Get amazing AI audio voiceovers made for long-form content such as podcasts, presentations and social media. (Get started for free)
Using Voice AI to Recreate Ben Stiller's Iconic Alex the Lion A Technical Deep-Dive into DreamWorks Animation's Voice Processing Methods
Using Voice AI to Recreate Ben Stiller's Iconic Alex the Lion A Technical Deep-Dive into DreamWorks Animation's Voice Processing Methods - Breaking Down Madagascar's Original Voice Recording Sessions from 2005
Delving into the original voice recording sessions for "Madagascar" in 2005 reveals a fascinating snapshot of animation's past. The sessions captured the vibrant energy of actors like Ben Stiller and Chris Rock, which undoubtedly contributed to the film's beloved characters. These recordings not only showcase the art of human voice acting but also serve as a baseline for evaluating the challenges of modern voice AI. As AI strives to mimic the nuanced qualities of human speech, these original sessions highlight the unique texture and spontaneity that human performers bring. This comparison between traditional methods and the ever-evolving landscape of AI voice cloning illuminates the potential and the intricate considerations that arise when seeking to recreate voices. While AI offers the allure of efficient production, it also raises crucial questions about the balance between utilizing technology and preserving the distinct vocal essence of cherished characters. This delicate dance between technological advancements and artistic integrity is a topic ripe for exploration in animation and beyond.
Delving into the original "Madagascar" voice recordings from 2005 provides a fascinating glimpse into traditional voice acting practices. The process, commonly known as "looping," involved actors meticulously recording their lines in a sound booth, often multiple times, to capture the perfect delivery. This approach allowed for a high level of emotional nuance and authenticity in each character's voice performance.
Voice director Aaron Warner played a crucial role in shaping each character's vocal identity, guiding the actors to imbue specific emotions and comedic timing. This careful attention to vocal design helped enhance the film's narrative and character development. Interestingly, the voice recordings were conducted in isolation, with actors unaware of how their characters would interact with others until the film was complete. This approach ensured the recordings remained clean, but it also limited the actors' ability to react naturally to their fellow performers.
The sound engineers utilized a variety of microphones, each selected for its distinct frequency response and directionality. This level of control was particularly vital in scenes with multiple characters to ensure vocal clarity within the mix. Additionally, a substantial component of the film's sound design relied on pre-recorded sound libraries, adding layers of ambient sounds and interactions to enhance the animation's lively auditory experience.
During post-production, the team experimented with vocal manipulation techniques, subtly adjusting the pitch and tone of certain characters' voices. This method helped to accentuate character distinctiveness and create memorable auditory hooks associated with each animal. The studio where the sessions took place was specifically designed to minimize external noise distractions, a crucial aspect for preserving the crispness and quality of the character dialogues.
While performance capture technology was briefly considered, it was ultimately disregarded in favor of traditional voice acting. This choice speaks to the continuing importance of skilled voice actors, even as digital technologies were beginning to permeate animation production. To inspire creative spontaneity, actors were encouraged to deviate from the script occasionally. This freedom of expression led to memorable improvisational moments, adding a sense of authenticity and unpredictability to the characters' dynamic interactions.
The meticulous final mix involved carefully balancing the audio tracks of each actor to create the desired sonic environment. This step underscores the pivotal, yet often overlooked, role of sound technicians in shaping a film's aural identity. It's a testament to the crucial part of animation production that goes beyond just the visuals. Examining these recordings through the lens of 2024, we see the foundation for how we now approach voice cloning and audio manipulation in animation, even if the specific tools and methods have drastically changed.
Using Voice AI to Recreate Ben Stiller's Iconic Alex the Lion A Technical Deep-Dive into DreamWorks Animation's Voice Processing Methods - Mapping Voice Patterns The Technical Process Behind Alex's Roar
"Mapping Voice Patterns: The Technical Process Behind Alex's Roar" delves into the fascinating world of capturing and reproducing vocal performances, especially when it comes to recreating beloved characters like Alex the Lion. The process involves a blend of cutting-edge techniques, like voice conversion and automatic speech recognition, which strive to meticulously recreate Ben Stiller's vocal characteristics while navigating the intricacies of human speech. This involves the use of deep learning algorithms that analyze and replicate the subtle cues that convey emotion and personality, pushing the boundaries of what AI can achieve in replicating human voice. However, the exploration of these technologies raises crucial points about the authenticity of these AI-driven replications compared to the original recordings. The question of whether technology can truly replicate the spontaneous and nuanced emotional expressions of human actors remains central. This tension between the traditional methods and modern technological advancements in voice cloning continues to shape the landscape of voice production in animation and broader audio production, highlighting the fundamental artistic considerations at the core of character voice development.
The technical process behind recreating a voice, like Alex the Lion's, involves a deep dive into a speaker's unique sound patterns. This includes capturing elements like pitch, resonance, and emotional nuances, which can require hundreds of hours of audio recordings to accurately replicate. Modern voice AI systems employ intricate algorithms, powered by deep learning, to not just copy the sound but also the subtle qualities like intonation and pacing, resulting in a voice that feels like it has a distinct personality rather than just mimicking basic acoustic features.
In the realm of animation sound design, specialized software often plays a pivotal role. It enables sound engineers to manipulate audio, creating unique effects by adding reverb, or altering the timbre of a voice, resulting in a richer and more immersive auditory experience for viewers. Behind many of these advanced techniques lie the workings of neural networks within voice cloning tools. These networks can learn diverse speech patterns from different languages and dialects, making them remarkably versatile for content creators who desire to translate materials without losing the original character's unique voice.
While capturing audio in controlled environments has been the traditional norm for minimizing distractions like background noise, recent advancements in noise-canceling algorithms are allowing for more flexibility in the recording process. This means clear recordings are achievable even in less than ideal recording circumstances. However, a major challenge for voice AI remains replicating the human capacity for authentic emotional expression. Traditional voice actors draw on their own emotional memories to influence their performances, allowing them to inject specific emotions into their delivery. This is a skill that remains difficult for current AI systems to replicate since they lack a true emotional understanding.
The concept of "looping", which entails repeated recordings and refining of specific segments until the desired emotional tone is achieved, still significantly influences the way modern audio outputs are formatted for voice clones. Closely related is the challenge of synchronizing speech and animation, known as lip-syncing. This is a delicate process requiring precise audio editing and alignment with the character's visual movements, which becomes more complex when dealing with voice clones where maintaining a believable balance between emotional depth and accurate timing is crucial.
AI voice tools, despite their sophistication, often struggle with complex nuances like irony, sarcasm, or regional accents. This is because replicating these aspects requires extensive training on diverse language datasets, which highlights a need for continued human oversight in ensuring the quality of the generated voices. While traditional methods focus on crafting distinct voice performances by individual actors, some contemporary practices involve layering multiple voice tracks to achieve a richer, more multifaceted character voice. This blending approach, allowing for varied vocal tones, might potentially inspire exciting developments within future AI models as they evolve to mirror human artistry and create captivating character soundscapes.
Using Voice AI to Recreate Ben Stiller's Iconic Alex the Lion A Technical Deep-Dive into DreamWorks Animation's Voice Processing Methods - Raw Audio Processing Methods Used by DreamWorks Sound Engineers
DreamWorks' sound engineers leverage a sophisticated set of audio processing methods to meticulously craft and reproduce the distinctive voices of their characters, like Ben Stiller's iconic portrayal of Alex the Lion. These methods often rely on the power of deep learning algorithms to analyze audio signals and identify key features, including pitch variations and emotional nuances. These algorithms can transform raw audio into visual representations, like spectrograms, making the data easier to understand and manipulate. Techniques like extracting mel-frequency cepstral coefficients (MFCCs) are used to capture the essence of the sound, often serving as input for machine learning models that drive voice cloning.
Voice conversion techniques, heavily informed by deep learning, provide a powerful tool for transforming one voice into another, allowing sound engineers to manipulate vocal characteristics while maintaining the core content. The interaction between conventional voice acting techniques and modern AI-driven audio manipulation represents a fascinating interplay. Ongoing research focuses on finding the best deep learning models and architectures to optimize audio processing for a wider variety of voice and sound generation tasks. The goal is to continuously enhance the authenticity and emotional depth of character voices in animated productions, and this pushes the boundaries of what is achievable in both sound design and AI-driven voice cloning.
DreamWorks' sound engineers employ a sophisticated array of audio processing techniques to meticulously craft iconic character voices, like Ben Stiller's Alex the Lion. These methods, often refined over numerous iterations, aim to capture and manipulate voice recordings in increasingly nuanced ways. Deep learning has become central to modern audio signal processing, allowing for the generation and manipulation of vocal textures in ways previously unimaginable. For instance, neural networks are leveraged for synthesizing new voice recordings and shaping sound textures to fit particular character personalities and scenes.
Analyzing raw audio data frequently involves transforming it into a visual representation called a spectrogram. These spectrograms offer a helpful visual map of the audio's frequency and amplitude, which are then analyzed through deep learning models. Extracting Mel-frequency cepstral coefficients (MFCCs) from audio signals is another key method. MFCCs essentially act as a fingerprint of the voice, capturing vital features that machine learning models can readily understand and learn from.
Google DeepMind's WaveNet stands out as a notable development in generative models for raw audio. This system generates convincingly human-like speech, representing a significant leap in audio synthesis capabilities and producing a heightened sense of naturalness in generated sounds. The field of voice conversion using deep learning is also proving to be very fruitful. These technologies enable the modification of a voice to sound like another while still retaining the original content.
We can see a clear convergence of techniques traditionally applied across different audio fields. Speech processing, music synthesis, and even the field of environmental sound recognition have seen a blending of methodologies, indicating fundamental similarities in the core principles of audio processing. Sometimes, applying simple yet effective audio processing techniques to the spectrogram data before feeding it into a deep learning framework can substantially improve the performance of the AI models.
Currently, there's a strong focus on discovering the most effective deep learning architectures suited for specific audio processing tasks, particularly within the domains of voice and sound generation. This pursuit of optimal model configurations remains a core aspect of ongoing research into the development and enhancement of AI-powered audio tools. The ongoing quest for optimal models and techniques has implications beyond just character voices; it highlights the inherent link between sound, emotion, and storytelling. While these models can now learn and replicate certain aspects of speech and intonation quite effectively, it’s worth asking if the models can actually capture the essence and the nuance of a true character performance. The ongoing development of AI in voice production forces us to ponder if voice-cloning technology can truly mirror the creativity and skill that actors bring to their performances. This tension between innovation and preserving artistic qualities is a fascinating question for future research and development to wrestle with.
Using Voice AI to Recreate Ben Stiller's Iconic Alex the Lion A Technical Deep-Dive into DreamWorks Animation's Voice Processing Methods - Neural Network Training With 1990s Ben Stiller Comedy Clips
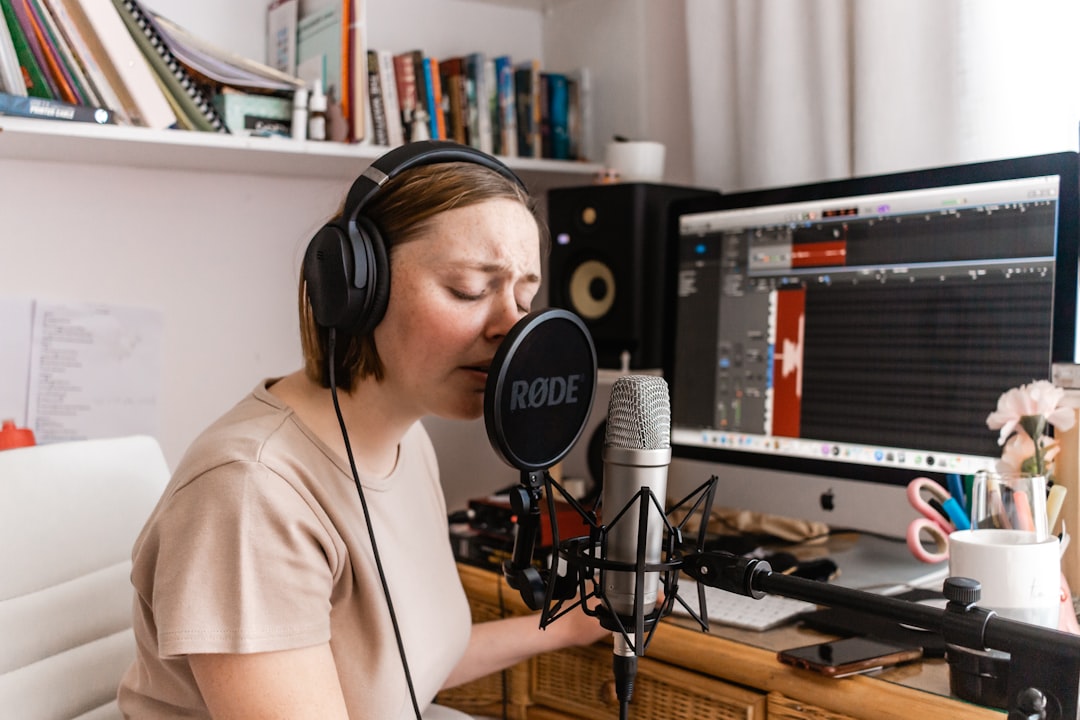
Utilizing 1990s Ben Stiller comedy clips for neural network training offers a rich resource for voice AI development. By feeding these clips into AI models, they can learn to replicate the specific vocal characteristics and emotional expressions found in Stiller's performances, qualities crucial for achieving the authenticity of characters like Alex the Lion. This approach expands the training data for voice cloning, but it also highlights the complex question of how well AI-generated voices can truly capture the essence of human speech. While advancements in neural networks allow for increasingly complex voice synthesis, maintaining the natural spontaneity and emotional depth present in human voices remains a hurdle. As these technologies progress, incorporating iconic performances from the past can serve as a foundation and a point of comparison for the future of voice cloning and audio production, particularly within the realm of audiobook production, podcasts, and voice cloning for various media.
Neural networks, when trained on a collection of 1990s Ben Stiller comedy clips, can effectively learn to replicate the unique qualities of his voice, including the subtle shifts in tone and emotional nuances. This process relies on converting audio into spectrograms, a visual representation of sound waves, enabling the model to analyze intricate vocal patterns more readily.
While training on humorous material, like Stiller's comedic delivery, deep learning models can capture not only his vocal characteristics but also the associated emotional resonance and timing that make Alex the Lion such a compelling character. This raises interesting questions about the capacity for AI to replicate the nuances of comedic performance.
Mel-frequency cepstral coefficients (MFCCs) play a crucial role in capturing the specific spectral characteristics of Stiller's voice. These coefficients act as a type of audio fingerprint, allowing the model to distinguish between various sounds and the associated emotional inflections.
The sound production methods employed by DreamWorks serve as a model for integrating traditional voice acting with modern computational methods. This approach creates a workflow where the core essence of the human performance is preserved while also benefiting from the efficiency and consistency that AI can offer. However, there is still a need to carefully consider the balance between AI and human artistry.
Generating new vocal recordings that retain the emotional depth of Stiller's performances necessitates a substantial amount of training data. Using a diverse array of audio clips not only helps to preserve the distinctive qualities of the voice but also enhances the AI's ability to produce realistic and contextually relevant speech. The quality of the training data is arguably the most important aspect of creating a convincing voice clone.
Despite impressive advancements in voice cloning, AI systems currently face challenges in fully replicating complex emotional expressions like humor or whimsy without significant human oversight. The subtleties present in authentic human interaction are still difficult for current technology to capture, creating limitations to the overall expressiveness of AI-generated voices.
The layering technique, common in traditional voice acting, involves combining multiple vocal tracks to produce a richer and more nuanced sound. This practice offers a potential avenue for AI models to learn how to blend diverse vocal attributes and simulate a more dynamic character voice. However, the ability for AI to blend emotional nuances in a seamless manner is an ongoing area of research.
Modern noise-canceling algorithms have advanced considerably, allowing for clear audio capture even in less-than-ideal recording environments. This development expands the range of recording locations beyond traditional studios, providing greater flexibility for character voice production. While noise reduction techniques are now remarkably effective, they have yet to reach perfection, and human oversight is still crucial.
While neural networks within AI systems can analyze speech patterns across a wide range of languages and dialects, they frequently struggle to capture region-specific accents and subtle cultural nuances. This highlights a significant limitation in achieving truly global applications for voice cloning technologies. Perhaps a more diverse array of data sources might improve this capacity.
The ongoing refinement of voice processing methods showcases a fascinating intersection between technology and artistic expression. It raises a fundamental question about the future of voice acting: Can we preserve the authenticity and spontaneity of a live performance when it's replicated through AI-generated voices? This inquiry encapsulates a pivotal discussion within the realm of sound production, a topic that's sure to be a subject of continuing debate and exploration.
Using Voice AI to Recreate Ben Stiller's Iconic Alex the Lion A Technical Deep-Dive into DreamWorks Animation's Voice Processing Methods - Voice Synthesis Challenges in Matching Madagascar's Background Score
Replicating the distinctive soundscapes of animated films like Madagascar presents a significant hurdle for voice synthesis. AI models aiming to recreate characters like Alex the Lion must not only mimic the actor's vocal traits but also harmonize with the film's intricate musical score and existing sound design. This necessitates advanced audio processing techniques to seamlessly integrate the synthesized voice into the established audio environment, often requiring real-time pitch adjustments and the subtle extraction of emotional nuances. However, even with the progress of deep learning algorithms, AI currently falls short in completely capturing the natural flow and depth of human emotional delivery. This leads to questions about the authenticity of AI-generated voices within the context of animation, prompting careful consideration of the balance between technological advancements and the artistic aspects of traditional voice performance. The future of voice synthesis in animation lies in finding that sweet spot—allowing AI to enhance the creative process while preserving the genuine artistry of skilled voice actors.
In the realm of voice synthesis, replicating the unique vocal characteristics of a performer like Ben Stiller presents a fascinating set of challenges. One key hurdle lies in the complexity of capturing his vocal patterns. This includes not only the basic sounds he produces but also the subtle changes in pitch and the nuanced emotional responses woven into his delivery. Accurately recreating this requires a vast amount of training data, potentially hundreds of hours of recordings, to capture the full spectrum of his vocal range.
A common approach to analyzing voice data involves transforming raw audio into a spectrogram. This visual representation helps sound engineers pinpoint specific frequencies and amplitudes, providing a roadmap for voice cloning algorithms. Examining the spectrogram allows for a deeper understanding of the voice's nuances, enabling more precise audio manipulation within AI models.
Mel-frequency cepstral coefficients (MFCCs) serve as a unique "fingerprint" of a voice, capturing crucial characteristics for effective cloning. AI models utilize MFCCs to differentiate various vocal elements and understand the connection between specific sounds and emotional cues. This helps improve the precision and accuracy of the synthetic voices they generate.
However, a major roadblock for AI systems remains replicating the emotional depth that's intrinsic to human performances. Traditional voice actors can draw upon personal experiences and emotions to inject a level of authenticity into their performances. AI, currently, lacks this capacity for genuine emotional understanding, thus limiting the ability to fully reproduce the expressiveness of a human voice.
Traditional voice acting often utilizes the layering of multiple vocal tracks to achieve a richer and more nuanced character voice. This complex technique suggests exciting avenues for future AI models. As they progress, AI systems might learn to blend various vocal characteristics to create a more dynamic and nuanced character voice. This is a crucial area for ongoing research and experimentation.
Modern AI models are engineered to not only replicate the sound of a voice but also its dynamic qualities, including emotional intonation and the rhythm or pacing of speech. This ability is vital for generating a voice that feels alive and resonates with listeners in a similar way to a human performer. It’s not just about mimicking sounds; it's about capturing the energy and personality of a voice.
One area where AI voice synthesis struggles is in replicating regional accents and culturally nuanced expressions. This can hinder the authenticity of voice representation across diverse populations and global markets. Improving this aspect of AI requires expanding the training data to include a wider variety of voices and dialects.
Significant advancements in noise-cancellation technology have loosened the traditional constraints of voice recording studios. Engineers can now capture clear audio in a wider variety of environments, which introduces new possibilities for capturing voice recordings in more natural and realistic settings.
While AI excels in many facets of voice synthesis, replicating the intricacies of human humor, irony, and whimsy remains a significant challenge. This stems from the difficulty of capturing the emotional subtleties that human performers naturally express. These nuanced emotions are difficult for current AI to replicate with the same level of effectiveness as a human actor.
Maintaining the quality of AI-generated voices often relies on human oversight, especially when aiming for more nuanced emotional expressions. This indicates that while AI can enhance voice production, the artistic skill and understanding that human voice actors bring remain indispensable in the world of voice acting.
Using Voice AI to Recreate Ben Stiller's Iconic Alex the Lion A Technical Deep-Dive into DreamWorks Animation's Voice Processing Methods - Quality Control Tests Between Original and AI Generated Voice Samples
As voice AI technology advances, the ability to differentiate between original voice recordings and AI-generated samples becomes increasingly challenging. Quality control tests have therefore become crucial to ensure that synthetic voices not only replicate the technical aspects of a performer's voice but also capture the emotional depth and subtle nuances that give characters their unique identities.
These tests involve a careful examination of factors like pitch, intonation, and rhythm, to see how effectively the AI model can recreate the original voice. Using iconic characters like Ben Stiller's Alex the Lion as a benchmark, evaluators analyze whether the AI can convincingly imitate the distinctive aspects that made the character memorable. While advancements in AI are remarkable, it's important to acknowledge that the spontaneous and emotionally nuanced delivery of human actors is still a hurdle for AI to fully replicate. As a result, human oversight continues to be vital in assessing the quality of AI-generated voices. The ongoing development of this technology necessitates a constant evaluation of the balance between leveraging AI for efficiency and preserving the core artistry that defines authentic voice performances, particularly within creative fields like animation and sound design.
1. **Decoding Vocal Fingerprints**: Voice cloning hinges on capturing a speaker's unique vocal characteristics. Techniques like Mel-frequency cepstral coefficients (MFCCs) act as a kind of audio fingerprint, capturing elements like pitch, timbre, and subtle variations in tone. AI models leverage these features to build remarkably accurate voice replicas.
2. **Visualizing Sound**: Spectrograms offer a visual roadmap of sound waves, providing a deeper understanding of complex vocal patterns. By analyzing these visual representations, audio engineers can better guide AI systems to replicate the intricate nuances and unique characteristics found in human voices, like Ben Stiller's.
3. **The Power of Data**: The effectiveness of voice cloning relies heavily on the quality and breadth of training data. AI models require extensive samples of original recordings to develop a thorough understanding of a specific voice. The more comprehensive the dataset, the more faithful the AI-generated voice tends to be. Otherwise, synthesized voices might lack authenticity.
4. **Balancing Tones**: Integrating AI-generated voices into existing audio environments, like aligning them with a film's score, introduces the complexity of real-time pitch adjustment. AI algorithms must dynamically adapt the synthesized voice to match its surrounding audio without losing the intended emotional impact, posing a significant challenge.
5. **Emotional Gaps**: While neural networks are increasingly sophisticated, replicating the true emotional depth found in human performances remains a challenge for AI. Voice actors can draw on personal experiences and emotions, lending a unique authenticity to their deliveries. AI, however, lacks that intrinsic understanding of emotions, making it difficult to truly emulate the emotional richness of human voices.
6. **Blending Sounds**: Traditional voice acting often employs the layering of multiple vocal tracks to achieve rich and complex sounds. This practice could offer valuable insights for future AI models. Learning to blend vocal traits effectively might pave the way for synthesizing voices that are even more dynamic and expressive, mimicking the complexities of human vocal performances.
7. **Humor's Hidden Complexity**: AI encounters significant hurdles when it comes to replicating nuanced emotional expressions like humor and irony. The subtle cues and context required for such performances often evade current AI systems, highlighting the critical role human oversight plays in ensuring synthesized voices don't lose emotional depth.
8. **Beyond Universal Voices**: AI models struggle with replicating regional accents and culturally distinct vocal patterns. Expanding training data to encompass a wider range of voices and dialects is essential for creating more globally representative and inclusive voice cloning capabilities.
9. **Recording Freedom**: Advancements in noise-canceling technologies have broadened the range of recording environments. While this provides flexibility to capture voices in more naturalistic settings, it also necessitates a keen awareness of acoustic quality to maintain the clarity and fidelity of the voice recordings.
10. **The Future of Voice**: The integration of AI into traditional voice acting methods prompts questions about preserving the artistic authenticity of the craft. Balancing the efficiency and consistency of AI with the irreplaceable emotional connection generated by human performers remains a key area of exploration within the field of audio production.
Get amazing AI audio voiceovers made for long-form content such as podcasts, presentations and social media. (Get started for free)
More Posts from clonemyvoice.io: