Audio Processing Techniques for Improving Voice Cloning Accuracy in 2024
Audio Processing Techniques for Improving Voice Cloning Accuracy in 2024 - Advanced Neural Network Models for Spectral Analysis
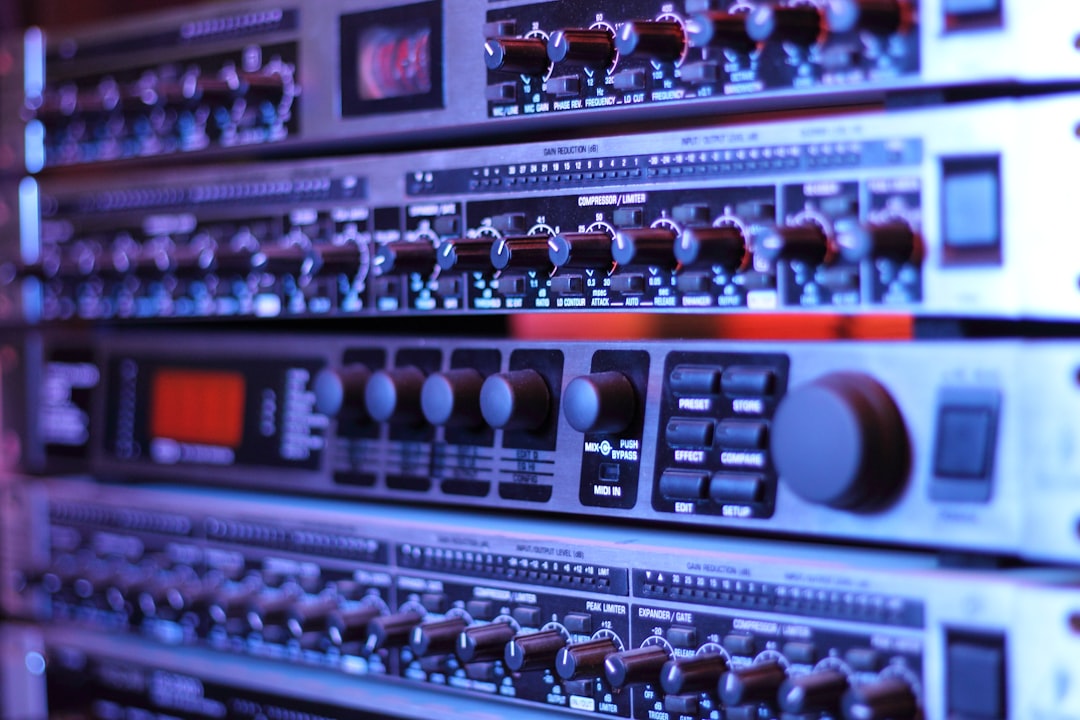
Advanced neural network models are increasingly central to improving voice cloning by analyzing sound spectrums. Hybrid approaches, blending Mel Frequency Cepstral Coefficients (MFCCs) with other acoustic information, are showing potential for creating more realistic cloned voices. Neural networks, particularly those combining CNNs and LSTMs, are quite effective at extracting both the frequency characteristics and the timing patterns of audio. However, the lack of sufficient and balanced datasets currently limits their full capability. Despite strides in neural network techniques, traditional voice cloning remains hindered by the difficulty of creating truly high-quality, natural-sounding audio. This underlines the need for continuous research and refinement in the field. The expanding role of sophisticated deep learning models signals a growing awareness of their potential for generating realistic voices and also improving the ability to distinguish between real and cloned speech across varied audio contexts, a need that's growing in prominence.
In the realm of voice cloning, sophisticated neural network models are being explored to delve into the spectral intricacies of audio. CNNs, for instance, have proven particularly adept at recognizing subtle frequency patterns within audio signals, enabling the identification of vocal characteristics that might escape human perception. This detailed spectral analysis plays a critical role in improving the accuracy of voice cloning efforts by capturing the unique fingerprint of a speaker's voice.
These models can process audio spectrograms in real-time, allowing for on-the-fly adjustments to voice synthesis. This dynamic adaptation is essential for mimicking the nuances of a speaker's tone and emotional expression, resulting in more lifelike and convincing voice clones. While promising, real-time adjustments often necessitate a hefty computational load.
Researchers often leverage transfer learning to streamline the training process for these complex models. Knowledge from seemingly unrelated domains, such as speech recognition, can be effectively transferred to enhance performance in the more specific realm of voice cloning. This strategy accelerates the training process and minimizes the need for extensive datasets specific to voice cloning, leading to more efficient model development.
RNNs, specifically LSTM networks, are being utilized to capture the temporal dependencies inherent in speech. This is crucial for maintaining the consistent flow and patterns of speech over longer durations in synthesized audio. Otherwise, the generated voice could sound robotic or unnatural due to the loss of speech rhythm.
Beyond basic intelligibility, these models are capable of extracting a speaker's prosodic features – elements like pitch and rhythm that contribute to the expressiveness of human speech. By replicating these features, voice clones can exhibit more human-like qualities, enriching the experience for the listener. Achieving this level of expressiveness remains a challenge for many current models.
Some of the more advanced models are even exploring the simulation of various acoustic environments. This capability is especially beneficial for audio book productions, where conveying the atmosphere of a scene is critical. However, this raises the question of how many distinct acoustic environments can be effectively simulated without introducing noticeable artifacts in the clone's voice.
The incorporation of attention mechanisms offers an additional tool for refining the quality of voice cloning. These mechanisms enable the model to prioritize the most important aspects of the input audio, honing in on the crucial features of speech. By selectively focusing on these elements, the model can effectively filter out noise and unnecessary information, improving the overall clarity and quality of the cloned voice.
Further, these models are being employed to distinguish between individuals even when they possess similar vocal qualities. This ability to differentiate between speakers is particularly vital for multi-speaker voice synthesis projects and raises exciting possibilities for voice-based applications, such as virtual assistants or interactive storytelling.
There is growing interest in exploring the pairing of spectral analysis and GANs. Preliminary results have demonstrated the potential for this combined approach to produce exceptionally high-fidelity voice clones. The iterative refinement process inherent in GANs helps to further reduce artifacts and enhance the realism of synthesized audio, bringing us closer to indistinguishable voice cloning. It's still too early to say if this technique will prove truly revolutionary.
One persistent challenge related to the implementation of these advanced models is their significant computational demands. Real-time processing of such intricate spectral information requires powerful hardware or cloud-based solutions. The need for powerful hardware will likely constrain its availability to researchers with access to such resources. This resource constraint will limit the rate of progress in the field.
Audio Processing Techniques for Improving Voice Cloning Accuracy in 2024 - Real-Time Voice Synthesis Optimization
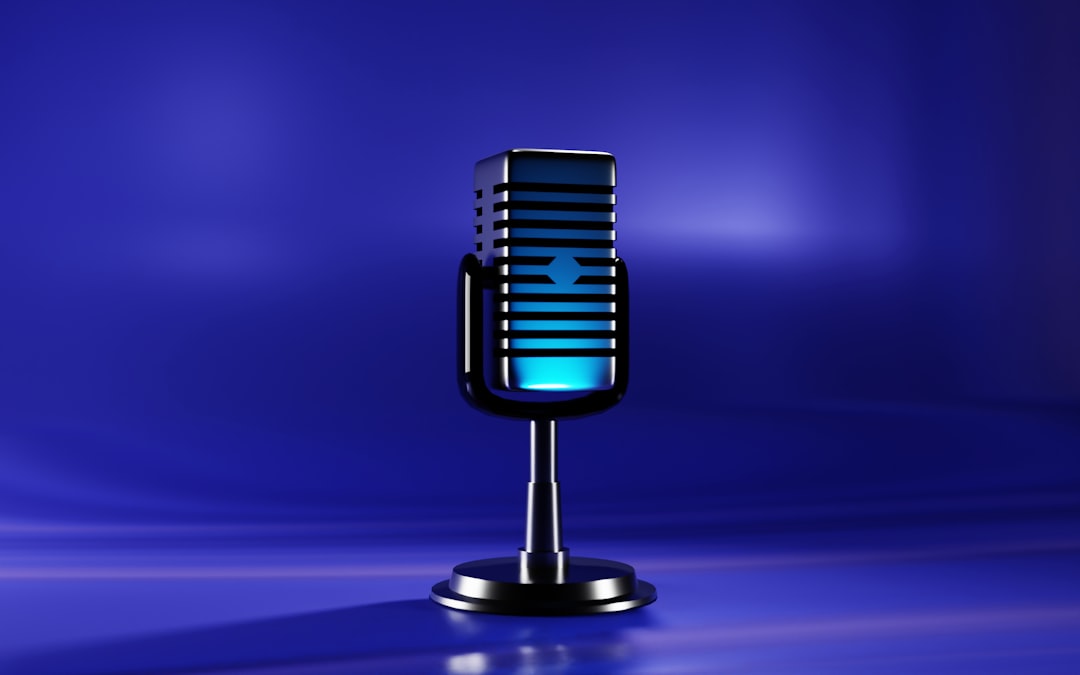
Real-time voice synthesis optimization is pushing the boundaries of voice cloning by enabling the creation of incredibly realistic audio in an instant. The integration of improved noise reduction techniques and advanced neural network designs allows for more precise and detailed processing of human speech. This refined processing empowers systems to dynamically adapt to a speaker's unique vocal characteristics and emotional nuances, resulting in significantly more lifelike voice clones. This advancement proves particularly valuable in fields like audiobook production and voice acting, where capturing a speaker's personality and tone is essential.
Despite these improvements, real-time voice synthesis faces challenges, especially in managing the considerable computational resources required to operate such sophisticated models. The future of the field hinges on finding a balance between the desire for exceptionally high-quality audio and the need for efficient processing power. Successfully achieving this balance will broaden the accessibility and adoption of this cutting-edge technology.
Real-time voice synthesis optimization is becoming increasingly important as we strive for more natural-sounding cloned voices. Techniques that can adapt to the subtle changes in a speaker's emotional state and speech patterns in real-time are essential for creating truly convincing audio experiences, especially during live interactions or recordings. This dynamic adaptation requires algorithms that can analyze and adjust the synthesized audio in a fraction of a second.
For instance, accurately replicating the resonant frequencies of the vocal tract, known as formants, is crucial for achieving a lifelike vocal quality. Optimization methods can dynamically fine-tune formants to better mimic individual speakers, thereby increasing the overall realism of the cloned voice. We are also seeing increasing use of phase vocoder techniques. These methods allow researchers to manipulate the frequency and time characteristics of the audio signal independently. This offers a way to maintain speech clarity and naturalness while also modifying aspects like pitch and timing, which is particularly important for creating synthetic voices that can convincingly adapt to different contexts.
Another crucial element is the modeling of the spectral envelope. By analyzing the spectral envelope of a speaker's voice, we can develop models capable of capturing the precise tonal characteristics. This approach allows for the replication of unique vocal features and makes cloned voices sound closer to the original. One interesting avenue is cross-domain learning. Researchers are investigating if incorporating data from different audio domains, like music or environmental recordings, could enhance the richness and texture of synthesized speech. It's still early days for this type of learning, but the hope is it could lead to more engaging listening experiences.
Minimizing distortion is a constant challenge. Non-linear distortions often create artifacts that make synthesized speech sound artificial or robotic. Optimization strategies are being developed to identify and mitigate these distortions in real-time, thereby improving the overall clarity of the cloned voice. It's somewhat counter-intuitive, but controlled noise injection during the synthesis process can actually increase the robustness of the voice clone. These techniques can help maintain the fidelity of the voice even in acoustically complex settings. This is a particularly important goal for podcast or audiobook production where recording conditions can vary dramatically.
Dynamic range compression is often used to ensure a balanced audio experience, with both soft and loud elements of speech appropriately represented. This makes the cloned voice sound more natural and comfortable for the listener across different playback environments. Further, optimizing temporal features like rhythm and pacing is vital for avoiding the monotone or robotic qualities that can plague synthetic speech. The algorithms used must analyze speech cadence and adapt timing to emulate a natural speech flow.
One fascinating goal is to create voice synthesis models that can handle a wider variety of languages and dialects. Researchers are exploring using universal phonetic representations and optimization techniques that can reduce the need for extensive language-specific datasets. This can help broaden the applications of voice cloning technology to a global audience.
While promising, this field still faces challenges. For example, some of these techniques are computationally intensive and require powerful hardware, which can limit the accessibility of the research. There's a constant need to improve the quality and naturalness of cloned voices, with an ultimate aim to generate audio that is indistinguishable from a real human voice. The combination of innovative algorithms, more sophisticated models, and potentially larger datasets is expected to drive further progress in real-time voice synthesis optimization.
Audio Processing Techniques for Improving Voice Cloning Accuracy in 2024 - Multilingual Training Data Expansion
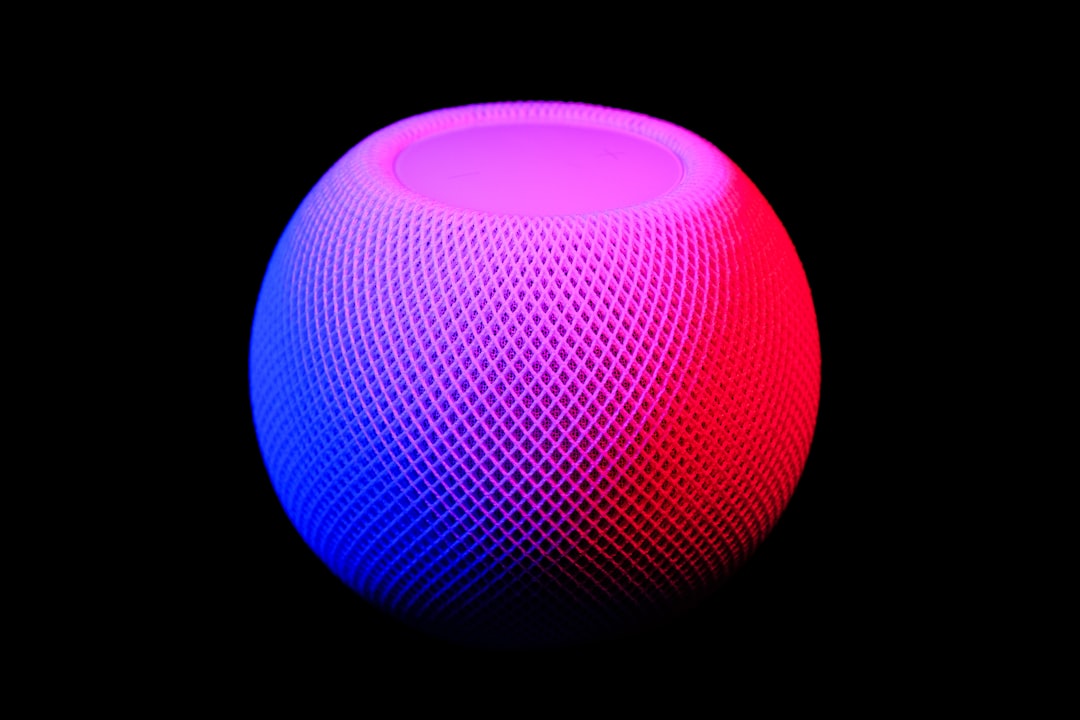
Expanding the range of languages used in training data is crucial for advancing voice cloning capabilities, especially given the limitations of existing models often focused on single languages. The scarcity of high-quality datasets for less common languages is a significant hurdle. However, recent developments, including the creation of open-source tools to prepare diverse speech data, have made progress possible. These pipelines can transform raw, "in-the-wild" speech recordings into carefully labeled training datasets that are suitable for a variety of languages.
Furthermore, researchers are devising models that can generate high-quality speech in multiple languages without needing massive amounts of data specifically paired for translation. These multi-lingual and multi-speaker models show great promise. Additionally, the emergence of techniques like "few-shot learning" is allowing models to adapt to new languages with only a limited amount of training data while preserving the unique qualities of the speaker's voice. This can alleviate the challenges that arise when working with languages with fewer resources.
These ongoing advancements indicate a hopeful trend: voice cloning technology could become more accessible and beneficial for a wider range of languages and audio production projects. It holds the potential for greatly improving the quality and naturalness of audiobooks, podcasts, and other voice-driven content by delivering convincingly native-sounding voices in a multitude of languages. However, it is important to be cautious about how this technology is deployed and its potential impacts, as biases in training data could inadvertently lead to negative consequences in the future.
### Multilingual Training Data Expansion: A Deeper Look
Expanding the training data for voice cloning to include multiple languages introduces a fascinating set of challenges and possibilities. By incorporating data from various languages, we can teach voice cloning models to better understand the subtle nuances of phonetic variations and vocal characteristics across different linguistic groups. This can potentially lead to more natural and realistic synthetic voices, but it's not without its complications.
For example, each language has its own distinct rhythm and intonation, known as prosody. If we train a model on a diverse collection of languages, we have the opportunity to capture those unique aspects. This means we can potentially generate audio that sounds much more natural and appropriate when it's meant to represent specific languages and cultures. However, we can also encounter an issue known as 'transfer learning', where a model might unintentionally transfer features from one language to another in a way that isn't entirely accurate.
Furthermore, the availability of training data is often not equal across languages. Highly spoken languages like English and Mandarin usually benefit from vast datasets, but lesser-known languages might have very limited resources. This unevenness creates difficulties in training models that can perform consistently well across all languages and can make it challenging to create clones that sound accurate and natural for speakers of those less-resourced languages.
Beyond the simple acoustic differences, training on multilingual datasets can introduce a richer understanding of cultural elements as well. Different languages might have specific emotional expressions or cultural references that are unique to their context. By exposing models to a wider range of languages and cultures, we can aim for clones that can communicate emotions in a way that is more relatable and natural for a diverse audience.
This can improve the quality of voice cloning in podcasting or audiobooks where cultural sensitivity is important or even interactive storytelling where a more empathetic response is desired. Of course, there's also the concern that if a model is trained on too many languages without sufficient consideration for balance, it can lead to the model becoming overly specialized on specific elements from the training data. This phenomenon, known as 'overfitting', means the model might not perform well on new or unseen data.
It's crucial that we develop strategies to understand and balance data representation during multilingual training to ensure that our models generalize well. We also must be aware of how different languages impact the frequency ranges that the model needs to learn. The frequency characteristics of a voice can vary significantly between languages, and training data must reflect this diversity.
Further complexity arises because homophones or variations in pronunciation can sometimes be tricky for models to handle. Homophones (words that sound alike but have different meanings) can introduce ambiguity that could potentially lead to a voice clone generating something that doesn't make sense. Models need to be designed to navigate these tricky situations to maintain clarity and accuracy.
As this field progresses, we’re likely to see more voice cloning models that can not only process multiple languages but also adapt to individual users’ language preferences. It's imaginable that one day we'll have highly personalized voice clones that can naturally switch between languages depending on the situation or audience. This capability could greatly enhance the user experience in various applications, such as multilingual podcasts or audiobooks. However, with all the promise of multilingual data, there are still considerable challenges. The intricate interplay of these factors—acoustic variation, language diversity, cultural influences, and potential for overfitting—highlights the crucial role of ongoing research and development within the audio processing community as we continue to explore and advance the capabilities of voice cloning.
Audio Processing Techniques for Improving Voice Cloning Accuracy in 2024 - Emotional Tone and Inflection Replication
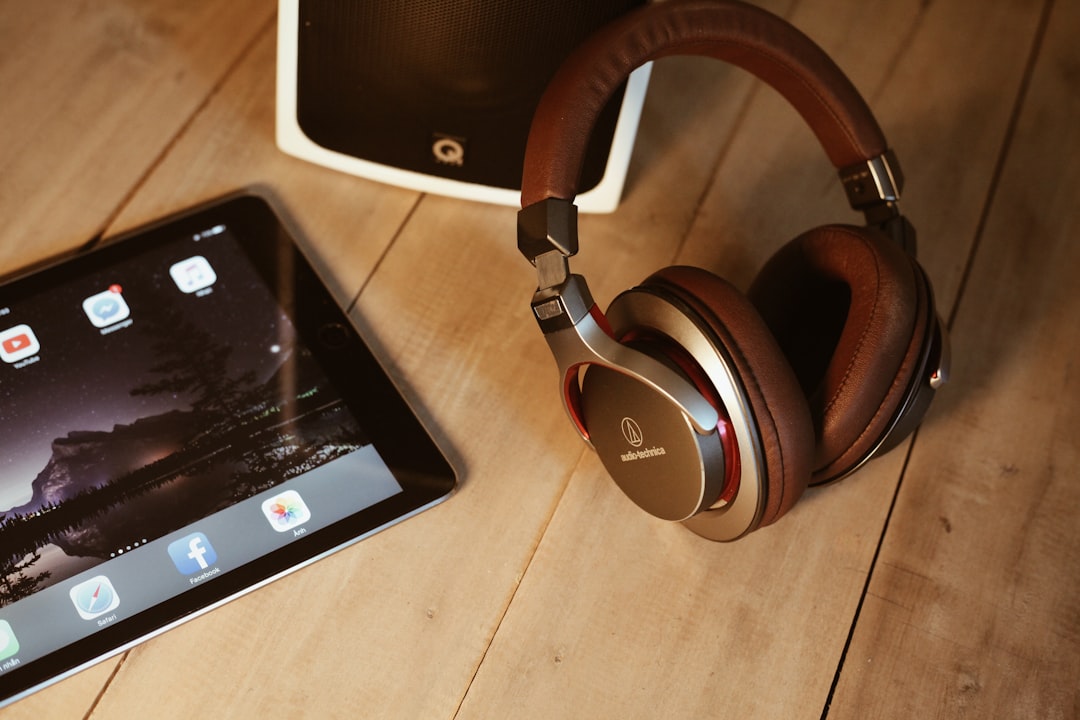
Replicating emotional tone and inflection in voice cloning remains a significant challenge. While current voice cloning can achieve a degree of accuracy in mimicking a person's voice, the ability to capture and reproduce the nuances of emotional expression in speech is still under development. Traditional techniques often struggle to adequately replicate the subtleties of human emotion conveyed through tone and inflection.
However, progress is being made with the use of advanced AI models. Deep neural networks and hybrid approaches combining acoustic features with other data are showing promise in capturing and replicating more nuanced emotional expression. These advancements are particularly crucial in applications like audiobook narration and podcasting where conveying emotion authentically is critical for listener engagement and experience.
Despite these developments, truly achieving natural and convincing emotional depth in a voice clone remains a complex issue. Developing algorithms capable of understanding and reproducing the subtleties of human emotional communication is an ongoing area of research and requires continued innovation within audio processing techniques. The goal is to move beyond simple imitation and towards generating cloned voices that express genuine emotional range.
### Replicating Emotional Tone and Inflection in Voice Cloning: A Look Under the Hood
While replicating a person's voice is a core goal of voice cloning, capturing the emotional nuance of their speech is a more challenging task. The emotional content of speech is largely conveyed through prosody, encompassing pitch variations, stress patterns, and timing. It's estimated that around 80% of emotional meaning is embedded in these prosodic features. Accurate voice clones need to precisely replicate these dynamic aspects to genuinely convey the intended emotion.
However, replicating emotions across different languages presents a unique hurdle. Emotional expression and inflection often differ significantly between languages. A model trained mostly on English speech might not be well-equipped to reproduce the same emotional cues in, say, Mandarin or Spanish. To overcome this, training models on a diverse array of multilingual datasets is crucial. This requires a considerable effort to collect and curate datasets representing various language families and cultures.
One of the pitfalls of training models on limited emotional datasets is overfitting. When a model becomes excessively specialized on a narrow range of emotions, it can lose its ability to generalize effectively. This leads to a less versatile voice clone, one that might struggle to produce emotions outside its initial training regime.
Attention mechanisms have become valuable tools for improving the accuracy of emotional expression. By allowing the model to focus on the most emotionally salient parts of the speech, they can hone in on specific words or phrases carrying the emotional charge. This contextual awareness helps synthesize more authentic-sounding emotional inflections while simultaneously minimizing the impact of background noise that might interfere with emotional recognition.
Moreover, capturing the full range of human emotional expression in a voice clone is complicated by the fact that each individual's vocal characteristics can change depending on their emotional state. Stress, for example, can alter pitch and speaking rate. Advanced models are needed to dynamically adjust these attributes in real-time to ensure authenticity in the cloned voice.
Formant tuning is another essential component of recreating emotional tone. Formants are the resonant frequencies that create the unique sound of a person's voice. They can shift subtly depending on the emotion being expressed. Optimizing these frequencies in real-time is crucial for maintaining a sense of realism in the synthesized voice.
Interestingly, voice cloning techniques can be used to generate artificial emotional expressions not necessarily present in the original speaker's voice. This offers a fascinating opportunity for creative applications, such as infusing dramatic emotional elements into audiobook narrations that the original text might not explicitly suggest.
Contextual data plays a critical role in enhancing the accuracy of emotional expression in voice clones. Combining audio signals with corresponding visual cues or narrative content allows models to understand the emotional context better. This integrated approach helps to refine and adapt the synthesized emotional inflections for a more natural and convincing outcome.
Achieving real-time adjustment of emotional tone during voice synthesis is a complex undertaking. It necessitates sophisticated algorithms that can rapidly analyze and adapt the speech patterns in response to changes in emotional content. While this adds complexity to the infrastructure, it substantially contributes to the realism of the final output.
To effectively replicate a diverse range of emotional expressions, voice cloning models benefit from being trained on datasets that include a wide variety of speech styles. This includes energetic presentations, subtle comedic timing, and emotional interactions, exposing the model to a broad spectrum of human emotional delivery. This approach ensures that the resulting voice clones can adapt to different scenarios and content, creating a more versatile and useful tool for audio production.
The field of emotional tone and inflection replication in voice cloning is still in its early stages. As we develop more sophisticated models and training datasets, we can anticipate even more realistic and nuanced expressions of emotion in synthetic speech. This promises to enhance the appeal and application of voice cloning across a variety of audio-related fields.
Audio Processing Techniques for Improving Voice Cloning Accuracy in 2024 - Noise Reduction and Audio Cleanup Algorithms
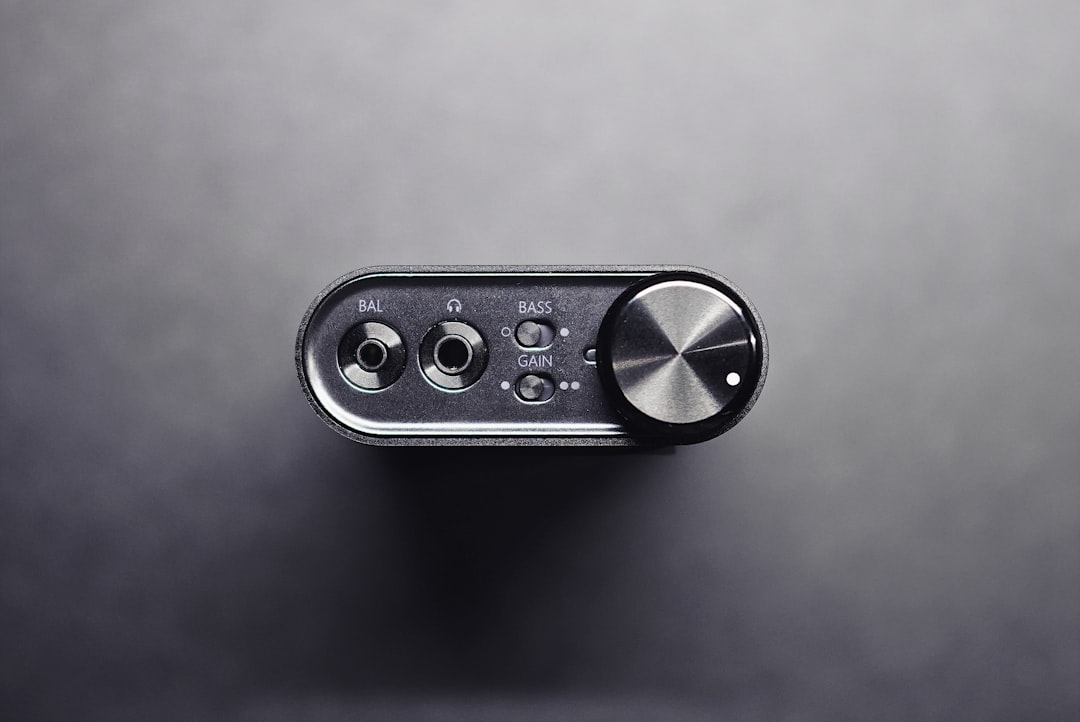
Noise reduction and audio cleanup algorithms are vital for improving the accuracy of voice cloning, especially when dealing with recordings made in noisy environments. These algorithms are essential for separating speech from unwanted background sounds, which is critical for creating clear and understandable cloned voices. Traditional noise reduction methods, such as spectral subtraction, often struggle to effectively manage dynamic noise scenarios. This limitation has spurred the development of newer approaches, such as hybrid techniques that combine deep learning with sophisticated filtering techniques. Recently, noise reduction models specifically tailored to voice cloning have been created, capable of removing noise in real-time, which significantly improves the final audio quality. These advancements in noise reduction algorithms are integral to producing more natural and believable synthesized speech, which enhances the overall listening experience in applications like podcasts, audiobooks, and various voice-driven content. Continued refinement in this field remains crucial for the future of high-quality voice cloning.
### Noise Reduction and Audio Cleanup Algorithms: A Look Under the Hood
Noise reduction and audio cleanup algorithms are becoming increasingly important for enhancing the quality of audio, especially within the context of voice cloning. These tools help to improve clarity and reduce unwanted background sounds, which is crucial for obtaining accurate and natural-sounding clones. However, noise reduction in itself is a complex field.
One of the key factors influencing modern noise reduction algorithms is the concept of psychoacoustics. Psychoacoustic models aim to mimic human hearing, and they help algorithms focus on removing frequencies that are less perceptible to our ears. This selective noise filtering helps retain audio quality in a way that would be more difficult if we blindly removed all noise.
Traditional noise reduction methods often utilize the Fourier transform, breaking down the audio signal into simpler sine and cosine waves. But for more intricate audio cleaning, wavelet transforms are gaining traction. Wavelets analyze signals across multiple frequencies at once, which allows them to preserve essential audio characteristics more effectively. This is important in voice cloning because preserving subtle details in speech signals leads to more lifelike and nuanced clones.
Adaptive filtering is a technique that allows noise reduction algorithms to automatically adjust to changes in the noise environment. This means that they are particularly useful for applications like live recordings where background noise can be unpredictable. Algorithms can dynamically refine their parameters to achieve optimal noise reduction, leading to a smoother and cleaner audio experience.
In recent years, we've seen the use of deep learning techniques significantly improve noise reduction. Neural networks are now being trained to not only reduce noise but also selectively enhance parts of the audio crucial for human perception. The goal is to improve both clarity and naturalness simultaneously, helping audio recordings sound more like what a human would hear in a clean environment.
One particularly exciting development is the use of de-noising autoencoders. These are a type of neural network specifically designed to reconstruct clean audio from noisy versions. These autoencoders are a form of unsupervised learning and have proven to be effective at enhancing speech quality, finding application in tasks such as cleaning up podcast and audiobook recordings.
The spectral subtraction method is a classic approach to noise reduction that involves subtracting an estimated noise spectrum from the noisy audio. While straightforward, the effectiveness of this method is strongly tied to the accuracy of the noise estimate. Recent work has improved spectral subtraction by developing algorithms that dynamically update the noise estimate, adapting to the shifting characteristics of the audio signal.
Another powerful tool for audio manipulation is the phase vocoder. While commonly used for tasks like time-stretching audio, phase vocoders can also be employed for noise reduction. They enable separate adjustment of frequency and timing elements within an audio signal, making it easier to refine the audio while preserving phonetic detail. This is essential in voice cloning, where preserving the character of the voice is crucial.
Temporal masking, the phenomenon where a loud sound can make quieter neighboring sounds less perceptible, is also leveraged by audio algorithms. By identifying and incorporating these masking effects, noise reduction algorithms can reduce the noticeable presence of noise while maintaining a relatively high overall audio quality.
There is an increasing interest in developing cross-domain noise reduction techniques. Borrowing ideas from different areas, like image processing, has shown great promise. For example, using wavelets which come from image denoising has proven helpful in audio cleanup, highlighting the potential of transfer learning in audio technology.
Finally, noise reduction algorithms are moving beyond being solely post-processing tools. With the rapid development of teleconferencing and live streaming applications, there is a strong demand for real-time noise reduction. Many audio applications now incorporate these algorithms to improve the communication experience in live environments, offering significantly improved clarity for voice interactions.
While noise reduction continues to be a challenge for many voice cloning applications, the constant innovation and refinement of algorithms are leading to consistently improved quality. This not only benefits the realm of voice cloning but also offers wide-ranging applications across a variety of media creation tasks. The ability to create high-quality, noise-free recordings is crucial for applications ranging from creating podcasts to generating natural-sounding audiobooks, and these improvements are central to achieving more realistic and usable cloned voices.
Audio Processing Techniques for Improving Voice Cloning Accuracy in 2024 - Low-Resource Speaker Adaptation Techniques
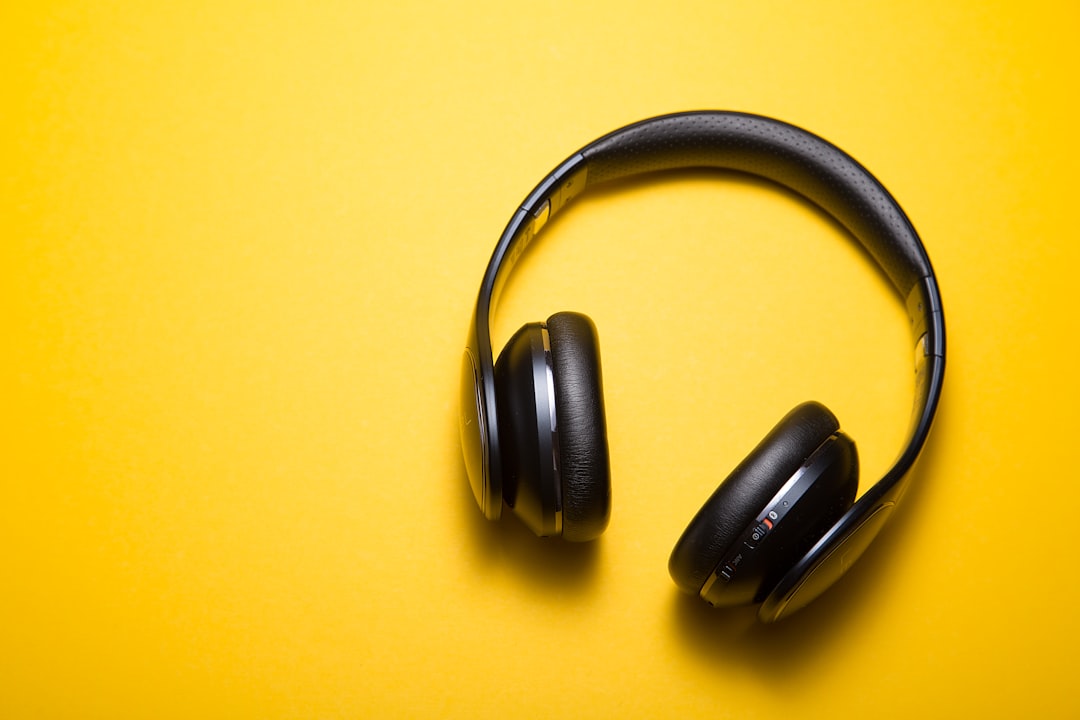
Within the domain of voice cloning, techniques for adapting models to new speakers using limited audio data are becoming increasingly important. These "low-resource speaker adaptation" techniques are especially valuable as the desire for personalized audio, whether in audiobooks, podcasts, or voice cloning applications, continues to grow. The core of these methods is adapting multi-speaker generative models to perform well even with a scarcity of audio samples. This often involves leveraging few-shot learning, a process where the model attempts to learn the essence of a new voice from very little input, sometimes just a few seconds of audio.
While full speaker adaptation can be computationally expensive and data intensive, speaker encoding has emerged as a promising alternative for low-resource settings. Speaker encoding techniques prioritize efficiency, leading to faster cloning times and requiring less memory. This is critical for broader adoption of the technology. However, adapting voice cloning to languages with limited datasets is a persistent hurdle.
Fortunately, the field of transfer learning offers hope. Transfer learning allows us to use insights from the training of models in seemingly unrelated areas, such as speech recognition, to bootstrap the learning process for new speakers or languages. Furthermore, zero-shot voice cloning, which aims to generate speech from speakers not seen during the model's training phase, is another avenue to tackle this challenge. It focuses on extracting speaker-specific information from audio samples, which can lead to more robust and adaptive voice cloning systems.
As voice cloning technology continues to mature, efforts to replicate the emotional tone and inflection of a speaker with greater fidelity will be crucial. The goal is to move past basic voice imitation toward creating synthetic audio that accurately conveys a range of human emotions, resulting in a much more engaging listening experience. Continued innovation in low-resource speaker adaptation is critical for making voice cloning more accessible and effective for a wider variety of applications and languages.
When dealing with limited audio data, known as low-resource conditions, adapting voice cloning models to new speakers becomes a crucial task, especially for applications like voice cloning, audiobook creation, and podcast production. These low-resource scenarios present unique challenges and have spurred the development of various techniques.
One primary approach is **speaker adaptation**, which involves fine-tuning a model trained on a larger, more diverse dataset to better replicate a specific speaker's voice using only a small number of audio samples. While effective, it can be computationally demanding, particularly when dealing with very limited data.
In contrast, **speaker encoding techniques** provide an appealing alternative in such low-resource settings. They're known for their efficiency, using fewer computational resources and offering quicker cloning speeds. These methods extract key characteristics of a speaker's voice and apply them to a pre-trained model, achieving faster adaptation compared to traditional speaker adaptation techniques.
**Transfer learning** has emerged as a significant strategy for bolstering the performance of voice cloning in low-resource scenarios. Particularly useful for monolingual speech datasets, transfer learning involves leveraging knowledge gained from other, related audio processing tasks. This allows models to perform well despite limited training data specifically focused on voice cloning.
Another area of focus is **few-shot learning** where models are trained to create unique voices from minimal input. Sometimes, only a few seconds of audio are needed to adapt the model to a new speaker. This technique's efficacy makes it ideal for tasks requiring quick adaptation to new voices, like in real-time interactions or rapidly evolving audio productions.
However, low-resource situations become more challenging when applied to languages with minimal available audio resources. Models have to adapt to speakers in these scenarios with limited datasets. These difficulties are particularly prevalent in the area of **voice cloning for low-resource languages**. There is a need to create models that can quickly adapt to new speakers using very little available audio data.
A related concept is **zeroshot voice cloning**, where the aim is to generate speech for speakers previously unknown to the model. This involves extracting key speaker characteristics from the target speaker's audio. A lot of research is dedicated to improving the accuracy of this type of cloning, which is especially important for quick-turnaround projects.
**Out-of-domain speaker adaptation** can also be viewed through the lens of few-shot learning, presenting its own challenges in low-resource environments. In essence, the model is being tasked with learning about a new speaker in a context where it previously had little experience. It's a tough problem for the model, but a highly relevant one given the dynamic nature of many audio projects.
In the realm of **Emotional Text-to-Speech (TTS)** and **speaking style-based TTS**, pre-trained models are often used in conjunction with transfer learning. This hybrid approach can effectively improve voice cloning for new speakers, as the pre-trained components are well-suited for transferring learned information to new scenarios.
Beyond traditional methods, some researchers are delving into **multi-reference audio techniques** as a means to improve cloning accuracy. This promising approach is attempting to use different versions of the target audio to improve mutual information for the cloning model.
Ultimately, advances in low-resource voice cloning have contributed to the democratization of voice cloning technology. However, the ease with which voice cloning technology can be deployed has also made it increasingly difficult to distinguish between real human voices and AI-generated ones. This has created some concerns as regards consent and the potential for malicious use of such capabilities. While exciting, it's essential to approach this field responsibly, acknowledging its potential impact on society, especially given the blurred lines it creates between genuine human interactions and AI-generated counterparts.
More Posts from clonemyvoice.io: