond mimicking the basic pitch and tone, and is now able to capture unique voice characteristics like breathing and pauses, making conversational AI far more realistic.
Attention mechanisms within these neural networks give the model the ability to focus on important parts of the input, resulting in improved understanding and better pronunciation. However, the development of these complex voice synthesis models relies on substantial amounts of training data – often thousands of hours of audio. This can create challenges in data collection and ethical implications relating to rights and permissions related to these voice recordings.
There has been ongoing effort to develop synthetic voice systems that can adjust to various speaking styles, allowing for seamless transitions between casual and formal speech. This is becoming vital for customizability in systems like voice assistants and digital personas. Finally, the growth of computational resources, especially improved GPUs, has drastically reduced the time needed to train complex speech models. This has opened the door for more developers to explore and implement high-quality voice synthesis in their own applications. There's still much to discover in terms of improving vocal characteristics like emotional expressiveness and nuanced articulation, and these innovations hold exciting potential for areas like voice acting and personalized audio experiences.
Vocaloids vs AI Voice Synthesis Key Technical Differences in Digital Voice Production - Voice Recording Techniques Pre Production Differences
The preparatory phase of voice recording significantly impacts the final product, especially when comparing traditional techniques to AI-driven methods. Older approaches, like those found in VOCALOID, rely on capturing individual speech sounds, like vowels and consonants, across various pitches. This segmented approach, while foundational, can constrain the natural flow and emotional depth achievable in the resulting vocalizations. AI voice synthesis, on the other hand, utilizes machine learning to sculpt vocal nuances and articulation with greater precision, leading to more expressive and realistic outcomes. This shift away from solely relying on extensive pre-recorded sound libraries allows for greater creative freedom, particularly when applying these methods to applications such as creating audiobooks or podcasts. As voice cloning continues to mature, the line between human and AI voices becomes increasingly indistinct, prompting a discussion on the very nature of voice artistry and its authenticity in a digitally-driven world. While both approaches hold their strengths, the ability to achieve complex, nuanced expression through AI synthesis undeniably expands the creative possibilities within sound design.
When it comes to creating high-quality voice recordings, the steps taken before hitting the record button are crucial. The choice of microphone plays a significant role, with condenser mics often preferred in studios for their sensitivity, while dynamic mics are more robust for live settings. The recording environment itself also matters greatly. Poor acoustics with lots of reflective surfaces can cause unwanted echoes, muddying the sound. A space designed to minimize those reflections and enhance clarity is ideal.
Vocalists, like musicians playing an instrument, need to warm up their vocal cords. These exercises improve performance quality and endurance. Additionally, modern recording often uses a technique called overdubbing, where multiple takes of a vocal part are layered to create a fuller, richer sound. This can be quite labor-intensive, but it's key for crafting the desired sonic texture, particularly in music styles like pop or electronic music.
Careful microphone placement is another key element. Placing a microphone close to the performer creates a more intimate vocal tone, while positioning it further away can incorporate more of the surrounding environment. The distance and angle chosen have a major impact on the final audio. A related concern is dealing with plosives—the sharp bursts of sound produced by sounds like 'p' and 'b'. Tools like pop filters are helpful here, reducing the harshness and minimizing the need for post-recording clean up.
During recording, monitoring what you're capturing is critical. High-quality studio headphones let the performer hear themselves alongside the backing track, enabling them to refine their delivery and overall performance in real time. This feedback loop is instrumental in getting the best possible result.
Voice cloning efforts, a fascinating aspect of current voice synthesis technology, are further enhanced by pre-production coaching. This helps both the voice performer and the AI training process. The performer learns how to effectively capture the key characteristics and styles they need to reproduce. The goal is to extract the unique aspects of a person's voice – its subtle inflections, breathing patterns, emotional cues – and transfer them to the synthesized output as accurately as possible.
Moreover, before recording, understanding how dynamic a vocal performance will be is helpful. Knowing how loud and quiet parts of the vocal will be affects decisions about things like compression and the addition of effects like reverb or delay. These effects, when carefully applied, can enhance the natural sound rather than overwhelming it. With voice cloning specifically, creating custom voice profiles is essential. This means meticulously capturing the details that make an individual’s voice distinct. The goal is to train AI systems to synthesize highly accurate clones by capturing the individual's characteristic inflections and tonal nuances. These careful pre-production efforts can make a big difference in the final voice output, be it for voice acting, audiobooks, podcasts, or the increasingly sophisticated world of AI-generated voices.
While the technology behind voice synthesis is continually evolving, the foundations of creating quality recordings remain rooted in well-planned pre-production. Understanding the subtle details of microphone choice, room acoustics, and vocal performance are all key to generating the best possible results.
Vocaloids vs AI Voice Synthesis Key Technical Differences in Digital Voice Production - Real Time Processing Speed and Resource Requirements
### Real-Time Processing Speed and Resource Requirements
The move towards AI in voice synthesis has brought about changes in how quickly we can process audio and the resources needed to do so. Modern AI voice synthesis systems, like some VOCALOID versions, are designed for low-latency operation, meaning that you can manipulate the voice in real-time without significant delays. This is a big benefit for tasks like podcasting or audiobooks, where the timing of the voice is crucial to the story. However, the intricate nature of these AI models usually necessitates powerful computers, especially during the initial training phase of the model. This can lead to higher energy use and a need for specialized hardware. Finding the sweet spot between the speed of processing and the amount of energy consumed is an ongoing challenge for engineers who are trying to improve AI voice synthesis.
When exploring the world of digital voice creation, particularly with vocaloids and AI-based systems, the speed and resources needed for real-time processing reveal some fascinating insights. Let's delve into a few aspects that often get overlooked.
First, the matter of latency is crucial, especially in applications that demand responsiveness like live performances or voice assistants. A delay of even a mere 20 milliseconds can be jarring to listeners, as our brains perceive interruptions quite readily at that threshold. This means achieving seamless interactions requires remarkably fast processing to keep up.
Second, the computational demands of neural-based voice synthesis, like those found in systems like Tacotron or WaveNet, can be substantial. Generating voice in real-time can necessitate upwards of 100 GigaFLOPS (billion floating-point operations per second). Such demanding operations typically call for powerful GPUs, which can increase costs for developers and those employing this technology.
Third, there's an intriguing adaptability found in some advanced voice synthesis systems. These can dynamically adjust their resource consumption based on the complexity of the spoken content. Simpler sentences might run smoothly with less computational effort. However, narrations that demand a greater depth of emotional expression or nuanced articulation can quickly ramp up the demands on CPUs and GPUs.
Fourth, the sheer memory footprint required for these models can vary considerably, ranging from hundreds of megabytes to several gigabytes, depending on the model's sophistication. This stands in stark contrast to older concatenative synthesis techniques that rely on smaller voice banks, allowing them to operate efficiently with a far smaller memory footprint.
Fifth, many modern voice synthesis approaches have leaned on parallel processing to lessen the effects of latency. This involves dividing the tasks into smaller, concurrent processes and harnessing the power of multi-core processors. The ability to tackle multiple calculations simultaneously is a capability that wasn't as readily available in more traditional voice synthesis techniques.
Sixth, the data used to train a voice synthesis model plays a substantial role in how efficiently it operates in real time. Models trained on large, diverse datasets—often thousands of hours of recorded speech—are better equipped to accurately and quickly predict the next piece of vocal output, reducing the overall resource burden.
Seventh, a trade-off often arises between audio quality and the resources a system uses in real-time applications. Generating high-resolution audio, like 24-bit, requires more computational power and storage compared to standard 16-bit. However, in contexts like podcasts or audiobooks, the increased fidelity may not be readily apparent to listeners.
Eighth, in certain applications, like voice assistants, real-time processing needs to keep up with a voice update frequency of 16kHz or higher. This rapid output is needed for clear communication, but naturally necessitates a system with substantial processing capabilities.
Ninth, even the surrounding acoustic environment can impact how accurately real-time voice synthesis performs. Noise in the environment can increase the challenges for the model and often leads to a greater need for noise reduction algorithms to maintain the desired quality and clarity of the synthetic voice output.
Tenth, we are seeing new advancements in hardware acceleration, specifically with things like tensor processing units (TPUs). These specialized processors show the potential for significantly increasing the speed at which neural networks used in voice synthesis can run. The advantage is that they can often handle specific types of tasks far more efficiently than typical CPUs or GPUs, leading to reduced latency and more efficient use of resources.
In conclusion, the interplay between technological capabilities and practical applications in the world of voice synthesis is quite intricate. The considerations and challenges engineers face in developing responsive and high-quality synthetic voices underscore the complexity of the field.
Vocaloids vs AI Voice Synthesis Key Technical Differences in Digital Voice Production - Phoneme Mapping Approaches in Digital Voice Production
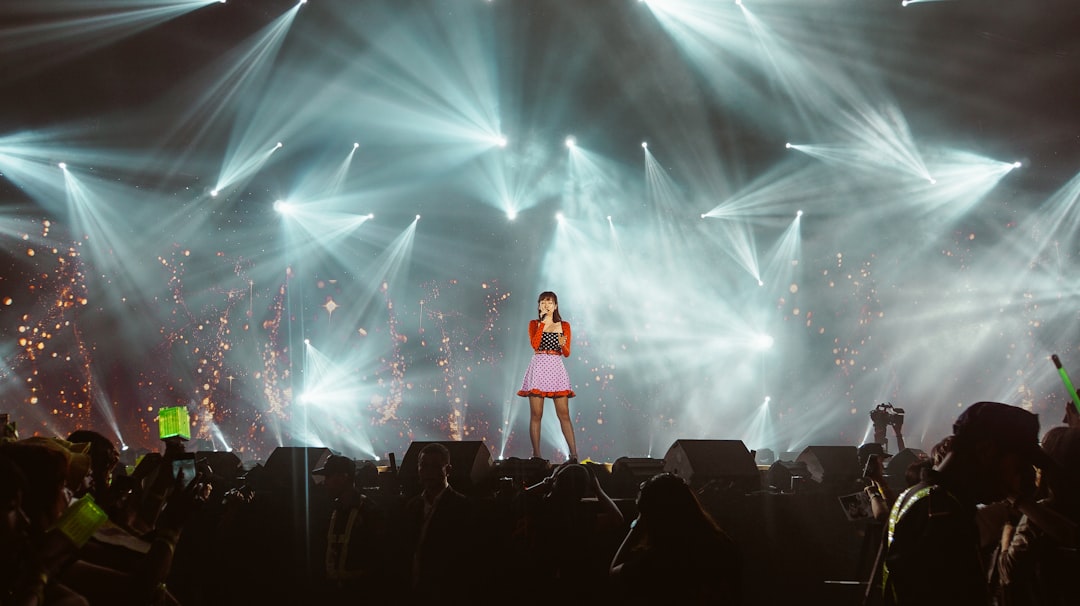
Phoneme mapping, the process of associating text-based phonemes with corresponding audio, stands as a central element in the creation of synthetic voices. Traditional approaches, frequently employed in Vocaloid software, rely on a segmented method where individual phonemes, like vowels and consonants, are recorded separately. This approach, while foundational, often results in a less natural flow and restricted emotional range in the output speech.
In contrast, AI-powered phoneme mapping has brought about a shift. Advanced machine learning techniques, including deep learning models, enable more accurate phoneme boundary detection. This leads to a significant improvement in the smoothness and naturalness of synthesized speech. By leveraging vast datasets and neural network architectures, AI systems can learn complex relationships between phonemes and their acoustic manifestations, enhancing the accuracy and fidelity of the generated speech. This increased precision allows for a finer degree of control over articulation and prosody, leading to more nuanced expression and a heightened sense of realism.
The implications of improved phoneme mapping are far-reaching. For voice cloning applications, the ability to map phonemes more precisely enhances the accuracy with which a target voice can be replicated. Similarly, the creation of audiobooks or podcasts benefits greatly as synthetic voices are better equipped to convey a wider range of emotions and inflections. However, there are continuing challenges to overcome. Achieving truly seamless emotional expression in synthesized voices remains a complex task. Yet, the continuing advancements in AI phoneme mapping suggest that future synthetic voices may become increasingly indistinguishable from human speech, blurring the line between human and artificial vocal performance.
Here are 10 interesting points about how phonemes are mapped in digital voice production, especially as it relates to voice cloning, audio books, and podcasts:
1. **Fine-Grained Control**: Phoneme mapping offers incredibly precise control over individual sounds in synthetic speech. This allows for adjustments to specific phonemes to mirror a speaker's unique pronunciation or intonation, making the voice sound more authentic.
2. **Reducing Reliance on Data**: Newer phoneme mapping methods are lessening the need for massive amounts of training data in voice cloning. Instead of requiring a huge collection of recordings from the target voice, some models can achieve good results with fewer samples by cleverly reusing and modifying existing phoneme data.
3. **Emotion and Rhythm**: Recent developments in phoneme mapping are allowing us to encode emotional nuances and rhythmic patterns into synthesized speech. This means synthetic voices can change pitch and tone during speech, leading to better expression of emotions, which is important for storytelling in audio formats.
4. **Contextual Phoneme Variations**: Phonemes don't always sound the same. They can change slightly depending on the surrounding sounds. This is called "coarticulation." Good phoneme mapping accounts for this, improving the naturalness of synthetic speech by simulating these subtle changes.
5. **The Importance of Vector Quantization**: Phoneme mapping frequently uses vector quantization to represent phonetic data efficiently. This not only minimizes storage requirements but also boosts the processing speed of the voice generation software, making real-time applications more viable.
6. **Blending Phonemes for Smoothness**: Some phoneme mapping approaches use techniques to blend adjacent phonemes, making transitions in synthetic speech smoother. This reduces the robotic quality often found in older synthetic voices, leading to more fluid and natural-sounding speech.
7. **The Key to Voice Cloning**: The success of voice cloning relies heavily on the precision of phoneme mapping. Techniques that capture not just basic phoneme sounds but also subtle elements like breathing and pauses significantly boost the realism of cloned voices.
8. **Adapting Speech on the Fly**: Advanced phoneme mapping techniques can adapt synthetic voices in real-time based on user interactions. For instance, they can adjust emotional tone or speaking speed based on a listener's feedback, creating a more interactive experience for listeners of podcasts and audio books.
9. **Linguistic Contributions**: Insights from the field of linguistics have strongly influenced phoneme mapping methods. Understanding how people produce and perceive sounds has helped engineers create models that better replicate natural speech patterns, which is crucial for audiobooks and conversational AI systems.
10. **Deep Learning and Phoneme Mapping**: Modern phoneme mapping often employs deep learning frameworks that combine phonetic information with broader contextual cues from the written text. This integration increases the accuracy of predicting how phonemes should sound in different contexts, reducing errors and improving the overall quality of the synthetic voice.
These intricate details highlight the fascinating development of voice production technologies and the role of phoneme mapping in achieving natural and expressive synthetic voices.
Vocaloids vs AI Voice Synthesis Key Technical Differences in Digital Voice Production - Target Applications From Music Production to Voice Assistants
The applications of voice synthesis technology have broadened significantly, extending from music creation to the development of interactive voice assistants. AI-powered voice generation systems now utilize machine learning to produce remarkably realistic vocalizations, finding use in creative areas like audiobook production and podcasting. The enhanced expressiveness of these synthetic voices can make listening experiences more engaging and impactful. Music production has been influenced by both traditional approaches, such as Vocaloid, which provides specialized tools for composing with synthesized voices, and newer AI methods which enable the creation of personalized voice clones, capturing the unique nuances of a person's speaking style. This growing sophistication in voice synthesis sparks crucial conversations around the nature of vocal performance in a world where the line between human and artificial voices becomes increasingly difficult to discern. As the technology continues to mature, we can expect even more innovative applications within sound design, but with these advancements come the need for careful consideration of the potential implications for vocal artistry and its future.
The application of AI in sound production, particularly voice synthesis, has expanded beyond basic speech generation. We're now seeing it utilized across a wide range of domains, from crafting music to powering voice assistants, and it's constantly evolving.
One of the intriguing developments is the use of AI in music production. While there has always been skepticism around the long-term viability of AI generated music, particularly due to its somewhat imitative nature, these systems are capable of revolutionizing how musical scores and vocal elements are created. They’ve certainly impacted how music is created, offering possibilities for new types of sound design and potentially assisting in the creative process.
AI voice cloning, another aspect of this field, has the potential to accurately recreate a person's voice, capturing their unique characteristics such as tone and vocal nuances. The ability to create a digital replica of a person's voice has made AI voice cloning a hot topic, especially when considering its impact on voice acting and the creation of personalized audio experiences.
Voice assistant technology has also benefitted from advances in AI-powered voice synthesis. By utilizing human-like vocal patterns, AI voice assistants can engage users in a more intuitive and emotionally resonant way. These virtual companions are finding applications across diverse areas like interactive storytelling or acting as guides through immersive environments.
Audiobooks and podcasting are also being reshaped by these technologies. The ability to generate realistic and expressive voices is allowing for the creation of new storytelling experiences. This increased control over audio output makes it easier for storytellers to achieve specific vocal tones or emotional nuances, providing listeners with a richer and potentially more immersive auditory environment.
However, the application of these technologies also gives rise to some questions. The human-like qualities of AI-generated voices raise ethical concerns, including the potential for manipulation and misuse. Further, the need to collect vast quantities of audio data for model training brings up important considerations about privacy and the intellectual property rights of vocal performers.
Furthermore, as AI-powered voice synthesis continues to mature, it's become apparent that models trained on large datasets can handle simple sentences fairly well. Yet, the moment you introduce content requiring more nuanced articulation or emotional expression, the demands on the computational resources used to generate the voices increases. Balancing the level of desired expressiveness against the computational costs required to achieve it is an ongoing area of research for those developing these technologies.
Another aspect being studied is real-time processing, which is essential for tasks that need quick responses, like virtual assistants. The development of specialized processors like TPUs shows promise for making these systems even more efficient.
Despite the challenges, continuous advancements in generative music models and AI voice synthesis are expanding the boundaries of digital voice production. This innovation is driving creative fields forward, pushing the limits of what is considered possible in terms of creating sounds and bringing audio narratives to life. It is a rapidly evolving area that will undoubtedly continue to shape how we experience sound in the coming years.
Vocaloids vs AI Voice Synthesis Key Technical Differences in Digital Voice Production - Output Quality Analysis Natural Speech vs Stylized Singing
When comparing the output of voice synthesis systems, the distinction between natural speech and the stylized singing often found in Vocaloid or other AI-driven music applications reveals crucial differences in how the audio is generated. The goal of singing voice synthesis (SVS) is to produce audio that emulates the qualities of human singers, a task increasingly aided by the use of sophisticated deep learning methods. One key difference lies in the fact that the majority of sounds produced during singing are voiced—roughly 90%—demonstrating the complex interplay between vocal production techniques needed to achieve the expressive and dynamic qualities expected in musical contexts. Newer SVS systems, like VISinger2, are utilizing self-supervised learning methods to create synthesized singing voices that are more natural and expressive, highlighting a trend toward more sophisticated integration of the musical and linguistic aspects of vocal performance. The quest to synthesize audio that is nearly indistinguishable from real human singing remains a significant technical challenge, especially as the application of this technology spreads to domains such as audiobook production and podcasting, which demand high levels of naturalism.
### Examining the Output Quality of Natural Speech versus Stylized Singing
When comparing the output quality of natural speech synthesis with stylized singing, like that produced by Vocaloids, some interesting patterns emerge. Researchers have observed that in certain situations, synthesized speech can actually be perceived as more natural than stylized singing, particularly when aspects like pitch variations are faithfully replicated. This highlights the importance of subtle nuances in sound production in creating a sense of realism for the listener.
Interestingly, AI voice synthesis models that are designed to incorporate emotions into the generated speech frequently surpass stylized singing in terms of conveying feelings. The capacity to dynamically adjust pitch and inflection allows these voices to evoke a stronger emotional response within listeners, making them better suited for narrative applications.
One of the challenges encountered with stylized singing is that it requires more sophisticated models due to the complexities of capturing vocal aspects like breath control and vibrato. This added complexity can lead to higher processing needs and longer latency compared to speech synthesis, demonstrating a trade-off between expressive capabilities and performance speed.
AI voice synthesis models for natural speech have a strong tendency to adapt the phonetic outputs based on the words and phrases around them, which is a less common feature in stylized singing. This feature enhances clarity and improves the listener's ability to grasp the content, particularly beneficial for applications like audiobooks where conveying information effectively is paramount.
The advancements in neural voice synthesis have brought about a significant decrease in the unwanted sounds that often pop up in stylized singing, issues that can be distracting to the listener. This improvement in smoothness leads to a listening experience that can often feel closer to human performance.
There's a noticeable difference in vocal range. Natural speech synthesis has the capacity to cover a wider variety of voices more smoothly compared to stylized singing. Stylized singing can sometimes sound confined if the voice data doesn't match the natural range of human vocalists included in the training data.
In audio book production, the editing process with naturally generated speech is often easier than with stylized singing. Stylized singing might need extra work to preserve the sound quality when changes are made, especially when there are large variations in the intensity of the audio, creating a more complex editing workflow.
The process of voice cloning for stylized singing calls for larger datasets that are specific to the vocal style desired compared to the creation of natural speech, which often draws from more general voice datasets. This disparity brings to light the challenges of finding high-quality singing data that reflects a wide range of styles.
It's notable that audiences often show a preference for the authenticity of natural speech in storytelling applications, whereas stylized singing is more frequently associated with musical contexts. This audience expectation affects how developers construct their AI models for particular tasks, impacting the desired quality and realism of the output.
Real-time processing presents unique difficulties for stylized singing. The computational requirements can be notably higher than for natural speech synthesis, especially when features like pitch correction and effects like reverb are utilized in real time. This presents engineers with unique challenges, particularly for applications requiring immediate performance feedback.
These observations reveal crucial differences in the quality and performance attributes between natural speech and stylized singing, impacting their respective roles in voice cloning, audiobook production, and podcasting. A deeper understanding of these intricacies helps developers to refine their approaches and ultimately build more engaging and higher-quality audio experiences.
More Posts from clonemyvoice.io: