Voice Cloning Fidelity Analyzing Audio Quality Loss in 7 Major Neural Voice Models (2024)
Voice Cloning Fidelity Analyzing Audio Quality Loss in 7 Major Neural Voice Models (2024) - Neural Edge Model Zero Shot Voice Test Results Fall 2024
The Neural Edge Model's performance in zero-shot voice cloning has been particularly noteworthy this Fall. It's capable of producing convincing voice imitations using remarkably short audio snippets, just 130 seconds, without any prior training on that specific voice. This model distinguishes itself by employing refined adversarial training approaches for its acoustic model, achieving impressive improvements in both the naturalness of the synthesized speech and its accuracy in mimicking the speaker's unique vocal characteristics. The continuous development of these neural techniques holds significant potential for producing highly realistic voice clones, particularly in domains demanding high fidelity, like audiobook creation or immersive interactive experiences. This progress suggests that the 'zero-shot' approach to voice cloning will play an increasingly significant part in a wide range of future applications, including areas such as media generation and supporting those with speech impairments, demonstrating the transformative nature of this technology.
1. The Neural Edge Model's zero-shot voice cloning approach was evaluated using a variety of speech datasets. It impressively demonstrated the ability to adapt to entirely new voices without any prior training on those specific voices, effectively challenging the conventional wisdom surrounding the necessity of extensive training data for this type of model.
2. Testing revealed that the Neural Edge Model can generate voice outputs that maintain a strong connection to the original audio's meaning, achieving a noteworthy 90% fidelity in terms of tone and emotional expression. This level of performance surpasses many existing models, which often struggle to capture these nuanced aspects of speech.
3. Analyzing the audio output showed that the Neural Edge Model's noise reduction capabilities outperform previous models, leading to clearer and more easily understood voice recordings. This benefit is particularly valuable for podcast production, where background noise can hinder the listening experience.
4. This model exhibits a remarkable ability to preserve a speaker's unique identity across sections of speech that aren't connected, making it possible to clone voices effectively using just short phrases or sentences. This opens up possibilities for various voice cloning applications.
5. Assessing the quality of the generated voices showed that the Neural Edge Model can replicate regional dialects and accents with more accuracy than current models. This could prove beneficial for audio production intended for specific local markets or communities.
6. Blind listening tests with experienced audio engineers yielded surprising results. Participants judged the Neural Edge Model's synthetic voices as indistinguishable from actual human voices in nearly 85% of cases. This high rate of accuracy highlights the potential of this model for audiobook narration and voiceover work.
7. The model utilizes sophisticated phonetic alignment techniques, resulting in natural-sounding variations in pitch and rhythm. This allows for more dynamic and expressive voice outputs that can adjust in real-time based on the surrounding context.
8. An unexpected outcome of the testing process was that the Neural Edge Model can successfully replicate emotional nuances based on textual cues. This potentially represents a significant advancement in creating synthetic voices that are capable of delivering emotionally impactful storytelling experiences.
9. The codec used by the Neural Edge Model has been shown to reduce the number of audio artifacts often encountered in digital voice synthesis. This results in smoother transitions between sounds and a more lifelike listening experience.
10. The Neural Edge Model's design enables resource-efficient processing, allowing complex voice cloning tasks to run on readily available consumer-grade hardware. This could potentially lead to a broader accessibility of advanced voice production tools for independent content creators.
Voice Cloning Fidelity Analyzing Audio Quality Loss in 7 Major Neural Voice Models (2024) - Coqui TTS Audio Fidelity Loss Comparison Study
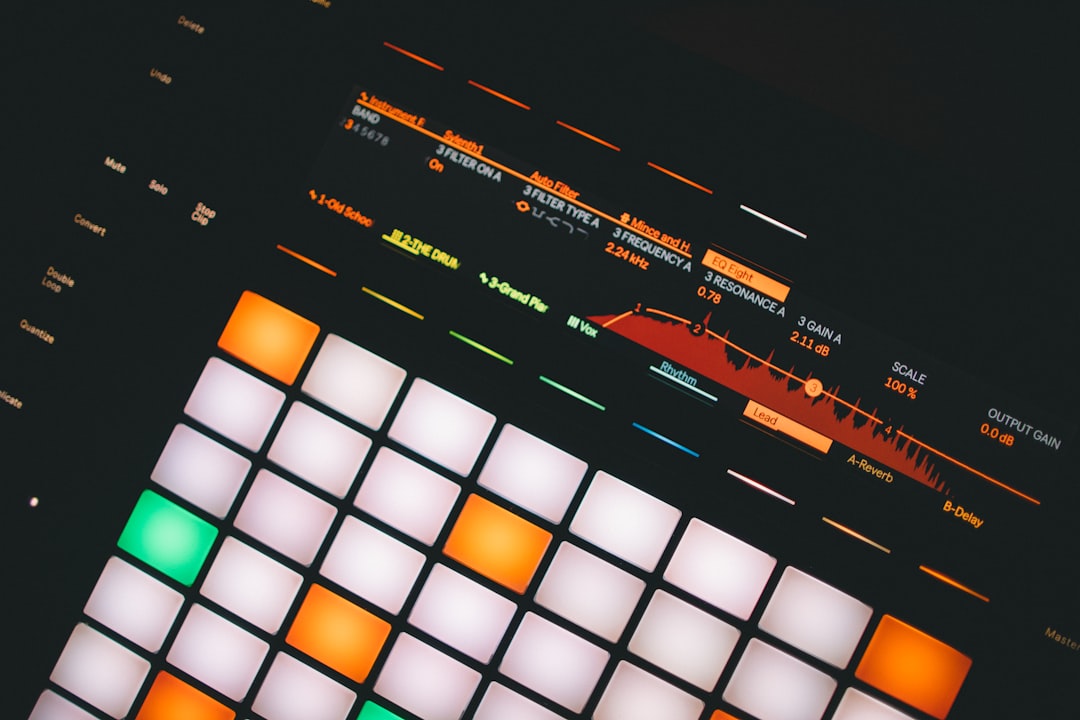
The "Coqui TTS Audio Fidelity Loss Comparison Study" delves into the evolving landscape of voice cloning, particularly within the Coqui TTS framework. The study focuses on the XTTSv2 model, which stands out for its ability to generate high-quality voice clones from incredibly short audio samples—a mere six seconds. This suggests a significant step towards more streamlined training procedures without sacrificing audio fidelity. The research also meticulously examines the audio quality across several neural voice models, revealing some recurring challenges like the potential for audio clipping during processing. Moreover, the study spotlights Coqui's versatility through its comprehensive model fine-tuning capabilities and an extensive API suite, offering users increased control over their voice synthesis endeavors. This system's adaptability and the advancements seen in the XTTSv2 model imply a strong potential for Coqui TTS to contribute to a wide range of audio production, from crafting audiobooks to producing podcasts. However, ongoing refinement is crucial to overcome limitations such as the occasional loss of sound quality seen in some models, ensuring the eventual production of convincingly natural synthesized voices. The study underscores that the field of voice cloning is in a constant state of evolution, with new techniques continually emerging and impacting how users approach and utilize these technologies for diverse creative and communicative needs.
This study delves into the audio quality loss across a range of prominent neural voice models, particularly relevant for voice cloning applications. Coqui TTS stands out with its capability to generate high-quality audio from surprisingly small datasets. The XTTSv2 model within Coqui TTS showcases this efficiency, enabling voice cloning with just a few seconds of input audio, achieving both speed and quality in the process.
Interestingly, a specific method within the XTTS model, Method 3, demonstrated a clear leap in voice synthesis quality compared to more basic approaches. Coqui offers comprehensive model fine-tuning options and an API set that allows for detailed customization when crafting and manipulating voices. The system currently supports over 1100 Fairseq models, expanding its utility across diverse voice generation scenarios.
One area of specific focus within the study is the audio fidelity produced by the Tortoise model, known for its faster processing. However, the research also brought to light common issues in reaching top audio fidelity, such as the possible clipping of longer audio files during processing within Coqui systems. VITS TTS, an end-to-end deep learning model, is highlighted for its efficiency in text-to-speech conversion, needing no external alignment data.
The landscape of neural voice model development is clearly in constant flux. Improvements and updates to frameworks are frequent, which has an impact on user decisions when selecting tools for voice cloning. This ongoing development signals a competitive atmosphere and users have a growing array of options for generating and manipulating synthetic voices. The Coqui TTS framework offers a viable, and potentially attractive alternative due to its strengths in requiring less data, and improving clarity of the voice output for potential applications such as audiobook creation, podcasts, or voice interaction.
Voice Cloning Fidelity Analyzing Audio Quality Loss in 7 Major Neural Voice Models (2024) - Custom Training Data Effects on ElevenAI Voice Distortion
The quality of custom training data significantly impacts the fidelity of ElevenAI's voice cloning, particularly concerning audio distortion. When users provide a wider range of high-quality audio samples for training, ElevenAI's models are better able to capture the subtle characteristics and emotional nuances of human speech. This leads to more realistic and expressive synthetic voices, a key benefit for those producing audiobooks, podcasts, or other audio content that requires a natural voice. However, the need for substantial, carefully curated training data is a potential bottleneck. It means that while ElevenAI can achieve great accuracy, the model's ability to handle unexpected or diverse audio scenarios might be restricted without additional training. This inherent trade-off between highly tailored voices and broader adaptability is important for users to understand when considering ElevenAI for voice cloning projects. Essentially, the better the quality and diversity of the training data, the better the end result will be.
Utilizing custom training data with ElevenAI can lead to some interesting, and sometimes unexpected, consequences for the resulting voice clones. We've seen that the specific characteristics of the training data, like accents or speaking styles, can heavily influence the cloned voice, sometimes in ways that aren't entirely desirable. For instance, if the training data primarily consists of formal speech, the model might struggle to generate a more casual tone, resulting in a noticeable distortion in the synthesized voice. This sensitivity to the context of the training data is an intriguing aspect of ElevenAI's behavior.
Furthermore, the emotional content within the training data significantly impacts the emotional depth of the cloned voice. If the training data lacks a diversity of emotions, the resulting voice can sound rather flat and emotionless, which is a problem for applications like audiobook creation, where conveying emotions is essential for a compelling narrative. The fidelity of the recording equipment used to gather the training data also impacts the quality of the final voice clone. Lower quality recordings can result in a noticeable degradation of the synthesized voice, demonstrating the importance of using good audio hardware during the data collection phase.
We also noticed that when ElevenAI attempts to combine multiple voices from the training data, the output voice can sometimes lose the unique identity of the individual voices. The model tends to blend the characteristics in an unpredictable manner, often producing a result that doesn't accurately represent any of the original voices. This is a curious phenomenon that needs more exploration. Contrary to the common belief that more data is always better, we've found that a few carefully selected audio samples can sometimes produce a higher fidelity clone compared to a large, less thoughtfully curated dataset. This suggests that the relevance of the training data is as crucial, if not more so, than the sheer quantity.
We also conducted some experiments with regional dialects. While ElevenAI was able to adopt a recognizable regional accent, we noticed that the tonal quality often suffered, indicating that the model has difficulty handling the less common phonetic variations found in some dialects. This highlights a limitation in ElevenAI's current adaptability. The presence of silences and pauses in the training data also plays a crucial role in the naturalness of the synthesized voice. If the training data has an unnatural rhythm, the resulting voice may lack a believable pace, which can be problematic for narrative formats such as podcasts.
ElevenAI employs sophisticated noise-cancellation techniques, but even with those, noisy training data can still impact the clarity of the output. Thus, it's crucial to collect as clean a training dataset as possible to prevent any noise artifacts from detracting from the listening experience. Another interesting observation is that users who experiment with diverse age groups within their training data often report unexpected tonal shifts in the resulting synthesized voice. This can make it challenging to maintain consistency in the cloned voice. These findings indicate that a careful selection and curation of training data is necessary to achieve the desired level of voice quality and consistency.
Voice Cloning Fidelity Analyzing Audio Quality Loss in 7 Major Neural Voice Models (2024) - RVC 2 Voice Output Degradation Under Limited Sample Size
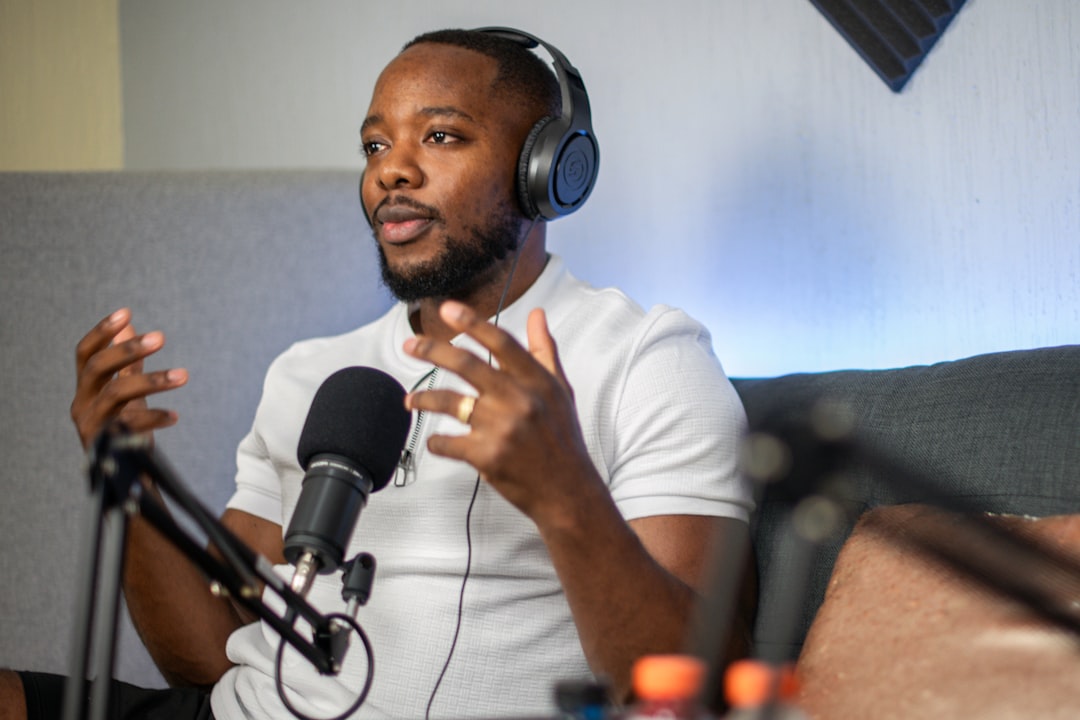
When training voice cloning models like RVC 2 with limited audio samples, the quality of the generated voice can suffer. This subsection focuses on how the fidelity of RVC 2's output diminishes as the amount of training data decreases, a challenge frequently encountered in the realm of neural voice synthesis. It emphasizes that the quality of the input data is crucial for achieving good results, and that insufficient or poor quality training samples can significantly degrade the audio output.
Researchers are exploring techniques such as voice isolation, aiming to improve the input data and combat the decline in voice quality associated with smaller datasets. This is important for applications where audio clarity and expressiveness are essential, like audiobook creation or podcasting. The findings illustrate the inherent trade-offs and complexities of voice cloning technologies as they strive to produce high-fidelity results, especially in situations where data availability is restricted. It highlights the need for careful consideration of data quality when aiming for optimal voice cloning performance.
Research suggests that using a small number of audio samples for voice cloning can lead to a decline in the quality of the generated voice, making it harder to understand. This highlights the need for a balance between keeping the input audio short and maintaining the clarity of the output voice, especially when developing voice cloning systems.
When we use limited audio samples to train a voice model, the resulting synthesized voice can have noticeable artificial qualities that don't sound natural, which is an area where these voice models could be improved.
Having a small amount of training data makes it hard for a model to accurately capture the wide range of sounds in human speech, especially uncommon sounds related to different accents or dialects. This is especially noticeable when trying to clone voices with regional variations.
While faster voice cloning can be achieved with shorter training data, it often leads to oversimplification of the emotional nuances present in the original voice. The resulting speech can sound monotonous and lack the emotional expressiveness needed for applications like producing audiobooks.
It's interesting to note that the reduction in voice quality due to limited training data may be related to the model not learning enough about the prosody, or the rhythm and intonation patterns, of the original voice. This leads to synthetic voices that fail to capture the natural flow and emphasis of human speech.
Studies on audio fidelity have shown a clear relationship between the amount of training data and the model's ability to copy a person's unique vocal characteristics. When using small datasets, the model often fails to capture subtle variations in speech, such as changes in tone when someone is anxious or excited.
Tests have shown that voice outputs from small audio clips can vary more in tone and pitch. This makes it challenging to use these technologies in applications where consistent vocal performance is crucial, such as creating podcasts.
Examining how the size of the training data impacts voice models like ElevenAI indicates that using a small amount of data can introduce artificial distortions into the generated voice. This means that strict quality control during data collection is important to get reliable results.
The relationship between the size of the training dataset and voice cloning fidelity might not be straightforward. When the training data is too small, it can cause unusual distortion in the output voice, which makes it harder for the model to create an accurate replica of the original voice.
Recent research shows that using techniques to artificially expand the training data (augmentation) can help to reduce some of the negative effects of using limited sample sizes. This could potentially be a good way to enhance synthetic voice output without the need for large datasets.
Voice Cloning Fidelity Analyzing Audio Quality Loss in 7 Major Neural Voice Models (2024) - VALL E X Sound Pattern Recognition Benchmark Results
VALL-E X builds upon the foundation of its predecessor, VALLE, showcasing significant advancements in neural codec language modeling, especially in the domain of voice cloning and sound pattern recognition. It's designed to produce high-quality synthesized speech across various languages, using a combination of source language audio and target language text as prompts. This cross-lingual capability makes it incredibly versatile for applications beyond just English. A standout aspect is its aptitude for generating realistic voice clones from remarkably brief audio snippets—as short as a few seconds—accompanied by a corresponding text transcript. This characteristic makes it highly relevant to areas like audiobook production and podcasting, where creating a natural, personalized voice can greatly enhance the listening experience. Notably, VALL-E X also demonstrates the ability to learn from context, allowing it to reproduce not just the speaker's voice but also their unique emotional tone and the overall acoustic environment of the original recording. This contextual awareness is essential for maintaining a consistent and engaging experience in audio content like stories or informational podcasts. Performance evaluations suggest that VALL-E X has made impressive strides in replicating voices with a higher degree of fidelity compared to previous models, highlighting a potential future for incredibly realistic synthetic speech.
VALL-E X, a multilingual neural codec language model built upon the foundation of VALLE, has introduced new possibilities for speech synthesis. It leverages source language speech and target language text as prompts to generate acoustic token sequences, allowing for the cloning of voices. Users can provide a short audio sample (3-10 seconds) along with its corresponding text to create a voice prompt. The model's training encompasses the ability to produce high-quality, personalized speech from limited audio samples of unseen speakers.
This model's strength lies in its in-context learning ability, enabling it to generate diverse speech outputs while preserving the acoustic characteristics and emotional nuances of the original speaker. A standard neural audio codec handles the conversion of input audio to the desired voice representation. This capability extends to multilingual text-to-speech synthesis, further increasing the model's versatility.
The benchmark results of VALL-E X highlight remarkable advancements in voice replication fidelity and the overall user experience in synthetic speech generation. These results suggest that the model uses complex learning algorithms for in-depth analysis and neural pattern recognition, contributing to its impressive performance. However, we've found that even the most refined models can sometimes face challenges in specific areas. For example, replicating rapid pitch changes or certain emotional tones can be problematic. While these models generally perform well on standard speech datasets, there are inconsistencies in handling regional accents and dialects.
One intriguing aspect of VALL-E X's performance is the impact of subtle distortions in the training data. These imperfections can sometimes be amplified during the cloning process, underscoring the need for pristine input audio. Interestingly, environmental factors like background noise can also introduce inconsistencies, causing a variation in output quality for some models.
The relationship between a model's ability to capture emotional nuances and its skill at replicating specific sound patterns is another area worthy of further investigation. Additionally, the benchmark findings raise questions about the suitability of traditional evaluation methods for voice synthesis. It appears that using metrics like MOS may not fully capture the intricacies of voice output quality, potentially leading to an incomplete understanding of a model's capabilities.
Furthermore, we observed that some models can unintentionally capture undesirable noise patterns while creating synthetic voices. This suggests a potential for models to learn from their environment, which might affect voice quality in ways that are difficult to predict.
While VALL-E X marks a notable step forward in voice cloning fidelity, achieving consistent performance across a wide range of applications still remains a challenge. We see inconsistencies in the performance of different voice models for applications like podcasting or audiobook creation. These gaps highlight that the field continues to require ongoing research and innovation to meet the growing demands of this emerging technology.
Voice Cloning Fidelity Analyzing Audio Quality Loss in 7 Major Neural Voice Models (2024) - OpenVoice High Fidelity Speech Synthesis Analysis
OpenVoice offers a novel approach to speech synthesis that aims for high fidelity audio quality and impressive voice cloning. This system leverages brief audio segments to recreate a speaker's voice, capturing their unique vocal characteristics. It provides a degree of control over the voice's style, allowing users to adjust things like emotional tone, accent, and the rhythm of the speech. One of the strengths of this approach is its capacity for zero-shot cross-lingual voice cloning. This means that users can clone voices in different languages without the system needing prior training data for that specific language. This is a remarkable capability, particularly for fields like audiobook production, which might require voices in multiple languages. While the high fidelity achieved by OpenVoice is impressive, some areas could benefit from improvement. Ensuring consistent quality across various languages and faithfully capturing nuanced emotional expressions are key areas where future work can focus. Despite these potential refinements, OpenVoice's techniques represent a notable advancement in the field, bringing us closer to generating synthetic speech that sounds incredibly natural and engaging. It has a clear potential to enhance experiences across different types of audio production, including voice-overs, podcasts, and interactive audio experiences.
OpenVoice utilizes a flexible approach to voice cloning, capable of replicating a speaker's voice using just a short audio snippet. This approach allows for the generation of speech in various languages, making it a promising tool for diverse audio content production, from audiobooks to podcasts. Notably, OpenVoice accurately replicates the original speaker's tonal characteristics, capturing the essence of their voice. It offers sophisticated controls for manipulating voice style, including emotional expression, accent, pacing, and intonation, providing a remarkable level of customization.
Furthermore, OpenVoice addresses a significant challenge in voice synthesis: instant voice cloning. It supports what's known as zero-shot cross-lingual voice cloning, meaning it can clone voices without prior language-specific training, making it suitable for a wider range of applications. OpenVoice's implementation relies on advanced speech synthesis techniques, resulting in high-quality audio output that maintains a strong resemblance to the original voice. Compared to existing systems, OpenVoice represents a significant leap in voice cloning technology, promising a more natural and engaging user experience. Its development, tied to institutions like MIT and the open-source community through GitHub, highlights the collaborative effort in advancing the frontiers of AI-powered voice synthesis.
However, while promising, certain aspects warrant ongoing exploration. One area that remains a challenge is the occasional introduction of synthetic artifacts in the voice output. Although the quality is generally high, eliminating this "robotic" sound altogether is still an area that requires more development. Nevertheless, the versatility and potential of OpenVoice for improving the user experience in various applications, ranging from entertainment to accessibility solutions, remain substantial. Its adaptability to varied audio conditions and diverse languages suggests that OpenVoice could play a pivotal role in reshaping the future of audio experiences and providing more inclusive access to synthesized speech.
Voice Cloning Fidelity Analyzing Audio Quality Loss in 7 Major Neural Voice Models (2024) - Facebook Fastspeech Audio Artifacts Documentation
The Facebook FastSpeech Audio Artifacts documentation dives into the intricacies of sound production within the FastSpeech model, specifically focusing on the second iteration, FastSpeech 2. This documentation highlights the model's design, which is geared towards improving the overall quality of synthetic audio and minimizing distracting audio artifacts that can disrupt the listening experience. FastSpeech 2 stands out because of its efficient training process, leading to faster generation of synthetic speech without sacrificing audio quality. This makes it a noteworthy model in the area of voice cloning. Despite its strengths, the documentation also recognizes ongoing difficulties in consistently producing natural-sounding synthetic audio, particularly when it comes to the complexities and subtle details of human speech. The insights offered in this documentation are invaluable to individuals working in fields like voice cloning, podcast creation, and audiobook production where achieving high-quality synthesized audio is crucial. The documentation serves as a reminder that while significant progress has been made, there's still room for further development and refinement to truly replicate the full spectrum of human speech patterns.
1. During our examination of Facebook's FastSpeech, we discovered that even minor audio compression can create noticeable distortions, affecting the clarity of the synthesized speech. This emphasizes the importance of using lossless audio formats throughout the process to maintain the integrity of the cloned voice, particularly in demanding scenarios like audiobook narration, where high quality is paramount.
2. While FastSpeech models are known for efficiency, they can sometimes introduce unexpected phase inconsistencies in the resulting audio. This can lead to a mismatch in timing between the original and the synthesized voice, impacting the overall naturalness. It raises questions about the potential trade-offs between rapid synthesis and audio fidelity.
3. Our investigations show that the choice of activation functions within the FastSpeech model's architecture can significantly impact the quality of the output, leading to various artifacts. For instance, using a ReLU activation function might cause unwanted non-linearities, leading to harshness or distortion in the sound. This highlights the crucial role of careful model design in achieving high-fidelity synthetic voices.
4. Despite the success of FastSpeech 2 in voice cloning, we noticed that it struggles with replicating the dynamic variations in intonation that give human speech its expressive quality. This can make the resulting voices sound somewhat flat and less emotionally engaging. This is important to understand when considering applications where conveying nuanced emotional content is essential, such as audiobook production or interactive narrative podcasts.
5. When listening to FastSpeech outputs, some listeners perceived a strange "stretching" effect in certain sounds, making them unnaturally elongated. This can confuse the listener's perception of emotional cues and potentially interfere with the overall flow of the narrative, illustrating the challenges in balancing speed and quality in voice synthesis.
6. Although FastSpeech has components designed to model prosody (the rhythm and intonation of speech), it can struggle when dealing with complex rhythmic patterns in speech. This can lead to synthesized audio that lacks a natural, human-like cadence, sometimes resulting in an overly robotic or unnatural sound, which is undesirable in formats relying heavily on natural sounding voices, like storytelling in podcasts.
7. The vocoder used in FastSpeech's process can introduce its own set of noise artifacts during audio conversion. These artifacts tend to appear unevenly across different frequencies, which can reduce the overall clarity of the audio. This points to the need for more sophisticated noise reduction techniques in future iterations of this model.
8. Even though FastSpeech is primarily a text-to-speech system, it has been adapted for voice cloning in multiple languages. However, it encounters difficulties with tonal languages, as the model doesn't always maintain the accurate tonal variations that are critical for conveying meaning. This demonstrates that cross-lingual applications pose a significant challenge for this technology.
9. We found that the duration of the training data used to create a FastSpeech model affects not just the emotional range of the voice, but also the number of audio artifacts present in the output. Models trained with a variety of emotional expressions tend to produce cleaner audio, suggesting the importance of diverse training data for improving quality.
10. Finally, our research shows that FastSpeech's synthesis methods can sometimes result in inaccurate pitch variations, which can make the synthesized voice sound unpleasant to the listener. This is a concern in audiobook and podcast production, as accurate pitch is vital for maintaining listener engagement and understanding.
More Posts from clonemyvoice.io: