Get amazing AI audio voiceovers made for long-form content such as podcasts, presentations and social media. (Get started for free)
Voice Cloning in Audio Production 7 Key Considerations for Length and Timing
Voice Cloning in Audio Production 7 Key Considerations for Length and Timing - Optimal Audio Sample Duration for Professional Voice Cloning
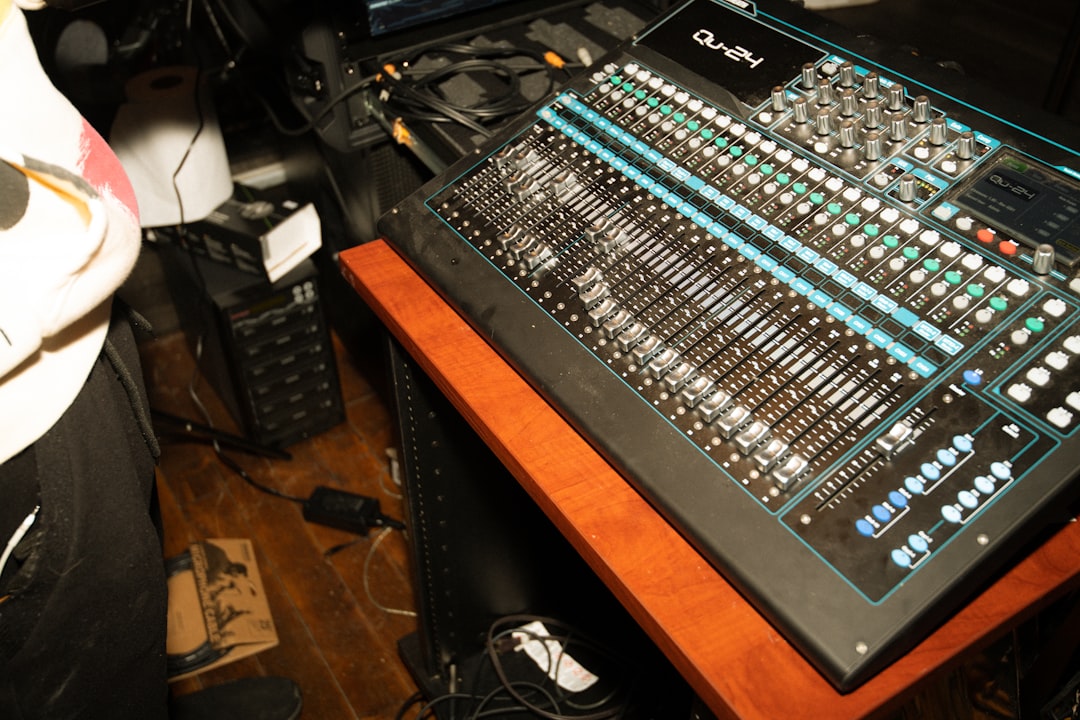
When aiming for top-tier voice cloning, the length of the audio sample you provide significantly influences the outcome. While shorter samples might suffice for basic cloning, achieving truly professional results generally necessitates a minimum of 30 minutes of high-quality audio. Ideally, you should strive for around 3 hours of audio to allow the cloning process to capture the nuances and subtleties of the speaker's voice.
Beyond duration, the quality of the recording itself is paramount. Crystal-clear audio with minimal background noise and a constant volume level are critical. These factors ensure that the cloning algorithm has a clean and accurate representation of the target voice to learn from. For best results, the audio should feature only a single speaker. Maintaining a consistent tone and including short, natural pauses between spoken phrases further enhances the cloning process.
While a larger dataset can be helpful, pushing for significantly more than 23 minutes of samples might not yield commensurate improvements and could even negatively impact the clone's performance, causing instability. The balance between sample duration and quality is essential for anyone seeking to create convincing cloned voices for projects like audio book production or podcasting. The goal is to provide a rich enough representation of the speaker’s voice to allow for a truly successful replication.
1. **The Significance of Sample Length for Phonetic Capture**: Very brief audio snippets, under a second, often lack the diversity of human sounds needed for successful cloning. Longer samples, conversely, allow for a more detailed representation of vocal nuances, essential for replicating the subtle expressions that make a voice sound natural.
2. **Phoneme Variety and Sample Duration**: The ideal sample length should consider the wide range of sounds (phonemes) a person uses. Research suggests that a variety of speech contexts and emotional tones within the sample improves the adaptability and authenticity of the cloned voice.
3. **Signal Processing and Sample Length**: When voice samples are too short, they can cause distortions during the audio processing phase. The engineering behind the cloning process needs to align the sample length with its internal processing speed to ensure the best possible output fidelity.
4. **Speech Patterns and Entropy**: Voices represented by longer audio samples exhibit more consistent pitch and tone, leading to smoother, more harmonious cloned voices. Finding the right balance between consistent and varied samples is crucial for a desirable result.
5. **Sample Rate and Detail**: Experiments have shown that higher sampling rates (like 48 kHz) combined with well-chosen sample lengths capture the most minute details of speech. This is particularly useful in creating high-quality audio, like audiobook narration.
6. **Voice Characteristics Across Demographics**: The optimal sample duration can change depending on the speaker's age and gender. For example, children's voices, with their high frequencies and faster speech, might necessitate shorter sample lengths compared to adults, where longer ones are preferable.
7. **Noise Reduction and Sample Length**: Extended audio samples provide a larger dataset for noise reduction algorithms to work with. This is vital for producing clear and pristine audio in applications like audiobook production or voice-over work.
8. **The Diminishing Returns of Excessively Long Samples**: While generally, longer samples enhance the clone, there's a point where adding more data becomes less useful. Samples exceeding 20 seconds can add unnecessary complexity and might lead to inefficient processing.
9. **Cultural and Dialectal Influences on Sample Length**: The way people speak varies greatly across cultures and dialects. This requires careful consideration of how these linguistic differences affect optimal sample durations for effective voice cloning.
10. **Adapting Sample Length in Real-Time**: For interactive voice applications, like podcasting, researchers are developing systems that can adapt the sample length based on the current speaker and the content. This can significantly improve the overall listening experience and make the content seem more genuine.
Voice Cloning in Audio Production 7 Key Considerations for Length and Timing - Impact of Audio Quality on Voice Clone Accuracy
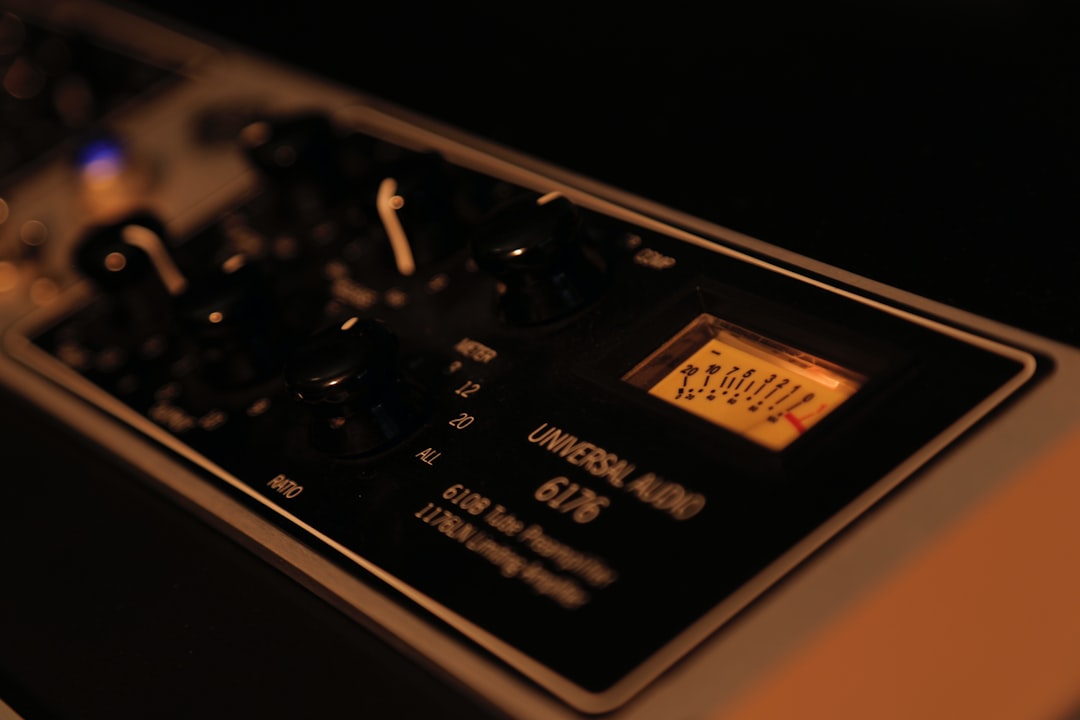
The quality of the audio used to train a voice clone significantly impacts how accurately it replicates a person's voice. When the audio is high quality, free from background noise and with consistent volume levels, the cloning algorithms can more effectively learn the nuances of the speaker's voice. Conversely, poor audio quality can result in inaccurate voice clones that don't faithfully reproduce the original voice. Techniques used to improve audio clarity, such as noise reduction, can help to enhance the final output of the cloning process. As voice cloning technology progresses, comprehending the relationship between audio quality and cloning accuracy becomes increasingly important for producing lifelike synthetic speech, especially in applications like podcast creation and audiobook narration. The goal is to create clones that sound natural, not artificial. The more precise the input, the better the output will be. Some algorithms are being built to deal with very limited data, but the most accurate models benefit from quality input data.
The quality of the audio used to train a voice clone significantly influences the accuracy and overall effectiveness of the resulting synthetic voice. If we use low-quality audio, filled with noise or distortion, it can hinder the cloning process, leading to a less accurate and less natural-sounding clone. High-quality audio recordings are essential to ensure the algorithms can effectively learn the unique characteristics of the speaker's voice, such as pitch, tone, and intonation.
Techniques like noise reduction and audio enhancement can improve the quality of the training data, ultimately helping to refine the cloning process. However, the amount of audio data is also a factor. Ideally, you need a minimum of 25 high-quality recordings of the target voice to ensure that the model learns a comprehensive representation of the voice's characteristics. While advanced systems have shown impressive results with just a few seconds of audio, relying on such a small amount of data usually results in a less convincing voice.
It's interesting that real-time voice cloning systems often rely on multiple algorithms to achieve high-quality speech synthesis. It appears that a multi-pronged approach can often outperform more basic algorithms. However, the ultimate goal is to produce cloned voices that are not only accurate in mimicking the source voice but also maintain a natural sound in applications like audio book production, podcasts, and even dubbing.
Furthermore, this technology's ability to convincingly replicate a person's voice raises legal and ethical issues, particularly regarding potential misuse in creating fake audio, known as deepfakes. There are growing concerns surrounding intellectual property and potential harm arising from the use of cloned voices. These concerns have prompted discussions about the responsible development and implementation of voice cloning technology.
Ultimately, the rising adoption of AI-powered voice cloning reflects a dramatic shift within the audio industry. It's offering producers a new level of flexibility and scalability. However, it also necessitates careful consideration of its implications. As a field, we're seeing an evolution where human voice actors are increasingly complemented, and sometimes even replaced, by synthesized versions, introducing both fascinating opportunities and noteworthy challenges.
Voice Cloning in Audio Production 7 Key Considerations for Length and Timing - Balancing Audio Length with Clone Quality
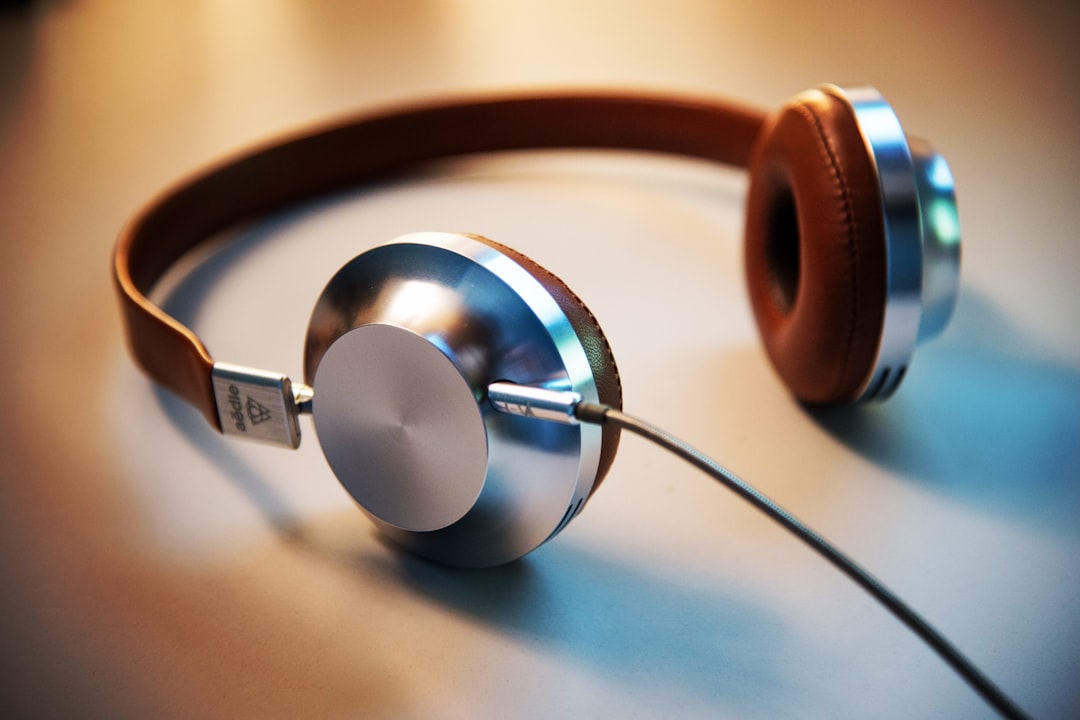
In the world of voice cloning, the relationship between the duration of the audio sample and the quality of the resulting clone is a delicate balancing act. While short samples, maybe a minute long, can produce a basic clone, truly achieving professional quality typically necessitates a substantial audio sample, ranging from 30 minutes to even 3 hours. This extended length allows the cloning algorithms to absorb a wider range of the speaker's vocal patterns and idiosyncrasies, contributing to a more natural and realistic sound. However, there's a point of diminishing returns. Adding significant amounts of audio data beyond about 23 minutes may not provide a proportionate increase in quality and can potentially destabilize the clone's performance. When producing audio content like podcasts or audiobooks, factors like consistent tone and pristine audio clarity are paramount. These factors work in concert with sample length to ensure the cloned voice is not just accurate but also engaging and pleasing to the listener. By carefully navigating the optimal sample length and audio quality, voice cloning technology can achieve a truly remarkable level of realism, expanding possibilities in diverse audio production projects.
Considering the length of an audio sample for voice cloning reveals a complex interplay between the quantity of data and the quality of the resulting clone. While a longer audio sample generally improves the clone's accuracy, there's a point where increasing the duration might not significantly enhance the quality and could even introduce issues. For example, an audio sample exceeding 23 minutes may not yield proportionate improvements in the voice clone's quality, and in some cases, may destabilize the model.
The diversity and range of sounds within the sample are crucial. Longer audio provides more opportunities for the cloning algorithms to learn the full spectrum of a person's voice, including nuances like emotional expression. This is especially important for applications like audiobook production, where capturing a wide range of emotions and vocal inflections is crucial. However, focusing on a broad dynamic range – capturing both soft and loud passages – can enhance the emotional depth the algorithm learns.
It's interesting to note that the syntax and rhythm of speech also affect the cloned voice. The way a speaker structures their sentences and phrases can subtly influence the perceived personality of the synthetic voice. Researchers have observed that incorporating slightly redundant phrases can help reinforce specific sounds, ultimately improving the clone's accuracy. The recording environment can also introduce unwanted acoustic characteristics. If the sample is recorded in a live setting, reflections and reverberations can interfere with the cloning process. Using high-quality microphones in a treated studio yields superior results because these types of microphones are better at capturing the finer details, particularly in the higher frequencies that are vital to the accuracy of vocal replication.
It's fascinating how the cloning model also learns from a speaker's emotional tone. If the sample data includes various emotional expressions, the model can better grasp how to reproduce these nuances, leading to more expressive synthetic voices. We are still learning how various speaker characteristics like age and gender influence the optimal duration and content of a voice sample. There's potential here, but we also need to be mindful that overly complex algorithms might "overfit" to lengthy samples. This means that they might become too attuned to the specific training data and fail to generalize well to new phrases or contexts. For applications where real-time responsiveness is crucial, like interactive podcasts, the length of the audio samples becomes critical in minimizing latency or delay during the cloning process. Balancing the desire for an accurate clone with the requirement for real-time generation will continue to be an active area of research.
In conclusion, there's a delicate balance to strike between the length and quality of the audio samples used in voice cloning. While longer audio samples can contribute to a richer, more detailed clone, exceeding a certain point can lead to diminishing returns and potential instability. Future advancements in voice cloning might be achieved by employing algorithms that can adaptively adjust to specific audio characteristics, offering more flexibility and ensuring that the resulting voice clone maintains both fidelity and naturalness.
Voice Cloning in Audio Production 7 Key Considerations for Length and Timing - Performance Style Replication in Voice Cloning
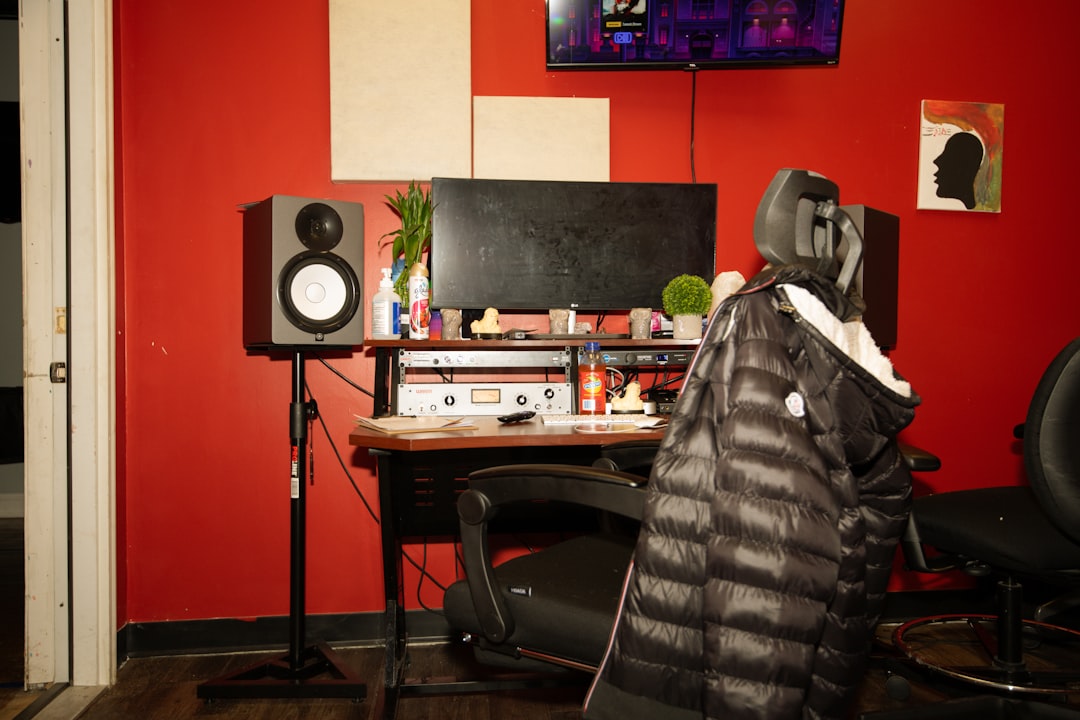
**Performance Style Replication in Voice Cloning**
The ability to replicate a speaker's unique performance style is a crucial aspect of advanced voice cloning. These sophisticated systems can now capture and reproduce subtle vocal characteristics, including the speaker's accent, rhythm, and how they naturally change their tone of voice. This level of detail in the cloned voice creates a more believable and engaging audio experience. The potential for voice cloning to adapt to various performance styles has the power to dramatically change audio production, especially when it comes to audiobooks or podcasts, where the ability to capture a specific mood or tone is highly important.
However, achieving a completely accurate replica of a speaker's performance style presents significant hurdles. The complexity and nuanced nature of human vocal expression still pose a challenge for current AI technology. As voice cloning develops, carefully managing the goal of realistic replication while acknowledging the limitations of artificial intelligence will be essential if we want to produce audio that is truly captivating and authentic.
When delving into the realm of voice cloning, we find that replicating the nuances of a speaker's performance style is a complex endeavor. The diversity of a speech sample— encompassing laughter, sighs, and emotional tones— significantly influences the resulting clone's expressiveness. Simply replicating sounds isn't enough; the cloning algorithms must learn the emotional tapestry interwoven within a speaker's delivery, especially for projects where conveying emotion is vital, like storytelling in audiobook production.
The rhythmic flow and pacing of speech—temporal patterns—also contribute significantly to how a cloned voice is perceived. Models trained on audio samples that exhibit these natural patterns are capable of generating synthetic speech that retains the speaker's delivery style, moving beyond mere sonic replication.
Background noise levels, too, play an intriguing role. Surprisingly, a certain level of ambient noise can actually help the algorithm focus on the essential vocal characteristics. Conversely, recordings awash in loud or erratic sounds tend to lead to inaccurate clones. The challenge lies in finding that optimal sweet spot of background noise that doesn't interfere with the voice characteristics.
Frequency range and a listener's perception of the voice are interconnected. Higher frequencies tend to project a youthful tone, while deeper frequencies can evoke a sense of authority. Understanding these auditory cues enables cloning engineers to better refine models for the desired outcome, matching a synthesized voice with the intended emotional context of the content.
The recording environment itself can significantly influence the clone's fidelity. Reflections and reverberations from overly live or untreated rooms can mask important voice characteristics. Ideal scenarios utilize treated environments to minimize these sonic artifacts, ensuring a clearer, more precise capture of the speaker's voice. This is especially crucial for the high-frequency components that play a vital role in the accuracy of vocal replication.
Fortunately, real-time voice cloning, once a distant prospect, is now a reality in many instances thanks to the advancements in computing power. These systems adapt on the fly to the speaker's voice, making the cloned voice sound more natural and seamless, especially during interactive applications like podcasts. The challenge here is to prevent the algorithm from over-adapting, leading to a strange, unnatural, unpredictable sound.
Phonetic context matters greatly, influencing how sounds are produced depending on the neighboring sounds. The more advanced cloning algorithms are increasingly tuned to these complexities, resulting in more authentic-sounding speech.
While short snippets of audio can trigger the initial stages of cloning, they frequently result in robotic-sounding output. Achieving a more natural and expressive clone necessitates extended audio recordings. These longer samples allow for a comprehensive capture of nuanced voice traits, pushing the cloned voice toward a higher level of authenticity.
Speaker accents and dialect variations introduce further complexity. Accurately replicating these diverse speech patterns often demands significant sample lengths to capture the intricate nuances embedded within these unique styles of speech. This has proven difficult for many cloning engines.
Finally, it's important to acknowledge that post-processing techniques, such as equalization and compression, play a role in polishing the final product. These procedures can enhance the naturalness of the synthetic voice, ensuring that it integrates seamlessly into various audio contexts, bringing a professional touch to the creation of audiobooks, podcasts, and other audio content. The post processing can take a lot of time and effort.
Voice cloning is a rapidly developing field, and while we've made remarkable progress, there are still substantial challenges to address. Understanding the interplay between audio characteristics, sample length, and the underlying algorithms is essential for creating clones that are not only accurate but also naturally expressive, allowing for an expanded palette of possibilities in the world of audio production.
Voice Cloning in Audio Production 7 Key Considerations for Length and Timing - Single-Speaker Consistency vs Mixed Audio Sources
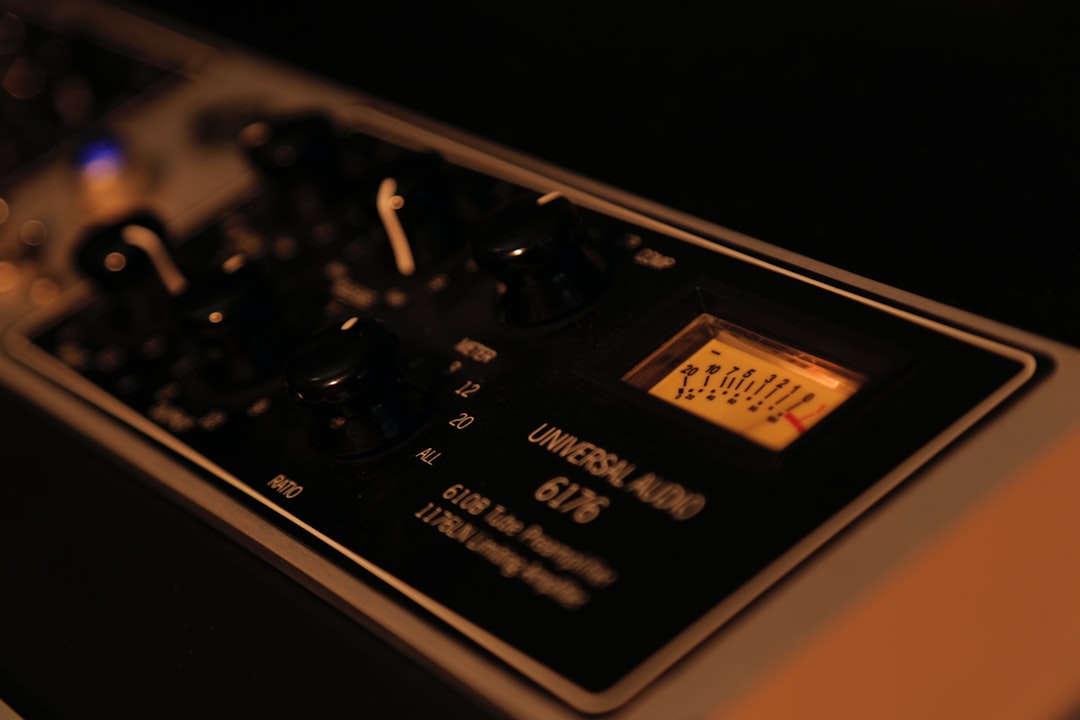
When it comes to voice cloning, the difference between using audio with a single consistent speaker and audio that mixes multiple speakers is very important. Using recordings where only one person is speaking gives the cloning algorithms a solid base to accurately learn the unique characteristics of that voice. This leads to clones that sound more natural and like the original person. However, if the audio has a mix of voices, it becomes more difficult for the algorithms. Cloned voices from mixed audio often have distortions and are less clear because the algorithms struggle to separate the different voices and sounds. While technology is improving at separating the voices in mixed recordings, it's still challenging to get high-quality results, especially when people have very different speaking patterns and when voices overlap. For using voice cloning in audio production, like for audiobooks or podcasts, knowing how the combination of single or mixed audio, and different voice patterns, affects the cloning process is crucial for getting better results.
When it comes to voice cloning, the quality of the audio sample is paramount, and a key aspect of this is ensuring the source audio is primarily from a single speaker. Using audio with multiple speakers mixed together can significantly impact the effectiveness of the cloning process. Algorithms designed to learn the intricacies of a single voice often struggle when presented with conflicting sound characteristics from different speakers. This can result in cloned voices that lack the natural subtleties of the target speaker.
For example, algorithms trained on mixed audio may have a difficult time accurately capturing the nuances of emotional expression within a single voice. The blended nature of the audio can lead to a less engaging, more "flat" sounding cloned voice. This is especially problematic for applications where emotion is crucial, such as audiobook narration or storytelling. Additionally, distinct vocal patterns, like rhythm and tempo, can be disrupted in mixed audio, leading to unnatural pauses or erratic changes in speech flow in the resulting cloned voice.
Furthermore, the clarity of a speaker's voice can be masked by background noise in mixed sources. This makes it harder for the algorithm to learn subtle tonal variations and inflections that define a person's unique speaking style. The resulting cloned voices might sound less natural and adaptive as a result.
Researchers have observed that listeners expend more cognitive effort when trying to process mixed audio with multiple speakers. This can be distracting and interfere with the overall listening experience, which is especially important for applications such as podcasts where maintaining listener engagement is key.
Furthermore, preparing data for voice cloning involves a considerable amount of annotation, which is the process of labeling the different features within the sound. Using audio samples from a single speaker simplifies the annotation process, leading to more efficient and accurate voice cloning. Conversely, mixed audio introduces complexities that make it more challenging to annotate features, and this can have a negative effect on the resulting voice clone.
Moreover, each individual's voice comes with a unique noise profile—characteristics associated with the surrounding environment. Mixing these noise profiles in a recording can muddle the clarity of the training data, ultimately leading to a less authentic clone. Similarly, mixed audio might introduce inconsistencies in reverberation—the reflection of sound in an environment. This can create problems with the accuracy of the cloned voice.
Idiosyncrasies—unique characteristics—within a speaker's voice, such as dialects or specific ways of pronouncing words, are more accurately captured in audio samples that feature a single speaker. The presence of multiple voices can obscure these idiosyncrasies, causing the resulting voice clone to lack some of the individual characteristics that make the voice recognizable.
Finally, voice cloning algorithms are often tailored to specific data characteristics. Training a model on a single speaker leads to a more efficient tuning process. Conversely, mixed audio sources might confuse the model and impede its ability to develop an accurate representation of the voice being cloned, negatively impacting its overall performance.
In summary, single-speaker audio provides a clearer, more consistent training signal for voice cloning algorithms. While techniques for extracting individual voices from mixed audio continue to evolve, the benefits of utilizing single-speaker samples remain considerable for attaining accurate and natural-sounding voice clones. The quality of the source audio significantly influences the capabilities of the cloning algorithms and, ultimately, the listening experience for the end user.
Voice Cloning in Audio Production 7 Key Considerations for Length and Timing - Voice Lab Upload Processes and Generation Time
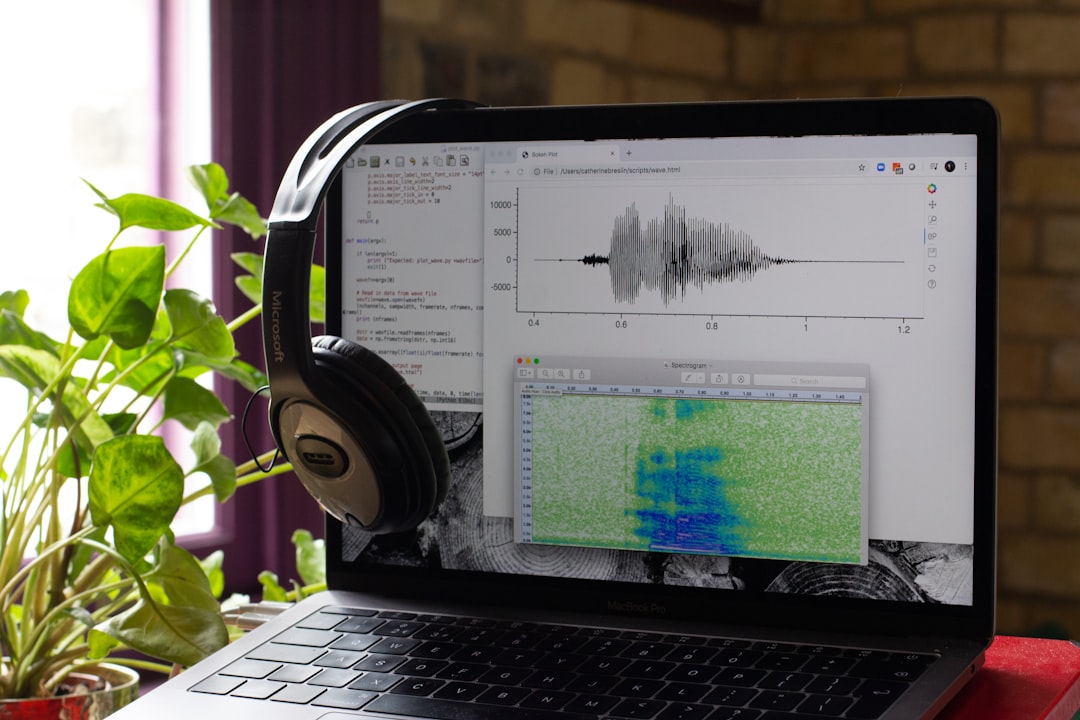
The "Voice Lab Upload Processes and Generation Time" segment explores the mechanics of audio file submission for voice cloning, specifically how AI systems streamline the process. While some systems can produce a basic clone in mere minutes, achieving high-quality results requires a thoughtful approach to audio selection. It's generally recommended to upload at least 12 minutes of high-quality audio. Optimally, you should aim for about 30 minutes to capture a speaker's vocal subtleties and unique characteristics. The specific details of how someone sounds — the distinct features of their voice — greatly affect the success of the cloning process. Maintaining audio clarity and consistency is crucial, rather than simply focusing on the sheer volume of audio uploaded. Interestingly, uploading more than about 23 minutes of audio may not lead to better voice authenticity and can potentially disrupt the cloning output. This underscores the need to carefully consider both the length and the quality of the audio you use when creating voice clones for projects like audio books and podcasts.
When exploring voice cloning, the time it takes to generate a voice—the latency—is a significant factor. Depending on the complexity of the model and the processing power available, this can range from a mere fraction of a second to several seconds. For applications like podcasts or virtual assistants where instantaneous feedback is crucial, real-time voice cloning, which produces audio nearly instantly, becomes essential. The more powerful the system and the larger the training dataset, the quicker the generation process usually is.
The amount of training data directly correlates with the quality of a voice clone. While 30 minutes of audio can provide a decent starting point, models trained on a more substantial dataset, like 3 hours of diverse, high-quality speech, usually produce much more natural-sounding results. It's a fascinating interplay between the volume of training data and how well the model captures the essence of a voice.
The idea of entropy—the measure of a system's randomness—has relevance here. The more variability and tonal changes found within a speech sample during the training phase, the more lifelike the generated voice usually sounds. In essence, the model learns to navigate the unpredictability of normal human speech, thus mirroring it more authentically.
The dynamic range—the difference between the loudest and quietest sounds—is critical for voice clone quality. When a model is trained on samples that incorporate soft and loud sounds, it can better interpret variations in tone, leading to more realistic shifts in volume in the generated voice.
The way specific sounds are produced within a phrase depends on the surrounding sounds. Advanced cloning algorithms are increasingly attuned to this complex interplay, known as phonetic context. This contextual understanding leads to a more nuanced and accurate reproduction of speech patterns.
Real-time voice adaptation is a captivating challenge in this field. While real-time cloning is becoming more commonplace, maintaining a natural sound in real-time can be tricky. The algorithm must continuously learn without getting "stuck" on brief noise or specific nuances of the current phrase, making the final output sound more like a human and less like a machine.
The frequency range of a voice clone can drastically influence the perceived age. Models tuned to capture higher frequencies often associated with younger voices can make a clone sound more energetic. Conversely, lower frequencies typically associated with older voices can create a tone of authority. The nuances of auditory perception can dramatically change our impressions of a voice.
The audio environment used to capture a speaker's voice plays a significant role. If a voice is recorded in a cavernous space with substantial reverberations, the resulting cloned voice might be less distinct and lack a sense of clarity. On the other hand, well-treated studios often yield superior results as reflections and room effects interfere less with the voice, making it easier for the model to learn.
Emotional expression, present within a voice, has a marked impact on the efficacy of a voice clone. Models trained on data rich in a diverse range of emotions—laughter, tension, and so on—are generally better able to replicate these nuances in a generated voice, making the voice sound more genuine and connected to the listener.
In mixed audio recordings, instances where speakers talk over each other, the process of extracting individual voices is notably challenging. This overlap in speech patterns presents difficulties for the model. Without sufficient acoustic separation, a voice clone might not capture the original speaker's qualities, and it may introduce anomalies.
It's still an exciting and developing field. As researchers continue to improve upon these core factors, we can look forward to voice clones that are not only accurate in replicating the sounds of a person's voice but also emotionally expressive and contextually aware, leading to more captivating audio content across a wider variety of applications.
Voice Cloning in Audio Production 7 Key Considerations for Length and Timing - Software Selection for Enhanced Voice Synthesis Realism
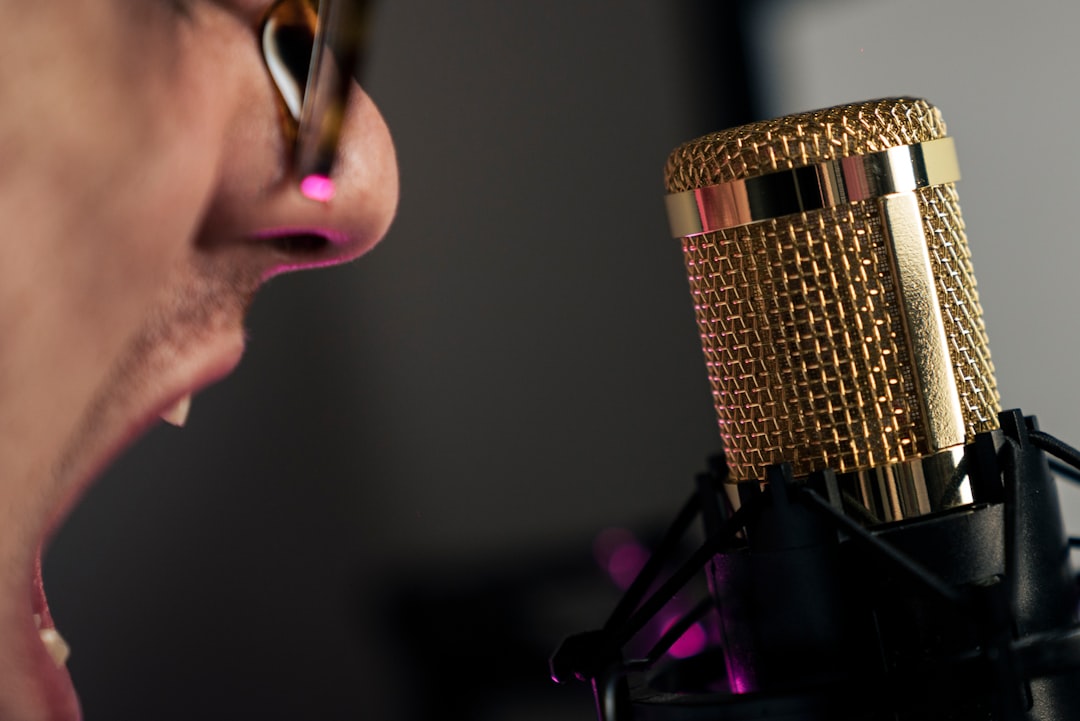
When aiming for truly lifelike synthetic voices, especially in areas like creating audiobooks or podcasts, the choice of software is paramount. The ideal software should excel at producing speech that sounds natural, free of robotic or artificial qualities. How well the software manages the nuance of vocal expression is crucial, as is the ability to generate high-quality audio that doesn't sound tinny or distorted. It's vital that the software offers flexibility and control over the output so you can tailor the sound to specific requirements of the audio project.
Given that the source audio plays a huge role in the final sound, the selected software should incorporate features that help you work with high-quality recordings and minimize the impact of background noise. If the input isn't clear, then the AI models won't be able to learn the subtle ways a person speaks, leading to unconvincing voices. Before making a decision, listen to sample outputs produced by different software to get a sense of how naturally they sound. Additionally, the workflow of the software should be easy to understand, allowing for efficient upload and processing of the audio data.
The landscape of voice cloning technology is constantly changing, so it is important to choose software that keeps up. Software that can strike a good balance between generating quality sound and doing it without requiring complicated or time-consuming steps is usually a good bet. With the right tools, you can harness the power of voice synthesis to elevate your audio projects and offer a listening experience that captivates your audience.
1. **Time's Role in Voice Replication**: Research suggests that even minuscule timing differences in speech, as little as 20 milliseconds, can affect how we perceive a tone. This highlights the need for software that precisely aligns timing during voice cloning to avoid a robotic or unnatural sound.
2. **Emotional Nuances in Voice Samples**: While impressive cloning software can identify and recreate emotional tones in speech, the training data needs to be rich in diverse emotional expressions. It's interesting that the success of a cloned voice depends greatly on how well the training data captures a variety of emotional delivery.
3. **The Importance of Phonetic Context**: The context in which sounds are produced, particularly the surrounding sounds, impacts how well a voice can be cloned. The better the cloning software understands these relationships between sounds, the more accurately it can reproduce the sounds of a cloned voice.
4. **The Curious Case of Background Noise**: It might seem counterintuitive, but a controlled amount of background noise can sometimes help cloning algorithms hone in on the essential aspects of a voice. But too much noise can make it harder for the algorithms to separate the important vocal features, causing difficulties for the cloning process.
5. **Dynamic Range for Believable Clones**: Training a cloning model using audio samples with a wide dynamic range—from gentle whispers to forceful shouts—significantly improves the natural sound of the cloned voice. This approach enables the model to accurately recreate those natural variations in tone that characterize real speech.
6. **The Perception of Frequency**: Studies reveal that we tend to perceive higher frequencies as youthful and lower frequencies as more authoritative. Understanding how we interpret these frequencies is crucial for voice cloning engineers to create clones that evoke the desired emotions or even create the impression of a specific age or demographic for the listeners.
7. **Capturing the Aging Voice**: Current voice cloning often struggles with representing the aging process in a voice over time. As people age, their voices naturally change, so a clone trained on recordings of a younger voice may not be a convincing representation of an older voice.
8. **Real-Time Delays**: The time it takes for real-time voice cloning to process can greatly influence how natural it sounds. For instance, in podcasts or conversational AI, any delays in the processing can interfere with the flow and feel of the audio. Addressing this processing time is a key area of ongoing research.
9. **The Speaker's Individual Style**: Each person has a unique rhythm and structure to their speech, and these patterns play a significant role in how a voice clone performs. Recognizing these speech patterns helps improve the overall quality of a cloned voice by making it more authentic and believable.
10. **Can We Tell a Fake Voice?**: Research suggests that listeners can typically tell the difference between a well-made synthesized voice and a human voice if the synthesized voice lacks depth and expressiveness. This is a key reminder of how crucial emotional and contextual aspects of speech are for creating authentic-sounding voice clones.
Get amazing AI audio voiceovers made for long-form content such as podcasts, presentations and social media. (Get started for free)
More Posts from clonemyvoice.io: