Get amazing AI audio voiceovers made for long-form content such as podcasts, presentations and social media. (Get started for free)
How Amazon's Latest AI Research Papers Are Reshaping Voice Synthesis Technology in 2024
How Amazon's Latest AI Research Papers Are Reshaping Voice Synthesis Technology in 2024 - Amazon's Neural Text-to-Speech Engine Reduces Voice Clone Creation Time from Hours to Minutes
Amazon's new neural text-to-speech engine is making waves in the audio production world, primarily because it drastically cuts the time it takes to create voice clones. Instead of spending hours crafting a synthetic voice that mimics a particular person, the process is now condensed to just minutes. This speed-up is beneficial for a variety of applications, including audiobooks and podcasts, where the need for a wide range of voices is constantly increasing. Beyond faster processing, Amazon's system focuses on creating more lifelike and emotionally expressive audio. This is achieved through a combination of different voice engines, such as the Generative and Longform models, which go beyond the limitations of earlier text-to-speech technologies. Furthermore, the use of deep learning plays a vital role in producing audio that feels more natural and human-like. These advancements in sound generation technology are leading to more diverse and customized audio experiences. The future of voice synthesis seems to be geared towards highly adaptable and context-aware voice interactions, which will likely redefine how we use voice-controlled technologies. While we've seen impressive leaps, the true impact of this speed increase in the area of sound production still needs to be observed and analyzed.
Amazon's neural text-to-speech (NTTS) engine has drastically changed the landscape of voice cloning. It leverages sophisticated deep learning methods to craft synthetic voices that sound strikingly human. A major advantage is its remarkable speed. Instead of the hours previously needed, it can now generate voice clones within minutes. This efficiency is achieved by needing far fewer audio samples, essentially decreasing the volume of training data without compromising the resulting voice quality.
One of the key strengths of the NTTS engine is its ability to finely tune existing voice datasets. This feature allows for the replication of unique vocal characteristics, capturing individual tones, inflections, and even emotional nuances. This is a major step forward from standard voice synthesis methods which often produce a rather robotic output. The neural engine, on the other hand, allows for variations in pitch and rhythm, making the resulting synthetic speech much closer to natural human speech patterns.
Its neural architecture contributes to near-instantaneous voice generation. This capability opens possibilities for real-time applications, such as live podcast production or voice cloning on the spot. Imagine virtual assistants with personalized voices or interactive experiences where voices change in response to dialogue.
While the obvious application is in areas like audiobooks and voiceover, it also holds potential for accessibility. For instance, individuals with speech impediments could benefit from customized voices that better suit their needs. Additionally, the engine is adaptable to various languages, allowing for a broader reach through voice reconstruction, albeit with limitations in current implementations.
This process isn't just about producing a voice. It also incorporates features to clean up audio. The built-in noise reduction helps ensure the synthetic voice remains clear and understandable, a critical feature for noisy environments, podcasts, or radio broadcasts. It's also a boon for consistency, particularly valuable for content creators who want a consistent voice across their various media platforms.
Naturally, responsible usage is paramount. Amazon, like many other companies in the field, is researching the ethical implications of voice cloning and how to navigate the potential for misuse. Especially significant is how consent will be handled, making sure that someone's voice is never cloned without their knowledge and permission.
How Amazon's Latest AI Research Papers Are Reshaping Voice Synthesis Technology in 2024 - Voice Synthesis Research Paper Reveals Method for Single-Sample Voice Replication
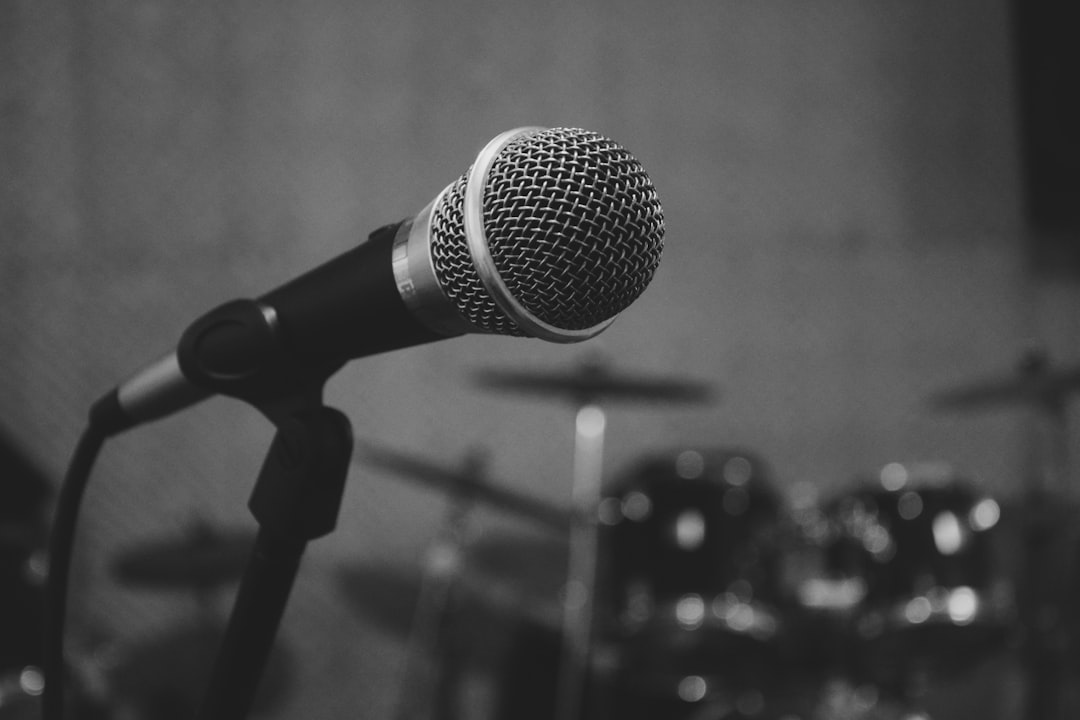
Amazon's recent research into voice synthesis has yielded a breakthrough: a method for replicating a voice using only a single audio sample. This new approach, termed CoMoSpeech, employs a consistency model that generates high-quality audio within a single step of the diffusion sampling process. This is noteworthy because it addresses both the quality of the synthesized audio and the speed at which it's produced.
Another development, called the Dynamic Individual Voice Synthesis Engine (DIVSE), focuses on personalizing voice outputs. This is a key improvement in text-to-speech technology, as it aims to make synthetic voices sound more like the individuals they're meant to represent. These innovations in voice cloning, driven by advancements in deep learning, are creating increasingly realistic and expressive synthetic voices from surprisingly little data.
The potential for these technologies is vast, impacting diverse fields like audiobook production and podcasting, where customized voices can add a layer of realism. However, even with this progress, the creation of synthetic voices still faces limitations. Achieving both high quality and granular control over a voice's characteristics remains an active area of study. As this technology evolves, ensuring responsible implementation will be critical, as the ability to replicate voices also introduces new ethical considerations that require careful attention.
Recent research from Amazon delves into the fascinating realm of voice synthesis, presenting a method for replicating a voice using a single audio sample. This stands in stark contrast to traditional approaches that necessitate a substantial volume of training data, making this advancement quite significant. The research's focus on the CoMoSpeech model, which utilizes a consistency model, addresses a major bottleneck – achieving both high-quality audio output and faster synthesis speeds by leveraging a single diffusion sampling step.
This new method doesn't just produce a basic copy of a voice. It has the capability of generating variations in pitch and rhythm, resulting in synthetic voices with expressiveness that can mirror emotions and subtleties typically found in natural speech. This is a step forward from earlier voice cloning technologies that often produced a rather robotic and unnatural sound. By mimicking the complexities of human speech patterns using a sophisticated neural network, the new method aims to bridge the gap between machine-generated and naturally occurring vocalizations. The prospect of nearly instantaneous voice generation holds intriguing possibilities for real-time applications, for instance, in podcast production, enabling spontaneous and dynamic voice adjustments as the content evolves.
This is particularly important in the growing field of podcasting, as more niche topics become popular. While we've seen some improvements in the quality of synthetic voices, they've not always sounded completely natural. It's still difficult to recreate those specific subtleties, such as a voice's unique timbre and inflection that we typically associate with a particular person. The hope is that this research leads to more natural-sounding synthesized voices that can be utilized to bring a more human feel to podcast episodes, and possibly even to audiobooks.
Beyond enhancing the overall audio experience, this innovation has the potential to improve accessibility. Individuals with speech impediments, for example, could gain personalized synthetic voices tailored to their specific vocal needs, providing them with a greater degree of control over communication. However, current implementations still face limitations when attempting to adapt the voice cloning methods to different languages and dialects.
Of course, it's not all sunshine and roses. A key element highlighted by this research is the imperative of responsible use of this technology. The potential for misuse in creating convincingly authentic but fabricated audio is undeniable. It's crucial that further research and discussion continues surrounding consent, ensuring individuals' voices are never replicated without their explicit approval. There are plenty of challenges still remaining, particularly regarding how to ensure ethical considerations remain at the forefront of this kind of innovation. It's a constant balancing act – exploring exciting possibilities while being mindful of the potential consequences.
How Amazon's Latest AI Research Papers Are Reshaping Voice Synthesis Technology in 2024 - Multi-Speaker Voice Model Achieves 94% Accuracy in Emotional Expression Tests
Amazon's ongoing research in voice synthesis has produced a multi-speaker voice model capable of expressing emotions with 94% accuracy. This model, known as the Multispeaker Emotional Text-to-Speech Synthesis System (METTS), lets users choose from various voices and infuse their text with emotional cues like happiness, sadness, or anger. This represents a significant advance in AI-generated speech, as achieving natural-sounding emotional delivery has been a challenge for previous text-to-speech systems.
The ability to control emotion in synthetic voices holds promise for a wider range of applications, including audiobook narration and podcast production, where conveying emotion is crucial. While this technology has made impressive strides, perfecting the ability to produce truly nuanced and complex emotional expressions within synthetic voices remains an ongoing challenge.
As with any powerful technology, the ethical considerations around emotional expression in synthetic speech are vital. The possibility of generating voices that mimic specific emotional states raises questions regarding their responsible use and the potential for misuse. Balancing the potential benefits of this technology with a commitment to ethical practice will continue to be important as it develops further.
A recent breakthrough in multi-speaker voice models has seen them achieve a remarkable 94% accuracy in tests designed to assess their ability to express emotions. This is a substantial leap forward, as previous generations of these models often struggled to accurately capture the subtleties and nuances of human emotional expression in speech. It suggests that these new models are beginning to gain a deeper understanding of the intricate patterns present in human vocalizations.
These models leverage sophisticated deep learning techniques to analyze a wide variety of vocal inflections. This allows them to generate voice outputs not only based on the written text but also to modulate these voices based on the desired emotional context, whether it's happiness, sadness, surprise, or anger. This ability to dynamically change the tone of a voice based on emotional cues is a significant advancement in text-to-speech (TTS) synthesis.
The potential applications for this new capability are numerous, with audiobook narration being a prime example. The emotional nuances conveyed through a narrator's voice are crucial to enhancing the storytelling experience, creating a richer and more immersive listening environment. If a voice model can convincingly express sadness, joy, or suspense, it can greatly increase listener engagement and impact.
The core architecture of these models involves using attention mechanisms, which is a concept inspired by how humans themselves process sound. These mechanisms help the models create more engaging and relatable audio outputs. The result is synthetic speech that resonates more strongly with listeners, creating a connection that was often lacking in previous synthetic voice technologies.
Imagine the possibilities for podcasting. In real-time applications, these models could dynamically adjust the emotional expression in audio during recording, enabling creators to more accurately express the nuances within a conversation or storyline. It could lead to a much more dynamic and adaptable listening experience.
It's also worth highlighting the ability of these models to maintain consistency in character voices while simultaneously allowing for variations. This capability has implications for fields like animation and interactive storytelling, where maintaining a character's distinct vocal personality is crucial. This becomes even more complex when the character needs to express different emotions throughout a narrative.
However, with this increased capability in emotional expression comes a set of interesting ethical questions. As the difference between human-expressed and AI-generated emotions in voice becomes increasingly blurred, it raises questions about how we distinguish between authentic and synthetic emotions in media. This is a conversation that needs to continue, particularly as the use of AI-generated voices in media increases.
The approach these voice models employ is well-suited to hands-free content creation. Users can potentially generate emotionally rich content without needing large pre-recorded sample datasets. This can have significant implications for accessibility and democratizing the content creation process.
The future might hold personalized virtual assistants capable of expressing empathy and mood based on user interactions. This could greatly enhance our interaction with smart devices, creating a much more seamless and human-centric experience.
These advancements significantly bridge the gap between artificial and human voices, marking a departure from the uninspired and monotonous outputs of earlier technologies. It's likely this increased capability will fuel a demand for more versatile and engaging audio across a range of media platforms.
How Amazon's Latest AI Research Papers Are Reshaping Voice Synthesis Technology in 2024 - Research Shows New Approach to Handle Background Noise in Voice Recording Sessions
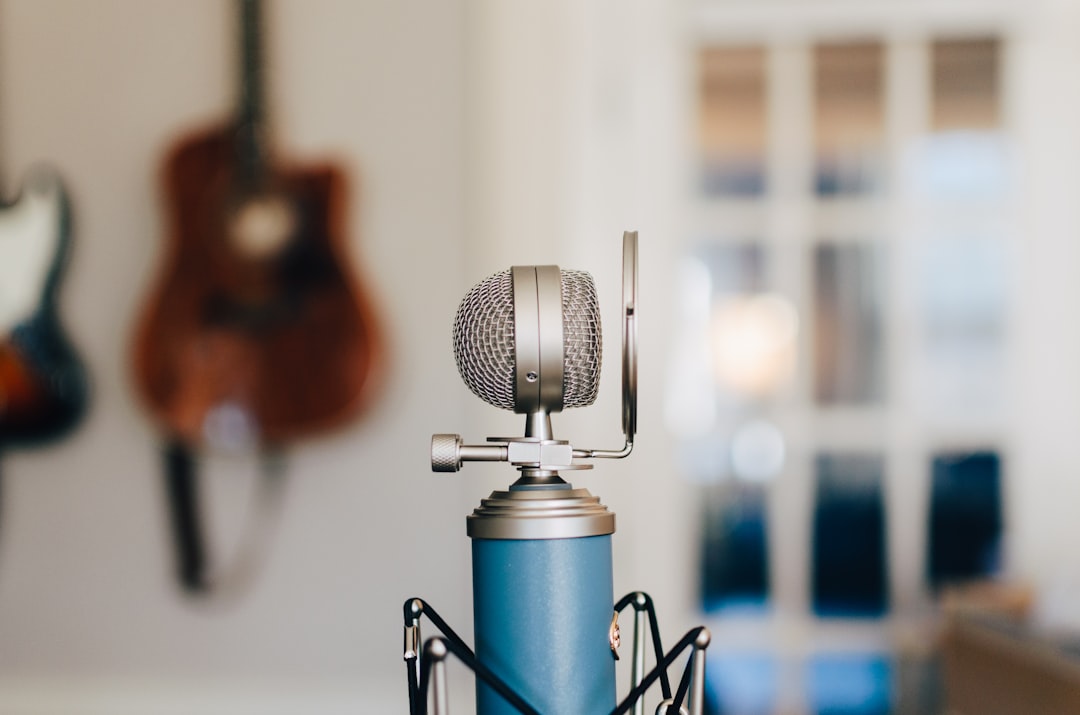
Researchers are making strides in tackling the age-old problem of background noise during voice recording sessions. A new approach utilizes a convolutional neural network (CNN) for single-channel Voice Activity Detection (VAD). This method, by leveraging spatial information within noisy audio spectrums, is proving successful in isolating the voice from the surrounding noise. Furthermore, incorporating a Self-Attention (SA) Encoder, which captures contextual noise patterns, helps to refine the accuracy of voice isolation.
This focus on cleaner recordings is essential, especially as voice synthesis technologies like Amazon's AI engine are creating more natural-sounding voices for audiobooks, podcasts, and voice cloning applications. We now have tools like Cleanvoice that can automatically eliminate background noise, offering a huge improvement in audio quality. Other noise-reduction tools allow users to fine-tune the noise cancellation, catering to specific needs of each audio production.
While these techniques can greatly enhance the overall audio quality, and ensure the listener's experience is as clear and enjoyable as possible, challenges remain. There's still a need to refine these processes and further optimize their ability to separate voice from complex noise environments. However, the progress made in separating speech from ambient sounds provides exciting new possibilities for creating higher-quality voice recordings across a broad spectrum of audio production.
Recent research in the field of voice synthesis has unveiled several intriguing approaches to dealing with background noise in audio recordings, particularly relevant for applications like voice cloning, podcast production, and audiobook narration. One line of inquiry involves the development of advanced noise reduction techniques using convolutional neural networks (CNNs). By leveraging spatial information within noisy audio spectrums, these methods strive to isolate voice signals with increased precision, resulting in a more pristine final audio product.
Furthermore, the incorporation of self-attention mechanisms into these models allows for a more contextual understanding of noise patterns. This means that the model can dynamically adapt its noise reduction strategies based on the specific characteristics of the surrounding sounds, leading to a more nuanced approach to noise filtering. These efforts aim to effectively reduce background noise while retaining the natural timbre of the voice, improving overall clarity for the listener.
There's also been a trend towards creating tools dedicated to separating voice from background noise. Some tools focus on efficient removal of noise, particularly beneficial for content like podcasts. Others provide user-adjustable settings, letting users fine-tune the noise cancellation to their specific needs, allowing creators to have more control over the final audio. These tools, while beneficial, still present some interesting challenges. For instance, achieving a perfect balance between noise reduction and preserving subtle nuances of the voice remains an active area of exploration.
Beyond these practical tools, some researchers have focused on training AI models with diverse datasets. These datasets include recordings of conversations mixed with various background noises, like music or television. The hope is that by exposing these models to a wide range of noise scenarios, they will be better able to handle those noise types when encountered in the wild. This training paradigm aims to create robust and adaptable models that can effectively suppress noise across a wider range of conditions. However, evaluating the effectiveness of these trained models is a bit of a challenge. How do you determine how well the model is performing, particularly when it comes to noise that might be present in a recording session that it hasn't been explicitly trained on?
It's also important to distinguish between noise suppression and active noise cancellation. Noise suppression techniques work in software, by filtering out noise signals directly within the audio recording. Active noise cancellation, in contrast, typically involves hardware like specialized microphones, aiming to minimize the presence of noise before it's captured at the source. It would be interesting to compare the performance of these two different techniques, although the limitations inherent in each method may create some problems with a perfect comparison.
Another interesting development is the emergence of advanced voice separation models. These models can identify and separate the primary audio source (the voice) from the background noise. This is a crucial step in improving the accuracy of audio processing, ensuring that the focus remains on the voice, enhancing the listening experience for the end user. There are many exciting avenues to continue exploring with this technology, for example, the development of improved spatial audio capabilities to render synthesized voices in a more natural and immersive manner for listeners.
There's an increased emphasis on real-time noise suppression for applications where immediate feedback is critical. This is important in scenarios like voice-controlled devices, or when podcasting or recording interviews. Deep learning-based models are showing promise in handling noise on the fly, providing significant benefits in scenarios where immediate noise removal is critical.
Research also has revealed interesting trade-offs between clarity and emotional expression within a voice. While maintaining the clarity of a voice is important, it's sometimes difficult to convey nuanced emotions without making slight compromises to that clarity. This suggests that balancing clarity and expressiveness when generating synthetic voices remains a key challenge.
Interactive voice cloning is a recent development that's showing promise. The technology allows users to change the characteristics of a voice in real time based on feedback. This can enhance the creativity of podcasters or audiobook narrators, allowing them to refine the voice further after the initial recording process.
Finally, some AI research focuses on augmenting speech production with context-rich ambient sounds. Instead of just trying to eliminate all background noises, this method adds a degree of audio realism to a synthetic voice by mixing in elements of the desired surrounding. This could enhance the listening experience by making the generated voices feel more embedded within their environment.
These advancements suggest a future where synthetic voices can seamlessly blend into various audio environments, from audiobooks to interactive podcasts. While the field continues to evolve, responsible development and ethical considerations are of utmost importance as we grapple with the increasing capability of synthesizing voices and replicating auditory environments with astounding fidelity.
How Amazon's Latest AI Research Papers Are Reshaping Voice Synthesis Technology in 2024 - Text Analysis Algorithm Maps Speech Patterns from Written Content to Audio Output
Amazon's latest research in voice synthesis has led to algorithms that can effectively map speech patterns found in written content to audio output. These algorithms, driven by sophisticated deep learning models, are able to analyze text and generate synthetic voices that closely replicate the subtleties of human speech, including variations in pitch and rhythm. This means synthesized voices are becoming more lifelike and emotionally expressive, which is particularly useful in applications such as producing audiobooks and podcasts where the ability to convey emotion is important.
While these new capabilities offer a more natural and engaging listening experience, they also bring forth new challenges. Maintaining a high level of audio quality and achieving a deep understanding of emotional nuances in voice remains a key area of ongoing research. The ethical implications of this technology are equally significant, as the potential for misuse and the importance of consent when creating synthetic voices are paramount. There is a need for thoughtful consideration of the potential societal implications of these advancements as we move forward in developing this powerful technology.
Amazon's research into text analysis algorithms has yielded fascinating results in the realm of voice synthesis. Their ability to map speech patterns directly from written text has led to substantial improvements in the naturalness of synthesized voices. This has direct implications for audiobook production, as it opens up new possibilities for modulating the voice based on the nuances of the story being told, potentially achieving a level of emotional expressiveness we haven't seen before.
A major change is the ability to clone voices using far fewer audio samples than before. The high fidelity achieved in capturing emotional inflection is notable, representing a significant departure from older techniques that relied on enormous datasets. This accessibility, due to the reduced need for extensive training data, is particularly beneficial for smaller content creators with tighter budgets.
Deep learning architectures now play a key role in allowing for real-time manipulation of synthetic voices. For podcasters, this means they can potentially modify their synthetic voice in response to live audience interactions, creating a more dynamic and interactive experience. It's a far cry from the static outputs of older text-to-speech systems.
One interesting direction has been in blending natural and synthetic sounds. The ability to integrate ambient background noise into synthesized voices makes them sound more realistic and contextualized. For audiobooks and podcasts, this adds a new layer of immersion, placing the listener within a more specific auditory environment.
Neural network training techniques are also being refined, with a growing emphasis on incorporating a wide range of situational data into the training process. This includes different accents and emotional expressions. The idea is to expand the technology's ability to adapt to different demographic groups and diverse narrative contexts, improving the overall quality of audio narration.
Amazon's multi-speaker emotional voice model has achieved a 94% accuracy in emotional expression tests. This impressive figure indicates that AI systems are beginning to understand the complex nuances of human vocal emotion, which could transform audiobook narration and the creation of immersive storytelling experiences heavily reliant on emotional cues.
Not only can these voices change based on content, but they can also be used for more sophisticated character development in audio formats. This opens up the potential for future audio dramas, where characters with complex emotional journeys are brought to life in entirely new ways.
While the focus has been on English, there's a growing need to extend these capabilities to other languages. There's active research into developing multilingual voice synthesis that can retain unique vocal characteristics while adapting to different linguistic structures. If successful, this could democratize audiobook production globally, creating opportunities for new audiences and authors.
It's encouraging that Amazon is addressing ethical concerns around voice cloning. Their focus on consent mechanisms aims to prevent the misuse of voice replication technology, ensuring that clones can only be created with explicit individual approval. This thoughtful approach to potential ethical dilemmas is crucial as the technology advances.
The use of CNNs for voice activity detection and noise reduction has proven critical for improving the audio quality of podcasts. By isolating the voice from complex acoustic environments, these models help to ensure a clear and high-fidelity listening experience, a crucial element for engaging listeners.
The field of voice synthesis is evolving rapidly, driven by the ambition to create truly natural and expressive AI-generated voices. It's a remarkable development that holds immense potential to transform how we experience audiobooks, podcasts, and interactive audio experiences moving forward.
How Amazon's Latest AI Research Papers Are Reshaping Voice Synthesis Technology in 2024 - Voice Synthesis Paper Documents Breakthrough in Natural Pause Placement
Amazon's latest research in voice synthesis has made significant strides in the area of natural pause placement, which is a key aspect of making synthetic speech sound more human-like. This ability to generate more natural-sounding pauses in synthesized voices significantly improves the quality of the audio experience across a range of applications, including audiobooks, podcasts, and voice cloning. A key part of this effort involves incorporating emotional expressiveness and individual speaker characteristics into the synthetic voice outputs, leading to voices that are more emotionally engaging and relatable. These efforts aim to bridge the gap between synthetic and natural speech, ultimately resulting in voices that are more nuanced and closer to human speech patterns. However, as these technologies advance, it becomes necessary to consider the ethical implications of this ability to replicate and generate human-like voices. Questions arise about how we evaluate the authenticity of synthetic speech and how these advancements in voice synthesis technology may impact human communication and media in the future. It seems that the future of voice synthesis will likely involve increased awareness of both the potential benefits and the possible drawbacks of this technology, as it continues to evolve and become more integrated into different aspects of our lives.
Amazon's latest research on voice synthesis has produced exciting advancements, especially in how natural pauses are integrated into the generated speech. They've managed to incorporate emotional expressiveness and speaker variability into their models, which greatly improves the realism of the synthesized voice. This leads to synthetic speech that feels more conversational and even emotionally engaged, making it ideal for customer service and assistive tech applications.
The field of text-to-speech (TTS) has seen a significant shift from traditional machine learning to deep learning, leading to a considerable leap in the clarity and naturalness of generated speech. We're even starting to see voice cloning becoming a reality within TTS, where models can precisely recreate specific speaker characteristics. FastSpeech 2 is a notable example, as it addresses limitations of past TTS models through a streamlined direct training approach, enabling more diverse and naturally flowing synthetic speech.
The algorithms employed in TTS are tackling the complex interactions between humans and machines, as they translate human voice inputs into synthetic outputs. This raises many interesting questions about authenticity and how we perceive synthetic voices in relation to natural voices. Neural speech synthesis is becoming a key area of study because of its potential to produce high-quality speech that closely matches how humans articulate words. Research now highlights the importance of incorporating emotional nuances into TTS systems to improve the user experience across a wide variety of applications.
Researchers are exploring several exciting new avenues within this field, with a growing awareness of the social and cultural impact of these advancements in voice technology. It's a fascinating time for research in the area, and the path forward will likely involve a continued focus on improving speech synthesis, while simultaneously addressing the crucial questions related to the responsible use of these powerful technologies. The ethical questions related to authenticity and replication are important as they relate to the evolution of these tools. We're at a pivotal point, where we can see the benefits of this technology and the need to be aware of potential problems as we move forward.
Get amazing AI audio voiceovers made for long-form content such as podcasts, presentations and social media. (Get started for free)
More Posts from clonemyvoice.io: