The Science Behind Real-Time Voice Cloning How Modern AI Models Process Speech in Under 3 Seconds
The Science Behind Real-Time Voice Cloning How Modern AI Models Process Speech in Under 3 Seconds - Neural Network Speech Pattern Analysis Models Turn Voice Into Numbers
Neural networks are transforming how we analyze speech, converting the complex sounds of human voices into numerical representations. These models break down the intricate features of speech, including intonation, pitch variations, and unique accents, into a numerical language that computers can understand. This ability to capture the essence of a voice in numbers forms the foundation for sophisticated voice cloning technologies. The insights gained through this numerical representation are crucial for various applications, including crafting realistic audiobooks, generating dynamic podcasts with personalized voices, and even replicating voices for entertainment purposes. Recent advancements, like factorized diffusion models, have further accelerated the process, making real-time voice cloning a reality with reduced latency and exceptional audio quality. The speed and accuracy of these models allow for the creation of voice clones that can seamlessly adapt to different contexts and styles, generating authentic-sounding voices that are remarkably lifelike. This ability to numerically dissect and recreate voices has opened new horizons in the realm of voice synthesis, making the creation of personalized and engaging audio experiences achievable.
In the realm of speech pattern analysis, neural networks transform the complexities of human voice into a numerical format, enabling machines to comprehend and replicate it. Techniques like MFCCs are frequently employed, distilling audio signals into a representation of frequency and tonal characteristics. This numerical mapping forms the foundation for the model to discern and emulate the subtle nuances present in human speech.
Training these deep learning models involves exposing them to massive datasets of spoken language, encompassing a diverse range of speaking styles and emotional expressions. This process helps the models capture not only the literal words but also the personality and intent embedded within the speaker's voice. The ability to replicate prosody, encompassing the rhythm, stress, and intonation patterns, has significantly enhanced the naturalness and appeal of synthesized speech, making it more suitable for applications like audiobooks and podcast production.
The burgeoning field of voice cloning enables the reproduction of a speaker's voice using just a few audio samples. With advancements in speech synthesis, these techniques can now generate synthetic speech across multiple languages and dialects, opening doors to greater accessibility and localization for creators. The efficiency of this process is noteworthy, with some systems capable of processing and producing speech within mere milliseconds. This responsiveness is crucial for interactive applications requiring real-time responses.
Unlike older text-to-speech systems that often generated robotic-sounding voices, contemporary neural network-based models employ waveform synthesis methods such as WaveNet. These methods produce smoother, more natural-sounding audio waves, mirroring human speech patterns with greater accuracy.
Addressing the variability of human emotions in speech remains a central challenge. Advanced voice cloning models now incorporate emotional cues, aiming for a more authentic reproduction of the speaker's feelings through subtle shifts in tone and pace.
Beyond capturing basic speech characteristics, neural networks can also discern and emulate individual speaking nuances like accents and idiosyncrasies. This not only improves the realism of cloned voices but also paves the way for developing personalized voice assistants that resonate more with users.
As voice cloning technology advances and incorporates more sophisticated audio analysis, the potential for misuse comes into sharper focus. Issues of security and ethical implications warrant careful consideration, leading to discussions on how to mitigate risks of disinformation and identity theft.
The ongoing advancements in hardware, particularly the development of faster GPUs, have played a pivotal role in speeding up the training process for neural networks. This has dramatically reduced the time required to develop high-quality voice cloning models, pushing the limits of what is achievable within real-time applications.
The Science Behind Real-Time Voice Cloning How Modern AI Models Process Speech in Under 3 Seconds - Converting Text to Mel Spectrograms With Deep Learning
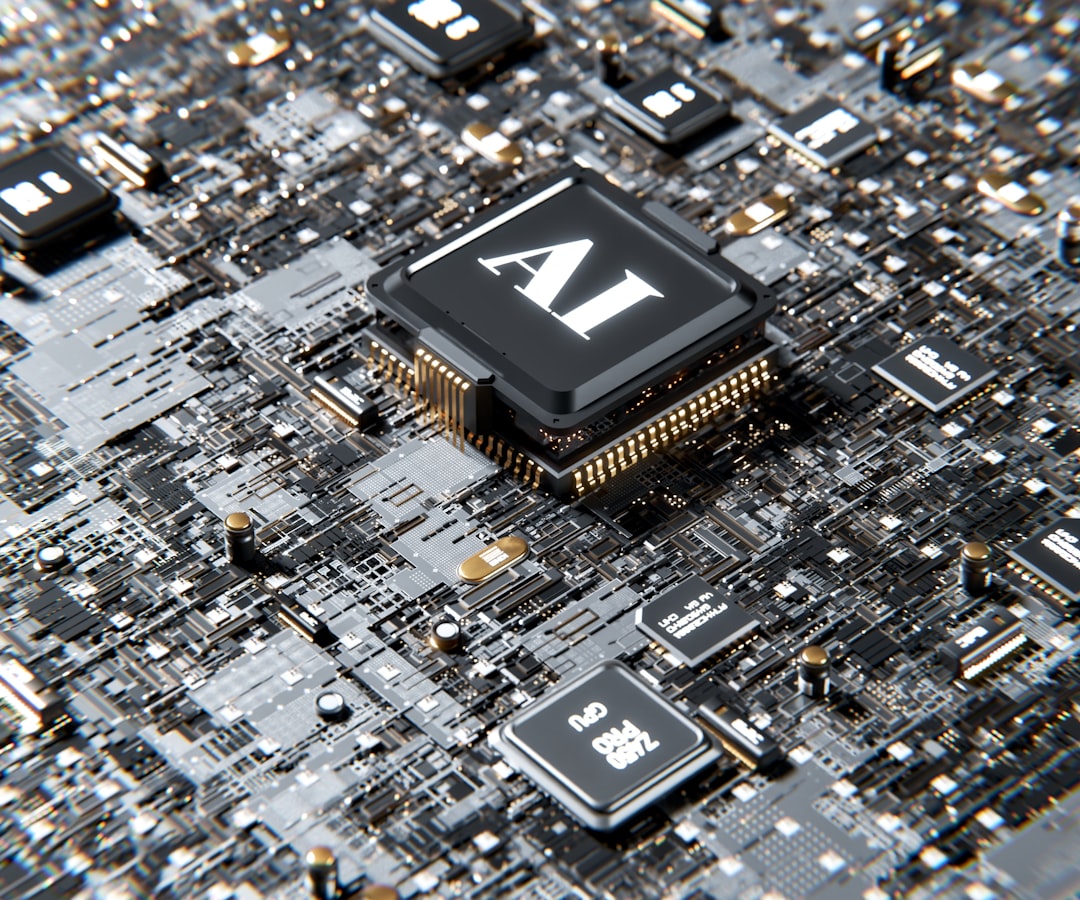
In the realm of voice cloning and other speech-related applications, converting text into Mel spectrograms has emerged as a pivotal technique. Mel spectrograms, essentially visual representations of audio, offer a more insightful way for deep learning models to process speech compared to raw audio waveforms. These models, often leveraging convolutional neural networks (CNNs), can analyze the frequency and amplitude of sound, encoded in a way similar to images. This capability is particularly important in Text-to-Speech (TTS) systems, where the goal is to synthesize human-like speech directly from written text.
The use of Mel spectrograms facilitates the production of high-quality, natural-sounding audio for a wide range of applications, including audiobooks, podcast creation, and, of course, voice cloning. By representing sound in a format easily digestible by CNNs, it allows for efficient processing and synthesis. Moreover, this approach has proven especially useful for real-time voice cloning, enabling quick cloning of voices with only minimal sample audio. This ability to quickly replicate a person's voice using a limited number of samples opens up new frontiers in voice generation technology.
However, as the sophistication of these models grows, a persistent challenge remains— faithfully capturing the inherent emotional nuances and individual characteristics of human voices. Striving for natural, expressive speech requires addressing the complexities of human emotion, including tone and pacing, which are subtle but crucial aspects of communication. Moving forward, successful advancements in voice cloning will depend on continued improvements in these areas, ensuring that the final synthesized voice sounds authentic and natural.
Mel spectrograms serve as a crucial bridge between raw audio and the deep learning models used in real-time voice cloning systems. They're designed to mimic how humans perceive sound, focusing on the frequencies we're most sensitive to, making them ideal for analyzing speech patterns. This representation retains a detailed temporal structure, allowing the models to distinguish between quickly spoken sounds – crucial for generating natural-sounding synthetic speech.
During the training process of voice cloning models, we often manipulate mel spectrograms through data augmentation techniques like pitch shifting and adding noise. These methods enhance model resilience and improve their ability to synthesize a diverse range of voices by simulating various speaking environments. Furthermore, they serve to reduce the sheer volume of audio data, enabling a more focused analysis on key speech elements which ultimately speeds up the model's learning.
The initial step in creating mel spectrograms involves using a Fast Fourier Transform (FFT) to switch the audio signal's representation from the time domain to the frequency domain. Subsequent application of mel filters fine-tunes the frequency information to capture those most pertinent to speech. This process ensures the output maintains the natural flow of sound over time – a key requirement for seamless voice cloning.
While mel spectrograms excel at capturing fundamental tonal features, expressing the complexity of human emotions in synthetic speech remains a challenge. However, recent advancements leverage nuanced variations in mel spectrogram features to encode various emotional states, illustrating the sophistication that modern voice cloning has achieved.
Beyond just mimicking individual voices, mel spectrograms also play a key role in voice conversion, where the characteristics of one voice are transferred to another. This process entails manipulating the mel spectrogram representation to create a synthesized voice that preserves the original message while adopting the features of a target voice.
Interestingly, generative adversarial networks (GANs) are now often used in conjunction with mel spectrograms to produce extremely realistic audio. The GAN architecture learns from feedback to create more lifelike synthetic voices.
The reverse process—generating audio from mel spectrograms—presents its own set of challenges. Reconstructing the audio waveform from the spectrogram with perfect fidelity is difficult, but techniques like Griffin-Lim have been developed to improve this step. Research continues to explore ways to enhance the quality of voice synthesis by refining this inverse spectrogram process.
In the realm of voice cloning, mel spectrograms are indispensable for representing audio in a format suitable for deep learning. While there's still much to explore in terms of achieving perfect sound replication, the mel spectrogram approach has undeniably propelled the advancement of voice cloning, offering a path to generate highly realistic and nuanced synthetic speech.
The Science Behind Real-Time Voice Cloning How Modern AI Models Process Speech in Under 3 Seconds - Voice Sample Processing Through Advanced Speech Recognition
Voice sample processing, powered by advanced speech recognition, has revolutionized how we interact with and replicate human speech. These systems leverage sophisticated deep learning models to analyze audio, capturing intricate details like pronunciation, intonation, and even subtle emotional nuances embedded within a voice. This detailed analysis allows for remarkably accurate voice cloning, enabling the production of incredibly lifelike audio for diverse uses, including audiobook creation and crafting engaging podcast experiences. However, this very power presents challenges – the increasing ability to create convincingly synthetic voices raises concerns about the potential for misuse and the need for better deepfake detection systems. The future of voice cloning will likely depend on further refinements in algorithms, ensuring that these technologies are used responsibly and ethically while further enhancing the quality and speed of synthesized audio. The quest for even more natural-sounding synthetic speech continues to drive research in this rapidly evolving field.
Mel spectrograms are designed with human auditory perception in mind, focusing on frequencies most crucial for understanding speech. This approach makes them ideal for speech recognition and cloning systems, enabling machines to prioritize the most relevant audio components for accurately reproducing human communication. Modern voice cloning systems can remarkably reconstruct a voice with only a few seconds of audio, showcasing the power of sophisticated algorithms to extract core vocal features.
Prosody, the musicality of speech, is a key factor in communication, conveying subtleties like sarcasm or excitement. Sophisticated voice cloning strives to meticulously capture prosody, resulting in more natural and contextually appropriate synthetic speech. Techniques like WaveNet have revolutionized waveform synthesis, moving beyond traditional concatenative methods. These models learn the temporal dynamics of speech directly from waveforms, resulting in synthetic voices that sound less robotic and more human-like.
While considerable progress has been made, incorporating emotional cues into real-time voice cloning still faces challenges. Subtle shifts in tone and pace, which are essential for communicating emotions, often get lost in the process, highlighting a critical area for future research. Generative Adversarial Networks (GANs) are increasingly paired with mel spectrograms to create incredibly lifelike audio. GANs work by training on real and synthesized samples, iteratively improving the quality of the output via competition between two neural networks, leading to more realistic-sounding synthetic voices.
Training voice cloning models involves techniques like adding background noise or manipulating pitch. This 'audio augmentation' strengthens the model's robustness, allowing it to handle a wider variety of real-world audio conditions. These methods improve their effectiveness across numerous applications, from audiobooks to virtual assistants. The Fast Fourier Transform (FFT) plays a crucial role in preparing audio data for processing. It translates audio signals from the time domain into the frequency domain, preserving essential speech features. This transformation allows the model to identify how frequency components relate to perceived speech sounds, which aids in the process of analysis and reconstruction.
The requirement for immediate audio generation in interactive applications like podcasts and live communication has fueled rapid advancements in processing capabilities. The goal isn't just accurate replication, but also reducing latency to near-human conversational speeds. Voice conversion, the process of modifying one voice to mimic another, relies heavily on detailed manipulation of mel spectrograms. This capability has implications for diverse fields, including film dubbing and gaming, where the seamless integration of various vocal styles is essential. While there are still intricacies in achieving perfect replication, the mel spectrogram approach has undeniably accelerated voice cloning advancements, opening doors to increasingly natural and nuanced synthetic speech.
The Science Behind Real-Time Voice Cloning How Modern AI Models Process Speech in Under 3 Seconds - Audio Pre Processing and Enhancement for Clean Voice Input
The quality of voice input significantly impacts the effectiveness of voice cloning and other speech-based applications. To achieve high-quality voice cloning, raw audio requires careful pre-processing and enhancement. This involves techniques like noise reduction, which helps eliminate unwanted background sounds, and feature extraction, where relevant aspects of speech are isolated. Voice activity detection (VAD) plays a crucial role by identifying periods of actual speech within the audio, enabling more efficient processing by the machine learning models.
These preprocessing steps are essential for optimizing the audio data for analysis by deep learning algorithms. By isolating core vocal features and minimizing the influence of background distractions, the models can focus on extracting the unique characteristics of a person's voice. This, in turn, allows for the creation of more accurate and lifelike voice clones. The pursuit of truly natural-sounding synthesized voices continues to drive research in this area. Innovations in audio enhancement are constantly emerging, aiming to further elevate the quality of synthetic speech.
However, achieving a balance between processing speed, accuracy, and capturing the nuanced emotional aspects of speech remains a persistent challenge. Finding effective methods to represent complex human speech in a format conducive for both fast and accurate analysis by AI is a focus of ongoing research. As voice cloning technology matures and finds wider adoption in diverse fields, from audiobooks to podcast production, the demands for high-quality, natural-sounding voices will only increase, spurring further advancements in audio preprocessing and enhancement.
Cleaning up raw audio before it's used in voice cloning or other speech applications is essential for getting good results. Techniques like Linear Predictive Coding (LPC) can analyze voice characteristics and compress the data, making it easier to process while still preserving the core features of the voice. This can be a crucial step in real-time applications.
Adapting to background noise is another challenge. Adaptive filtering methods can dynamically adjust to changing noise conditions, allowing for clearer voice inputs, even in noisy environments. This is quite useful for scenarios where voices are being recorded in less-than-ideal conditions. The human auditory system is remarkably sensitive to sound changes, so accurate capturing of voice requires audio sampling at relatively high rates. We usually aim for sampling rates of 44.1 kHz or higher to make sure we get all the nuances of the voice that would otherwise get lost.
Deep learning, especially convolutional neural networks (CNNs), is playing a major role in pre-processing. CNNs seem to process audio waveforms in a way that resembles human auditory processing. This allows for efficient feature extraction and improving the quality of synthesized speech. Researchers also investigate how to infer emotional qualities from voice by analyzing pitch and amplitude variations. These insights are helpful for replicating not just the voice, but also the emotions that were present during the recording, which improves the realism of audiobooks and podcasts that are generated from these models.
Techniques like spectral subtraction, which aim to remove background noise, are commonly used in the pre-processing pipeline. Psychoacoustics, the study of how humans perceive sound, can guide model development, allowing for a more natural-sounding voice. This approach to modelling human perception is quite clever.
Capturing the dynamic changes in speech rate and timing is critical for the naturalness of cloned voices. How these changes impact the model's ability to generate believable voices is something that is actively researched. FPGA technology is making real-time processing feasible. FPGAs are capable of running complex algorithms with very little latency, and this type of hardware acceleration is increasingly essential for interactive applications like real-time voice cloning and chatbots. While a lot of progress has been made, there's still plenty to discover when it comes to perfecting the process of voice replication. The field of voice cloning is exciting and constantly evolving, with ongoing exploration into even more sophisticated audio processing and enhanced synthesis methods.
The Science Behind Real-Time Voice Cloning How Modern AI Models Process Speech in Under 3 Seconds - Voice Pattern Recognition Using Synthetic Speech Models
Voice pattern recognition, powered by synthetic speech models, represents a major advancement in our ability to understand and recreate human communication using technology. These models utilize sophisticated deep learning techniques to analyze and synthesize speech patterns, capturing subtle elements like emotional cues, intonation, and individual pronunciation styles. However, achieving a truly natural-sounding synthetic voice remains a hurdle, especially when replicating the full spectrum of human emotions and the unique characteristics of each speaker's voice. As this area of research matures, it's crucial to address the potential for misuse, such as the creation of realistic deepfakes. This necessitates the development of reliable detection methods and a thoughtful discussion about the ethical implications of widespread voice cloning technology. The field is continually evolving, with the potential to revolutionize areas like audiobook production, podcasting, and personalized voice interfaces, while simultaneously requiring careful consideration of the ethical landscape surrounding synthetic voice generation.
Voice pattern recognition, a core component of voice cloning technology, relies on sophisticated techniques to analyze and understand the intricate details of human speech. These systems can detect subtle phonetic variations that even experienced listeners might miss, allowing for remarkably accurate replication of regional accents and dialects. This capability is particularly valuable in applications like audiobook production, where authenticity is paramount.
Moreover, advanced voice cloning models have the potential to capture and encode the emotional states embedded within a speaker's voice. By carefully analyzing fluctuations in pitch, tempo, and loudness, these systems can effectively synthesize voices that not only sound realistic but also convey a variety of emotions and attitudes. This enhances the user experience in applications like podcasting where engaging and nuanced audio is desired.
Central to the process of voice pattern recognition is the use of Mel Frequency Cepstral Coefficients (MFCCs). These coefficients act as a numerical representation of the short-term power spectrum of sound. Deep learning models utilize MFCCs to effectively extract essential vocal features, significantly improving the accuracy of synthesized voices.
To ensure that synthesized voices are robust and adaptable to diverse environments, researchers often employ data augmentation techniques during the training process. For example, adding synthetic background noise or artificially shifting the pitch of audio samples enhances a model's ability to handle variations in audio quality and creates more versatile applications for diverse environments.
Adaptive filter technology is another crucial aspect of voice cloning, particularly in real-world settings where background noise is unavoidable. These real-time filters can dynamically adjust to the acoustic environment, improving the clarity of the audio input and ensuring that the voice recognition or cloning process remains effective even in challenging conditions.
The preprocessing of audio signals is essential for efficient voice cloning. Techniques like Linear Predictive Coding (LPC) and Fast Fourier Transform (FFT) play a key role in preparing audio for analysis by neural networks. They effectively simplify complex audio data into a format that's easier for computers to process, accelerating the training process and making real-time applications feasible.
The reconstruction of audio from mel spectrograms—a process known as inverse spectrogram reconstruction—presents a unique challenge. Algorithms like Griffin-Lim are employed to enhance the fidelity of the synthesized audio, allowing for the retrieval of dynamic vocal characteristics after the synthesis process is complete.
Modern voice cloning models have the capability to learn the temporal dynamics of speech directly from raw audio waveforms. This contrasts with older methods, allowing for a more nuanced representation of human speech patterns and leading to synthetic voices that sound less robotic and more natural.
Capturing the subtleties of speech requires high-resolution audio sampling. Voice cloning systems typically employ sampling rates of 44.1 kHz or higher to capture the full range of vocal nuances, ensuring that even subtle shifts in tone and inflection are accurately reproduced.
Generative Adversarial Networks (GANs) are becoming increasingly popular in voice cloning because of their ability to create exceptionally realistic audio. The iterative nature of GANs allows for the continuous refinement of synthetic voices through a competition between two networks, pushing the boundaries of how convincing a synthetic voice can be. This opens new possibilities for enhancing the realism and quality of cloned voices, creating new frontiers in voice cloning applications.
While the field of voice pattern recognition continues to evolve, these advancements clearly demonstrate the power of deep learning and signal processing to create highly realistic synthetic voices. The potential applications of this technology are vast, ranging from enhanced audiobook and podcast experiences to creating personalized voice assistants and fostering creative endeavors. However, the ethical implications of this technology should be carefully considered, as the ability to realistically mimic human voices raises important questions about authenticity, security, and the potential for misuse.
The Science Behind Real-Time Voice Cloning How Modern AI Models Process Speech in Under 3 Seconds - Voice Output Generation With Zero Shot Voice Cloning
Voice output generation through zero-shot voice cloning marks a significant leap in the field of AI-powered speech synthesis. It allows for the creation of synthetic voices that mimic a specific speaker's voice with just a short audio sample, eliminating the need for prior training on a large dataset of that speaker's voice. This ability to rapidly generate a voice model from a small amount of audio opens doors for various uses, including quickly creating voice-overs for audiobooks, producing podcasts with a specific speaker's voice, and potentially enhancing other audio experiences.
Models like F5TTS are examples of open-source implementations that demonstrate the capabilities of zero-shot voice cloning. These models efficiently process audio, enabling quick and simple cloning of a voice from uploaded recordings. Other advancements, such as those found in OpenVoice technology, broaden the reach of voice cloning by allowing for the reproduction of diverse vocal characteristics, such as different accents, tones, and even emotions across various languages.
While zero-shot voice cloning offers exciting possibilities for content creation, it also raises important concerns. One major concern is the potential for misuse, such as creating realistic but potentially harmful audio deepfakes. The ability of these models to create convincing synthetic speech that mimics a specific person also underscores the need for ongoing research to enhance the ethical awareness of the technology and to develop ways to detect these AI-generated sounds. We must ensure that these tools are used for constructive purposes and that measures are in place to mitigate potential harms.
Zero-shot voice cloning is a fascinating development, allowing for the creation of a voice model using just a short audio snippet without any prior training specific to that speaker. This is particularly promising in areas like audiobook and podcast production where unique voices can be easily replicated. Models like F5TTS exemplify the potential of this approach, showcasing how easily accessible and flexible voice cloning can be. You can simply upload a high-quality audio clip and generate synthetic speech that mimics it with impressive accuracy.
Current models are incredibly fast, performing instant voice cloning in a matter of seconds, greatly improving the efficiency of text-to-speech (TTS) synthesis. OpenVoice is another interesting example, showcasing zero-shot voice conversion with the capability to accurately capture not only the tone but also accents and languages without needing extensive prior training for each individual voice. It even offers flexible controls over voice characteristics such as emotion, accent, pace, and intonation, allowing for a fine-tuning of the synthetic voice output.
Newer frameworks, such as AudioLM, which leverage language models, have shown remarkable advancements in zero-shot audio generation, suggesting that even higher speed and quality are within reach. The general concept of Instant Voice Cloning (IVC) has become a hot topic, referring to the ability of TTS models to instantly clone any voice from just a brief sample without needing speaker-specific training. The use of semantic and acoustic tokens in algorithms contributes to a more refined and accurate voice conversion process, enabling a better alignment of the generated speech with the target voice.
The success of zero-shot approaches in voice generation represents a shift toward more adaptive AI models, showcasing the growing agility and adaptability of these systems to produce diverse speech styles with exceptional speed. This rapid advancement continues to push the boundaries of what's possible with AI-driven voice cloning, raising new questions about potential use cases and the need for ethical considerations as these technologies become more prevalent. While the current speed and quality of voice replication is astonishing, it's still a young field, and ongoing research promises even more impressive advancements in the years to come, especially as they are increasingly applied to content production.
More Posts from clonemyvoice.io: