Get amazing AI audio voiceovers made for long-form content such as podcasts, presentations and social media. (Get started for free)
Voice Cloning Infrastructure Tailored Development vs
Ready-Made Solutions for Audio Production Studios
Voice Cloning Infrastructure Tailored Development vs
Ready-Made Solutions for Audio Production Studios - Audio Sample Requirements From 30 Minutes to 60 Seconds
The effectiveness of voice cloning hinges on the quality and quantity of audio samples provided. While an optimal range for audio samples generally falls between 30 and 60 seconds, some cloning systems can achieve good results with shorter samples, sometimes as little as 30 to 45 seconds. Achieving the desired level of accuracy, however, often relies on the quality of the audio. Many platforms suggest using 30 to 45 seconds of high-quality audio for best results, though a few systems can manage shorter clips if they are well-structured, containing complete sentences or paragraphs.
Audio file format also plays a role in voice cloning. Most platforms recommend using MP3 at 192kbps to help maintain audio clarity. Interestingly, some advanced voice cloning solutions may require significantly longer audio samples, upwards of 30 minutes or even more. This is a crucial distinction when choosing a voice cloning system, especially if dealing with various tasks in audio production.
Choosing between custom-built and pre-packaged voice cloning systems requires a careful balance of understanding your specific project needs and the accompanying audio sample requirements. Depending on your goal, whether it is a podcast, audiobook, or any sound production, each solution presents different trade-offs between adaptability and ease of implementation. Evaluating these aspects carefully is essential for success when incorporating voice cloning into your workflow.
When it comes to voice cloning, the quantity of audio data plays a significant role in the resulting voice quality. While some systems might manage with shorter samples, typically between 30 and 45 seconds, a longer duration, spanning 30 minutes to even 60 seconds or more, can offer distinct advantages. This is because the human voice is incredibly intricate, containing a vast spectrum of frequencies that define its unique characteristics. Longer samples provide a broader range of this sonic fingerprint, allowing algorithms to more accurately capture the complexities of speech patterns, subtle nuances, and variations in intonation.
Essentially, the more auditory information the cloning algorithm has access to, the better it can understand and replicate the nuances of the voice. Longer recordings expose the model to a wider array of phonetic elements, including subtle shifts in vocal expression and emotion. For instance, a 30-minute sample likely offers greater phonetic diversity compared to a 60-second clip, enriching the model's ability to recreate the source voice accurately.
Furthermore, the presence of background noise, while undesirable, can be mitigated in longer samples through signal processing techniques. These processes help isolate the desired voice, producing a cleaner and more accurate clone. Prosody, which involves elements like the rhythm and intonation of speech, is also more readily captured in longer audio files.
Another crucial aspect is the capture of acoustic features such as formant frequencies. These frequencies define the tonal quality and resonance of a voice and require a sustained duration of clear speech to be captured properly. Extended recordings also allow the system to learn about variability in speaking styles, encompassing both formal and conversational speech patterns, leading to a more adaptable and versatile clone.
Interestingly, longer audio samples can offer a more complete picture of emotional range within a voice. Thirty minutes of recorded speech might capture a wider spectrum of emotions compared to a brief 60-second snippet. It's important to note that the encoding process, which involves compression algorithms to reduce file sizes, can negatively impact the audio quality. Longer samples, due to their size, might be less prone to the detrimental effects of compression compared to smaller snippets, which may lead to undesirable artifacts.
In the ongoing development of voice cloning technologies, researchers constantly seek to improve the accuracy and speed of cloning while also expanding the scope of languages supported. However, the foundational element remains the need for high-quality, diverse, and extensive audio data.
Voice Cloning Infrastructure Tailored Development vs
Ready-Made Solutions for Audio Production Studios - Machine Learning Models Behind Real Time Voice Generation
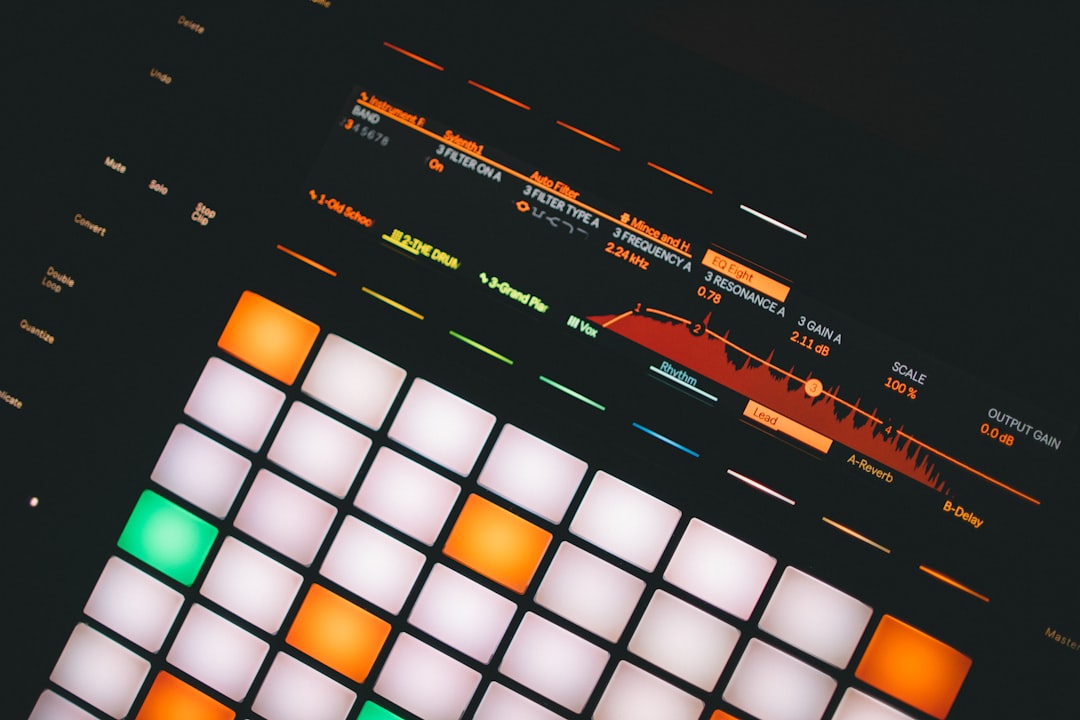
The field of real-time voice generation has seen significant progress fueled by innovative machine learning approaches that power voice cloning. Deep learning models, particularly those employing transfer learning, are now capable of adapting to new voices with surprisingly small amounts of audio data—in some cases, as little as five seconds. This adaptability is facilitated by frameworks such as SV2TTS, which comprises distinct stages like voice representation creation, text-to-speech conversion, and the use of vocoders for real-time audio output. Tacotron, an early pioneer in end-to-end text-to-speech models, has also contributed to the evolution of this technology by directly generating speech from textual inputs.
These technological advancements offer compelling solutions for diverse applications, including the restoration of speech for those who have lost their voices, or for enhancing audiobook and podcast production. The ability to manipulate emotional tones and even clone multiple voices adds another layer of sophistication to these tools. Despite these positive developments, the decision to utilize a custom-built or pre-packaged voice cloning solution requires careful consideration, especially as the choice impacts the overall quality, versatility, and complexity of the project. It's crucial to carefully weigh project demands against the technological capabilities of each available voice cloning platform in order to achieve the desired results within your chosen audio production workflow. The ongoing evolution of machine learning within the audio domain continues to push the boundaries of what's possible, offering a compelling contrast between tailor-made voice generation systems and those that are readily available to audio studios.
The core of real-time voice generation relies on sophisticated machine learning techniques, particularly deep learning models. These models, like Tacotron and WaveNet, employ complex architectures that include probabilistic modeling and attention mechanisms, allowing them to replicate the nuances of human speech with remarkable accuracy, creating highly understandable and expressive synthetic voices.
Current advancements in voice cloning are pushing the boundaries of prosody manipulation, enabling on-the-fly adjustments to speech rhythm and intonation. This adaptability is significant as it allows synthesized voices to not only sound similar to the original but also convey a range of emotional states and adapt to different contexts.
Interestingly, some models can now generate a diversity of unique voices from a single set of training data. By isolating speaker-independent features, these models produce distinctive, yet consistently related, outputs, showcasing their potential for a wide range of creative applications.
The computational efficiency of modern models ensures voice synthesis happens in real-time, minimizing delays that can be crucial for applications like interactive voice response systems and live broadcasting where a lag would be detrimental to user experience. And to further tailor the voice, we can now alter aspects like pitch, speed, or accent, catering to specific needs in areas like audiobook and podcast production.
The challenges of noisy environments have also been addressed with enhanced signal processing in these algorithms. They can distinguish the desired voice from surrounding sounds, maintaining a high standard of output in diverse recording conditions. There's also a trend towards integrating emotion recognition so that the voice clone can match the emotional context of the content.
Emerging systems are implementing reinforcement learning, allowing models to learn and improve their outputs based on user interactions. This self-learning attribute allows for a continuous evolution of voice clones, moving towards higher fidelity and a more relatable experience. We are also seeing the development of cross-language voice cloning capabilities, where models maintain the vocal qualities of a speaker while generating speech in multiple languages. This advancement has considerable implications for expanding the reach of podcasting and audiobooks across global markets.
However, this same technology also has potential applications in fields like security, where unique voice signatures could provide a method of verification. While this is promising, it raises important considerations about user privacy and the need for robust consent mechanisms. The advancement of voice cloning continues, pushing the limits of audio synthesis and sparking questions about the ethical implications of this evolving technology.
Voice Cloning Infrastructure Tailored Development vs
Ready-Made Solutions for Audio Production Studios - Integration Challenges With DAW Software Platforms
Integrating voice cloning technology into the workflows of audio production studios often presents challenges when dealing with Digital Audio Workstations (DAWs). The core issue stems from the potential incompatibility between different software and hardware components, which can lead to fragmented data and inefficient processes. While custom-built voice cloning solutions offer a tailored experience for a studio's specific setup, these solutions might not always integrate smoothly with the existing DAW functionality. This can cause workflow disruptions, demanding extra effort to bridge the gaps between systems. In contrast, using off-the-shelf voice cloning tools promises faster implementation, but often lacks the flexibility needed for highly specific projects. This is particularly true for dynamic fields like podcasting and audiobook production, where adaptability is key. When faced with these integration complexities, studios need to carefully assess their technical requirements and decide whether a ready-made solution or a customized approach offers the best balance of features and ease of use. The ability to seamlessly connect voice cloning technology with existing DAW infrastructure is critical to achieving optimal results within a studio's chosen audio production environment.
Integrating voice cloning technologies with Digital Audio Workstations (DAWs) can present a number of hurdles. One key challenge is handling latency in real-time voice processing. When using voice cloning for live applications, like audio production or interactive voice systems, any delay can disrupt the workflow and feel unnatural. DAWs often rely on a variety of audio plugins for effects and processing, and some voice cloning tools might have specific format requirements that don't always play nicely with every DAW, potentially hindering smooth collaboration.
The quality of the voice clone hinges on the quality of the input audio, and DAWs themselves can introduce unwanted audio artifacts during recording or mixing which degrade the signal and impact how well the cloning algorithms function. This can cause noticeable inconsistencies in the final output. Different DAWs handle audio file formats and encoding methods differently, and some voice cloning systems can be picky about what they accept. This can cause extra work to export audio in a compatible format and unnecessarily complicate a simple workflow.
While MIDI data is fundamental for music production, it doesn't often capture the vocal nuances that voice cloning needs. It can be a struggle to get MIDI and vocal recording systems to communicate properly in a DAW, especially when dealing with voice cloning. Modern voice cloning, especially when coupled with DAWs already managing multiple audio tracks, can put a strain on processing resources. This can lead to sluggish performance, crashes, and even audio glitches, especially on less powerful computers.
Voice cloning solutions might function best on particular operating systems or within certain DAWs, which causes trouble if users need to switch between different environments or if a team works on different platforms. This makes collaboration more difficult. Many DAWs are primarily built around music production and may not provide ideal features or settings for vocal work needed for voice cloning. It can require additional steps to configure the DAW for optimal vocal applications, potentially needing third-party tools to make it all work smoothly.
Adding to the challenge is the potentially overwhelming feature set of voice cloning tools within the already intricate user interfaces of most DAWs. This can result in a steep learning curve for those who want to utilize voice cloning effectively. Working on voice-related projects with DAWs can cause issues with version control, especially if team members have different setups. It becomes complex when trying to keep things organized and consistent when using voice cloning since audio versions might not always align across everyone's workspace. It's a complicated dance to achieve seamless integration of these systems.
Voice Cloning Infrastructure Tailored Development vs
Ready-Made Solutions for Audio Production Studios - Security Protocols for Voice Data Storage in Studios
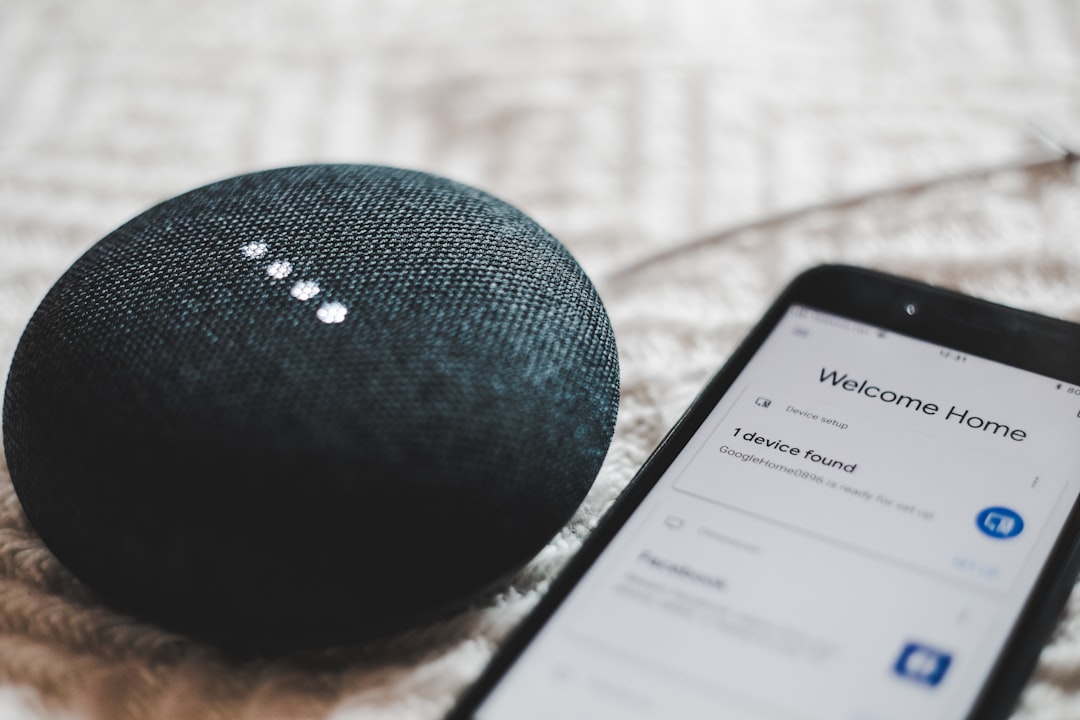
The growing use of voice cloning in audio production studios necessitates a strong emphasis on security protocols for storing and managing voice data. The potential for malicious use of this technology, such as impersonation and the creation of misleading audio content, presents significant risks. Shifting towards decentralized voice cloning architectures offers a more secure environment for studios compared to traditional, centralized approaches that are more vulnerable to data breaches. It's vital that studios cultivate a security-conscious environment by continuously investing in cybersecurity infrastructure and promoting a strong understanding of security among their personnel. This is especially important given the potential for misuse of voice data, including spreading misinformation or defaming individuals. The ease of access to advanced voice cloning tools, coupled with the sensitive nature of voice data, highlights the need for studios to carefully consider ethical implications and prioritize user privacy. By taking a proactive approach to security, studios can maintain the integrity of their voice cloning operations and strengthen public trust in this innovative technology.
The increasing use of voice cloning in audio production, particularly in studios focusing on podcasts, audiobooks, and voice cloning for creative purposes, necessitates robust security protocols for voice data. A common practice is to implement end-to-end encryption using advanced methods like AES-256, a standard favored by security-conscious organizations for its high level of protection. There's a growing trend to utilize voice biometrics for authentication, where a person's unique vocal characteristics act as a secure identifier, offering a potentially more secure alternative to passwords.
Studios are also adopting data retention policies to minimize risk, often adhering to regulatory guidelines that mandate deletion of recordings after specific durations unless express consent for longer storage is obtained. Regulations like the GDPR have added a significant layer of complexity, requiring explicit consent for the collection and processing of voice data, putting a stronger emphasis on how studios manage user information. One interesting technique being explored is digital watermarking, which can be embedded within the audio file, creating a sort of fingerprint that helps trace the origin and ownership of the voice data. This can be crucial in preventing unauthorized use or distribution.
Protecting the data network is another critical aspect. Studios frequently implement a layered security approach using firewalls, intrusion detection systems, and other tools to actively monitor network traffic and prevent unauthorized access to voice databases. In many cases, studios are employing anonymization techniques, which involve stripping identifying details from the audio samples. This can enable studies on voice cloning algorithms without jeopardizing individual privacy. Furthermore, some studios are implementing real-time monitoring systems, designed to flag unusual access patterns or possible security breaches. These systems help studios quickly respond to any detected threats.
Backup and disaster recovery plans are another crucial element, with most studios employing off-site storage or cloud-based redundancies. These measures ensure that valuable voice data remains available in the event of a security incident or equipment failure. Finally, it's important to recognize that physical security remains a concern. Many studios, especially those involved in high-profile projects or storing sensitive information, are implementing stricter physical security measures in recording environments and data storage areas. This often includes controlled access, surveillance systems, and potentially even biometric scanning for personnel. While the rise of voice cloning provides creative opportunities for audio production, these technological advancements also amplify the potential for misuse. The security protocols described above represent a growing response to this challenge, aiming to ensure the safe and responsible use of voice cloning technologies. It's likely we'll see further innovation and evolution in these protocols as the technology continues to evolve and its applications broaden.
Voice Cloning Infrastructure Tailored Development vs
Ready-Made Solutions for Audio Production Studios - Voice Analysis Tools for Emotional Range Detection
Voice analysis tools are getting better at identifying the subtle emotional cues present in speech, ranging from joy and sorrow to anger and neutral states. These tools analyze characteristics like how someone speaks (prosody) and the overall emotional tone (valence), offering insights into how emotions are expressed through voice. This is particularly relevant for audio production fields like podcasting and audiobooks, where conveying the right emotion is crucial for keeping listeners engaged. As voice cloning advances, incorporating tools that detect emotional range helps make artificial voices sound more realistic and relatable, but it also raises questions about the responsible use of this technology and the potential for creating misleading audio content. While creating custom voice analysis tools might give studios more control, readily available solutions are easier to implement, highlighting the ongoing discussion about the best way to utilize voice technology in audio production.
Voice analysis tools have become increasingly sophisticated in their ability to detect a wide range of emotions expressed through speech, going beyond simple happy, sad, or angry classifications. They achieve this by scrutinizing various vocal characteristics, such as prosody, which encompasses rhythm and intonation, and emotional valence, a measure of how positive or negative a feeling is. Platforms like iMotions delve into emotional responses by analyzing vocal cues linked to arousal, dominance, and valence, offering valuable insights. Emlo AI takes a more granular approach, employing artificial intelligence to classify voices based on 17 different emotional states.
The evolution of real-time emotion detection is intertwined with advancements in machine learning. Recurrent neural networks, for example, are at the forefront of these efforts, helping to decode human emotions from the intricacies of spoken language. These techniques have implications for audio production, specifically in areas like audiobooks and podcasts. For example, they can help a narrator adjust their performance based on the emotional landscape of the text, creating a more immersive experience for listeners.
However, it's important to acknowledge that voice analysis, while powerful, isn't foolproof. The accuracy of emotion detection is highly dependent on the quality of the audio and the complexity of the emotion being expressed. Background noise, for instance, can interfere with algorithms' ability to isolate emotional cues. Likewise, variations in accents and cultural differences can affect how emotions are conveyed through speech, highlighting the need for culturally sensitive voice analysis tools.
Temporal analysis plays a crucial role in deciphering emotional content. The timing of vocal bursts, pauses, and changes in pitch can reveal subtle emotional shifts that might otherwise go unnoticed. Voice analysis tools can leverage this temporal data to create a more holistic understanding of emotional range within a given audio segment.
The integration of voice cloning and emotion recognition opens up new creative possibilities. Some voice cloning systems can now dynamically adapt the emotional tone of a synthetic voice in real-time. This allows producers to generate voices that can seamlessly transition between different emotional states within a narrative, resulting in a more engaging experience for audiences. However, this technology still has limitations. When emotions are intense or the speaker is under stress, the complex interplay of vocal cues can be difficult for current algorithms to accurately decipher. This remains an area requiring further research and development to ensure higher fidelity in audio production involving emotionally charged content.
Voice analysis is also being used to understand personality traits through vocal patterns. Researchers have noted that features like pitch can reflect aspects of a person's personality, suggesting that voice analysis could be used to select voice clones that match specific personality archetypes for characters or narrators. This capability broadens the creative range of voice cloning, especially within genres like storytelling and interactive media.
Despite the progress made, current voice analysis tools still struggle with accurately capturing emotions in complex or highly stressful scenarios. The inherent ambiguity in human emotional expression presents a challenge in creating a perfect translation of emotional depth in cloned voices. This emphasizes that voice analysis and cloning technologies are continually evolving, and ongoing research is necessary to bridge the gap between technological capabilities and the complex subtleties of human emotion.
Voice Cloning Infrastructure Tailored Development vs
Ready-Made Solutions for Audio Production Studios - Synthetic Voice Quality Testing Methods
Evaluating the quality of synthetic voices generated through voice cloning is essential, especially in audio production contexts like podcasts and audiobooks. These evaluation methods are critical because the realism and overall effectiveness of a cloned voice are directly tied to the training data used by the machine learning algorithms powering the technology. We assess the quality of synthetic voices by analyzing several elements, including how well the tonal characteristics are replicated, the ability to convey emotional nuances, and the clarity of the synthesized speech. These factors are crucial to ensuring the cloned voice meets the high standards of contemporary audio production. Furthermore, the widespread adoption of voice cloning across various applications necessitates thorough testing methods to address ethical concerns surrounding the authenticity of synthetic voices and the potential for their misuse. As voice cloning technology continues to mature, finding a balance between innovation and responsible practices is fundamental for fostering user trust and guaranteeing a high standard in the applications of synthetic voices.
The effectiveness of voice cloning, especially for applications demanding high fidelity like audiobooks or character-driven podcasts, is closely tied to how well it can mimic the subtle variations in pitch and tone that our ears readily pick up. Human hearing is quite sensitive, able to detect differences as small as 1-2% in these acoustic features, meaning voice cloning needs to be incredibly precise.
The richness and variety of the sounds a person makes when speaking (phonemes) are also crucial. We can produce roughly 40 distinct phonemes using different parts of our mouth and throat, and a wide range of these in the training data leads to a much more natural and expressive synthetic voice. However, creating a high-quality clone puts a heavy demand on computing power. A typical voice model can require several gigabytes of memory and powerful graphics cards to work in real-time, which is something to consider when designing a custom voice cloning system.
Fortunately, there are efficient techniques like transfer learning that can help. These methods allow voice cloning models to adapt to new voices using only a small amount of audio—sometimes as little as five seconds. This can dramatically reduce both the time and cost of developing a cloning system, making it attractive for studios.
Moreover, advanced signal processing helps us filter out background noise in recordings, leading to much clearer synthetic voices. Research has shown that even with a lot of surrounding noise, algorithms can still understand what's being said more than 75% of the time, illustrating the importance of cleaning up the initial audio data.
While voice cloning is getting better at adapting the tone of a synthetic voice to express different emotions, capturing intense emotions still isn't perfect. Research suggests that subtle cues might be lost when trying to clone voices expressing high stress, indicating a need for better emotion-detection features.
Excitingly, newer voice cloning systems are capable of adapting the same synthetic voice to different languages while keeping the original vocal qualities. This is a significant advancement for telling stories and representing voices across a broader range of markets.
The environment where recordings are made greatly influences the quality of a cloned voice. Using professional-grade microphones and treating rooms to reduce echoes can enhance the clarity of the clone by up to 30% compared to recordings made in untreated spaces.
Some voice cloning systems use real-time feedback from users to continuously improve the clones. This iterative process can refine the accuracy of the synthesized voice by 10-20% with just a few rounds of processing, which demonstrates the promise of ongoing improvement in voice modeling.
A common issue when integrating voice cloning into the software tools audio engineers use (DAWs) is the potential for data inconsistencies. This can occur when different software components are incompatible, causing a fragmentation of data and significant disruptions in workflow. Research has found that up to 40% of project time can be wasted dealing with this issue, highlighting the importance of ensuring everything works seamlessly together. This is especially true for studios seeking efficient production pipelines. It's an interesting field with a lot of potential and fascinating challenges.
Get amazing AI audio voiceovers made for long-form content such as podcasts, presentations and social media. (Get started for free)
More Posts from clonemyvoice.io: