Get amazing AI audio voiceovers made for long-form content such as podcasts, presentations and social media. (Get started for free)
The Science Behind Voice Recognition How AI Identifies Unique Vocal Signatures
The Science Behind Voice Recognition How AI Identifies Unique Vocal Signatures - Acoustic Analysis Techniques for Voice Identification
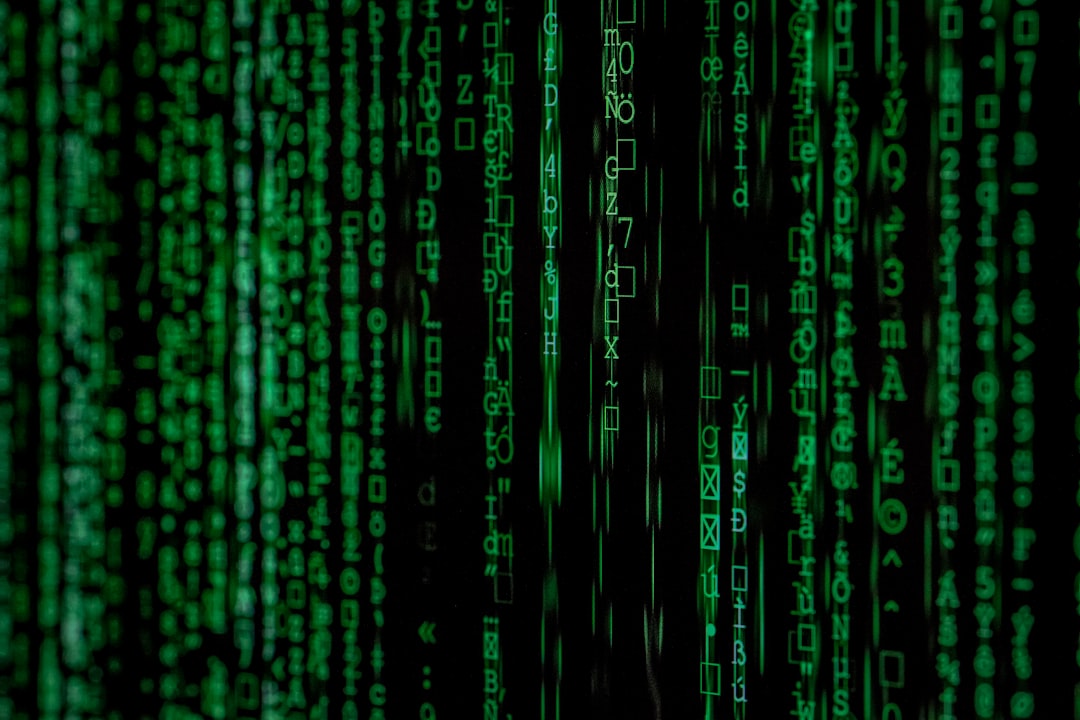
Voice identification relies on acoustic analysis to extract the unique characteristics of a person's voice. This process involves measuring properties like pitch, the spectral envelope (which reflects the shape of the voice's frequency spectrum), and formants (resonances within the vocal tract). These techniques are increasingly powered by machine learning, particularly deep learning algorithms like recurrent neural networks (RNNs) and convolutional neural networks (CNNs). These advanced methods have significantly improved the accuracy of automatic speaker identification systems.
The ability to dissect the intricacies of sound waves has wide-reaching implications. It helps forensic experts in criminal investigations, but also offers potential in healthcare for monitoring vocal cord health. However, despite its progress, voice identification faces challenges. Data quality is a significant concern, with issues like corruption, sparse measurements, and missing information complicating the analysis. As technology advances, we can expect further refinement in these methodologies, paving the way for even more diverse applications, such as enhancing user interaction across various domains.
Acoustic analysis, the backbone of voice identification, delves into the intricacies of sound production to discern the unique vocal signatures that set us apart. Imagine a symphony of frequencies and their subtle variations - that's the essence of what's analyzed. The human voice, a complex instrument, isn't solely shaped by our anatomy; it's also a reflection of our linguistic history and cultural influences. This intertwining of biological and environmental factors creates a truly unique vocal fingerprint, making it invaluable in voice recognition systems.
Beyond just pitch and timbre, we also have formant frequencies - resonant frequencies of our vocal tract - that paint a picture of our vowel sounds. Visualizing these formant frequencies through spectrograms provides a deeper insight into our individual vocal traits, much like a fingerprint.
The very act of breathing, with its subtle variations in airflow and intensity, can also be measured and analyzed to further differentiate between voices. The way we breathe shapes our voice, contributing to its unique character and even reflecting our emotional states. It's fascinating to consider that these subtle nuances of breath, often overlooked, can provide a deeper understanding of human speech.
But it's not all smooth sailing. The challenges of noise are significant. Background sounds can muddy the clarity of our voice signals, highlighting the vital role of signal processing in enhancing recognition accuracy. Just as important is the understanding of the limitations of our perception. The phenomenon of "phantom word perception," where listeners hear sounds that aren't present, reminds us of the inherent subjectivity of human hearing and the need to develop robust systems that can accurately interpret vocal inputs.
The Science Behind Voice Recognition How AI Identifies Unique Vocal Signatures - Neural Networks in Speech Pattern Recognition
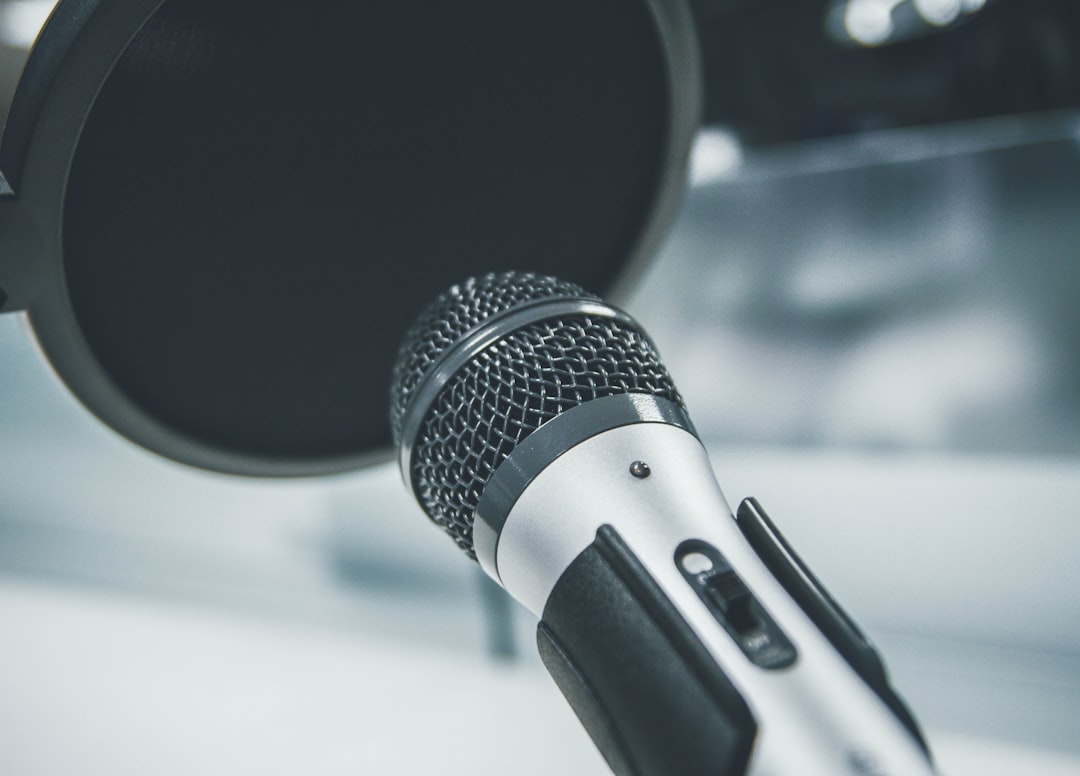
Neural networks have revolutionized how we analyze speech patterns. Deep learning methods, like DNNs and RNNs, allow computers to understand and process the intricate details of human voices. These techniques have significantly enhanced automatic speech recognition systems, enabling them to convert spoken language into text with impressive accuracy. However, challenges remain. Variations in dialects, natural speech patterns, and background noise can still interfere with the systems' performance. Despite these hurdles, research continues to refine these neural networks, aiming to improve their ability to recognize speech accurately in various contexts. This holds exciting potential for voice cloning applications, the production of audiobooks, and the development of more intuitive interactive voice systems. The evolution of these technologies will inevitably shape the way we communicate and create in the world of audio.
Neural networks are becoming increasingly sophisticated in their ability to analyze and understand the complexities of human speech. Beyond simply identifying the speaker, these systems are now delving into the nuanced world of vocal nuances, allowing them to recognize and interpret the unique features of individual voices.
For instance, they can model the resonating frequencies within our vocal tracts, known as formants. These resonances are crucial for distinguishing between similar-sounding voices, particularly important for applications like voice cloning where replicating the fine details of a voice is paramount.
The ability to analyze how pitch and tone fluctuate over time is another key area of progress. Recurrent neural networks (RNNs) excel at capturing these temporal dynamics, enhancing the system’s ability to identify speakers even in situations where emotional states or speech patterns vary. This allows for more robust speaker identification in dynamic situations like podcasts or audiobooks.
Moreover, neural networks have started to exploit the power of self-supervised learning. This means they can learn from unlabeled audio data, significantly reducing the need for extensive and often cumbersome labeled datasets. This has the potential to revolutionize voice recognition, making it more accessible and practical.
The ultimate goal, however, goes beyond simple identification. Neural networks are being trained to extract emotional information from speech. By analyzing variations in pitch, tone, and rhythm, they can infer a speaker's emotional state, demonstrating that a voice carries far more than just linguistic content.
There's still much to explore in this exciting field, but the potential applications are far-reaching. From enabling more natural interactions with voice assistants to enhancing user experience in podcasts and audiobooks, the capabilities of neural networks in speech recognition continue to evolve and refine, bringing us closer to a future where technology truly understands and responds to the human voice.
The Science Behind Voice Recognition How AI Identifies Unique Vocal Signatures - Phoneme Segmentation and Feature Extraction
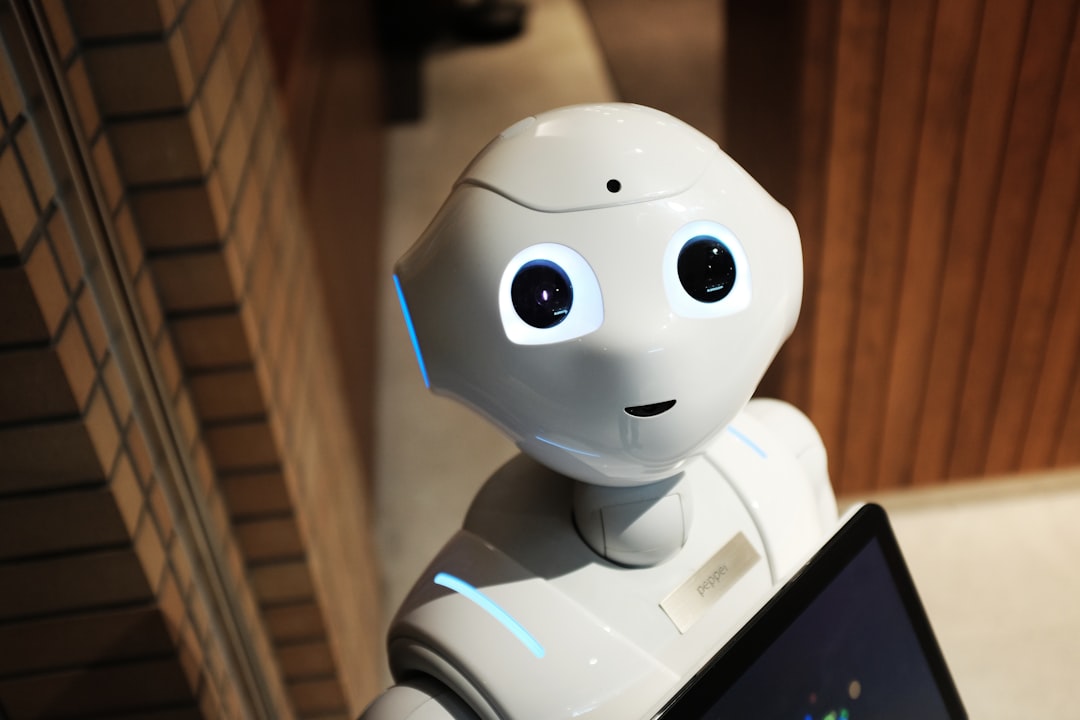
Phoneme segmentation and feature extraction are the core processes that enable machines to understand human speech. Imagine these processes as the foundation of voice recognition technology, taking a continuous stream of sound and breaking it down into its smallest meaningful units – phonemes. These phonemes, like the individual letters of a word, are then analyzed to identify unique characteristics that define a voice.
Getting phoneme boundaries right is crucial. It's like accurately placing the spaces between words in a sentence. Without precise segmentation, the whole meaning gets lost. This is especially important for tasks like voice cloning, where capturing every nuance of the original voice is critical. Audiobooks also benefit from accurate phoneme recognition, making the synthesized voice sound more natural and engaging.
Feature extraction goes beyond simply identifying phonemes. It delves into the intricacies of how these sounds are produced, analyzing aspects like pitch, tone, and the resonance of the vocal tract. These features are like a unique fingerprint, allowing the system to differentiate between voices and even understand emotional states.
The process of phoneme segmentation and feature extraction is not without its challenges. It's difficult to reliably identify phonemes when they sound similar, especially when trying to separate subtle variations in speech. However, researchers are continually refining algorithms, leveraging powerful deep learning techniques to create more accurate and robust systems. The future of this field is promising, holding the key to more intuitive voice interfaces, advanced speech synthesis, and ultimately a deeper understanding of the human voice.
The fascinating world of voice recognition relies on the ability to break down speech into its fundamental building blocks: phonemes. Phoneme segmentation, the process of identifying and separating these distinct sound units, is critical for accurately characterizing a person's voice. Imagine dissecting a spoken word into its individual sounds, like separating the syllables of "hello" into "h-e-l-l-o". Each phoneme holds unique acoustic properties, allowing algorithms to analyze a speaker's voice in great detail.
This process of phoneme segmentation is particularly important for voice cloning applications, where replicating the fine details of a voice is crucial. Machine learning algorithms, trained on vast amounts of audio data, learn to distinguish between these subtle variations in sound, ultimately capturing the essence of a speaker's unique vocal signature. The ability to analyze these intricate sound patterns, from the briefest pauses between phonemes to the fluctuations in their duration, is what enables voice cloning systems to create remarkably realistic simulations of human voices.
However, accurately extracting and interpreting these phonemes isn't without its challenges. Background noise, for instance, can significantly hinder the process, adding unwanted interference to the signal. Advanced signal processing techniques are essential to filter out these extraneous sounds, ensuring that the phoneme segmentation is accurate and reliable. This task becomes even more complex in dynamic environments like podcasts, where multiple sound sources often compete for attention.
Beyond noise, another hurdle is the human element. The phenomenon of "phantom word perception," where individuals perceive words that are not present in the audio, reminds us that human hearing is inherently subjective. To create robust and accurate voice recognition systems, it's essential to acknowledge these limitations and build systems that can consistently and reliably interpret vocal inputs, regardless of these perceptual biases.
In essence, phoneme segmentation plays a critical role in unraveling the complexities of human speech. It allows us to delve into the very fabric of our voices, revealing the nuanced acoustic patterns that make each of us unique. The continuous pursuit of improvements in phoneme segmentation is essential for the evolution of voice recognition technology, paving the way for even more sophisticated and versatile applications in the future.
The Science Behind Voice Recognition How AI Identifies Unique Vocal Signatures - Biometric Voice Authentication Systems
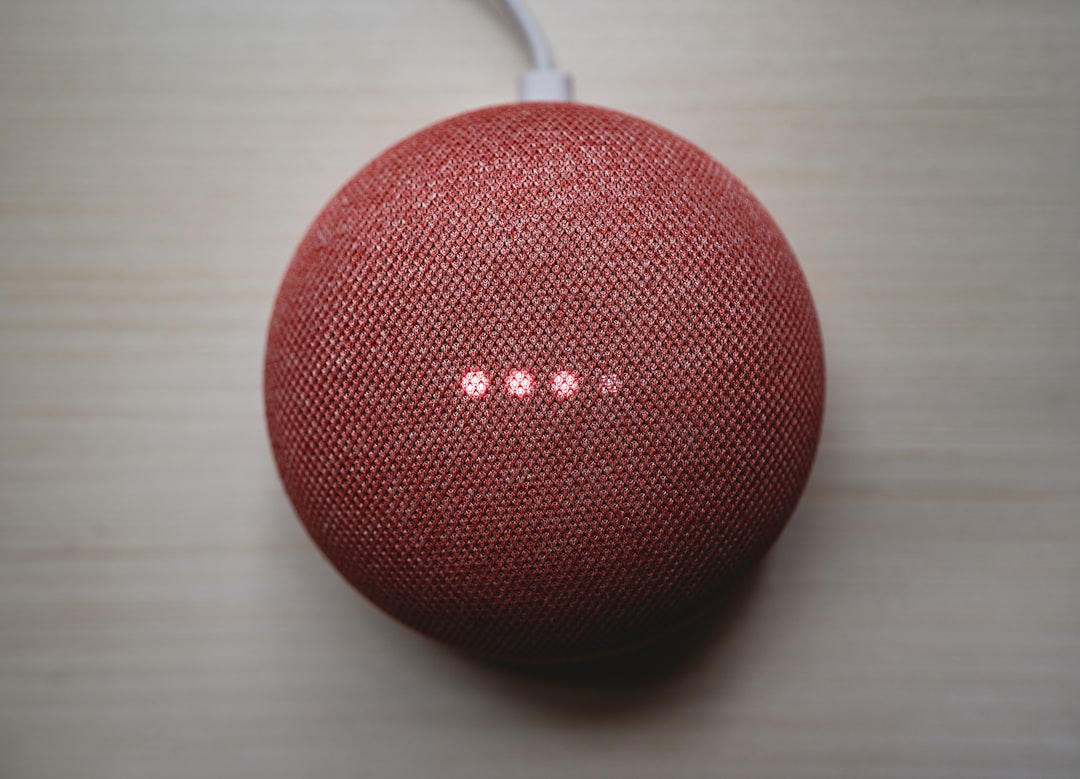
Biometric voice authentication systems work by recognizing the unique patterns in someone's voice. Just like fingerprints, each person has a distinctive vocal signature that can be used to verify their identity. These systems are becoming increasingly popular because they are convenient and user-friendly, requiring only a spoken phrase. They can help streamline the authentication process and provide a more engaging user experience.
However, while voice biometrics holds promise, it faces its own set of challenges. Background noise can significantly interfere with the system's accuracy, making it harder to accurately identify the speaker. There's also the growing concern of unauthorized access through voice cloning, where someone can create a synthetic voice that mimics another person's.
The future success of biometric voice authentication hinges on addressing these concerns and striking a balance between convenience and security. The technology holds potential for widespread use in diverse applications, from customer service and personal device security to creative industries like podcasting and audiobook production.
Biometric voice authentication systems, fascinatingly, go beyond simply recognizing the content of what is spoken. They analyze the unique nuances of how someone speaks, much like a fingerprint identifies a person. This "vocal signature" is affected by a variety of factors, making voice authentication both intriguing and complex.
For instance, the size and shape of our vocal tract, that complex system of throat, mouth, and nose, creates individual formant frequencies—a sort of "sound signature" that distinguishes voices, even if two people utter the same words. Like a musical instrument's unique resonance, our vocal tracts act as natural resonators, producing distinct sounds. This is one of the key ways voice authentication systems can differentiate between speakers.
Beyond physical factors, voice analysis can also delve into the emotional content of speech. It's like picking up on a speaker's emotional "vibe" through their voice. By analyzing pitch and tone variations, biometric systems can potentially infer feelings like happiness or anxiety. Imagine the possibilities for creating more intuitive applications that respond to human emotions!
However, even the most advanced systems face challenges. The quality and type of microphone used to collect voice data can greatly influence the accuracy of the analysis. Directional microphones, for example, pick up sound from specific directions, which is helpful for minimizing background noise, thereby boosting the performance of speaker identification.
Additionally, our voices are not static; they evolve with time. Just as our fingerprints remain unique but change over our lifetime, our voices can be affected by aging, health changes, or even medication. These alterations mean that voice authentication systems must constantly adapt to maintain their accuracy.
Then there's the intricate question of language. Voice recognition systems are becoming more adept at handling variations in accents and dialects within a single language. This adaptability is crucial for designing voice interfaces that work effectively in multilingual settings, such as applications used globally.
And then there's the everyday reality of noise. Imagine trying to have a conversation in a busy street, or even just a noisy office. Voice authentication systems must be able to sift through these distracting sounds to identify the speaker's voice. Fortunately, techniques like noise suppression and sound source localization are being developed to improve voice recognition performance in less than ideal environments.
These systems are increasingly capable of processing voice inputs in real time, vital for applications like live transcription services or voice assistants. This allows for a smoother, more immediate user experience, where the technology feels responsive and natural.
Despite these advancements, the inherent complexities of human speech present a constant challenge. Speech patterns can be irregular, with pauses, filler words, and individual differences. Sophisticated algorithms are needed to interpret these variations accurately.
Moreover, there's the exciting possibility that voice analysis could be used to assess health. Research suggests that voice patterns could indicate certain vocal cord disorders or even emotional distress. If voice analysis were integrated into healthcare, it could potentially provide valuable insights into our well-being.
As voice authentication technologies become more prevalent, ethical and legal questions surrounding privacy and data security arise. Just like with fingerprints, how we collect, store, and use voice data requires careful consideration. Balancing the benefits of these powerful tools with individual privacy concerns is a crucial aspect of their responsible development and deployment.
The Science Behind Voice Recognition How AI Identifies Unique Vocal Signatures - Voice Cloning Applications in Audio Book Production
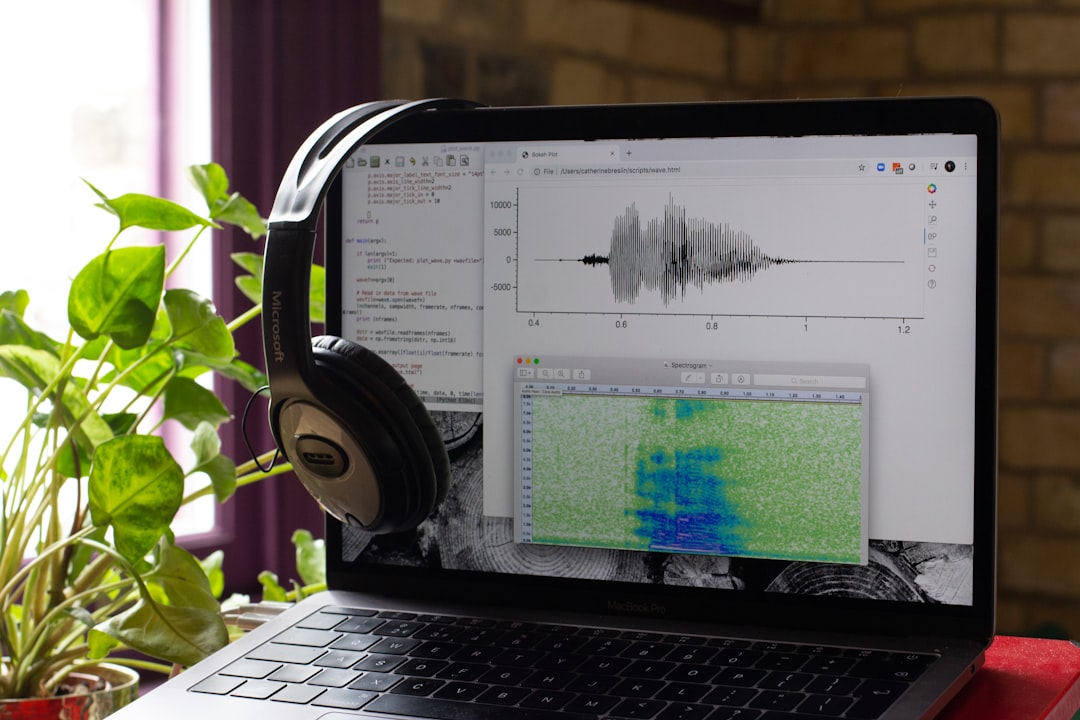
Voice cloning in audiobook production is changing how stories are heard and told. Artificial intelligence allows creators to make realistic voice copies that mimic the natural flow and nuances of human voices. This is a cost-effective way to make audiobooks, speeding up production and letting more stories be shared. However, with synthetic voices becoming more commonplace, there are ethical issues about authenticity and the potential for misusing voice cloning. The lines between real voices and artificial ones are blurring, so we have to think carefully about these challenges while embracing the creative potential of voice cloning.
Voice cloning has opened a new world of possibilities for audiobook production, pushing the boundaries of what's possible in storytelling. It's fascinating how this technology allows us to create a synthetic voice that mirrors a real person's speech patterns, even capturing the subtle emotional nuances that make a voice unique. It's not just about recreating the voice; it's about capturing its essence. Imagine an audiobook where each character has a distinct voice, perfectly matching their personality and background, even if all of them are voiced by the same person. That's the magic of voice cloning.
The beauty of it is that these technologies can be fine-tuned to match a particular narrator's style. This means audiobooks can be produced with a consistent voice, regardless of when each chapter is recorded, creating a truly immersive experience for the listener. And the speed of it all is astounding! Audiobooks that might have taken weeks or months to produce can now be completed in a matter of days. This efficiency is not just about speed, it's about giving creators more flexibility and opening up opportunities for them to bring their stories to life.
But how does it all work? Many voice cloning systems rely on extensive phonetic databases that cover a wide range of spoken language, including accents and dialects. This means even characters with unique voices can sound authentic, adding an extra layer of realism to audiobooks. And the real magic happens when real-time adaptation algorithms step in. They adjust the synthesized voice as the content is read, fine-tuning pitch and tempo based on the pacing of the narration. It's like having a real narrator, but without the limitations of time and location.
The link between voice cloning and automatic speech recognition (ASR) is fascinating. They share a lot of underlying technology, particularly neural networks. These "brain-like" structures learn how voices are produced, allowing the technology to both accurately recognize and replicate them. This synergy is what makes voice cloning increasingly powerful, paving the way for more sophisticated and natural-sounding voices.
There's even more to explore, like creating multiple voice personas from a single model. Imagine an audiobook where one voice narrates different characters, each with distinct vocal traits, adding depth and complexity to the storytelling. And to make the audiobook sound truly professional, post-processing tools are integrated into the process, modifying the audio output to match different environments, giving it that studio-quality feel.
And let's not forget about the future! Voice cloning can handle multiple languages, which opens up the possibility of audiobooks being translated and enjoyed by a wider audience. The same synthetic voice can read texts in different languages while still maintaining its unique character, bringing new voices and stories to life across borders.
But as with any powerful technology, ethical concerns need to be addressed. We must consider issues of consent and misuse, especially as voice cloning becomes more accessible. Legal frameworks are being developed to protect vocal identities and prevent unauthorized replication. It's a complex challenge, but one that needs to be addressed as voice cloning continues to evolve.
The Science Behind Voice Recognition How AI Identifies Unique Vocal Signatures - Challenges in Handling Accents and Dialects
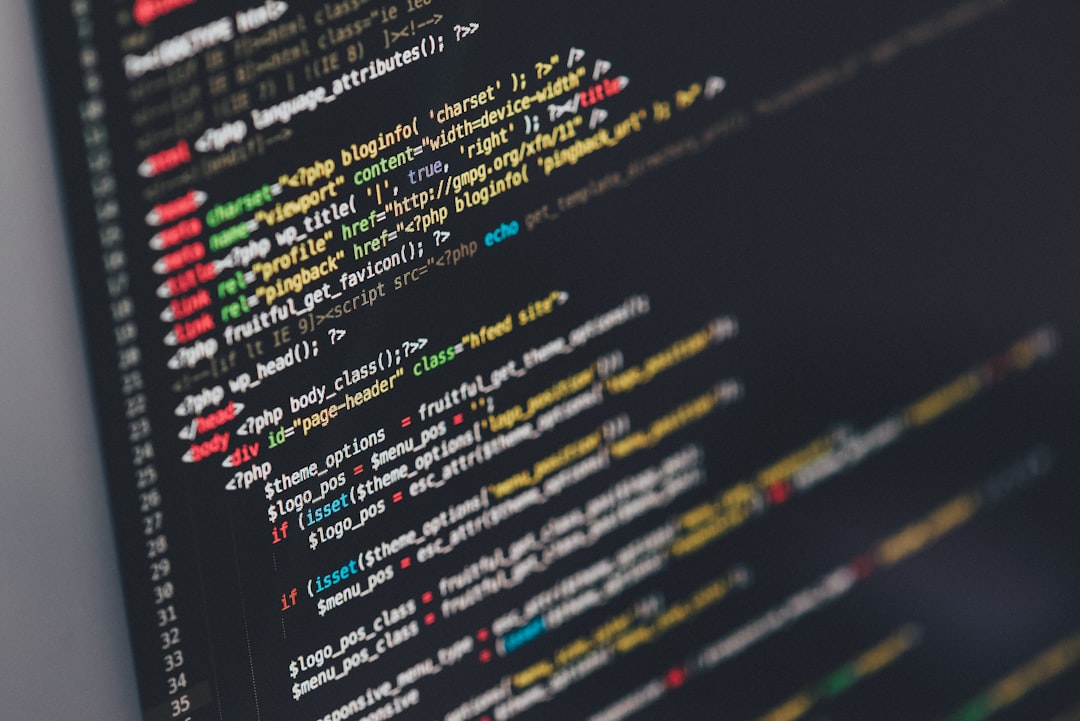
Handling accents and dialects remains a significant challenge for voice recognition systems. While AI advancements have improved the ability to recognize diverse speech patterns, inconsistencies in performance remain, particularly for dialects associated with specific ethnicities. This disparity raises concerns about bias in speech recognition technology, highlighting the need for more inclusive solutions that account for the full range of human speech. Efforts to improve the accuracy of voice recognition across diverse accents are crucial for applications such as audiobooks and podcasts, where capturing the nuances of different voices is essential for a truly engaging experience. The goal of building voice recognition systems that effectively and accurately process the rich tapestry of human speech remains a key challenge for researchers.
Accents and dialects pose significant challenges to voice recognition systems. While these systems are getting better at understanding the subtle variations in pronunciation, they still have a long way to go. Imagine trying to distinguish between the "a" in "cat" as it's pronounced in different parts of the country - the formant frequencies that define that sound can vary quite a bit, even for the same phoneme. And let's not forget about noise! Background sounds can muddle the accuracy of voice recognition, especially when the speaker already has a heavy accent. This issue is only exacerbated by the fact that dialects are constantly evolving, meaning systems need to be able to adapt to these changes in real-time.
It's also important to understand the context of a conversation. We're not just dealing with the sounds themselves, but how they fit into a larger picture, especially when we're talking about podcasts or live broadcasts where the conversation is dynamic. Accents can even carry emotional connotations that vary between cultures, meaning we need systems that not only identify who is speaking, but also can figure out how they're feeling.
However, a lot of voice recognition technology is built on datasets that don't represent enough diverse dialects, which creates bias in the systems. To make things even more difficult, we need to meticulously transcribe phonetics of different dialects to train these AI models, and this process takes a lot of time and linguistic expertise. And even with the most advanced technology, we have to consider the fact that how we perceive accents can be influenced by our own biases, something that needs to be factored into the development of these systems to ensure fairness and inclusivity.
Get amazing AI audio voiceovers made for long-form content such as podcasts, presentations and social media. (Get started for free)
More Posts from clonemyvoice.io: