ZillExit's Audio Processing Features A Deep Dive into Its Voice Detection and Analysis Tools
ZillExit's Audio Processing Features A Deep Dive into Its Voice Detection and Analysis Tools - Advanced Voice Fingerprinting Learns 700 Unique Characteristics From Each Sample
Voice fingerprinting has evolved into a sophisticated tool for analyzing audio, now capable of identifying up to 700 unique characteristics within a single voice sample. This level of granularity allows for a much more precise understanding of individual vocal patterns, pushing the boundaries of voice recognition and authentication. Behind this advancement lies complex software that breaks down speech into its constituent parts – the rhythm of words, pauses, and even the subtleties of breathing. This detailed dissection helps build a highly specific vocal profile for each speaker. The potential applications are diverse, including improving the quality and authenticity of voice-based content in areas like audiobook production and podcasting, where a nuanced understanding of the human voice is critical. This technology highlights a fascinating trend: the ability to not only recognize speech but to also decipher its intricate components, leading to a future where voice-based technologies are more sophisticated and dependable. While the promise is considerable, challenges around data privacy and potential misuse of this powerful technology remain.
It's fascinating how advanced voice fingerprinting delves deep into the intricacies of human speech. Instead of just focusing on basic vocal patterns, these methods can now dissect and learn up to 700 unique attributes from a single audio snippet. This goes beyond simply recognizing a voice; it encompasses subtle elements like the emotional tone conveyed or even slight variations in speaking style.
Imagine the potential for replicating a voice with astonishing realism. These algorithms can capture not just the basic sound of a voice, but the nuanced emotional coloring and habits of the speaker. For things like audiobook production, this could mean characters coming to life with a far more genuine and believable feel.
One area that's piqued my interest is how the voice model adapts to subtle shifts in the speaker's voice. Studies show that stress, even fleeting mood changes, can leave a mark on how we speak. These systems are capable of detecting and learning these subtle changes, paving the way for richer and more nuanced audio experiences. Perhaps this could eventually lead to audiobooks that respond dynamically to listener interactions, offering a truly personalized narrative experience.
Voice cloning, with its reliance on in-depth spectral analysis, is able to reproduce the distinctive texture and resonance that defines a unique voice. The result is a remarkably personalized sound that adds a layer of authenticity to various audio applications. For podcasting, this can make characters and narratives feel genuine and relatable, as if they're being spoken directly to the listener.
However, the potential doesn't stop there. The complexity of human vocalization begs a question – how much of what we've learned from speech technology can be extrapolated to other species? Primate vocalizations, for instance, show varying degrees of complexity, and it's intriguing to consider the potential for studying those to better understand the evolution of human communication.
Of course, these technologies are still under development and refining their accuracy through continuous machine learning, which helps them adapt to natural changes in a voice over time. The challenges associated with aligning and processing audio samples to build a unified voice model highlight the importance of quality audio and advanced signal processing techniques to ensure crystal-clear output.
A promising advancement is the possibility of real-time voice analysis during recording. Imagine the benefits for podcasters and audiobook creators: immediate feedback during recording sessions could potentially lead to smoother, more natural delivery and reduce the need for extensive post-production editing. It's a compelling example of how technology can streamline creative processes.
It's important to acknowledge that applications for voice fingerprinting are broader than just imitation. Authenticating speakers using their voice provides another level of security in areas like interactive voice assistants and other sensitive interactions, creating a trust layer that relies on the uniqueness of individual voices. We're in a period of fascinating experimentation, and voice technology continues to surprise with its power to unlock new possibilities across a wide range of disciplines.
ZillExit's Audio Processing Features A Deep Dive into Its Voice Detection and Analysis Tools - Real Time Audio Separation Creates Studio Quality Voice Isolation
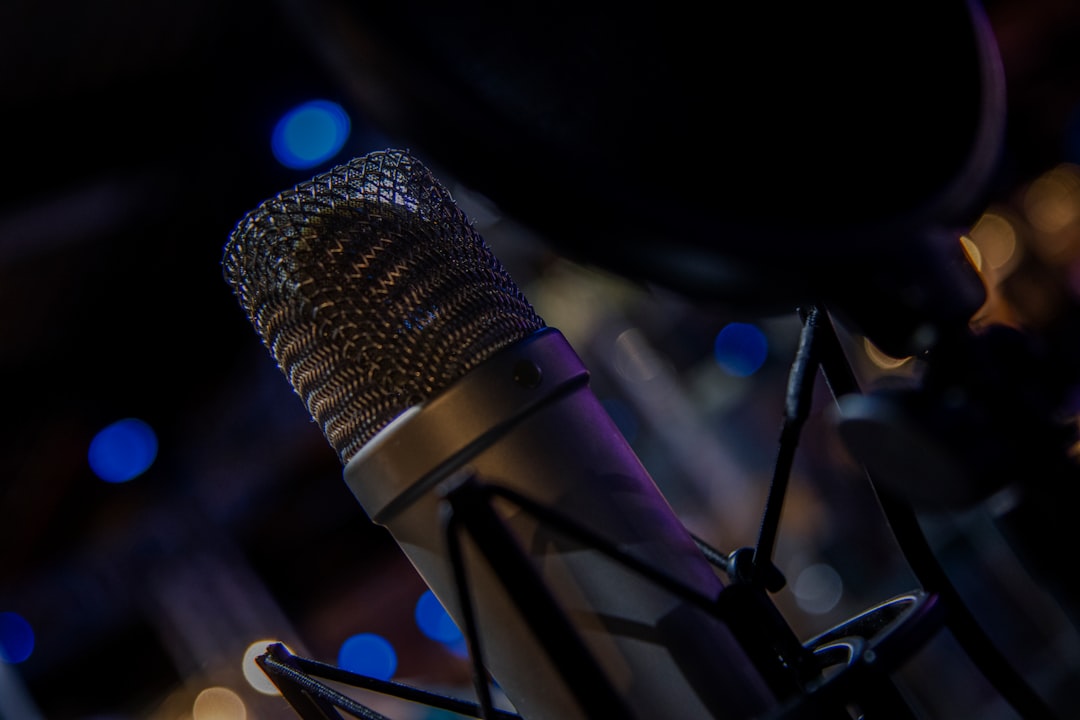
The ability to separate audio components in real-time has ushered in a new era of sound production, particularly in achieving pristine voice isolation that rivals studio-grade quality. This development empowers individuals involved in creating audiobooks, podcasts, or even voice-cloning projects to attain a level of audio clarity previously unattainable, especially when starting with less-than-perfect source material. ZillExit's tools, which are built upon advanced voice detection and analysis, have been instrumental in this evolution. They dissect audio with sophisticated algorithms to not only identify specific voices but also to isolate and refine them.
The ongoing development of machine learning further refines these audio separation techniques. This progress leads to even more nuanced and accurate control over audio elements, whether blending or extracting voices within a complex sound mix. For example, in podcast production, the capacity to seamlessly isolate individual speakers allows for intricate edits that enhance the listening experience, or for voice-cloning projects, to create more natural and convincing output.
While the immediate application is for the audio professional, it begs the question of whether these advancements can be translated into the realm of broader communication and understanding of different types of vocalizations. However, it's important to acknowledge the inherent challenges and potential ethical dilemmas that may arise. Despite this, the evolution of real-time audio separation has undeniably transformed the audio creation process. It has equipped content creators with a potent instrument that empowers them to articulate their creative visions with unparalleled fidelity.
The ability to separate audio components in real-time has opened up new possibilities, especially when it comes to isolating a voice from a complex audio mix. This is becoming increasingly important in various audio production areas, such as podcasting, audiobooks, and even voice cloning. The core of these separation techniques involves sophisticated algorithms that analyze the different sound sources within a recording.
One intriguing approach to voice isolation is phase cancellation. By carefully manipulating sound waves, the system can essentially "cancel out" unwanted sounds while preserving the voice. This is akin to sound waves colliding and partially or completely nullifying each other. For instance, in a podcast recording with background music, the system could be designed to negate the music's waves while leaving the speaker's voice intact.
Furthermore, the process heavily relies on spectral analysis. This involves breaking down the audio into its constituent frequencies and identifying which components correspond to the voice. Through understanding the unique frequency characteristics of a voice, the system can effectively distinguish it from other elements like music or environmental noise.
However, to achieve high accuracy in these audio separation processes, developers rely on machine learning models. These models are trained on huge datasets of voices, enabling them to learn diverse vocal characteristics and ultimately improve their capability to isolate voices within challenging audio scenarios. It's a never-ending refinement process where the system is constantly adapting and improving its algorithms.
It's worth noting that real-time processing still faces certain hurdles, such as latency. Any delay in the processing can hinder the creative workflow, especially during recording sessions. Optimizing these algorithms for speed and efficiency is crucial to minimize these interruptions.
Moreover, these systems are now delving into understanding the nuances of emotion conveyed through the voice. Advanced algorithms can analyze shifts in tone and pitch to identify emotional expressions. This opens up exciting avenues in audio production, potentially enabling creators to produce more nuanced and engaging experiences where the emotional depth of a speaker's voice is fully captured and presented.
Adding to the complexity, these audio separation tools are also getting more sophisticated in their ability to adapt to various recording conditions. They can learn from the audio context, adjusting their performance based on elements like room acoustics or background sounds. This adaptive learning feature ensures a consistent level of quality in the final audio output.
It's not just about separating the voice from background noise – real-time audio separation is also becoming increasingly effective at dealing with environmental noise like breath sounds or wind. Especially for outdoor recordings or situations where unwanted noises are unavoidable, isolating and minimizing these artifacts significantly helps to clean up the audio.
Intriguingly, this technology has begun to seep into live performance scenarios. Musicians and performers can now leverage these tools during a live set to isolate their voice and enhance their on-stage sound in real-time. Imagine a vocalist effortlessly separating their voice from the roar of a crowd or a musician cleanly splitting their instrument from the band mix. It's an exciting prospect that can shape the future of live performances.
Ultimately, the goal of these efforts is often to create a more engaging experience for listeners. Studies suggest that clear voice recordings improve audience understanding and retention of information. By reducing the cognitive load that listeners encounter when trying to filter out background noise or extraneous elements, creators can deliver content that is easier to process and more impactful. This holds true for podcasts, audiobooks, and other audio-centric content, showcasing the growing importance of real-time audio separation in shaping future audio experiences.
ZillExit's Audio Processing Features A Deep Dive into Its Voice Detection and Analysis Tools - Neural Networks Transform Single Voice Recordings Into Multi Character Dialogues
Neural networks are increasingly capable of transforming a single voice recording into a multi-character dialogue, a feat that was previously unimaginable. These sophisticated algorithms dissect and learn the intricate patterns of a voice, capturing not only the basic sound but also the subtle emotional nuances and stylistic variations present in speech. This allows for the creation of dialogues with distinct characters, each possessing a unique vocal identity. For applications such as audiobook production and podcasting, where immersive storytelling is essential, this technology is a game-changer, enabling a richer, more realistic and engaging auditory experience.
Despite the exciting potential, there are still obstacles to overcome. Generating multiple believable voices from a single source is a complex task, demanding significant computational resources and leading to challenges in ensuring optimal audio quality. Researchers are constantly striving to improve the processing efficiency and accuracy of these neural networks to maximize their ability to create compelling, multi-character narratives from a single voice recording. The field of audio processing is continuously evolving, with exciting advancements being made in how we can leverage artificial intelligence to create and enhance auditory experiences.
Neural networks are increasingly being used to transform a single voice recording into a multi-character dialogue, offering intriguing possibilities for audio production. It's fascinating how these systems can learn to model the emotional nuances within a single voice, allowing them to generate characters with distinct emotional tones. This ability to replicate not just the speaker's voice but their emotional state adds a layer of depth and engagement for the listener.
Furthermore, these models demonstrate a capacity to derive and represent different personality traits from a single voice sample. This opens the door for a broader array of characters, adding richness and complexity to audio narratives. Think about developing a whole story with a cast of varied characters, each with their distinct personality, yet all stemming from a single voice source.
These systems also show promise in seamlessly blending voices to simulate natural conversations. Rather than requiring a separate voice actor for every character, a single voice can be manipulated to create multiple characters interacting in a seemingly authentic way. It simplifies the production pipeline, yet retains the complexity of natural human conversations.
Interestingly, they can also adapt a voice's characteristics to replicate different genders and age ranges, further increasing their versatility. A single voice can now credibly portray a diverse range of characters, expanding the creative scope of projects relying on these tools.
One of the exciting applications is the integration of text-to-speech technology. Scripts can be fed into the model, which can then assign distinct voices to characters based on the narrative's description, essentially producing complete dialogues automatically. Imagine producing a children's audiobook with different characters spoken with unique voices, all from a single initial recording.
These systems are also becoming more contextually aware. They learn to adjust the tone and cadence based on the emotional flow of a story, resulting in a dialogue that's not just realistic but also congruent with the narrative arc. This is a vital feature in any kind of audio drama, audiobook, or even interactive storytelling application.
The ability to mimic specific speaking patterns and unique quirks further enhances the immersion of the listener. A single model can create multiple voices with distinguishable cadences, adding subtle layers to character development.
As processing power increases, these systems are pushing towards real-time dialogue generation. This opens up a range of scenarios, including live interactive performances or audiobooks that dynamically respond to user inputs. Imagine an audiobook that branches and adapts the narrative based on listener choices, in a sense creating a truly personalized experience.
The adaptability of these models isn't limited to specific voices, they can also be tailored for different genres. Think of how a thriller's dialogue might require a specific pace and tone compared to a romantic comedy. This ability to tailor the output to specific genres further improves the quality and realism of the resulting audio.
Finally, these systems show promise in incorporating cultural and linguistic nuances, making them potentially useful in producing audio content for a truly global audience. They can be taught to reflect the subtleties of spoken language across cultures, contributing to a wider reach and more immersive experiences across a range of applications.
While these are early days for neural network-driven voice transformations, they represent a compelling shift in the possibilities of audio production. As these models become more refined and sophisticated, we can expect to see even more nuanced, compelling, and creative use in everything from podcasting to audiobook production and beyond. However, just as with any powerful new technology, careful consideration must be given to ethical implications as these tools are further developed and implemented.
ZillExit's Audio Processing Features A Deep Dive into Its Voice Detection and Analysis Tools - Automated Audio Editing Reduces Podcast Production Time By 40 Percent
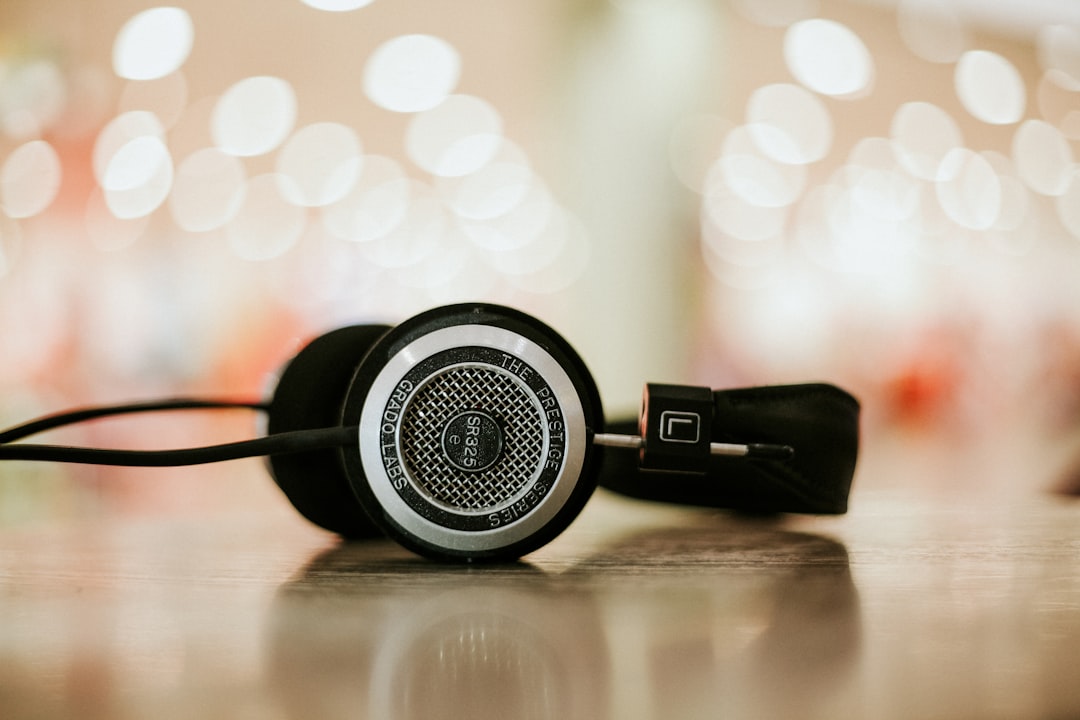
Automated audio editing tools are transforming the way podcasts are produced, with the potential to reduce production time by as much as 40%. This significant time savings allows creators to shift their focus from the often-tedious process of audio editing to the core aspects of content creation. Tasks like eliminating unwanted background noise or trimming unnecessary parts of a recording can now be handled by AI, freeing up creators to focus on developing compelling storylines and refining their narrative style. ZillExit's advanced voice processing capabilities, specifically the voice detection and analysis tools, demonstrate how these technologies can improve audio quality while simplifying the editing workflow. Real-time audio separation and AI-driven features streamline the audio production process, making achieving studio-quality sound more accessible. However, while this automation offers clear advantages, it also prompts us to consider the possible trade-offs. The increased use of AI-powered tools in audio production raises questions about whether the final audio experience will maintain the desired levels of authenticity and human touch that listeners appreciate.
The automation of audio editing, particularly in podcast production, is proving to be a game-changer. Tools like those emerging from ZillExit have the capacity to cut podcast production time by as much as 40%, a significant reduction. This shift in workflow means creators can prioritize content creation rather than getting bogged down in the often tedious editing process.
The core of this efficiency gain rests on powerful algorithms designed to isolate and refine audio. This technology has become highly effective in isolating a voice within a complex mix, a critical step in ensuring clarity. For instance, a podcast recorded in a coffee shop can be processed to remove the ambient sounds, allowing the listener to focus on the speaker without distractions. This is achieved in part through a technique called phase cancellation where carefully orchestrated sound waves can effectively nullify undesirable sounds.
A fascinating development in this area is how neural networks have begun to transform single voice recordings into multi-character dialogues. The ability to produce several unique character voices from one voice recording is an exciting, if complex, new capability. While it's still early days in this arena, the potential is transformative. Imagine audiobooks or podcasts with diverse casts of characters, all seamlessly generated from a single actor. It allows for intricate storytelling and potentially drastically reduces production costs and efforts.
The core of these neural network approaches involves sophisticated algorithms that analyze audio components, including emotional nuances. Not just replicating the sounds of a voice, but also capturing the nuances of a speaker's emotional delivery or other stylistic details is an intricate task. The result of these models is that the synthesized dialogue can convey a variety of emotions, thereby making a story more engaging.
Advanced voice fingerprinting is an exciting related technology. By identifying up to 700 distinct features in a voice, this method offers the possibility of creating incredibly lifelike voice clones. While this approach promises improvements to audiobooks or voice-based cloning technology, there are ethical questions around this technology that need to be considered moving forward.
The application of machine learning algorithms is another crucial development in audio production. These adaptive algorithms allow audio tools to analyze a recording environment and automatically adjust for consistent quality, even with challenging acoustics. The ability of these algorithms to adjust their responses based on things like the room's acoustics or the presence of background noise is extremely important and contributes to the overall quality of the final product.
The future of this field seems to be trending toward real-time audio separation and dialogue generation. Imagine a live podcast where the host can eliminate unwanted sounds instantly during the recording process, minimizing editing post-production. Or a user experience where an audiobook dynamically adjusts to listener choices, leading to a personalized story.
Moreover, researchers continue to push the boundaries of these techniques to better represent cultural and linguistic features within voices. The idea of making audio readily accessible across cultures is extremely important for spreading knowledge and understanding.
In conclusion, this evolution of AI in audio editing presents numerous opportunities to make audio-centric experiences more rich, immersive, and accessible. While the ethical considerations of these developments are still being examined, the technical advancement of AI is allowing creators to make compelling audio experiences for audiences across various fields, from podcasting to interactive stories.
ZillExit's Audio Processing Features A Deep Dive into Its Voice Detection and Analysis Tools - Machine Learning Models Convert Text To Natural Speech In 27 Languages
Machine learning models have made remarkable progress in converting written text into natural-sounding speech across 27 languages. This capability holds immense potential for various audio-related applications, including audiobook production, podcast creation, and even voice cloning. The foundation for these advancements lies in deep learning techniques that empower these models to generate remarkably realistic audio. The quality of synthetic speech has seen a dramatic leap, making it increasingly difficult to differentiate from human voices. While these innovations offer the promise of more immersive and interactive audio experiences, they also spark valid concerns regarding the authenticity of synthesized voices and the potential ethical ramifications of voice cloning technologies. As these models continue to develop, we can anticipate an even more complex and responsive landscape for audio production, where the line between human and artificial voices continues to blur, prompting deeper reflection on the nature of auditory communication.
ZillExit's audio processing capabilities extend to converting text into natural-sounding speech across 27 languages. This is a testament to the advancements in linguistic modeling and machine learning, which are essential for dealing with the unique phonetic structures, intonations, and cultural nuances present in each language.
Interestingly, these models are not just replicating sounds but also capturing the subtle emotional textures within a voice. This opens up intriguing possibilities for audiobook narration and other applications where conveying a range of emotions is critical. Think of how a story can come alive with a narrator who can realistically express happiness or sadness, making the experience richer.
Moreover, the models demonstrate a remarkable ability to adapt to a speaker's emotional state or even mirror subtle changes in their mood over time. This kind of flexibility is particularly important in environments like podcasts where maintaining audience engagement requires shifts in tone and pacing.
From a production standpoint, text-to-speech features can significantly streamline the creation of audiobooks. The ability to automatically generate high-quality narrations could potentially reduce the need for human voice actors, freeing up content creators to focus on plot development and narrative refinement.
The technology can also be used to clone individual voices. By capturing distinctive features from a short audio sample, remarkably lifelike replicas of a voice can be generated. This capability creates new opportunities for personalizing audio content, particularly in podcasting, where host voices often build a strong sense of identity.
Furthermore, these models integrate seamlessly with scriptwriting, allowing for real-time synthesis and modifications to dialogue during live recording or event narrations. This provides greater adaptability and flexibility during the content creation process.
Another noteworthy aspect is that the models are designed to incorporate prosodic elements, such as stress, rhythm, and intonation. This leads to a more nuanced and authentic experience compared to simpler synthetic voices, making the generated speech sound more like a natural human voice.
The ability to represent diverse accents and dialects further expands the reach of audio content. This precision in language modeling creates possibilities for developing audio productions that are culturally relevant and accessible to a broader audience.
While the focus is on enhancing audio experiences, a critical application could be in fighting spam. By employing verbal hesitations and fillers in synthetic voices, content creators might find innovative ways to make generated audio more convincingly human, thus providing another level of defense against automated spam or malicious content.
These text-to-speech models are generally built on the foundation of neural networks that are continuously trained and refined. As they are exposed to larger and more varied datasets of human voices, their ability to faithfully reproduce human speech with a wide range of characteristics and emotional contexts will only continue to improve over time.
ZillExit's Audio Processing Features A Deep Dive into Its Voice Detection and Analysis Tools - Custom Voice Libraries Enable Consistent Audio Narration Across Projects
The ability to build custom voice libraries is changing how consistent audio narration is achieved across various projects. This means that whether it's for audiobooks, podcasts, or even voice cloning efforts, we can now generate synthetic voices that retain a consistent sonic identity across multiple recordings. Advanced neural networks and complex algorithms allow us to capture not only the basic sound of a voice, but also subtle emotional nuances and personal speaking styles. This translates into a more engaging audio experience for listeners, especially when different characters are needed or a consistent brand voice is desired. While the potential of custom voice libraries is immense, concerns about maintaining the authenticity and human element in audio remain valid. The ongoing evolution of this field suggests exciting advancements lie ahead, especially in the ability to personalize audio content with tailored voices and refine the interactions between human and synthetic speech.
Custom voice libraries are becoming increasingly important in audio production, enabling a level of consistency across projects that was previously difficult to achieve. These libraries are built on advanced voice cloning and analysis techniques that allow us to capture and replicate the intricacies of a human voice. This is done through the creation of detailed voice profiles, which can incorporate up to 700 unique characteristics of a speaker's vocal patterns.
One of the intriguing aspects of custom voice libraries is the ability to meticulously control the emotional nuances of a synthetic voice. In the context of audiobooks, for instance, this allows for characters to be portrayed with a far wider range of emotions, making them feel more lifelike and relatable. AI algorithms play a crucial role here by automatically adjusting the voice characteristics based on the context of the narrative, ensuring that the voice delivery complements the story in a fluid way.
The ability to adapt to different accents and dialects is another important aspect. This means that a voice clone can be trained to sound authentic in diverse cultural contexts, making audio content more accessible to a global audience. This is extremely valuable for educational resources, for example, where consistent pronunciation and intonation can be vital.
Moreover, the use of custom voice libraries can significantly streamline audio production workflows. By automating the creation of consistently high-quality voice outputs, editors can reduce the time spent on tedious post-production tasks. This shift can free up creative teams to focus on storytelling and narrative development rather than spending their time on meticulous sound editing.
Perhaps the most notable advantage of custom voice libraries is the potential for single voice actors to create multiple distinct character voices. This offers a novel way of producing dialogues in audiobooks or podcasts, effectively enabling solo artists to achieve the complex sound design of a multi-actor cast. This ability to synthesize multiple unique voices from a single source has implications for production costs and can open opportunities for a new kind of audio production workflow.
We're seeing early indications that future technologies could enable real-time responsiveness in audio. Imagine audiobooks that adapt and adjust narratives based on listener choices, leading to truly personalized story experiences. Likewise, voice actors could benefit from the ability to create custom voices that adapt to gender, age, and character traits with ease.
While these advancements are exciting, they also raise important ethical considerations. As voice cloning technology becomes increasingly sophisticated, it's vital that we address issues surrounding authenticity, consent, and potential misuse. It's crucial to maintain transparency in how these technologies are being used, as the lines between human and synthetic voice continue to blur.
Overall, the development of custom voice libraries presents a compelling set of advancements that can significantly impact the future of audio production. We're poised to experience an era of more engaging, accessible, and creative audio experiences across a range of media formats. As with any powerful technology, it's vital that we approach these developments with a critical and balanced perspective, considering both the creative potential and the ethical ramifications that accompany this level of control over the human voice.
More Posts from clonemyvoice.io: