Get amazing AI audio voiceovers made for long-form content such as podcasts, presentations and social media. (Get started for free)
The Rise of AI Voice Cloning in Audiobook Production A 2024 Analysis
The Rise of AI Voice Cloning in Audiobook Production A 2024 Analysis - AI Algorithms Behind Voice Cloning Technology
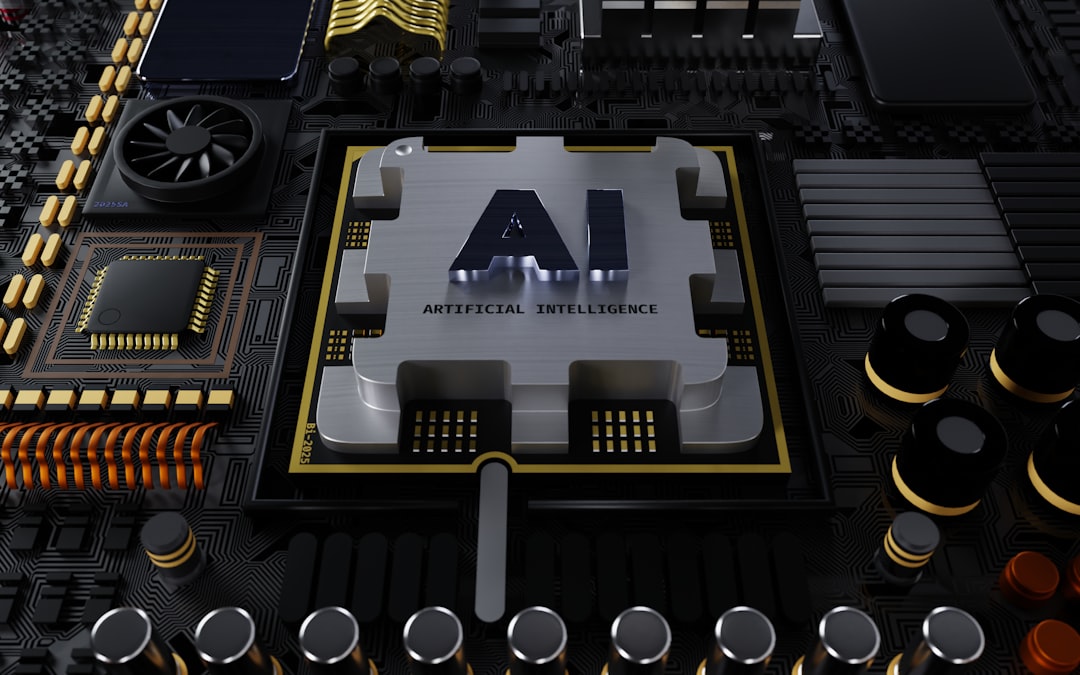
AI voice cloning technology hinges on intricate algorithms that enable the creation of remarkably realistic synthetic voices. These algorithms, often powered by deep learning methods, meticulously analyze vast amounts of audio data, dissecting the speaker's vocal characteristics. This includes aspects like tone, pitch, rhythm, and even subtle emotional cues present in their voice. Through this process, the algorithms learn to replicate the unique acoustic fingerprint of the speaker, effectively generating a digital twin of their voice.
The creation of a synthetic voice involves a multifaceted approach. Multiple algorithms work together to decode the speaker's voice patterns and then reconstruct them when prompted with new text. This complex interplay allows the creation of a voice that sounds eerily similar to the original speaker, paving the way for applications like audiobook narration and podcast production.
While offering exciting new possibilities in audio content creation, voice cloning also brings up valid ethical dilemmas. Concerns about the potential for misuse of this technology, such as generating fake audio content, are prominent. Furthermore, questions about authenticity and the potential for undermining trust in audio sources necessitate a cautious and deliberate approach to deploying these algorithms. Despite these concerns, the continued advancement of voice cloning promises to reshape the future of audio production, making it both more accessible and diverse in the years to come.
AI voice cloning, a fascinating field, often utilizes neural networks, particularly GANs (Generative Adversarial Networks), to create convincingly realistic audio. These networks consist of two parts: one generating synthetic voices, and another discriminating between real and fake ones. This constant competition pushes both models to refine their abilities, leading to more refined synthetic voices.
The effectiveness of a voice clone hinges significantly on the volume and diversity of the training data. Models exposed to a wider variety of audio recordings of a specific voice produce superior results. Conversely, limited data leads to artifacts and inconsistencies, impacting the natural flow of synthesized speech.
Techniques like emotional modeling are crucial for refining voice synthesis. By adjusting parameters like intonation, tempo, and emphasis during speech synthesis, these models can impart a range of emotions, giving the synthetic voice a more lifelike and nuanced feel.
Beyond replicating sound, voice cloning algorithms can capture a speaker's distinctive mannerisms and idiosyncrasies. This ability is quite impressive but, unfortunately, also opens up concerns about the potential for misuse, such as impersonating someone's voice without consent.
The frequency spectrum of cloned voices can be carefully adjusted to match the characteristics of the original voice. This involves meticulous analysis of the source voice's acoustic features, aiming to recreate its unique tonal qualities in the clone.
Some advanced systems can seamlessly manipulate the speed of synthesized speech without losing the intended emotional content. This adaptability is highly beneficial for audiobook production, allowing producers to tailor the pace to different listeners or story segments.
Evaluating the quality of synthesized voices requires specialized metrics that assess the realism of the audio. These automatic metrics aid developers in fine-tuning their models, resulting in more natural-sounding outputs.
Adding synthetic breathing sounds at appropriate intervals during audiobook narration can contribute to a more realistic and natural experience for the listener, further enhancing the immersive feel.
Combining voice cloning with text-to-speech technology creates exciting possibilities for dynamic audiobooks, where the voice's tone and inflection can change based on the story's context. Such adaptive narration promises a richer and more nuanced storytelling experience.
We see a growing trend in utilizing voice cloning to create personalized digital assistants. These assistants can mimic the voices of notable figures, injecting a unique personality into podcasting and other audio productions. While technically impressive, this trend raises ethical questions about potential misuse.
The progress of voice cloning is captivating, and it's exciting to ponder the possibilities. However, it is equally crucial to consider the responsible use of the technology, to prevent unintended consequences.
The Rise of AI Voice Cloning in Audiobook Production A 2024 Analysis - Data Collection and Processing for Synthetic Voices
The creation of convincing synthetic voices relies heavily on the quality and breadth of the audio data used to train AI models. Voice cloning techniques thrive on access to substantial audio recordings that capture a variety of speaking styles, emotional inflections, and contextual nuances. The effectiveness of a voice clone directly hinges on the quality of this input data, with high-quality recordings yielding vastly superior results compared to lower-quality sources. Current research is exploring ways to improve the selection and processing of data, specifically aiming to mitigate the effects of limited or imperfect datasets. This area is crucial because it impacts the degree to which the synthetic voice can accurately mirror the nuances and subtle intricacies of the source speaker.
The ability to generate remarkably realistic synthetic voices opens new pathways in audiobook production, podcasting, and other audio applications. However, this same capacity raises serious questions regarding potential misuse and ethical considerations. The creation of convincingly fake audio presents challenges related to authenticity and trust, making it increasingly important to ensure that advancements in this field are accompanied by a strong awareness of the risks involved. The future of AI voice cloning depends on the responsible application of this powerful technology, striking a delicate balance between technological innovation and preserving the integrity of audio content.
The creation of synthetic voices relies on complex algorithms that meticulously extract and analyze various vocal features from a source audio dataset. These features include phonetic elements, pauses, and even the rhythm and flow of speech (prosody). The goal is for the synthesized voice to not only accurately pronounce words, but also to capture the speaker's unique way of speaking.
Research suggests that exposing the voice cloning model to a diverse range of audio data, encompassing accents, emotional expressions, and ambient noise, leads to a more natural and less robotic-sounding synthetic voice. This emphasizes the critical need for diverse and comprehensive data collection for high-quality voice cloning.
Certain advanced voice synthesis models incorporate methods of emotional intelligence, allowing the synthesized voice to dynamically adjust its delivery based on contextual information from the text being read. For instance, during a suspenseful scene in an audiobook, the synthetic voice might naturally lower its pitch or slow down to reflect the tension.
Noise in the recording environment is a common hurdle in the voice cloning process. By incorporating advanced noise reduction techniques into both the data collection and processing stages, we can achieve clearer audio that improves the overall quality of the synthesized voice.
Modern voice cloning technologies have made it possible to produce synthetic voices in real-time. This feature is particularly helpful for text-to-speech applications such as audiobooks, where the narrative pace might fluctuate frequently.
A clever trick used to improve the realism of synthetic voices involves adding artificial breaths to the audio at appropriate intervals. This simulates the natural breathing patterns in human speech, which can reveal emotional states or subtle shifts in the story's narrative.
Spectral analysis, a technique that meticulously dissects a speaker's vocal timbre and frequency characteristics, is essential for achieving high-fidelity voice clones. This rigorous analysis allows the creation of synthetic voices with an impressively accurate sonic fingerprint, making them feel relatable to listeners.
A challenge that researchers are actively addressing is that voice cloning models trained primarily on data from a restricted demographic, such as a specific gender or age group, can have difficulty adapting to diverse voices. This limitation highlights the importance of incorporating a broad spectrum of voice data into the training process to prevent bias and ensure inclusivity in voice generation.
After the initial synthesis process, the voice clone typically undergoes post-processing to refine its quality. Techniques like pitch correction and equalization enhance the audio's clarity, ensuring the final product remains enjoyable and easy to listen to.
One of the remarkable aspects of modern voice cloning algorithms is their capacity to adapt and improve over time based on feedback. This means that the technology can be trained to become increasingly personalized and optimized for specific applications, potentially leading to more tailored audiobook narration or unique podcast voices.
The Rise of AI Voice Cloning in Audiobook Production A 2024 Analysis - Advancements in Speech Synthesis Naturalness
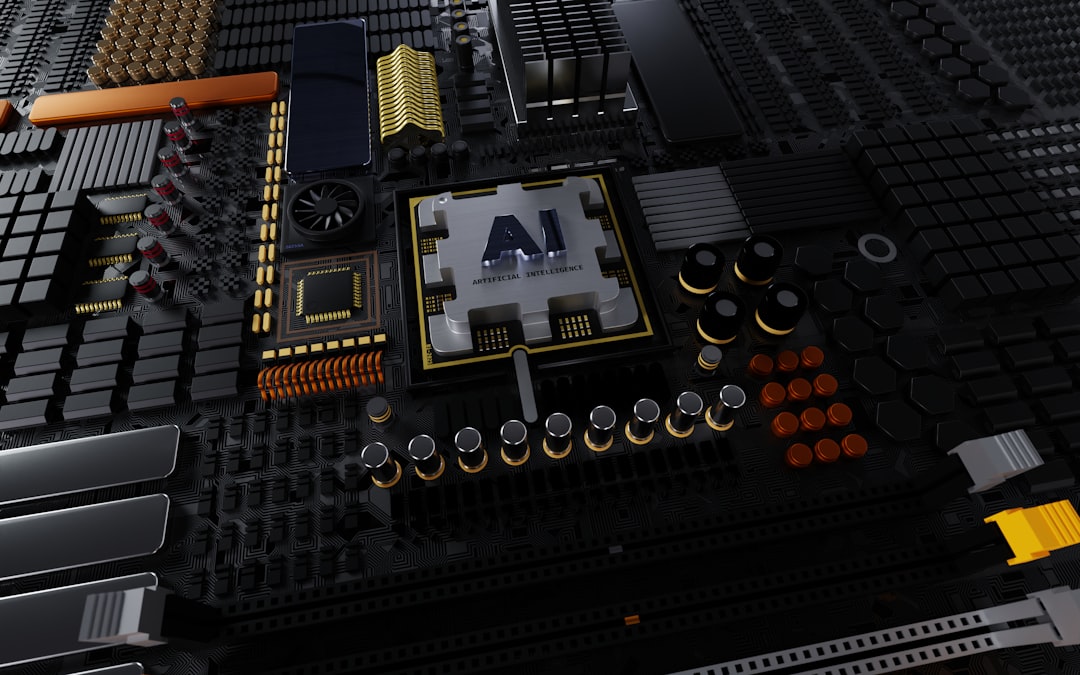
The field of speech synthesis has experienced a surge in advancements, notably in the naturalness of the produced voices. This progress is largely attributed to the implementation of sophisticated deep learning methods, allowing for real-time voice cloning that replicates human vocal nuances with remarkable accuracy. We're seeing AI-generated voices that capture not just the pronunciation of words, but also the unique intonation, rhythm, and even emotional expression of a human speaker. This increased fidelity is leading to more compelling listening experiences in audiobooks, podcasts, and other audio content.
The incorporation of techniques like emotional modeling allows these synthesized voices to convey a wider range of feelings, making the narration of stories or the delivery of information feel more human and less robotic. Moreover, features such as simulating breathing during pauses further enhance the authenticity of the audio, resulting in a more immersive listening experience.
However, even with these advancements, the challenge of seamlessly integrating emotional expressiveness into synthesized voices in dynamic contexts remains. This complexity, especially within applications like assistive technologies, calls for ongoing research to ensure these voices are consistently able to convey a full spectrum of emotions appropriately. The ethical considerations surrounding voice cloning technology continue to be paramount, as we move toward a future where AI-generated voices increasingly blur the lines between human and synthetic speech.
The field of speech synthesis has experienced remarkable strides in crafting voices that sound incredibly natural, a crucial advancement especially in the emerging realm of AI voice cloning. These improvements stem from a deeper understanding of how human speech is produced, allowing synthesized voices to capture intricate details that were previously elusive. For instance, the ability to replicate coarticulation, where sounds influence each other in a seamless flow, helps create synthesized speech that sounds far more conversational and less robotic.
Furthermore, we're seeing a growing ability to inject emotional nuance into synthetic voices. Through methods inspired by emotional intelligence, these AI systems can adjust aspects like pitch and pace based on the surrounding text. Imagine an audiobook where a tense scene is narrated with a naturally lower pitch, creating a sense of anticipation – this type of context-aware delivery adds a layer of realism absent in earlier speech synthesis models. Clever techniques, such as integrating artificial breathing patterns at natural intervals within the synthesized audio, also add to the realism, making the listening experience far more engaging.
Another intriguing aspect is the capacity for continuous learning in some advanced voice cloning systems. These AI models can learn and adapt based on listener feedback, continuously refining aspects like pacing, tone, and style, enabling customization for individual listeners. This highlights a move towards personalized and adaptive audio experiences.
Moreover, researchers have honed methods to painstakingly recreate a speaker's unique voice using spectral analysis, a technique that breaks down a voice into its constituent frequencies. This level of detail enables creation of synthetic voices that retain a speaker's distinct acoustic qualities, a crucial element for achieving a recognizable and natural listening experience. The ability to achieve real-time synthesis is further enhancing interactive applications, such as audiobooks and podcasts that change their flow based on listener choices.
However, the quest for natural-sounding voices also presents challenges. For example, imbalanced training datasets, perhaps limited to a particular demographic, can create limitations in the model's ability to adapt to a diverse range of voices and accents. Engineers are actively working to combat this bias by expanding the range of data used to train these models. And after synthesis, the voice often undergoes various post-processing steps like pitch correction and equalization to ensure it reaches a quality suitable for high-quality audio experiences. These improvements are not just refining the synthesized audio, but ensuring the listening experience remains engaging and enjoyable.
The future of speech synthesis looks increasingly promising with these improvements, especially within the context of AI voice cloning. While there's still much to explore, the capacity to create truly human-like voices in a variety of contexts, holds immense potential for audio content creators in diverse fields. However, with such remarkable advancements, comes the need for continued vigilance around ethical concerns and potential misuse.
The Rise of AI Voice Cloning in Audiobook Production A 2024 Analysis - Expanding Applications Beyond Audiobook Production
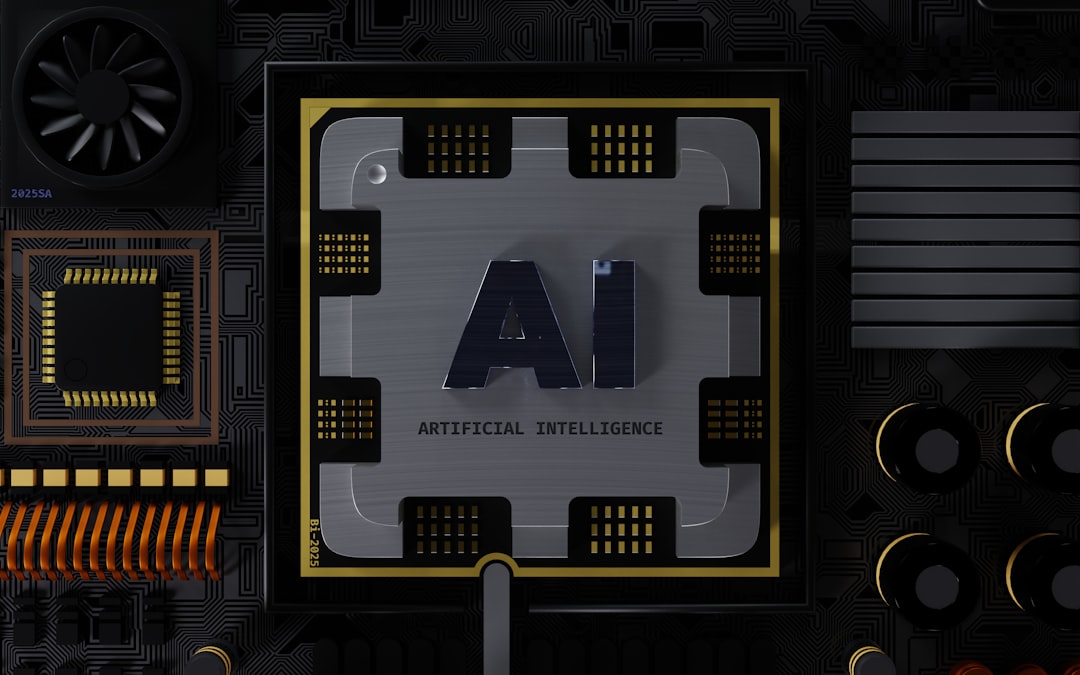
The rise of AI voice cloning is not limited to just audiobooks. Its potential reaches far beyond, impacting fields like podcasting, education, and entertainment. This technology's capability to create a diverse range of voices and deliver dynamic narrations is particularly useful in educational settings, especially for multilingual content. AI-generated voices can make storytelling more engaging and creative. While the ability to create audio content with ease presents a wealth of new opportunities for content creators, it's crucial to acknowledge and address the ethical concerns that arise. Questions about authenticity and the potential for malicious use of the technology are legitimate and require careful consideration. Moving forward, the future success of AI voice cloning depends on how we balance the exciting advancements with the need to maintain trust and integrity in audio content. Only with a responsible approach can we fully utilize this technology to create increasingly immersive and accessible audio experiences across a broader spectrum of platforms.
AI voice cloning, initially developed for audiobook production, is showing its versatility across a wider spectrum of audio applications. While it still faces challenges in fully replicating the intricate nuances of human speech, it's becoming increasingly sophisticated. The capability to create personalized podcast experiences with on-demand guest voices is one example. This approach expands podcasting possibilities, enabling collaborations that were previously difficult or impossible due to geographical or scheduling constraints.
Another intriguing area is real-time language adaptation. Voice synthesis models are being developed to switch between different languages and dialects instantaneously. Imagine a single audiobook narrated in multiple languages, each retaining the original speaker's characteristics. This development offers significant advantages in making audio content globally accessible.
The entertainment industry is also embracing AI voice cloning. It's now possible to create distinctive character voices for animation, gaming, or other forms of media without extensive voice actor sessions. This streamlines production pipelines and provides a more agile approach to character design, which can evolve alongside a storyline.
Furthermore, assistive technologies are benefiting from this evolution. Individuals with speech impairments can benefit from personalized synthetic voices that reflect their individual vocal patterns and emotional cues, fostering more natural and effective communication.
Some of the more cutting-edge research involves incorporating dynamic emotional ranges into voice synthesis. This involves training models to adjust tone and intonation in response to narrative cues. For instance, an audiobook could seamlessly transition to a darker tone during a suspenseful scene, making the listening experience far more immersive. Such advancements also have implications for training programs that help speakers improve their delivery.
The field of relaxation and sensory experiences is also witnessing the emergence of ASMR applications. Synthesized voices can be finely tuned to deliver calming narratives designed for specific audiences seeking a tranquil listening experience.
Even the field of therapy is exploring the potential of AI voice cloning. Personalized synthetic voices may be useful in providing emotional support or simulating conversations for patients undergoing cognitive behavioral therapy.
The capacity to create interactive audiobooks is another fascinating development. These narratives adapt to user choices in real-time, adjusting tone, pacing, and narrative branches to optimize engagement and personalize the experience.
Authors are starting to use voice cloning to develop distinctive, brand-specific narrator models for their books. This allows for a unique sonic identity that could potentially set their works apart in a competitive market.
The future trajectory of this technology hinges on enhancing model adaptability to diverse data inputs. Researchers are working to address the challenges of biased training datasets and limited voice representation. This effort seeks to establish more inclusive AI models that can produce a broader range of human voices, creating content that reflects the diversity of global audiences.
These examples showcase the evolving potential of AI voice cloning. It's clearly not confined to traditional audiobook production, with exciting implications for diverse fields and creative practices. Nevertheless, the ethical implications of this powerful technology must be consistently acknowledged and addressed as it evolves further.
The Rise of AI Voice Cloning in Audiobook Production A 2024 Analysis - Impact on Audiobook Creation Workflow and Costs
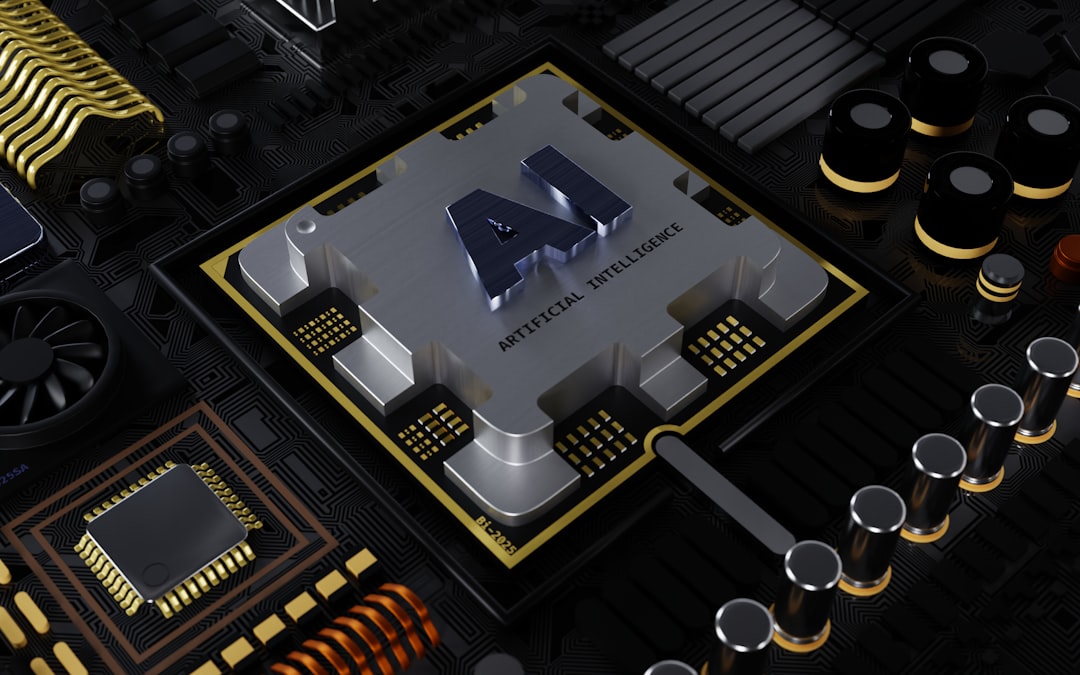
AI voice cloning is significantly changing how audiobooks are made, impacting both the process and the costs involved. The ability to quickly generate realistic synthetic voices using AI reduces reliance on human narrators, which can lead to lower production costs and faster production times. This opens up audiobook creation to authors and smaller publishers who previously couldn't afford the expense of traditional voice acting. However, current AI systems still struggle to replicate the subtle nuances and emotional depth found in human narration. This limitation raises questions about whether AI-narrated audiobooks can deliver the same kind of immersive experience that listeners are accustomed to. As AI voice cloning becomes more integrated into audiobook production, finding the right balance between efficiency and the quality of the listening experience will be crucial. The challenge moving forward will be to leverage AI's speed and affordability while preserving the emotional resonance and authenticity that make audiobooks compelling.
The integration of AI voice cloning is fundamentally altering the workflow and economics of audiobook creation. We're seeing a shift towards more automated processes, reducing the reliance on traditional voice actors and their lengthy recording sessions. Production timelines are becoming shorter as the entire process from manuscript to audio becomes more streamlined.
Modern AI-driven voice synthesis tools can adapt on the fly, modulating the tone and emotional nuances of the narration based on the story's context. This dynamic approach allows for a richer listening experience without the need for extensive post-production edits or multiple recording sessions. It's fascinating how a single audio file can now effectively change its character based on what's being read, adding a depth of expression that previously required multiple voice actors or painstaking editing.
Beyond just the words, certain voice cloning systems are starting to integrate subtle cues like pauses and simulated breaths, creating a more lifelike and natural-sounding experience for listeners. These features are helping to reduce that slightly robotic feel inherent in older text-to-speech technologies. This has huge implications for immersion, especially in longer-form audiobooks where a consistent and natural cadence is essential for holding listener engagement.
The ability to customize a narrator's voice for different language variations is also making audiobooks far more globally accessible. Imagine an audiobook where the narrator seamlessly shifts between languages, while still maintaining their unique vocal characteristics. This opens up opportunities for creators to reach a far broader audience than ever before.
Voice cloning isn't confined to audiobooks. It's being utilized to develop distinctive character voices in gaming or animation, leading to faster and more flexible creative processes. No longer are lengthy voice recording sessions the primary bottleneck, as AI models can create a range of voices quickly and with adaptability to match changing storylines.
Some of the most promising models are incorporating the concept of emotional intelligence into their synthesis, automatically adapting elements like pitch or speed based on emotional cues from the script. This is creating a deeper and more engaging interaction with listeners, bringing the audio to life in ways that were impossible with earlier technology. It's a particularly exciting area as we start to bridge the gap between purely synthetic and human speech in terms of expressive capacity.
Moreover, AI-powered voice systems are starting to utilize real-time listener feedback to refine their performance. This implies a future where a voice adapts its tone or pace based on an individual's preferences. It's a testament to the rapidly increasing capacity of these systems to personalize and optimize the listening experience.
Voice cloning is proving incredibly valuable in assistive technologies, particularly for individuals with speech impediments. These systems can generate highly personalized voices that mimic natural speech patterns and emotional expressions, enhancing communication capabilities in a powerful way.
One area of active research is creating more equitable and inclusive AI models. This involves refining the training datasets used to develop voice clones. When the training data is limited to a specific demographic, it can lead to biases that impact the quality and scope of generated voices. The focus now is on training with more diverse vocal samples so that the generated voices can accurately represent a broader spectrum of humanity.
Finally, the potential for therapeutic applications is an intriguing area. There's early-stage research exploring the use of personalized synthetic voices to deliver comfort or facilitate simulated conversation in therapy, particularly in situations where a person might feel more comfortable interacting with a synthesized voice. This field is still in its early phases but presents the exciting possibility of creating more relatable and engaging experiences within mental health settings.
All in all, it's an exciting time in the field of voice cloning. The impact on audiobook creation, and countless other audio applications, is already profound and will likely continue to reshape the way we create and experience audio content. However, this potent technology comes with an inherent need for caution and awareness of potential ethical concerns. The future of AI voice cloning depends on a responsible approach, one that balances exciting technological advancements with a commitment to maintaining the authenticity and integrity of audio content.
The Rise of AI Voice Cloning in Audiobook Production A 2024 Analysis - Challenges in Replicating Human Narration Nuances
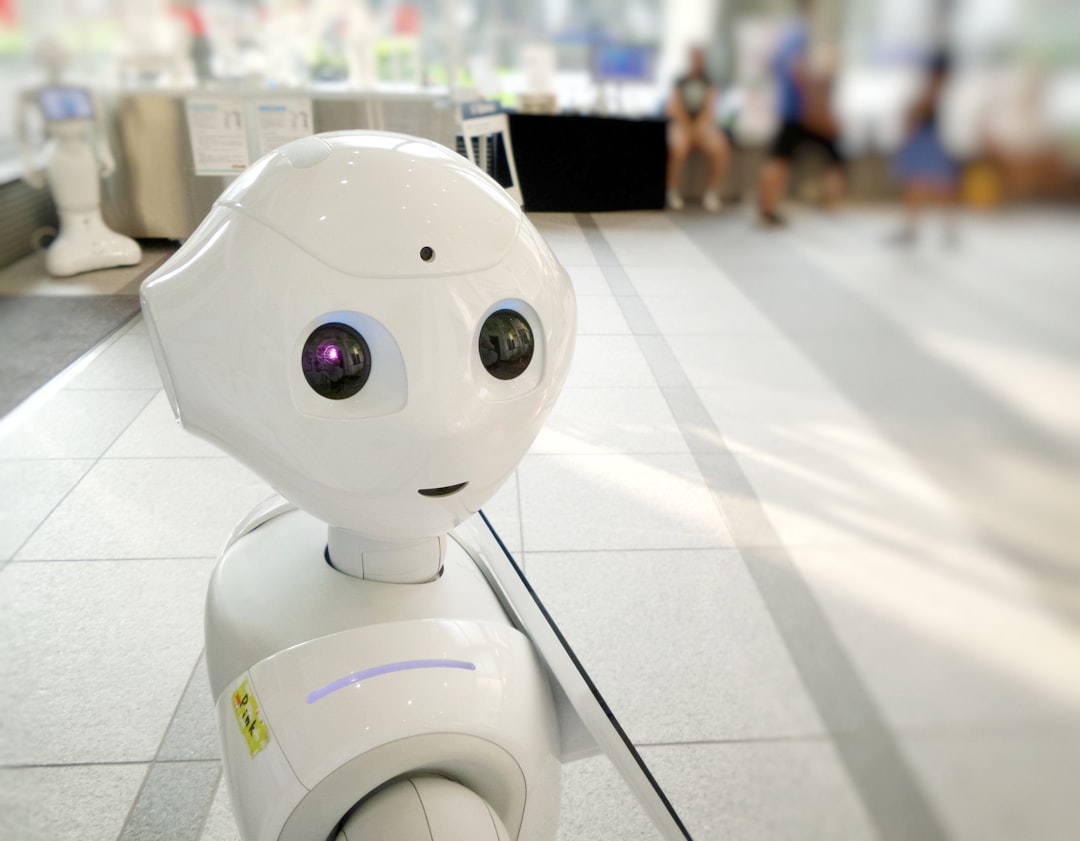
AI voice cloning has made impressive strides in replicating human speech, but accurately capturing the subtle emotional nuances of a human narrator remains a hurdle. While current technology can generate synthetic voices that sound remarkably realistic, recreating the intricate interplay of tone, pacing, and emotional inflection found in human narration is still an evolving challenge. The ability to seamlessly integrate emotional cues into synthesized audio is crucial for creating immersive audiobook experiences, and achieving this with the same finesse as a human narrator is proving difficult. The audiobook industry is at a crossroads as AI-powered voice cloning continues to gain traction. Balancing the benefits of faster, more affordable production with the listener's desire for a genuinely engaging and emotionally rich experience will be essential. Ultimately, the future of AI in audiobook narration will hinge on whether it can bridge this gap and ensure that the listener's experience is not compromised in the pursuit of efficiency.
While AI voice cloning has made remarkable strides in replicating human speech, several challenges remain in capturing the subtleties and nuances that characterize human narration. For instance, accurately reproducing the intricate details of prosody, pitch fluctuations, and speaking pace remains a hurdle for AI models. These elements are vital for audience engagement and emotional connection, and their absence can result in a slightly artificial feel in the resulting audio.
Though AI systems can simulate emotions through alterations in tone or tempo, replicating the full complexity of human emotional expression in nuanced contexts remains difficult. This gap can significantly hinder the listener's ability to connect with and be fully immersed in the narrated content.
Current efforts are focusing on developing AI models that can dynamically adapt their narrative style based on textual cues. This would involve, for example, a synthetic voice naturally increasing tension during thrilling passages. However, these systems are still in their early stages and often encounter inconsistencies in achieving the intended emotional shifts, revealing a key area that requires further refinement.
Adding artificial breathing sounds to synthesized voices is not merely a cosmetic trick. It's a technique that aims to replicate natural speech patterns and enhance the audio's organic feel. However, coordinating the timing of these breaths with the narrative flow and pacing remains a difficult problem.
Spectral analysis, used to painstakingly recreate the unique timbre of a voice, is essential for achieving high-fidelity voice cloning. But achieving a complete match to the intricate tonal characteristics of a human speaker remains challenging. These discrepancies can lead to synthetic voices that lack the richer and more detailed sonic properties present in human speech.
While personalized synthetic voices offer the potential to tailor narration to individual listeners, challenges arise when addressing culturally and regionally diverse speech patterns. This raises questions about the authenticity of the resulting voice. Expanding the diversity of data used to train these models is crucial to ensure inclusivity and avoid perpetuating biases.
Many voice clones go through substantial post-processing, involving steps such as pitch correction and equalization. While these steps enhance clarity, they can also inadvertently diminish the natural vocal variations that contribute to the expressiveness of human speech.
As voice cloning systems incorporate real-time listener feedback and become increasingly adaptive, it raises ethical questions about their capacity to accurately reflect the nuances of human narration and storytelling. There's a growing need for careful evaluation and monitoring to prevent unintended misrepresentation of a narrator's intended message.
Researchers are actively working on integrating more dynamic emotional ranges into AI-generated speech, aiming to create narratives that seamlessly shift between, for example, lighthearted and sombre tones. However, most current systems lack the finesse required to effectively execute such transitions.
Humans possess a remarkable innate capacity to communicate nuanced meanings through a combination of vocal inflections, body language, and emotional depth. Replicating this complex interaction through AI remains a considerable challenge, leaving questions about the extent to which synthetic voices can genuinely achieve a sense of authenticity. The ongoing work in this field represents a quest to push the boundaries of AI capabilities, and its success in replicating human narration's complexities is a fascinating and crucial area of research.
Get amazing AI audio voiceovers made for long-form content such as podcasts, presentations and social media. (Get started for free)
More Posts from clonemyvoice.io: