Get amazing AI audio voiceovers made for long-form content such as podcasts, presentations and social media. (Get started for free)
How Voice AI Training Methods in 2024 Are Revolutionizing Podcast Production Workflows
How Voice AI Training Methods in 2024 Are Revolutionizing Podcast Production Workflows - Adobe Research Lab Launches Custom Voice Model Training With 5-Minute Sample Requirements
Adobe's research lab has unveiled a new system for training custom voice models, needing only a brief 5-minute audio sample. This development is poised to change how podcasts and other audio content are produced. The focus is on making audio production smoother by integrating more sophisticated AI tools. Adobe aims to enhance the capabilities of voice models, enabling quicker development of more personalized audio experiences, be it podcasts, audiobooks or other formats. Reducing the training time needed for these models marks a notable advancement, lowering hurdles for content creators. This clearly highlights the growing importance of AI in the audio creation realm in 2024. The broader trend is to see how AI-powered voice technology can boost both creativity and access to sound production. While this advancement might seem helpful, one must consider the implications of more readily available AI voice cloning tools and its potential impact on original content.
Adobe's research lab has unveiled a new approach to voice model training that's quite intriguing. It seems they've managed to reduce the necessary audio sample size for effective training to a mere 5 minutes. This is a significant departure from traditional voice cloning methods that often demanded extensive recordings.
The implications for projects like podcast and audiobook production could be substantial. Not only can voices be customized for specific projects with a minimal time commitment, but it appears the system is flexible enough to potentially handle various accents and languages. This could be particularly helpful for creating multilingual podcasts.
Another interesting point is the focus on capturing and recreating a wider range of emotional nuances within voice models. This capability could greatly enhance the richness and impact of spoken narratives, a crucial element in storytelling for podcasts and audiobooks. It seems the models can now adjust voice parameters on the fly, offering real-time customization for pitch, speed, and tone. This provides creators with a level of dynamic control we haven't seen before.
From a privacy standpoint, this development could be a step in the right direction. Since fewer samples are required, the volume of personal data involved in the voice cloning process is considerably reduced, potentially alleviating some privacy concerns. However, questions of accessibility arise. The ability to tailor audio output to suit individual listener preferences – particularly those with hearing difficulties – opens exciting possibilities.
The consistency of the trained voice across multiple episodes and projects is another key benefit. It ensures a more unified listening experience and helps preserve the identity of a podcast or brand. Additionally, the reduction in voice recording time can lead to substantial cost savings in production workflows, potentially making high-quality audio more widely accessible.
However, while the technological advancements are compelling, the ethical questions around voice cloning are only getting more complex. As we continue to enhance our capacity to reproduce human voices, the issues of consent, ownership, and the potential for misuse become increasingly vital. We need careful consideration of the policies around how this technology is deployed to prevent misuse and to ensure transparent handling of voice data.
It's fascinating to see how this technology might empower creative storytelling within podcasts and audiobooks. It allows for the development of more nuanced and varied characters while staying within the limitations of a smaller voice acting pool or even offering alternative ways to narrate stories. The ability to craft narratives with specific character voices, while potentially retaining the authentic timbre of the original voice actor, offers a fascinating creative frontier.
The implications of this technology in terms of audio production are undoubtedly far-reaching. It will be interesting to observe how this technology evolves, how it's adopted, and the various challenges and ethical questions that it raises as it continues to reshape the landscape of audio production.
How Voice AI Training Methods in 2024 Are Revolutionizing Podcast Production Workflows - Multitrack Recording Studios Replace Traditional Voice Actors With Neural Networks
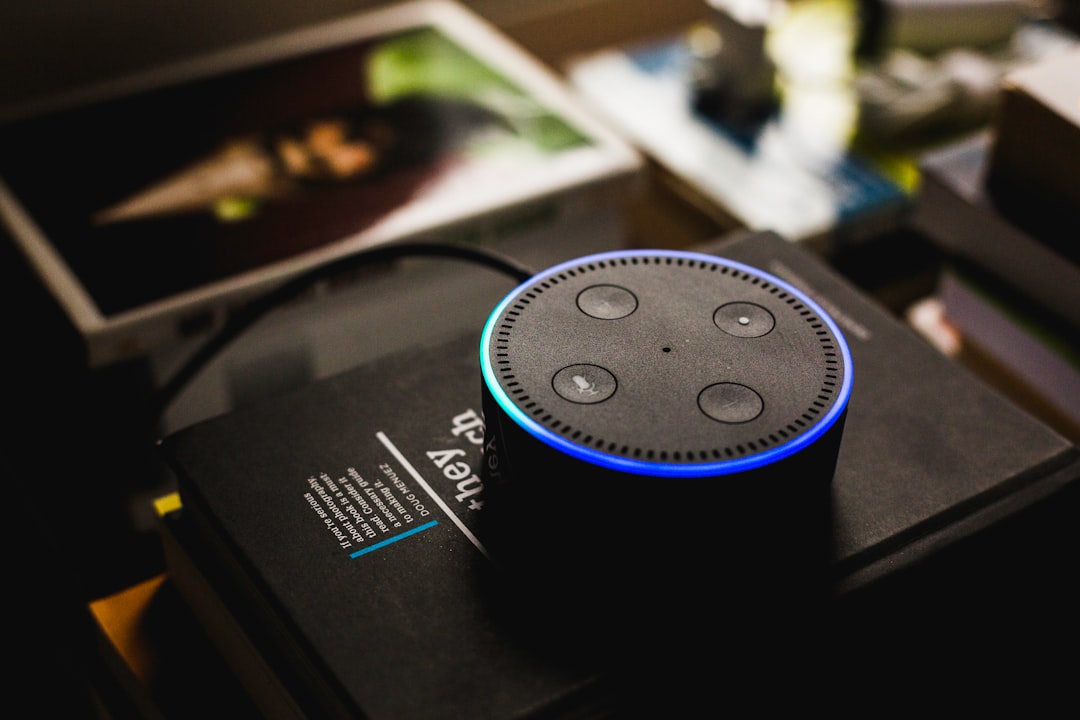
The integration of neural networks into multitrack recording studios is causing a significant disruption in the voice acting industry. Studios are increasingly utilizing AI-powered voice generation, leveraging the ability to create voices with distinct personalities and emotional depth. This shift has prompted many studios to explore AI as a potential replacement or a supplementary tool for human voice actors. The consequence of this trend is a growing sense of insecurity amongst voice actors. Many are now participating in recording sessions that essentially contribute data for the very AI systems that could eventually render their skills obsolete. This transition also raises challenging ethical considerations surrounding consent and ownership of voice data. As AI continues to reshape podcasting and audiobook production, the impact on narrative creation, character development, and audio workflows as a whole is likely to be substantial. This technological evolution forces a necessary reconsideration of the role of human voice talent in the future of audio content.
The integration of neural networks into multitrack recording studios is leading to a fascinating shift in how audio content is created, particularly for podcasts, audiobooks, and voice cloning applications. Sound engineers are now able to manipulate individual voice layers generated by AI models with unprecedented precision. This level of granular control allows for intricate edits and adjustments that would be practically impossible with traditional voice actors, paving the way for more complex and layered narratives.
One of the remarkable aspects of these neural networks is their ability to mimic subtle emotional cues within voice delivery. They can adapt their tone and inflection based on the context of the script, enhancing the storytelling process. Humor, tension, and sadness can now be conveyed with a nuanced realism, achieving a deeper emotional connection with listeners. This capability is particularly valuable for audiobook narration and immersive podcast experiences.
Another benefit is the sheer speed at which AI voice cloning can generate a variety of vocal options. This process is significantly faster than traditional methods involving human voice actors, streamlining the production timeline and allowing for quick adaptation to changing project needs. Producers can experiment with various accents and dialects, catering to diverse audiences. This is particularly appealing for creating podcasts that resonate with a wider spectrum of listeners, expanding into new markets with more authenticity.
The AI's capacity extends beyond basic voice production. It incorporates advanced audio restoration capabilities, effectively cleaning up imperfections or background noise. This is extremely useful for achieving professional-grade sound quality even with recordings made using synthetic voices. The AI can analyze and automatically enhance audio, minimizing the need for extensive manual edits.
Beyond post-production, we are also seeing AI-powered real-time manipulation of voice attributes. Producers can modify pitch, speed, and tone during the recording process itself, allowing for instant feedback and creative adjustments on the fly. This can lead to more dynamic and interactive experiences, ideal for live podcast formats or even interactive storytelling applications.
One major advantage of this technology is the consistency it brings to serialized content like podcasts. By using the same AI-generated voice model across multiple episodes, creators can achieve a uniform and recognizable auditory identity. This consistent sonic signature solidifies brand identity and contributes to a more unified listening experience for the audience.
Collaboration also becomes much simpler with AI-powered voice production. Voice models can be easily shared and modified through cloud-based platforms, enabling remote teams to seamlessly work together regardless of physical location. This streamlined workflow cuts down on traditional project timelines and encourages greater efficiency.
Furthermore, AI voice technology simplifies the process of incorporating voiceovers into projects. Gone are the days of extensive casting calls and actor scheduling. Producers can quickly deploy a range of voice options tailored specifically for the project, unlocking creative freedom without the usual logistical headaches.
However, these advancements also introduce some unsettling ethical dilemmas. The capacity to perfectly replicate any individual's voice raises a multitude of questions about consent and ownership. As AI-generated voices become increasingly commonplace, a robust regulatory framework becomes essential. Clear guidelines and policies are needed to protect against potential misuse and ensure that the technology is used responsibly.
It's fascinating to see the creative potential unleashed by this technology. Yet, it's equally important to acknowledge and address the complex ethical landscape it presents. As this technology continues to reshape the audio production industry, careful navigation of these ethical considerations will be crucial to its long-term viability and responsible integration into our world.
How Voice AI Training Methods in 2024 Are Revolutionizing Podcast Production Workflows - Podcast Editing Software Integrates Real-Time Voice Separation Technology
Podcast editing software is integrating real-time voice separation technology, a development that's significantly improving audio quality. This means that software can now isolate individual voices from surrounding noise and music, leading to a cleaner, more focused sound for listeners. This feature is a welcome addition for podcasters, as it tackles a common challenge in audio production.
The integration of AI into the editing process is also simplifying workflows. Tasks once requiring considerable manual effort, such as enhancing audio clarity and removing unwanted filler words, are now being handled by automated systems. This shift frees up podcasters to concentrate more on content creation and less on technical editing. Furthermore, this technological evolution is changing the way podcasting projects are managed. It's now easier for multiple individuals to collaborate on editing, offering tools for real-time feedback and shared cloud-based projects. This has led to faster editing and approval processes.
The benefits of these AI-powered editing features are undeniable, but it's important to consider the ethical implications of these advancements. As technology allows for increasingly sophisticated voice manipulation and cloning, it raises concerns about authenticity and the potential for misuse. Striking a balance between using these technologies effectively and respecting the originality of audio content is crucial for responsible audio production in the future.
Podcast editing software has become significantly more sophisticated in 2024, incorporating real-time voice separation technology. This capability enables audio engineers to isolate individual voices from background noise and music during the recording process itself, rather than relying on post-production techniques. This dynamic approach not only simplifies workflow but also opens up new creative possibilities. The ability to finely tune individual voice tracks in real-time offers greater flexibility and responsiveness during recording sessions.
One of the noticeable improvements is the advancement of noise reduction algorithms within these voice separation tools. They are becoming increasingly adept at filtering out unwanted background sounds, ensuring that recordings remain clear and crisp even in less than ideal environments. While this is a welcome development, it's important to consider that these algorithms aren't perfect and may sometimes introduce artifacts or inadvertently alter desired sounds.
Furthermore, voice models are starting to demonstrate a nascent ability to understand emotional context. They are able to adjust their tone, pitch, and pacing in response to emotional cues within the content. While still in its early stages, this capability could potentially lead to more human-like, engaging narrations in podcasts and audiobooks. This is an area that's worth paying close attention to, as its potential for improving emotional connection within audio content is substantial.
The integration of voice separation also opens up opportunities for multilingual podcasts. It's now becoming easier to combine recordings in different languages or dialects without the usual post-production challenges. This could lead to a more globally accessible podcasting landscape, catering to a wider range of listeners and fostering cross-cultural exchanges.
The real-time nature of these tools has significantly impacted editing workflows. Engineers can expedite the editing process by addressing audio issues immediately, instead of laboring through hours of post-production edits. This translates into a quicker turnaround time and a greater capacity for producing complex audio projects without a massive increase in production time. This shift could fundamentally change how audio productions are structured, particularly for podcasts with many contributors or complex sound design elements.
Dialogue editing has become much more precise as well. We can fine-tune the placement of voices in a soundscape, seamlessly adjusting how dialogue interacts with other audio elements, ultimately leading to a richer auditory experience.
The introduction of cloud-based platforms has enabled collaborative editing for remote teams. Multiple contributors can work on audio projects concurrently, seamlessly adjusting voice tracks in real-time. This development drastically shortens traditional production timelines, promoting a more agile and efficient way of creating audio content.
These advancements are also instrumental in promoting consistency. Podcasters can employ AI-generated voices that sound similar across episodes, thereby reinforcing brand identity and helping build a loyal audience. This consistency is vital for the long-term success of a podcast, fostering familiarity and recognition amongst listeners.
We're also witnessing an increase in the use of data-driven customization. By leveraging real-time data about listener preferences, producers can refine their audio content to align with audience expectations. This allows for a more iterative approach to audio production, with ongoing refinement based on user feedback and listening habits.
However, these rapid advancements in voice technology are raising serious questions about ethical considerations. The capacity to clone and manipulate voices with greater precision necessitates careful examination of existing intellectual property laws and the development of guidelines for the responsible use of this technology. The challenges of consent and ownership in audio content become more pronounced with the advent of these advanced editing tools. A robust framework is needed to address these concerns, ensuring that the rights of content creators and individuals are protected.
It's clear that podcast editing and sound production are being fundamentally reshaped by these advancements in voice separation and related AI technologies. While the possibilities are exciting, it is crucial to keep an eye on the ethical implications as these technologies become increasingly widespread.
How Voice AI Training Methods in 2024 Are Revolutionizing Podcast Production Workflows - Voice Cloning Platforms Enable Cross-Language Podcast Adaptations
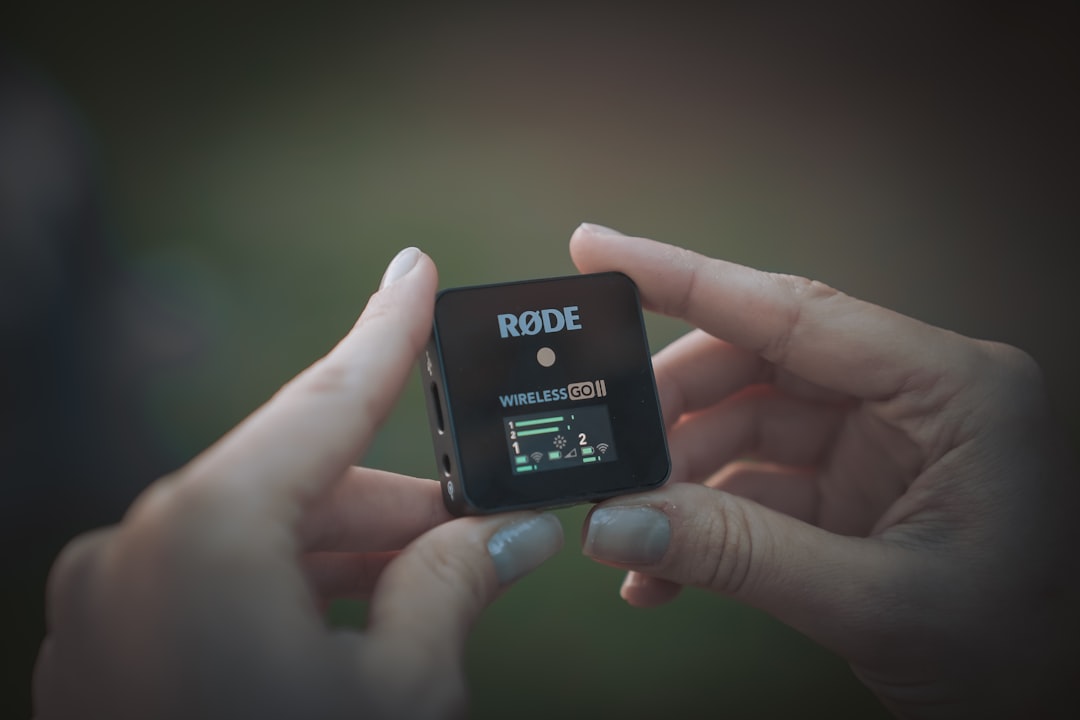
Voice cloning technology is enabling podcasters to create versions of their shows in multiple languages. This means a podcast originally in English could be easily adapted into Spanish, French, or any other language, while still sounding like the original host. This potential for broader reach and audience engagement is exciting, but it also raises complex ethical concerns. By using AI to replicate a voice, creators can maintain the distinctive qualities of their podcast, including the speaker's unique vocal tone and emotional delivery. It allows for greater inclusivity as content becomes accessible to people who don't speak the original language. The speed and efficiency of this process also open up opportunities to explore new markets and audiences quickly.
However, these innovative tools also bring with them questions about the appropriate use of voice replication technology. It's important to consider the ethical implications of cloning someone's voice without their consent. As voice cloning becomes more sophisticated, it's necessary to develop responsible guidelines for its use. The future of podcasting with voice cloning will depend on striking a balance between the creative possibilities and ethical considerations, and finding ways to safeguard against potential misuse.
Voice cloning platforms are increasingly becoming crucial tools in the world of podcasting, particularly for achieving cross-language adaptations. It's quite remarkable how these platforms can not only replicate a speaker's voice but also seamlessly translate it into different languages while maintaining a natural sound. This opens exciting avenues for podcasters, allowing them to reach audiences across the globe without needing to hire a large team of multilingual voice actors.
Furthermore, the incorporation of emotional detection within voice cloning systems is adding a layer of sophistication to audio production. These AI-powered systems can now analyze the context of a dialogue and adapt the voice's delivery accordingly. This allows for a more nuanced and emotionally rich listening experience, especially crucial for narrative-driven podcasts and audiobooks.
Creating distinct voice profiles with these systems has also become remarkably streamlined. It appears that just a short recording sample – a few minutes, perhaps – is enough for these systems to develop a highly customized voice profile. This not only empowers podcasters to retain their own vocal identity but also creates new avenues for crafting unique voices for fictional characters in podcasts and audiobooks, offering more freedom in storytelling.
Another intriguing aspect is the speed at which these systems can generate audio content. It seems that rapid prototyping and testing of audio segments is now a reality. This faster workflow can lead to more iterative design processes, allowing creators to explore various creative avenues and refine their work based on quick feedback loops, a significant shift from traditional production methods that often involved extended and expensive recording sessions.
These systems are not merely voice generators but also integrate sophisticated audio processing techniques. Many platforms now feature tools like automated noise cancellation and echo reduction, essentially cleaning up the audio output. This allows podcasters to achieve high-quality audio even if their recording conditions aren't ideal.
A number of platforms also offer the possibility of real-time voice manipulation during the recording process itself. This means that aspects like pitch and speed can be adjusted on the fly. This creates more interactive and dynamic recording environments, which might lead to more experimental approaches to podcasting, potentially leading to more engaging and immersive listening experiences.
These advancements have significantly lowered the barrier to entry for independent podcast creators. Anyone with access to a recording device and basic software can create professional-sounding audio. This democratization of audio production could help more creators share their stories, leading to more diverse content and broadening the range of voices in the podcast landscape.
Moreover, the ability to capture the nuances of various accents and dialects within voice cloning is increasingly refined. This is crucial for podcasters who want to connect with specific cultural communities or produce content that resonates with particular regional audiences.
Collaboration on audio projects has also become considerably smoother. Many platforms facilitate real-time collaborations on voice projects among multiple users, even across geographical boundaries. This fosters more cohesive and efficient audio workflows.
Lastly, we're beginning to see the impact of data-driven voice cloning techniques on podcasting. Some systems can analyze listener preferences and adapt the content in real-time, making it more personalized and engaging. This is a nascent area, but it could potentially lead to highly customized audio experiences and increased listener retention.
However, while the potential benefits are substantial, we must also acknowledge the inherent ethical questions that arise with these powerful technologies. The ease with which voices can now be replicated raises concerns about the potential for misuse, particularly in areas like impersonation or the creation of fake audio content. It's essential that guidelines and regulations are established for the responsible use of voice cloning technologies to prevent potential harm.
It will be interesting to see how these platforms continue to evolve and impact the podcasting landscape. The potential for creativity, innovation, and accessibility seems substantial, but we must proceed with caution, ensuring that this technology is used responsibly and ethically. The future of audio creation is likely to be dramatically shaped by AI, and it's a future that requires careful consideration and navigation.
How Voice AI Training Methods in 2024 Are Revolutionizing Podcast Production Workflows - Text to Speech Models Learn Regional Accents Through Pattern Recognition
Modern text-to-speech (TTS) systems are increasingly adept at replicating regional accents. They achieve this by employing sophisticated pattern recognition methods. These AI models are trained on vast amounts of audio data, allowing them to learn the unique characteristics of different dialects. This newfound ability to mimic the subtle inflections and pronunciations of various regional accents enhances the realism of synthetic voices, proving particularly useful in podcast production.
The desire for more authentic audio experiences, which include a wider range of regional accents, has led to this development. However, achieving this accuracy requires extensive datasets encompassing a wide variety of accents. The need for such large datasets raises concerns about the cost and potential for biases in the training process. There's a risk that underrepresented or less common accents might not be adequately represented, leading to skewed results and potentially perpetuating existing inequalities in voice AI applications.
Striking a balance between improving the technology's accuracy in capturing different regional nuances and avoiding bias is a crucial challenge. As voice AI technologies advance, it's vital that developers consider these implications to ensure fair and accurate representation of diverse accents in all areas where synthetic voices are employed.
The field of text-to-speech is seeing exciting developments in its ability to capture and reproduce regional accents. These models rely on massive datasets of spoken language, allowing them to pick up on the unique patterns of sound, rhythm, and intonation that define different dialects. This means we're getting closer to synthesizing voices that sound genuinely local and can better connect with specific audiences.
One fascinating aspect is how these models are now learning to incorporate emotional nuances into their speech. By analyzing things like stress and intonation, they can adapt their tone to reflect different emotions, making synthesized voices feel more natural and expressive. This is particularly interesting for applications like audiobooks and podcasts where conveying emotion is essential.
Some researchers are even exploring multi-modal approaches, combining visual information like lip movements with audio data to train models. The idea is that by linking visual cues with sound, they can achieve a more accurate understanding of how accents are produced, resulting in even more realistic synthesized speech.
It's also worth noting that these models are incredibly adaptive. They can quickly learn from errors and feedback. For example, if a user corrects a mispronounced word, the model can adjust its output without needing a complete retraining process. This rapid iteration is key to refining these models and achieving increasingly natural-sounding results.
The ability to replicate accents also allows these technologies to explore cross-language applications. If a model has been trained on, say, a British English accent, it might be able to apply some of the learned phonetic patterns to produce a French version of that voice while retaining some of its characteristic traits.
Even the subtle differences between, for instance, American and Australian English can be learned and reproduced by these models. This is a huge benefit for content creators who want to tailor their work to specific audiences.
Another interesting feature is their increasing resilience to background noise. These models are getting better at filtering out unwanted sounds, ensuring clear synthesized speech even in environments with distractions. This is highly advantageous for podcast recordings and other audio production scenarios.
While all this sounds incredibly exciting, it's essential to acknowledge the ethical implications. Replicating regional accents can sometimes be seen as a form of cultural appropriation if it's done without a proper understanding of the context and potential impact. It's a reminder that while technology can be remarkable, it's important to use it thoughtfully and responsibly.
One of the more recent developments is the emergence of generative voice design. This means voice cloning systems can now combine features from different regional accents to create completely new and unique voices, completely divorced from any existing individuals. This expands the creative possibilities for content creators.
Finally, some advanced voice synthesis systems are integrating real-time adaptation capabilities. This means the model can adjust accent characteristics on the fly based on speaker emphasis or even audience reactions. It's a compelling example of how we might see even more interactive and responsive audio experiences in the future, including in live settings.
It's fascinating to see how these technologies are rapidly advancing, offering an ever-widening array of possibilities. However, it's important to be mindful of the potential downsides and approach these innovations with a critical and thoughtful lens.
How Voice AI Training Methods in 2024 Are Revolutionizing Podcast Production Workflows - Audio Production Teams Cut Recording Time Using Voice Banking Libraries
Audio production, encompassing podcasts, audiobooks, and voice cloning, is experiencing a shift due to the rise of voice banking libraries. These libraries, coupled with AI-powered audio tools, are helping teams significantly reduce recording time. Creating unique and believable voiceovers and narrations has become a much quicker process, streamlining the overall workflow and likely impacting the cost of production. Voice cloning technology not only helps accelerate audio production, but also allows voice actors to potentially explore alternative ways to profit from their skills via passive income options. This new efficiency in sound production comes with a responsibility to address issues surrounding consent and data ownership as it becomes easier to replicate voices digitally. The ability to clone a voice raises a number of ethical questions, some we have not even begun to address. The development of these technologies represents a fascinating crossroads between innovation and responsibility, and will be a major driver of how audio production evolves in the future.
Audio production teams are finding innovative ways to streamline their workflows, with voice banking libraries emerging as a key player. These libraries, essentially collections of pre-recorded voice samples, significantly reduce the time needed for recording sessions. Instead of requiring a voice actor's presence for every nuance or edit, producers can now pull from these libraries, making audio content creation more agile and responsive.
This isn't just about speed; there's a growing capability for these libraries to capture a wider spectrum of emotional nuance. AI-powered tools within these systems are becoming better at recognizing and replicating emotions like happiness or sadness in the synthetic voice. This enhanced expressiveness significantly elevates the storytelling aspect of podcasts and audiobooks, fostering a deeper connection with listeners.
The possibilities for multilingual content have also seen a considerable leap. Podcast producers can now adapt their content into various languages using the same underlying voice model, ensuring a consistent listening experience across different language versions. This makes it easier to reach wider audiences, potentially unlocking global markets that were once challenging to penetrate.
We're also seeing a greater level of real-time control over the audio itself. Producers now have the ability to dynamically modify pitch, speed, and vocal tone during the recording process, much like a musician adjusts an instrument in a live performance. This allows for a more interactive and intuitive approach to audio creation, offering increased flexibility and responsiveness.
Interestingly, the amount of data needed to train these voice models has been dramatically reduced. Where previously extensive recordings were necessary, now just a few minutes of audio can be sufficient to create a distinctive voice profile. This reduction in data demands is particularly significant for smaller production teams or independent creators who may not have access to large-scale recording resources.
This approach also promotes greater consistency across different audio projects, especially when it comes to portraying distinct characters. For instance, audiobook narrators who have to embody a wide range of characters can leverage these libraries to maintain character consistency throughout a narrative without losing the individual character's voice qualities.
However, there's a growing need to address the potential for biases in these libraries. Recognizing this, developers are actively working to use more diverse datasets encompassing a wider range of accents and dialects. The goal is to generate voices that authentically reflect the rich variety of human expression across various cultural backgrounds.
The use of voice banking libraries also impacts post-production workflows, simplifying certain tasks. Routine processes like removing background noise or evening out audio levels can be automated, saving creators a substantial amount of time and effort. This translates to faster turnaround times and increased efficiency, allowing producers to focus on the creative aspects of their work.
Naturally, the proliferation of these libraries raises some important ethical concerns about consent and ownership of voice data. It's critical that we establish guidelines and best practices to ensure that voice samples are collected and used responsibly and ethically, preserving trust and protecting individual rights.
Finally, some systems are exploring the use of listener feedback to further refine the AI-generated voices. This feedback can inform adjustments to voice attributes or the style of delivery, leading to a more personalized listening experience. Although still in its early stages, this capability suggests a future where podcasts are increasingly customized to individual tastes and preferences.
The use of voice banking libraries and related technologies is undeniably impacting how audio content is produced. As this field continues to evolve, it's important to approach these advancements with a balanced perspective, recognizing both the potential benefits and the ethical considerations that need careful navigation.
Get amazing AI audio voiceovers made for long-form content such as podcasts, presentations and social media. (Get started for free)
More Posts from clonemyvoice.io: