Get amazing AI audio voiceovers made for long-form content such as podcasts, presentations and social media. (Get started for free)
The Impact of Pitch Variation on Emotional Recognition in Voice Cloning A 2024 Analysis
The Impact of Pitch Variation on Emotional Recognition in Voice Cloning A 2024 Analysis - Pitch Modulation Changes in Zero Shot Voice Cloning 2024
The year 2024 has witnessed a surge in zero-shot voice cloning capabilities, especially in the realm of pitch control and emotional expression. This means we can now synthesize voices with a level of stylistic flexibility previously unimaginable, moving beyond simply mimicking a specific speaker's traits. The ability to clone a voice using very short audio clips simplifies the cloning process and extends its reach to various languages, a boon for podcasting, audiobook creation, and a wide range of audio content production. While progress has been made in generating voices that sound natural and closely resemble the original, achieving truly indistinguishable synthetic speech remains an ongoing challenge. The integration of pitch adjustments that convey emotion is a promising development that has the potential to greatly enhance the realism and user experience in human-machine interactions. As this field advances, we can anticipate even more nuanced and expressive synthetic voices becoming a staple in our digital landscape.
In the realm of voice cloning, particularly within the zero-shot paradigm, the ability to manipulate pitch has become increasingly sophisticated in 2024. This capability is crucial for capturing the nuanced emotional expressions inherent in human speech. While earlier methods often relied on extensive training data for each voice, newer models can generate diverse pitch variations with remarkably little input, often requiring only a short audio snippet. This is made possible through the power of advanced neural network architectures that have become adept at mimicking the subtle intricacies of human vocal patterns.
The improved flexibility in pitch manipulation has opened exciting possibilities across various audio applications. For instance, audiobook productions can now leverage voice clones that retain the authentic emotional timbre of the original speaker throughout the narrative, enhancing the listener's immersion. However, achieving a truly natural-sounding pitch shift remains a challenge. The human auditory system is incredibly sensitive to pitch variations, able to detect shifts as small as 1%. This sensitivity presents a hurdle for developers aiming to faithfully replicate a speaker's voice while simultaneously implementing dynamic pitch modulation.
Podcast production has also seen a surge in interest surrounding pitch modulation in cloned voices. Research suggests that variations in pitch not only convey emotional cues but also play a key role in maintaining audience engagement. Well-crafted pitch manipulation can make a significant difference in retaining listeners' interest throughout an episode, highlighting the importance of mastering this aspect of voice cloning technology.
Moreover, recent advances in voice cloning algorithms have seen the implementation of emotional context models. These models can dynamically adjust pitch modulation, enabling more nuanced character portrayals in audio dramas and similar productions. Conversely, monotonous pitch can quickly lead to listener disengagement, highlighting the need for content creators to leverage pitch variation strategically to maintain audience connection. Emerging techniques now incorporate pitch tracking tools to analyze real-time vocal performances, pushing the boundaries of voice cloning by allowing for even more refined modulation that aligns with not only pitch, but also tempo and rhythm.
It is crucial to understand that pitch plays a pivotal role in shaping our perception of a speaker's identity. If the pitch modulation deviates substantially from the original speaker's natural patterns, listeners may struggle to recognize the cloned voice. Consequently, replicating the speaker's natural vocal characteristics with high fidelity remains a key priority within the development of voice cloning. Looking forward, the ongoing evolution of voice cloning technology is poised to bring about more sophisticated algorithms for incorporating emotional pitch variation. This refinement will enable a more accurate acoustic representation of distinct emotional states, thereby fostering improved storytelling and character development within digital media.
The Impact of Pitch Variation on Emotional Recognition in Voice Cloning A 2024 Analysis - Neural Network Training with Voice Actor Emotion Datasets
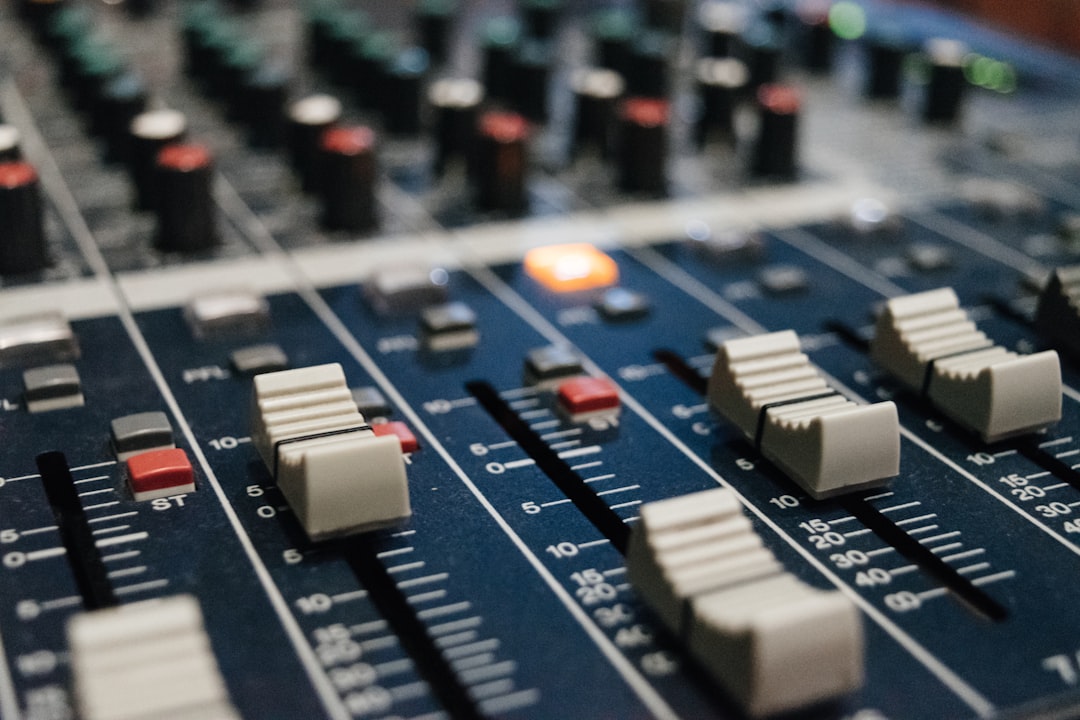
Training neural networks with datasets featuring voice actors portraying various emotions is becoming increasingly vital in the evolution of voice cloning. The goal is to equip these models with the ability to understand and recreate a wide spectrum of emotional expressions through the human voice, thereby significantly enhancing the emotional richness of audio productions. However, achieving this goal faces obstacles due to the diverse linguistic and recording environments inherent in these datasets. Despite these challenges, advancements like the Emotion2Vec model show promise in refining emotional detection and representation. This is especially relevant in fields like audiobook narration and podcast creation, where accurately conveying emotional nuance can significantly impact listener engagement and experience. As these emotion-focused datasets mature, we can anticipate more sophisticated methods for synthetic voices to mirror authentic human emotions, expanding the possibilities within audio storytelling and character portrayal.
Researchers are increasingly leveraging neural networks trained on voice actor emotion datasets to enhance voice cloning capabilities. These datasets can include a wide range of emotional expressions, allowing for the creation of synthetic voices that convey a spectrum of emotions, from happiness to sorrow. It's fascinating to explore how these datasets can help create more realistic, expressive voices.
One intriguing aspect is the use of advanced pitch manipulation techniques within these neural networks. By subtly altering pitch during crucial narrative moments, voice clones can potentially enhance the emotional impact and overall understanding of audiobooks. However, the human ear is incredibly sensitive to pitch variations, even minor changes around 1-3% can drastically alter perceived emotion, adding a layer of complexity to achieving natural-sounding emotional delivery.
The accuracy of emotional recognition from synthetic voices has seen promising improvements when trained on high-quality emotion-labeled datasets. Studies have suggested that accuracy can exceed 85% under these conditions, hinting at the importance of curating robust emotion datasets for developing effective voice cloning systems. This is a key factor driving progress in creating emotionally nuanced synthetic speech.
Interestingly, researchers are employing techniques like Generative Adversarial Networks (GANs) to improve the training process. GANs involve a sort of competitive training where two networks work against each other, aiming to improve the quality of the synthesized voice. This is crucial to minimize artifacts and create more natural-sounding cloned voices.
Beyond simply replicating emotion, some work is exploring how pitch variation can enhance genre-specific storytelling. In audio dramas and similar productions, voice clones can be trained to adapt their vocal styles to fit different narrative contexts and audiences. This implies the potential to fine-tune voices to match the unique tone and style of a particular genre.
Moreover, there's an emerging trend towards real-time pitch adjustment based on listener feedback. Imagine a podcast where the synthetic voice adapts its emotional delivery based on audience reactions – this is an exciting possibility that could revolutionize interactive audio experiences.
Recent research indicates that pitch modulation can also mimic subtle physical cues associated with human speech, such as lip and throat movements. This contributes to a more relatable and lifelike quality in synthetic speech, forging a stronger connection with the listener.
Furthermore, reinforcement learning models are increasingly utilized in voice cloning. These models allow for ongoing improvements in pitch modulation through iterative feedback loops, making the synthetic voices more adaptable to different emotional contexts over time. This could lead to even more sophisticated and context-aware emotional expressions in the future.
The integration of these emotion-specific datasets has yielded noteworthy improvements in audience engagement. Studies have shown a correlation between the effective use of emotional delivery through pitch variation and a significant increase in listener retention rates for audiobooks. This highlights the importance of focusing on these details in audio content production.
Despite the advancements, challenges remain in achieving truly seamless and indistinguishable synthetic speech that perfectly replicates the intricate emotional nuances of human voices. Further research is needed to refine these techniques and push the boundaries of emotional expression in synthetic speech. It is clear that these advancements in voice cloning, fueled by improvements in emotional recognition and pitch control, have far-reaching implications for how we create and consume audio content in 2024.
The Impact of Pitch Variation on Emotional Recognition in Voice Cloning A 2024 Analysis - The Integration of Human Speech Patterns in Audio Production
The incorporation of human-like speech patterns into audio production is a rapidly developing area, particularly within the context of voice cloning. The ability to manipulate pitch within these cloned voices allows audio creators to more accurately mimic the natural emotional expressions we find in human speech. This has opened exciting possibilities in fields like audiobook creation and podcasting, where conveying emotion is vital for listener engagement. Using cloned voices that convincingly replicate the subtle variations in pitch present in human speech can enhance storytelling, creating a more immersive and natural listening experience.
However, this also highlights the challenges of achieving truly convincing emotional expression through synthetic voices. Our ears are extraordinarily sensitive to subtle pitch changes, and the line between realistic and artificial vocalizations can be easily crossed. As the technology matures, a primary focus will be to refine algorithms so that emotional expression via pitch manipulation doesn't result in robotic or unnatural-sounding voices. The key is to find a balance between utilizing the advancements in voice cloning to create more emotionally engaging audio while simultaneously maintaining a level of authenticity that resonates with listeners who are increasingly discerning about the qualities of digital speech. The human element of speech remains a complex puzzle to truly replicate, making continuous improvements in this field critical for future advancements in audio production.
The human auditory system is incredibly sensitive to subtle changes in pitch, capable of detecting variations as small as 1%. This sensitivity poses a significant challenge for audio production techniques, particularly in voice cloning, where accurate pitch manipulation is critical for conveying emotions realistically. Research suggests that pitch variations not only impact emotional perception but also influence how we cognitively process audio information. Higher pitches tend to be associated with excitement or happiness, while lower pitches often suggest sadness or calmness, highlighting the crucial role of pitch in shaping the emotional narrative of audio content.
Modern voice cloning techniques, powered by deep learning, now enable us to retain a speaker's characteristic emotional nuances while manipulating pitch. This means that synthesized voices can not only match a speaker's pitch but also their emotional tone, enhancing the authenticity of audio productions. Furthermore, real-time pitch modification algorithms are emerging, allowing synthetic voices to dynamically adapt during playback. This opens the door for audio experiences that respond to listener feedback or narrative context, ushering in a new era of interactive audio experiences.
The development of accurate emotion detection algorithms for synthetic speech relies heavily on high-quality training data, specifically datasets of voice actors portraying different emotions. These datasets have proven valuable, with some systems achieving over 85% accuracy in emotional recognition through pitch manipulation. Techniques like Generative Adversarial Networks (GANs) are instrumental in improving the quality of pitch modulation by utilizing a competitive training process that reduces synthetic artifacts. This allows us to create more natural-sounding and less artificial cloned voices.
Beyond simply replicating emotional expression, researchers are also investigating how to tailor pitch styles to specific genres of storytelling. By adjusting the characteristics of cloned voices, we can enhance the emotional impact of audio dramas, audiobooks, and other forms of audio content to align with the specific expectations of each genre and audience. Studies have shown a link between effective emotional delivery through pitch variation and increased audience engagement, suggesting that mastering pitch modulation is crucial for maintaining listener attention in audio content.
Interestingly, emerging techniques in pitch manipulation aim to replicate not just pitch but also subtle physical aspects of human speech like lip and throat movements. This adds a layer of realism and relatability to synthesized voices, enabling them to create stronger connections with listeners. Reinforcement learning, too, plays a growing role in refining pitch modulation, leading to algorithms that can adapt to different emotional contexts over time. This adaptive nature promises future voice cloning systems that are capable of providing more nuanced and context-aware emotional expressions in audio content. While considerable progress has been made, further research is essential to refine these techniques and unlock the full potential of emotional expression in synthetic speech. The ongoing advancements in voice cloning, especially in the area of emotional recognition and pitch control, have wide-ranging implications for how we create and experience audio content in the future.
The Impact of Pitch Variation on Emotional Recognition in Voice Cloning A 2024 Analysis - Real Time Emotion Recognition in Podcast Recording Software
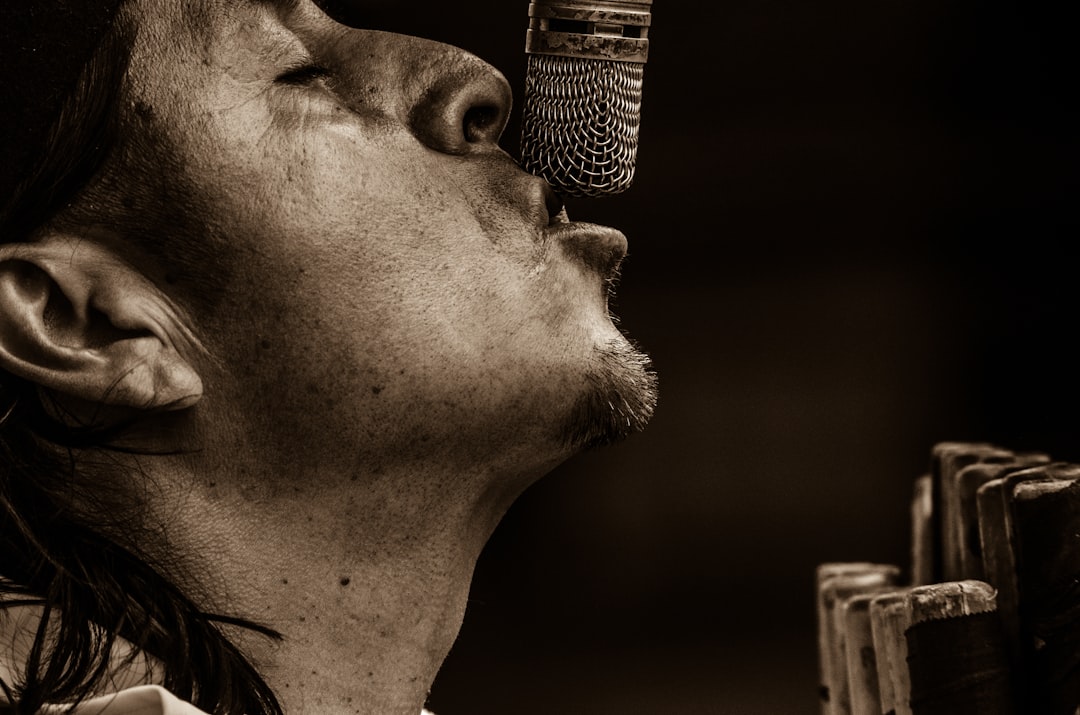
Podcast recording software is increasingly incorporating real-time emotion recognition capabilities, driven by improvements in speech recognition and machine learning. These tools analyze a speaker's voice in real-time, specifically focusing on pitch variations, which are crucial indicators of emotional states. The goal is to provide creators with greater insight into the emotional nuances of their recordings, allowing them to craft more engaging and impactful audio narratives.
Recent advancements allow for dynamic adjustments to pitch during recording, potentially opening up a new dimension in storytelling and audio production. Producers can experiment with conveying a broader range of emotions in their content, potentially leading to a more impactful and immersive listening experience. The accuracy of these emotion recognition systems is steadily improving, further enhancing the power of voice cloning technologies.
However, achieving a perfect balance between natural-sounding vocal expressions and dynamic pitch manipulation remains a hurdle. While these advancements offer exciting possibilities for podcasters and audiobook creators, it's crucial to consider the potential impact on audience engagement. If the pitch manipulation is too obvious or unnatural, it can disrupt the listener's immersion and diminish the overall impact of the story or message. Future development should strive to ensure that emotion recognition and pitch modulation work in harmony, fostering a seamless listening experience that strengthens the connection between the audio creator and their audience.
Real-time emotion recognition within podcast software is becoming increasingly sophisticated, utilizing machine learning to analyze speech patterns and instantly adapt audio elements like pitch and tone. This capability aims to improve storytelling and maintain audience engagement by tailoring the emotional delivery of the audio content.
The impact of even subtle pitch changes on listener perception is undeniable. Research shows that variations as small as a single percent can significantly alter the emotional interpretation of spoken words, underscoring the importance of precise pitch control in audio production.
Modern emotion recognition models are moving beyond just pitch analysis. They incorporate factors like tempo and rhythm, allowing for a more holistic understanding of emotional delivery in real time. This can be further refined through audience interactions, providing a feedback loop for dynamic adjustment.
However, the sensitivity of the human auditory system poses a challenge. Our ears can detect pitch differences as minute as 1/100th of a musical semitone. Voice cloning technology must account for this incredible sensitivity when aiming for emotionally authentic results.
By training voice cloning systems on a range of emotional expressions, researchers are striving to develop more nuanced synthetic voices. These models can capture not just basic emotional states but also more complex ones, like sarcasm or nostalgia, allowing for greater depth in narratives for podcasts and audiobooks.
The ability to adapt a synthetic voice's pitch and tone to different genres is emerging as a key feature. This means a voice clone could adjust its style to best fit a particular narrative, ensuring it connects with the target audience more effectively.
Generative Adversarial Networks (GANs) are proving useful in refining pitch modulation within voice cloning. The competitive training process inherent in GANs helps improve the quality of synthetic voices by constantly pushing for more realism in audio output.
Scientists are investigating the possibilities of integrating the physical cues present in human speech into voice cloning. This includes replicating the subtle movements of lips and throat, leading to synthetic speech that feels more authentic and relatable.
Podcast software incorporating interactive features could enable listeners to directly influence the emotional tone of a synthetic voice. The ability to adjust the voice in real time based on audience feedback represents a potential revolution in audio content creation and consumption.
Studies have clearly shown that a thoughtful approach to pitch variation aligned with emotional intent can lead to better listener retention in podcasts and audiobooks. This underscores the importance of focusing on emotional delivery through pitch manipulation for creating compelling audio content.
The Impact of Pitch Variation on Emotional Recognition in Voice Cloning A 2024 Analysis - Voice Pattern Analysis Through Machine Learning Frameworks
Voice pattern analysis is undergoing a significant transformation thanks to machine learning frameworks. These frameworks, particularly those employing techniques like convolutional neural networks, are being used to analyze the subtle variations in pitch that are closely tied to emotional expression in human speech. This capability is becoming increasingly important in the development of realistic voice cloning, where the ability to generate emotionally nuanced synthetic voices is a major goal.
The goal is to enhance the experience of audiobooks, podcasts, and other forms of audio content by making the synthetic voices sound more human. To this end, machine learning is being combined with techniques like multi-modal feature fusion, which integrate various acoustic features to improve the accuracy of emotional recognition within voice recordings.
While there are exciting advancements being made, we still have a ways to go in achieving truly natural-sounding voice cloning. Selecting the right features for analysis is crucial for achieving higher accuracy, but selecting these features without negatively impacting the natural flow of synthetic voices remains a challenge. The development of improved methods for feature selection and extraction continues to be an important research focus.
Machine learning frameworks are becoming increasingly important for analyzing voice patterns, especially in voice cloning, where recognizing emotion is key. Pitch variations are a crucial aspect of conveying emotion, with different emotional states often linked to specific pitch characteristics. We, as humans, communicate emotion not just through words but also through acoustic details like pitch, tone, and volume. Research often uses short, synthetic vocalizations representing various emotions like anger or fear to study these aspects.
Convolutional Neural Networks (CNNs) are a common tool for speech emotion recognition (SER), classifying emotional states based on voice signals. Combining various acoustic features through multi-modal feature fusion techniques has shown promising results in improving the accuracy of emotion recognition. The ability to recognize emotional states from voices has significant implications for improving how humans and computers interact and for personalized experiences across various applications.
Choosing the right features and extracting them effectively is essential for achieving high accuracy when using machine learning models for analyzing emotion in speech. The intricacies of human emotional expression are complex, meaning advanced analytical approaches are required for accurately interpreting vocal patterns. Speech emotion recognition is a field that's constantly evolving, with researchers continually developing more sophisticated algorithms and applications within affective computing. It’s still quite a challenging field given that humans can identify subtle pitch shifts in the 1% range. While algorithms have made great strides in audio book production, podcasting, and voice cloning applications, there’s still work to be done in refining emotional authenticity. There's still a noticeable difference between how humans naturally express emotion versus how artificial intelligence approaches this. This gap is often evident to listeners and can impact engagement.
It's important to note that even with the remarkable progress made in voice cloning technology, and in particular with zero-shot learning, there are still notable limitations. One of the most challenging aspects is achieving that seamless transition between emotional states with vocal patterns. Getting the pitch modulation correct is very tricky, and in some cases, it can disrupt the listening experience for listeners. There is a fine line between effective use of vocal inflection and something that sounds robotic. We still have a long way to go to truly bridge the gap between natural speech and synthetic speech in emotional depth and nuance. The use of Generative Adversarial Networks (GANs) can help, but these often require significant training resources, which can be costly. There's exciting research being done in this space, and I suspect that over the next few years, we will see refinements to the models being trained, which should lead to more natural-sounding voice clones.
Get amazing AI audio voiceovers made for long-form content such as podcasts, presentations and social media. (Get started for free)
More Posts from clonemyvoice.io: