Get amazing AI audio voiceovers made for long-form content such as podcasts, presentations and social media. (Get started for free)
Troubleshooting Common Voice Cloning Subscription Issues A User's Guide
Troubleshooting Common Voice Cloning Subscription Issues A User's Guide - Identifying Dataset Quality Issues for Successful Voice Cloning
The quality of a voice clone hinges heavily on the quality of the dataset used to train the cloning model. Using a dataset with a mix of high-quality and low-quality audio can lead to inconsistent and unreliable results. Studies have indicated that filtering out poor-quality audio clips from the training dataset can significantly boost the performance of the voice cloning algorithms. This is particularly important when dealing with issues like a substantial difference between the amount of real voice and cloned voice samples in a dataset. Such imbalances can hinder a model's ability to accurately replicate a voice.
Ongoing improvements in voice cloning techniques, including methods that address audio noise and refine the initial processing stages of the audio data, further emphasize the importance of a strong starting dataset. These enhancements highlight the need for a well-curated dataset to produce natural-sounding and nuanced cloned voices. As the field continues to progress, addressing potential flaws in the initial dataset will continue to be a critical step in producing high-quality voice clones for a variety of applications.
Voice cloning, while a fascinating field, is sensitive to the quality of the input audio data used to train the models. The presence of inconsistent audio quality, like ambient noise or varying microphone types, can make it hard for the algorithms to properly isolate and model the intricacies of a speaker's voice. For instance, a high-fidelity condenser microphone might capture a richer sound spectrum than a dynamic microphone, affecting the final model's precision.
Moreover, the range of phonetic sounds in the training data plays a significant role. If the dataset lacks a sufficient variety of sounds, the resulting model might struggle with less frequently used words or phrases, leading to unnatural and clumsy output. Interestingly, a larger dataset doesn't always equate to a better model. Without a good mix of different speech patterns and contexts, the model can become overly specialized in certain patterns, essentially memorizing the training data instead of truly understanding the speech characteristics.
Furthermore, the emotional range within the training data can impact the cloned voice's expressiveness. If the dataset only captures a limited set of emotional tones, the cloned voice might sound flat and inexpressive. The same goes for the sampling rate used in the recordings. Lower sampling rates can strip away subtle details in a voice that are important for creating a natural-sounding clone. Conversely, higher sampling rates retain more detail but require greater storage and processing resources.
It's important to ensure the audio data is properly labeled. Incorrectly categorized parts of the data can confuse the voice cloning algorithm and lead to errors in replicating speech. Similarly, the recording environment, such as room acoustics, can significantly alter the captured sound. Reflections and echoes can distort the sound in unpredictable ways, leading to complications in the voice modeling process. This challenge is especially relevant when dealing with language-specific features, for example, tonal languages which require precise modeling of pitch variations to be understandable.
Even a factor as simple as the time of day a recording is made can impact the final cloned voice quality. Our voices can change throughout the day, so if the training data includes recordings made at times when the speaker's voice is not at its best, the resulting clone might sound inconsistent or unnatural. This illustrates the delicate nature of voice cloning and the need for meticulous attention to all factors that can influence the outcome.
Troubleshooting Common Voice Cloning Subscription Issues A User's Guide - Navigating the Voice Clone Verification Process
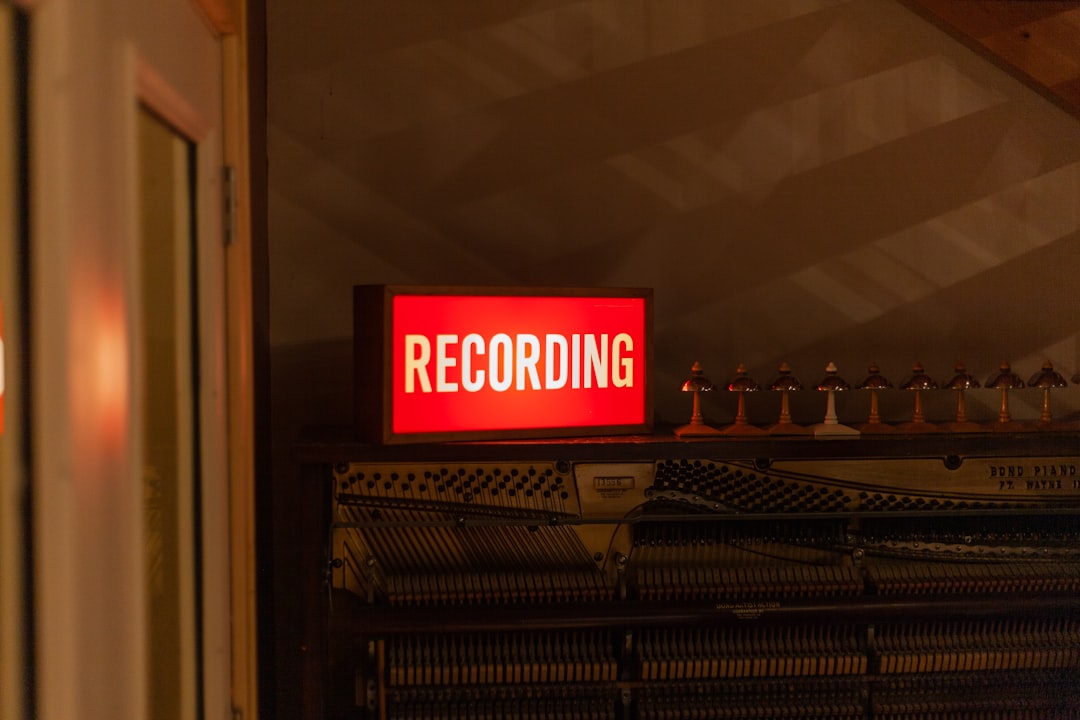
Successfully navigating the voice clone verification process is a key step in creating a high-quality Professional Voice Clone (PVC). Before you can fine-tune your model, you'll need to pass a voice Captcha, which acts as a safeguard against misuse of the technology. Failing this step multiple times will require contacting support for assistance. Keep in mind that fine-tuning is not instantaneous and can take several hours to complete, emphasizing the importance of patience and the complexities inherent in voice cloning technology. It's important to understand that the model may need additional training and could be re-entered into the queue for fine-tuning without user intervention. However, repeated failures during the fine-tuning stage will result in a "Failed" status, and unfortunately, the only option is to delete the failed attempt and restart the cloning process. These steps illustrate that voice cloning, despite its advancement, is a careful and deliberate procedure. Understanding the dynamics of the verification process and fine-tuning can prevent frustration and lead to a smoother and more rewarding experience for users aiming to leverage voice cloning for creative endeavors like audiobook production, podcasts, or even generating unique soundscapes.
1. **Vocal Fingerprint**: Each voice possesses a unique set of acoustic features, like the resonant frequencies that shape our vowel sounds. Accurately capturing and replicating these in a cloning model is crucial for producing a convincing artificial voice. Getting these nuances just right is part of the art and science of this technology.
2. **The Role of Silence**: It's not just about the spoken words; the silences and pauses within a dataset are equally important. They contribute to the natural flow of speech, impacting things like intonation and the timing of our utterances. A good clone needs to be able to reproduce these quiet moments as well as the spoken parts.
3. **Frequency Boundaries**: Human voices, generally, fall within a specific frequency range. Trying to incorporate frequencies outside this normal range in a cloning model can create unnatural and artificial sounds. It's a reminder that the goal is to capture and replicate the core features of human voice, not stray into artificially generated sonic landscapes.
4. **Recording Environment Matters**: The room's acoustics where recordings are made can drastically change the character of a voice. Untreated spaces might introduce unwanted echoes and colorations that can muddy the clarity of the voice. These distortions can cause problems when a model is trying to learn a voice's characteristics, creating a challenge in achieving clarity.
5. **Gender and Age**: Voice cloning algorithms have to be sensitive to the natural differences between voices based on factors like gender and age. The way a voice resonates and the sounds it produces shift, influencing the overall auditory impression of a speaker.
6. **Language and Dialect**: Languages and their dialects have unique sound systems and rhythm patterns. A model trained on a dataset from a specific language needs to capture these variations to sound genuine across different regional contexts. It's a detail-oriented process to capture the authenticity of a speaker.
7. **Emotional Nuances**: Expressing emotions through speech is complex. It's not just the words, but how we emphasize them and the variations in pitch and tone that give our voices emotional depth. Without enough emotional range in a training dataset, the resulting clone might sound robotic or lack the emotional expressiveness we associate with human communication.
8. **Sampling Rate Trade-offs**: The sampling rate used for recordings plays a key role. A common rate, like 44.1 kHz, captures a broad range of vocal frequencies, but higher rates, while offering more detail, necessitate greater storage and processing power, especially for substantial datasets. This highlights the need for a balance between data richness and computational requirements.
9. **Overlapping Speech and Noise**: Despite advancements, current voice cloning technology faces difficulties handling situations with overlapping voices or significant noise. These limitations can result in unnatural artifacts in the final output, sometimes impacting the clarity and intelligibility of the generated voice.
10. **Microphone Impact**: The microphone chosen for the recordings will affect the sound quality. Condenser microphones are often preferred for detailed voice capture, but dynamic microphones might be better for certain environments with lots of noise. This creates a balance between microphone capability and the recording environment which ultimately impacts the quality of the input dataset. These factors ultimately influence the final cloned voice.
As of October 2, 2024, the field of voice cloning continues to evolve, with researchers always looking for ways to improve the technology and address its potential downsides. It's important to remember that, while impressive, the technology isn't perfect yet and faces challenges like managing the nuances of human voices and preventing misuse.
Troubleshooting Common Voice Cloning Subscription Issues A User's Guide - Addressing Variability in Cloned Voice Output
Ensuring consistent and natural-sounding cloned voice output is a crucial aspect of voice cloning technology, especially when used for purposes like audiobook creation or podcast production. Continuous improvements in the algorithms are essential to boost the accuracy and consistency of cloned voices. The goal is for models to better replicate the intricate characteristics of human speech, including nuances in tone, intonation, and emotional expressions. A number of issues can contribute to variability in voice clones, including uneven audio quality in the training data, ambient noise, and a lack of diverse speech patterns. It's important that the dataset used to train the model contains a wide variety of phonetic elements and encompasses a broad range of emotional tones to generate more realistic voice clones. As the technology continues to advance, addressing these challenges and others inherent to voice cloning will contribute to more reliable and expressive synthetic voices.
Addressing the variability in cloned voice output remains a central challenge in voice cloning technology. Each human voice possesses a unique blend of pitch contours and vibrato, which contributes to individual personality and emotional expression. If a voice clone's training dataset doesn't capture these nuances, the generated voice can sound flat and lack that individualistic touch.
The range of sounds a language uses, its phonetic inventory, needs to be adequately represented within the training data. If certain sounds or sound combinations are missing, the model might struggle to accurately produce words with complex or unusual sound structures. This becomes especially critical in cases involving niche vocabulary or dialects.
Recent advancements in voice cloning allow models to analyze emotional contexts in speech. However, truly emulating human emotions in synthetic speech hinges on the emotional range present in the training data. It becomes imperative to include a wide spectrum of emotions to ensure the cloned voice can convincingly replicate a diverse emotional palette.
It's important to remember that speech isn't static. It's dynamic, with changes in tempo, rhythm, and other aspects that contribute to naturalness. These elements significantly impact how natural a cloned voice sounds, especially for narrative-focused applications like audiobooks where pacing and flow are vital.
The presence of background noise can create a sort of 'noise floor' that can interfere with the voice. This creates a challenge for the algorithm when trying to separate the desired vocal elements from the noise. As a result, the quality of the output can suffer, sometimes leading to a degraded, less-than-optimal cloned voice.
When a voice cloning task involves different dialects or accents, the dataset used to train the model needs to accurately represent those variations. If it lacks the essential dialectal details, the cloned voice might sound artificial or lose the authenticity that often links voices to a specific cultural context.
Voice cloning across languages introduces unique challenges, such as adjusting the pitch and tonal components that are characteristic of certain languages, particularly tonal languages. Ignoring these nuances can result in mispronounced words or unnatural-sounding patterns.
Some voice cloning systems use machine learning techniques that adapt and adjust their output in real-time. This capability enables the model to become more accurate over time. However, it also raises concerns regarding consistency, especially during the initial training phase of a voice clone.
There's also the issue of sampling artifacts, including aliasing and quantization noise. These artifacts can crop up if the sampling rate of the audio data is too low. These artifacts can result in distortions that may diminish the credibility of the generated voice, especially for professional applications.
Finally, the perception of a voice isn't solely based on its frequency; how a sound is perceived depends on elements such as the direction it seems to come from and its distance. More sophisticated systems can incorporate these spatial features to make the output sound more natural and realistic, particularly in immersive audio environments like 3D sound.
Despite the impressive progress, the technology continues to face challenges, including capturing and emulating the multifaceted nature of human voices and preventing any potential malicious applications of this fascinating technology. The future of voice cloning technology promises even more improvements.
Troubleshooting Common Voice Cloning Subscription Issues A User's Guide - Best Practices for Preparing Audio Samples
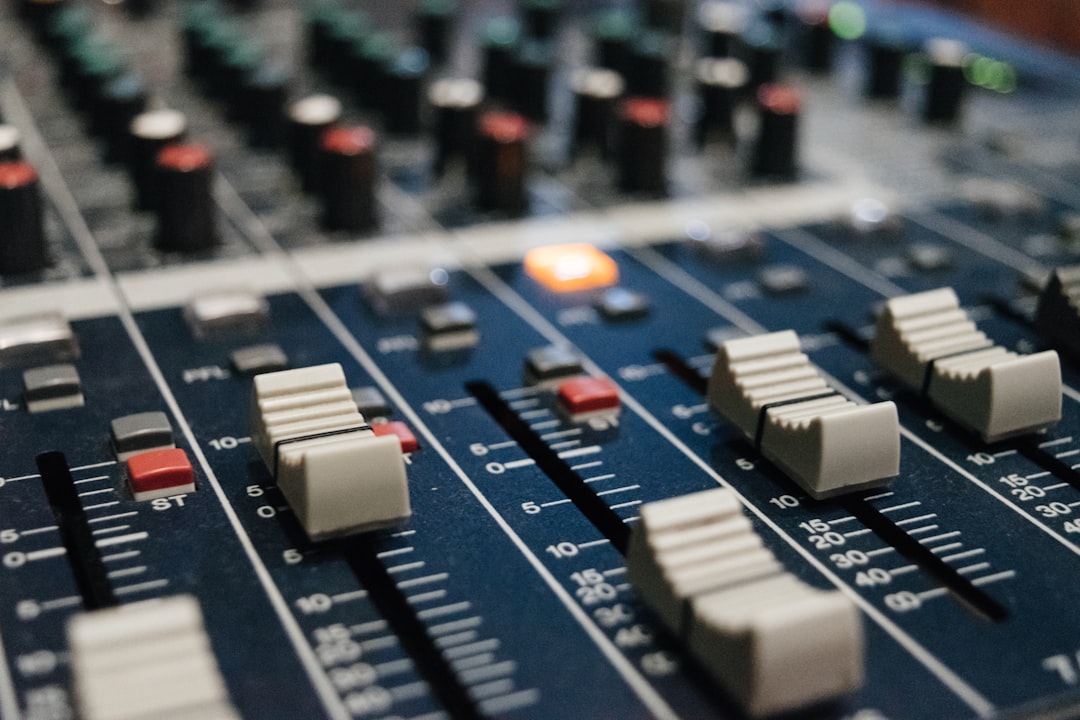
Creating effective voice clones hinges on the quality of the audio samples used to train the model. For the best results, stick to recordings with clear, mono audio. A standard sample rate of 22050 Hz works well, and the ideal format is WAV, as it preserves audio details without compression artifacts that can hinder the cloning process. It's also critical to ensure the audio is clean; background noises, music, and disruptions can confuse the AI and result in an inferior clone. The goal is to capture the speaker's voice in its purest form.
Depending on your goals, you'll need a certain amount of audio. For simple, casual voice cloning, you'll typically need at least 60 seconds of clear audio. However, if you're aiming for a more polished, professional voice clone, you'll likely need much more: potentially between 30 to 180 minutes of high-quality audio to give the model sufficient data to truly learn and accurately mimic a person's voice. By following these steps, users improve their odds of creating a successful voice clone that is natural and well-defined. This ultimately benefits a wide range of applications, such as enhancing audiobook production or enriching the experience of listening to podcasts. It's a subtle but important detail in the larger project of leveraging artificial intelligence to create new sonic environments.
The success of voice cloning hinges on the quality of the audio samples used to train the models. It's not just about having a lot of audio; it's about the characteristics of that audio. For instance, the dynamic range, the difference between the loudest and softest parts of the recording, is crucial. If the audio is excessively compressed, subtle details that give a voice its unique character can be lost, resulting in a clone that sounds less natural and more robotic.
Similarly, the range of sounds within the dataset is essential. A dataset lacking in phonemic diversity, a wide variety of consonants and vowels, can limit the model's capacity to recreate certain sounds. This becomes especially problematic for voice cloning projects involving specialized vocabularies or accents, making the cloning process less effective. The bit depth, which relates to the resolution of the recording, also plays a role. A higher bit depth offers a wider dynamic range, capturing more nuanced variations in the voice, leading to a cleaner cloning process.
Furthermore, the natural modulations in human speech—changes in pitch, tempo, and volume—are critical for creating convincingly natural voices. Voice cloning models benefit from datasets that include these variations, helping the models learn and replicate the rhythmic and expressive features of human communication. The recording environment can also heavily impact the dataset's quality. Background noise introduces unwanted artifacts that can interfere with a model's ability to isolate and learn the characteristics of a speaker's voice.
Understanding the temporal features of speech, particularly the rhythm and intonation patterns known as prosody, is key. Models trained on datasets with these elements are better equipped to produce clones that speak in a more natural manner. This is important for applications like audiobook creation where the pacing and natural rhythm are crucial. The room acoustics in which the recording takes place can affect the resulting sound through reflections and absorptions, which can distort the recorded audio. It's important to minimize these distortions for better audio sample quality.
Even a speaker's physical state, like fatigue, can affect a recording session. If samples are included where the speaker sounds tired, the resulting cloned voice can exhibit a similar lack of energy. Maintaining consistent vocal energy throughout the training data can result in a more engaging clone. And ensuring the training data incorporates a variety of speech tasks, such as reading, conversation, and emotionally charged speech, can lead to a more versatile clone. This variety helps the model better understand the different contexts in which human voices are used, ultimately improving the performance of the cloned voice in real-world applications.
Finally, natural human speech involves variations in speech rate. People talk faster or slower depending on the context or what they're feeling. Training a model with data that features this variation can result in a clone that's able to convey urgency or calmness. This enhances the overall emotional expression capability of the cloned voice. As of October 2nd, 2024, the ongoing work in the field of voice cloning focuses on addressing these factors to enhance the overall quality and realism of the cloned voices for a variety of applications, even with the inherent challenges of accurately mimicking the complexity of human communication.
Troubleshooting Common Voice Cloning Subscription Issues A User's Guide - Optimizing Voice Clone Training Parameters
Optimizing the settings used to train a voice clone is essential for achieving high-quality results. This starts with properly preparing the dataset, such as making sure your audio files are in mono format instead of stereo. Using stereo audio can increase file size unnecessarily and decrease the amount of useful data for training. It's also crucial to be mindful of the platform's data limitations; for instance, certain platforms like KitsAI have a 200 MB limit for training data.
Beyond managing dataset characteristics, adjusting the training process itself is critical. For example, fine-tuning the specific aspects of the training loop that generate spectrograms can lead to a substantial improvement in the model's performance. Additionally, implementing post-processing steps—using tools like VoiceFixer, for instance—can enhance the overall quality of the cloned voice. This is especially important for applications like audiobook production or podcast creation, where the clarity and expressiveness of the cloned voice are paramount to the listening experience.
When training models for voice cloning, optimizing the parameters is critical to achieving high-quality results. For example, the learning rate, a factor that controls how quickly the model adjusts its internal parameters, can be quite sensitive. Set it too high and the model might skip right over the ideal settings, leading to a poor voice clone. Conversely, if it's too low, training takes an extremely long time without necessarily improving much, wasting valuable time and resources.
The batch size, which refers to how many audio samples are processed at once, also plays a big role in model training. Smaller batches can create some helpful randomness, encouraging the model to be more adaptable to new voices. Larger batches, on the other hand, can stabilize the training process but might also make the model too focused on the training data, preventing it from generalizing well to new voices.
Expanding the training dataset with audio transformations, like adjusting pitch or speed, is a useful technique. This practice can be especially valuable for training models on voices with strong regional accents or emotional depth. It basically forces the model to learn more variability in how a voice can sound, making the resulting clone more versatile.
Recording samples at various volume levels is crucial for capturing how the speaker's voice naturally changes intensity. The goal is to capture the natural flow of speech dynamics, which is key to achieving natural-sounding speech. If the model isn't able to learn these changes, the output might sound artificial or robotic.
Human voices have unique acoustic characteristics, like formants that determine vowel sounds. Models need to capture these features accurately. Failing to do so results in clones that lack the typical richness and variety that give human speech its characteristic timbre.
Voice cloning models, often built on recurrent neural networks, rely on capturing the order of sounds in the training data. This sequential structure is crucial for natural-sounding speech. The size of the window used for looking back at previous sounds can have a profound impact on how well the model predicts the next sound. If the temporal window isn't carefully chosen, it can lead to a loss of natural speech flow.
Techniques like dropout, a regularization method, can improve generalization by preventing models from getting stuck in a "memorization" rut with the training dataset. Essentially, it helps the model focus on the underlying properties of human speech rather than simply regurgitating the training data.
The architecture of a model, particularly the number and organization of processing layers, also affects the ability of a model to accurately capture the voice's intricacies. A model with too few layers might struggle to learn complex patterns, whereas excessively deep models can become noisy and difficult to train effectively. This trade-off between complexity and efficiency is a consistent theme in the development of AI systems.
Leveraging pre-trained models can accelerate the training process and improve the final result, especially when training data is scarce. This concept, called transfer learning, uses the knowledge gleaned from models trained on much larger, more diverse datasets to get a head start on training.
Even after a model has been trained, some post-processing refinements can often greatly enhance the overall audio quality. Methods like equalization and dynamic range compression can help smooth out unwanted artifacts, and make the generated voice sound more human-like.
As of October 2nd, 2024, understanding the intricacies of these training parameters and the choices that influence the training process remains a core challenge in developing high-quality voice clones. It's an active area of research that continuously evolves, leading to exciting new capabilities for synthetic speech generation.
Troubleshooting Common Voice Cloning Subscription Issues A User's Guide - Troubleshooting Failed Professional Voice Clone Attempts
### Troubleshooting Failed Professional Voice Clone Attempts
Creating a high-quality professional voice clone can sometimes be a frustrating process. There are several reasons why a voice clone attempt might fail. One common issue occurs during the verification phase, where you're required to complete a series of checks to confirm the authenticity of the voice you're cloning. If you happen to fail all five of these checks, you'll need to contact support to reset your attempts and begin the cloning process anew.
Another situation involves a "Delayed" status. This means the system is automatically reprocessing the voice, putting it back in the queue for fine-tuning. No action is needed on your part during this automated step, and patience is key. However, if the fine-tuning process continues to fail, the voice clone status will transition to "Failed." At this point, your only option is to delete the failed attempt and restart the cloning process from the beginning.
The success of your voice clone depends heavily on several factors. Following the specific guidelines and procedures when submitting your audio is extremely important. It's crucial to ensure that your initial audio samples are high-quality and consistent, as this influences the ability of the model to accurately replicate your voice. By adhering to these requirements and exercising patience through the automated fine-tuning steps, you'll significantly increase the odds of a successful cloning outcome.
1. The unique characteristics of a person's voice, particularly how vowel sounds resonate, are crucial for a successful voice clone. These features vary across languages and dialects, making it important to capture them accurately in the initial recordings. Otherwise, the cloned voice might not fully resemble the original.
2. If a voice cloning model is trained on a limited or homogeneous set of audio, it can become overly specialized, leading to what we call overfitting. This can cause clones to sound too similar to the training voice or struggle to adapt to different speaking styles.
3. The timing of words and syllables (prosody) greatly impacts how natural a voice sounds. Cloned voices need diverse examples of natural rhythm and speech timing in the training data to accurately mimic these elements.
4. Languages have unique sounds (phonemes). A voice cloning model must learn all the sounds of a given language to generate believable clones. If some sounds are missing from the training data, the cloned voice may struggle with those sounds, creating awkward or unnatural speech.
5. Unwanted noises in the recording environment can significantly impact a clone's quality. Noise obscures the voice and can confuse the model during learning, resulting in inaccurate clones and poor emotional nuance.
6. The way a person articulates consonant sounds plays a vital role in voice clarity. These subtle articulations need to be precisely captured in the training data. Missing or inaccurately represented sounds can cause larger issues with the cloned voice's overall intelligibility.
7. Some advanced voice cloning techniques use adaptive learning, meaning the cloning process constantly adjusts the model's approach based on the data. This can lead to more natural-sounding cloned voices that better match the original speaker's voice.
8. Recording with low sampling rates can create artifacts like aliasing or quantization noise that harm the clarity of the audio. High-quality recordings are crucial for capturing the complexities of human speech, especially for professional applications like audiobooks.
9. When cloning voices that use different accents or dialects, the model's training data must include examples of these variations. Without them, the clone's speech can be inconsistent or nonsensical, making the cloning process significantly more complex, especially when dealing with narratives.
10. To capture the full spectrum of human emotional expression, the training data needs a wide range of emotions in the speaker's voice. Cloned voices without this diversity tend to default to a monotone delivery, resulting in a lack of the natural emotional expressiveness inherent in human communication.
Get amazing AI audio voiceovers made for long-form content such as podcasts, presentations and social media. (Get started for free)
More Posts from clonemyvoice.io: