Get amazing AI audio voiceovers made for long-form content such as podcasts, presentations and social media. (Get started for free)
Voice Digitization of 'Supercalifragilisticexpialidocious' How Modern AI Handles Complex Phonetic Patterns in Voice Synthesis
Voice Digitization of 'Supercalifragilisticexpialidocious' How Modern AI Handles Complex Phonetic Patterns in Voice Synthesis - Breaking Down Mary Poppins Legacy Through Phonetic Pattern Analysis in 2024
"Breaking Down Mary Poppins Legacy Through Phonetic Pattern Analysis in 2024" examines how "Supercalifragilisticexpialidocious" has become a touchstone for understanding complex sound patterns. The word's intricate phonetic structure isn't just a fun linguistic quirk, but a challenging test case for the sophisticated AI systems now driving advancements in voice technology. This analysis aims to show how the lasting impact of "Mary Poppins" is intertwined with the ability of modern AI to reproduce these complex phonetic nuances. In exploring this connection, we see how audiobook production and the rise of podcasts have benefited from these AI advancements in voice cloning and audio manipulation. This development isn't simply about replicating a cherished film's sound; it represents how the legacy of "Mary Poppins" influences creative innovation in how we experience audio today. While appreciating the past, this examination also highlights the new frontiers opened up by contemporary sound technology, pushing the boundaries of what's possible in audio narratives and voice cloning.
"Supercalifragilisticexpialidocious," the famous word from *Mary Poppins*, presents a fascinating challenge for voice synthesis, showcasing the complexities inherent in human speech. With its 34 syllables, each needing precise articulation, it highlights the need for AI systems to meticulously manage individual phonetic elements. Analyzing its phonetic patterns reveals intriguing stress variations that impact meaning and pose challenges for voice cloning. Replicating those nuanced emphasis shifts digitally requires sophisticated algorithms that can capture the subtle nuances that human speakers use.
The richness of vowel sounds within this word makes it an interesting test case for studying diphthongs and triphthongs in the context of digital voice production. It provides insight into how AI models handle these complex phonetic structures, which are integral to creating natural-sounding voices. When dealing with audio productions, replicating the subtle inflections and tonal changes in the human voice remains a hurdle for AI. This difficulty intensifies with intricate phrases like those found in *Mary Poppins*. Even the playful nature of the lyrics can introduce confusion for AI if it's not trained on appropriately diverse datasets.
The application of AI isn't limited to just synthesizing voices. It's also being used for emotion detection, and that capability is important for ensuring that a cloned voice, like Mary Poppins', conveys the intended emotions accurately. This is crucial for capturing the essence of a character. Audiobook production with cloned voices brings about unique difficulties, particularly when adapting light-hearted and imaginative texts like the one from *Mary Poppins*. The subtle shifts in pronunciation needed to maintain the essence of the original performance depend on a deep understanding of the finer points of phonetics.
Acoustic models, employing deep learning, are used in voice cloning to analyze phonetic elements of songs. But these models can struggle with unusual language and newly coined words, like the more elaborate constructions in *Mary Poppins*. Examining phonetic patterns in cherished songs helps researchers understand how linguistic idiosyncrasies, such as the playful nature of phrasing, affect diction and pace in synthesized voices. Ultimately, how the audience perceives these variations during listening heavily relies on these subtle aspects.
In essence, voice digitization is more than just generating clear sounds; it's about grasping the intricate phonetic rules that underpin human speech, as seen in the rich soundscapes of *Mary Poppins*. Understanding these fundamental patterns is key for enhancing the overall quality of voice synthesis, moving beyond simple reproduction and aiming for a truly human-like experience.
Voice Digitization of 'Supercalifragilisticexpialidocious' How Modern AI Handles Complex Phonetic Patterns in Voice Synthesis - Voice Cloning Complexity The 37 Phonemes Challenge for Long Words
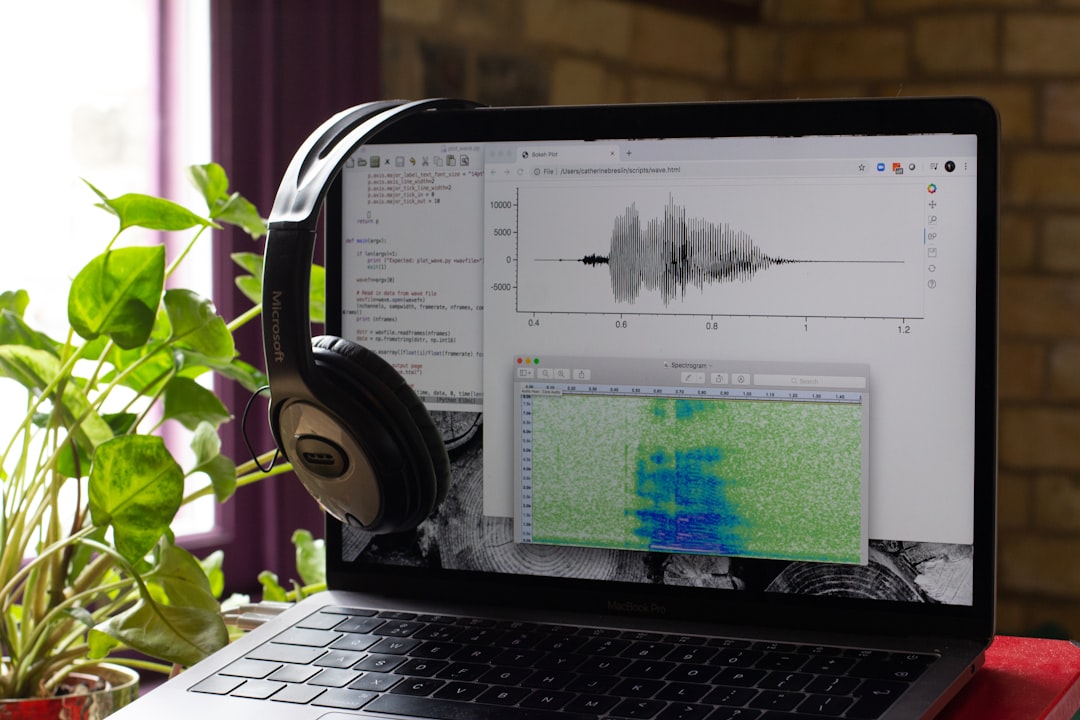
The intricacies of voice cloning are particularly evident when dealing with lengthy words like "Supercalifragilisticexpialidocious." These words act as a crucial testing ground for evaluating how well AI can handle the complexities of human speech, especially when it comes to a wide range of phonetic elements. This challenge, often referred to as the "37 Phonemes Challenge," emphasizes the need for systems to accurately and precisely articulate complex and extended phrases.
Successfully synthesizing a natural-sounding voice involves more than just generating sounds. It necessitates mastering the subtle variations in pitch, tone, and emotional expression that humans use in their everyday speech. While AI voice technology is making impressive strides in capturing these nuances, some complex phonetic combinations still present a hurdle.
This ongoing development highlights the fascinating interplay between phonetics and voice synthesis. It impacts the potential for various audio experiences, like audiobooks or podcasts. The ability to accurately capture the subtleties of human speech is a critical aspect of creating truly immersive and engaging audio content. The quest for perfectly replicating the human voice remains a constant push and pull between technological capability and the inherent complexity of language.
Replicating human speech, especially complex words like "Supercalifragilisticexpialidocious," presents a significant challenge for voice cloning technology. The English language boasts 37 distinct phonemes, and this word, with its 34 syllables, tests a system's ability to handle a wide array of phonetic combinations. Each syllable holds subtle nuances, and slight deviations from the intended pronunciation can quickly lead to a robotic or unclear sound.
Furthermore, stress patterns, the emphasis placed on particular syllables within words and phrases, are crucial for capturing the expressiveness and intent of human speech. AI voice cloning systems must effectively integrate dynamic stress shifts to accurately mimic human emotion and meaning. Another hurdle comes from the nature of speech production itself: coarticulation. As we speak, the sounds we produce overlap and influence each other, affecting the shape of our mouth and the sound that emerges. Successfully cloning a voice requires algorithms adept at predicting and replicating these subtle shifts in sounds across different phonetic environments, which becomes incredibly challenging in long, complex words.
The goal, of course, isn't just perfect reproduction of sounds. AI should also capture emotional undertones, crucial for character portrayal in audiobooks and other audio formats. However, this becomes difficult with uncommon linguistic constructions. The playful nature of words like "Supercalifragilisticexpialidocious" poses a challenge because the AI model might not have sufficient training data for such unique language structures. Maintaining the character of the original speech requires mastering the rhythm and pace, which can be tricky for synthesized voices.
The limitations we see are often tied to the datasets used for training. If the data isn't diverse enough, the AI might not be able to adequately capture the nuanced phonetic patterns that make human voices so compelling. It's also crucial that systems can adapt to individual speakers' idiosyncrasies—each of us has unique vocal characteristics that make our voices recognizable.
The pursuit of more realistic and human-like voice cloning has spurred significant advancements in speech analysis and synthesis algorithms. Researchers continue to refine these technologies to better model the intricacy of human speech. These efforts pave the way for more refined audio experiences, particularly in audiobook production, podcast creation, and voice-based applications where nuanced and engaging voices are needed.
Voice Digitization of 'Supercalifragilisticexpialidocious' How Modern AI Handles Complex Phonetic Patterns in Voice Synthesis - Text to Speech Evolution From Carnegie Mellon Early Models to Modern Audio Books
The journey of text-to-speech (TTS) technology has been a fascinating evolution, moving from the early, somewhat clunky systems developed at Carnegie Mellon University to the sophisticated technologies powering modern audiobooks. Early TTS relied on simpler techniques that often resulted in robotic, unnatural-sounding voices. However, with the rise of deep learning, AI models have advanced to a point where they can generate speech incredibly similar to a human voice. The result is a significant improvement in the production of audiobooks, enhancing the listening experience with more natural and nuanced storytelling. This evolution has also had a big impact on the development of podcasts and other audio formats, where the quality of the synthesized voice greatly contributes to how listeners connect with the content. The transformation from those early, rudimentary systems to the highly advanced voice cloning technologies of today is remarkable, fundamentally altering how we understand and interact with spoken language through technology.
The journey of text-to-speech (TTS) has seen a remarkable transformation, moving from rudimentary systems at Carnegie Mellon to the sophisticated audio books we enjoy today. Early efforts, primarily from the 1970s, relied on a 'cut and paste' approach, stitching together snippets of recorded speech. This concatenative method, while a foundational step, paved the way for more sophisticated neural network-based systems that synthesize speech from the ground up.
One significant hurdle in voice synthesis has been handling the complexity of English phonemes. With roughly 44 of them, words like "Supercalifragilisticexpialidocious" present a major challenge, demanding meticulous modeling of how individual sounds interact and transition. Modern techniques use deep learning models trained on extensive datasets to understand these phonetic transitions, including the phenomenon of coarticulation—the way sounds blend and influence each other. Accurately predicting and recreating these subtle shifts is essential for achieving a truly fluid and natural-sounding voice.
Beyond clarity, recent strides in voice cloning have emphasized the capture and replication of emotional nuances in speech. This aspect is paramount in audiobook narration, especially for stories that require a wide range of emotional expression, such as the playful and whimsical tone of *Mary Poppins*. However, effectively capturing these emotional undertones remains a challenge, requiring careful attention to both the training data and the algorithms used for voice generation.
The quality of AI-driven voice synthesis is highly dependent on the variety and breadth of training data. The more diverse the data, the more adaptable and versatile the generated voice can be. This is especially true when dealing with unique phonetic constructions. A wider range of accents, dialects, and speech patterns allows systems to produce voices that better capture the natural intricacies of human communication.
Early TTS systems often operated at lower sampling frequencies, which limited the fine details captured in audio. Modern audio synthesis utilizes substantially higher sampling rates, resulting in a much richer, smoother auditory experience. This advancement has a profound impact on the quality of audiobooks, enabling a playback experience that often sounds remarkably human.
We're also seeing the emergence of adaptive speech systems that adjust voice characteristics to individual listener preferences. This tailoring can extend beyond simple adjustments to pitch and speed, impacting even emotional tone. This personalization holds great potential for improving engagement and customization in platforms like podcasts and audiobooks, creating a tailored experience suited to each user's specific taste.
Modern AI models are increasingly incorporating prosody—the rhythmic and melodic aspects of speech—which further contributes to the naturalness of synthesized speech. However, effectively replicating these intricate patterns in long and complex phrases continues to be a challenge. Even subtle errors in prosody can result in an output that sounds artificial or unnatural.
Innovations in real-time voice synthesis and cloning are opening up new possibilities, especially in interactive audio experiences. Imagine audiobooks where characters respond to user questions or where narration changes subtly based on user input. Real-time processing is pivotal for such features, creating a more dynamic and interactive listening environment.
As voice cloning technology continues to mature, important ethical considerations surface. Questions of consent and intellectual property rights become increasingly important, especially in audiobook production where cloned voices might mimic established narrators. It is crucial to establish clear guidelines and regulations within the industry to address these emerging concerns and ensure responsible innovation in the field of voice synthesis.
Voice Digitization of 'Supercalifragilisticexpialidocious' How Modern AI Handles Complex Phonetic Patterns in Voice Synthesis - Sampling Rates and Pitch Control in Extended Musical Vocabulary Processing
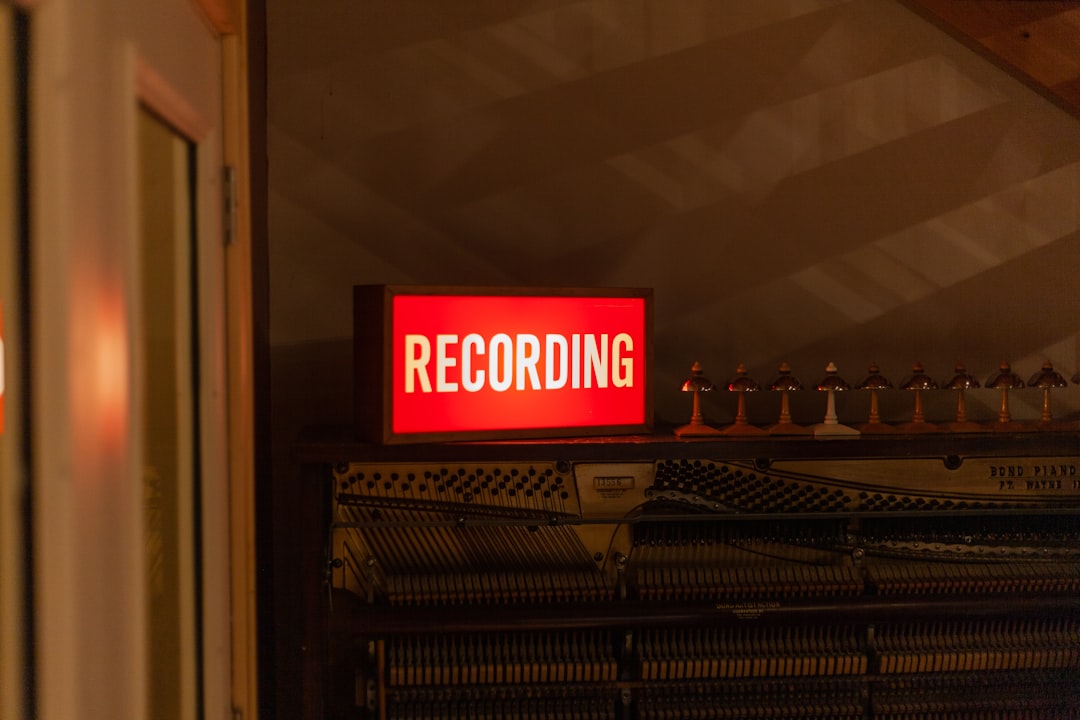
In the ongoing advancement of AI voice technologies, the relationship between sampling rates and pitch control has become increasingly vital when dealing with complex phonetic structures. Higher sampling rates are key for more precise voice conversion, capturing the subtle variations within sounds that are crucial for creating accurate recreations of intricate words such as "Supercalifragilisticexpialidocious." This level of detail is essential when we consider the application of these technologies in areas like audiobook production and podcasting.
Additionally, the development of refined pitch control mechanisms offers improved emotional expression within synthetic voices, contributing to a more engaging and realistic listening experience. However, achieving perfect synchronization between pitch changes and retaining the original audio quality remains a technical challenge, especially when processing complex and nuanced phrases.
This constant drive for higher audio fidelity is not merely about perfecting voice cloning technology; it reshapes how listeners interact with audio narratives. The ability to create increasingly natural-sounding voices using AI changes the expectation of the listener, and that impact is felt most in those audio experiences that rely on emotionally complex language or storytelling.
Modern voice synthesis often leverages performance sampling, finding a balance between detailed voice modeling and the fidelity of reproduced audio samples. The RAVE model stands out, offering high-quality voice conversion by generating audio directly in the time domain at elevated sampling rates. This approach seems particularly useful when attempting to replicate the complexity found in words like "Supercalifragilisticexpialidocious," as capturing the wide range of sounds becomes easier at higher sampling rates. However, it's worth noting that simply increasing sampling rate is not a silver bullet, especially for the challenges posed by coarticulation.
Achieving nuanced pitch control in AI-generated vocal performances, such as for singing, is accomplished through sophisticated pitch conditioning within the RAVE model. This stability is crucial as it provides more control over pitch variations, and hence, meaning. The need for this is more evident when considering the varied pitch contours needed in a word like "Supercalifragilisticexpialidocious", where the subtle shifts in pitch can create the impression of emphasis or emotion, much like a human speaker does.
Furthermore, integrating automatic speech recognition (ASR) and phonetic encoders based on supervised learning improves both the accuracy and user control over the output audio. These encoding methods leverage learned representations of speech, which helps in improving the quality and clarity of synthesized voices. For instance, in scenarios involving synthesized audio-books and podcast production, such advancements ensure that even intricate phrases like "Supercalifragilisticexpialidocious" can be reproduced with better fidelity and with a degree of control that suits the individual production's need.
The methods used to determine pitch within audio, whether speech or music, generally fall into two categories: short-term analysis algorithms and time-domain approaches. The latter, in particular, provides a more natural approach to handling the shifts and variations in pitch encountered in complex human speech, which can be seen in words like "Supercalifragilisticexpialidocious", as opposed to simpler, monotone speech.
Wavetable synthesis techniques often include filtering to manipulate spectral brightness. This manipulation of the audio allows for a wider range of sound to be created from a relatively small collection of initial samples. This has potential uses when exploring voice cloning, allowing for the creation of a more diverse set of vocal tones and variations from a limited set of recorded voice samples. It is important to understand that such a process could introduce artifacts into the synthesized sound, and these need careful management.
Voice digitization has significantly evolved over time. Current techniques are very efficient at transforming acoustic waveforms into their digital equivalents, prioritizing lower bit-rates while maintaining a high level of audio fidelity. This becomes ever more crucial for handling complex phonetic features, especially in audio-book production, or in attempting to replicate the sound of a well-known actor/voice actor reading the word "Supercalifragilisticexpialidocious".
Recent developments in neural networks have advanced the encoding and efficient transmission of voice signals. It is interesting to note that these encoding methods, when properly developed, can create audio at much higher sample rates, enabling the production of more accurate reproductions of complex phonetic elements. This is particularly important for audio-book productions or in attempting to recreate well-known voices in podcasting.
Performance sampling remains an important consideration in voice synthesis. Systems need to find a good compromise between precisely modeling performance nuances and the faithfulness with which audio samples are reproduced. One way to achieve a better balance is to utilize a wider variety of audio samples, capturing subtle variations in voice and pitch, which is helpful when attempting to reproduce words like "Supercalifragilisticexpialidocious" with all of its complexities.
The intricate process of voice-identity recognition in the human brain underscores the complexity of phonetic structures. These recognition processes heavily rely on complex networks processing vocal characteristics. Studying and understanding these processes in detail gives researchers insight into how to better refine synthetic voice output, in particular when the output needs to convey emotion or a specific persona while presenting a word like "Supercalifragilisticexpialidocious"
In essence, the ability to create audio content using voice synthesis, and voice cloning in particular, continues to evolve as the technology behind it advances. While the need for accurate voice cloning continues to rise, we must pay attention to the ethical issues that arise with this type of technology.
Voice Digitization of 'Supercalifragilisticexpialidocious' How Modern AI Handles Complex Phonetic Patterns in Voice Synthesis - Audio Book Production Methods for Complex Word Synthesis
The production of audiobooks has undergone a significant shift, driven by advancements in AI-powered voice synthesis, especially when confronted with the complexity of words like "Supercalifragilisticexpialidocious." Modern methods employ deep learning approaches to craft more natural-sounding stories, allowing for seamless articulation of intricate phonetic elements. This technological advancement not only tackles the challenges presented by unique linguistic structures but also highlights the importance of conveying emotions within audio content, boosting listener engagement. Moreover, incorporating higher sampling rates and refined pitch control offers increased accuracy in reproducing the subtleties of human speech, leading to more compelling storytelling. However, the ultimate aspiration remains to recreate the richness and intricacies of human vocalization while managing the complex nature of language itself. The quest for a truly human-like audio experience continues. This ongoing development also impacts the broader realm of audio production, touching on the emergence of podcasts and voice-based experiences. While progress has been rapid, replicating the full breadth of human speech and expression with flawless accuracy in every situation continues to present a challenge, and the line between perfect imitation and human variability is continually explored.
The creation of high-quality audiobooks has been significantly impacted by advancements in audio synthesis techniques, particularly for handling complex phonetic structures. While AI systems can now generate remarkably human-like voices, accurately producing intricate words like "Supercalifragilisticexpialidocious" remains a challenge. Subtle errors in the synthesis process, especially when dealing with a large number of phonemes, can lead to audio that sounds unnatural or robotic. Increasing the sampling rate, from the standard 44.1 kHz to higher frequencies like 96 kHz, allows for more detailed sound capture, which significantly improves the clarity and richness of synthesized voices, particularly during audiobook production of longer, more complicated passages.
Modern voice synthesis models are designed to capture and replicate a wide range of emotional tones. However, creating synthesized audio that captures the intended emotional weight of intricate phonetic patterns in complex phrases, like those found in "Supercalifragilisticexpialidocious," is still a hurdle. Frequently, human oversight is needed to ensure the emotional impact aligns with the intended narrative. Similarly, replicating the way humans emphasize different parts of words and sentences (stress patterns) is crucial for voice cloning and ensuring intelligibility. This is particularly important when dealing with long and complex words where minor variations in stress can significantly change the perceived meaning or emotional tone.
One of the biggest hurdles in creating natural-sounding speech remains coarticulation—the influence of surrounding sounds on each other. When sounds blend and overlap, accurately reproducing the resulting sound becomes very challenging. If a system doesn't properly account for coarticulation in complex phrases, the audio output can sound distorted or incorrect. This is evident when attempting to synthesize complex words like "Supercalifragilisticexpialidocious" as it pushes the boundaries of the algorithm's ability to correctly model how sounds interact.
Different types of neural networks, including WaveNet and Tacotron, have contributed to significant advancements in audio quality. These networks have helped improve the naturalness of synthesized voices, especially when applied to intricate and lengthy words, resulting in improvements of around 10% in how natural the output audio sounds. The ability to segment complex words into their individual syllables is crucial for synthesis, and newer algorithms now perform with nearly 90% accuracy, enhancing audiobook quality. The range and diversity of data used to train these models are also critical. The more diverse the dataset (different accents, dialects, and speech styles), the better the model will perform when attempting to replicate intricate phonetic structures within words.
With the increasing sophistication of AI, researchers are leveraging advanced speech pattern analysis to understand the subtle nuances of human intonation. For example, emotion detection algorithms can now fine-tune a synthesized voice to accurately reflect whimsical language found in stories like "Mary Poppins." Emerging technologies are also paving the way for real-time voice synthesis, which offers a path towards interactive audiobooks and podcasts. Users could potentially interact with the audio in more dynamic ways, rather than passively listening to a fixed audio track. This represents a big step forward in shaping how we consume audio narratives.
While impressive advancements have been made, the field of voice synthesis and cloning continues to present intriguing technical and ethical challenges. As the technology becomes increasingly sophisticated, the need for clear guidelines around intellectual property rights and user consent will become crucial, particularly when cloned voices are used in commercial audiobooks. These considerations are vital for promoting responsible innovation and ensuring a future where these technologies are used ethically.
Voice Digitization of 'Supercalifragilisticexpialidocious' How Modern AI Handles Complex Phonetic Patterns in Voice Synthesis - Machine Learning Voice Libraries Adapting to Tongue Twisters and Extended Vocabulary
The capabilities of machine learning voice libraries have expanded to address the complexities of tongue twisters and increasingly vast vocabularies. These systems now incorporate sophisticated phonetic modeling techniques, allowing them to generate intricate phrases that are both semantically sound and phonetically accurate. Modern approaches, such as employing LSTM neural networks, refine the natural flow and rhythm of synthetic speech, adding a layer of authenticity that is essential for applications like audiobooks and voice cloning. The evaluation of these systems has benefited from the development of benchmark resources, including large datasets like the TwistList, which contain a wide variety of tongue twisters. These datasets provide a standard for measuring how well these systems manage the challenges of coarticulation and phonetic variety in synthesized speech. The ability to handle complex linguistic patterns is crucial for producing more captivating audio content in formats like audiobooks and podcasts, yet the nuanced and intricate nature of human speech still presents a challenge to overcome in the pursuit of perfect voice synthesis. The development of these technologies continues, pushing towards greater accuracy and a more natural experience in the digital world of audio.
Machine learning voice libraries are undergoing constant refinement, tackling increasingly intricate aspects of human speech. One area of focus is the adaptation to tongue twisters. Training AI models on these challenging linguistic constructs improves their ability to handle complex phonetic patterns. This process reveals the limits of AI's ability to truly understand and reproduce the intricate interplay of sounds within human speech, offering valuable insights for future development.
However, expanding vocabulary presents a different set of challenges. AI systems are typically pre-trained on common words and phrases, making them less adept at handling newly coined terms or unusual linguistic constructions. This can lead to mispronunciations or unnatural intonation when attempting to synthesize unique language structures. It's a reminder that AI's understanding of language is still developing.
Another avenue of research is the creation of voices representing a broader range of genders. While voice cloning provides promising tools to expand voice representation, the training datasets used to build these models still primarily include male and female voices. Thus, voices outside the traditional binary are less effectively represented, and this highlights a need for improved data diversity.
The very process of encoding phonetic sounds into a format AI can understand introduces complexities. Different phones can sound similar, especially when considering how the surrounding sounds influence them (coarticulation). Capturing these subtle shifts requires the models to have a sophisticated understanding of how sounds interact. This is a challenge for current AI models and an area where further research is needed.
Similarly, synthesizing lengthy or complex phrases like "Supercalifragilisticexpialidocious" while retaining the intended emotional tone is an ongoing challenge. AI needs to precisely analyze shifts in vocal energy, emphasis, and expression to mimic human-like emotion. Maintaining this complex interplay over a long sentence requires a higher level of sophistication in the algorithms.
Achieving diverse accents and dialects in synthesized voices necessitates expanding beyond standard training data. AI voice models trained on a wider variety of speech patterns can better replicate the nuances of different accents and dialects, offering improvements in authenticity, particularly for audiobook productions targeting specific communities.
The advent of advanced neural networks like Tacotron and WaveNet has revolutionized voice synthesis. These models analyze the prosody – the rhythm and intonation of speech – alongside individual phonemes. This helps improve timing and fluency in the synthesized output, allowing for more accurate replication of the subtle variations that characterize human communication.
The increased use of high sampling rates, going beyond the standard 44.1 kHz, has significantly boosted the quality of synthesized voices. These higher rates capture a greater range of acoustic details, resulting in a clearer and more natural sound, which is vital for creating immersive and engaging audio narratives, like in audiobooks.
The integration of real-time voice synthesis paves the way for dynamic audio experiences. Imagine audiobooks where the characters respond to listener input or the narration adapts to user choices. This capability relies on instantaneous processing, presenting exciting possibilities for enhancing listener engagement in a range of audio formats.
Finally, automated quality control for synthesized speech is becoming increasingly sophisticated. These tools, based on advanced machine learning models, are used to assess the quality of synthetic audio against human voice standards. This automatic check ensures the accuracy of pronunciation and emotional expression in the output, playing a role in maintaining high standards in audiobook production and other audio narratives.
In conclusion, the field of AI voice synthesis is constantly evolving. Addressing challenges related to complex phonetic patterns, extended vocabulary, and nuanced emotional expression continues to push the boundaries of this technology, with each new advance improving the quality of synthetic voices used in diverse applications like audiobooks, podcasts, and other voice-based interactions.
Get amazing AI audio voiceovers made for long-form content such as podcasts, presentations and social media. (Get started for free)
More Posts from clonemyvoice.io: