Exploring Dynamic Assessment A Holistic Approach to Speech-Language Intervention Beyond Subtest Results
Exploring Dynamic Assessment A Holistic Approach to Speech-Language Intervention Beyond Subtest Results - Dynamic Assessment's Role in Voice Cloning Technology
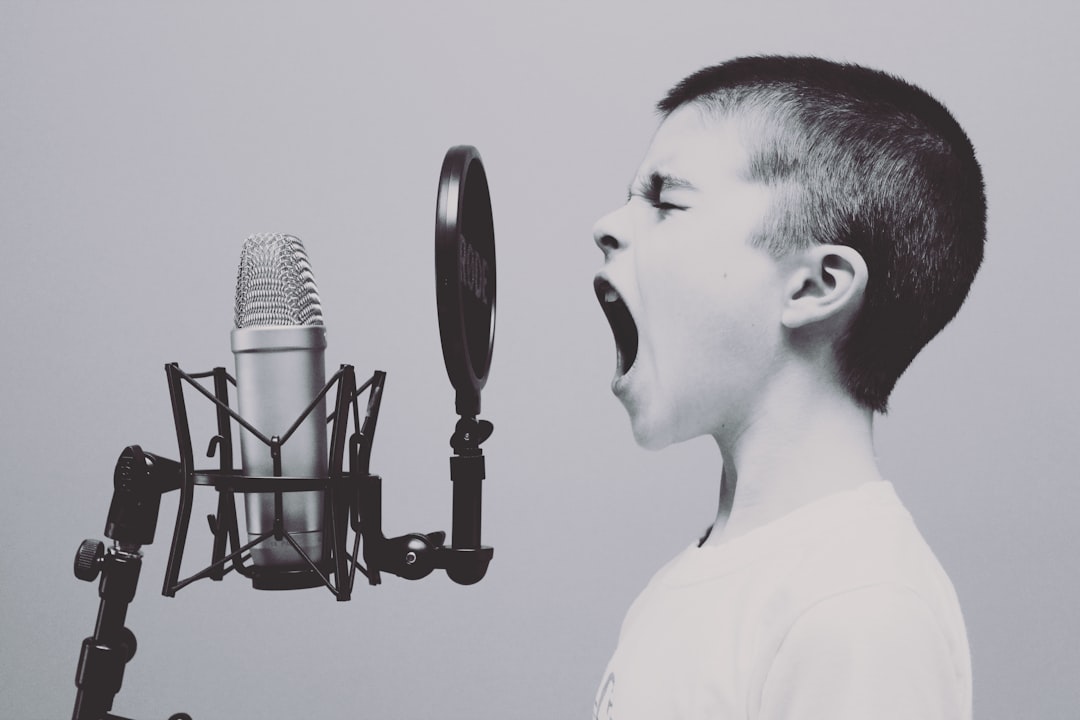
Dynamic assessment (DA) offers a compelling approach within voice cloning, especially when considering the need for diverse and nuanced voice models. Instead of simply measuring a static snapshot of vocal abilities, DA focuses on how individuals learn and adapt their sound production. This shift in perspective can lead to more authentic and natural-sounding cloned voices, which is crucial for applications like audiobook narration or podcast production. The interactive nature of DA provides a unique opportunity to capture the intricate details of human voice, including subtle intonations and speech patterns, ultimately enhancing the precision and effectiveness of voice cloning algorithms.
However, despite its potential to improve voice cloning, DA is not yet widely integrated into speech-language practices related to this technology. This presents a valuable opportunity for further exploration and development within the field. By leveraging DA's principles, researchers and developers may refine voice cloning methods to be more responsive to individual vocal characteristics and achieve a higher degree of authenticity and naturalness in the cloned voice.
Dynamic assessment offers a valuable lens for understanding an individual's sound production capabilities, going beyond a simple snapshot of their voice. This approach, focusing on the process of learning and adapting, can reveal intricate patterns in speech and sound that are crucial for refining voice cloning technologies. Instead of relying on static, predetermined prompts, dynamic assessment allows for real-time interaction and adjustments, mirroring the fluid and adaptable nature of human speech. By engaging in a collaborative process with the speaker, dynamic assessment captures a richer dataset, capturing subtleties in tone, pitch, and emotional inflection that traditional assessments often miss.
This interactive element provides valuable insights for voice cloning, showing how an individual modulates their voice in different contexts, which is vital for generating realistic and emotionally compelling synthetic voices. Through dynamic assessment, researchers can observe a speaker's learning journey and construct personalized voice profiles that encompass not only their unique vocal characteristics but also their developing sound production methods. Consequently, this approach has the potential to enhance applications like audiobook production by capturing the reader's distinctive style and emotional nuances for a more immersive listening experience.
Moreover, dynamic assessment sheds light on the intricate interplay between language and sound production, aiding voice cloning tools in simulating natural conversation patterns beyond simple phoneme replication. By pinpointing areas where sound production needs improvement, researchers can optimize machine learning models for more precise voice synthesis. The benefits extend beyond just improving synthetic voice quality. By uncovering potential needs of those with speech-language challenges, this approach fosters the development of more inclusive voice technologies. Finally, the insights gleaned from dynamic assessment can guide the development of adaptable voice cloning systems. These systems could adjust based on user preferences or emotional cues, leading to a more engaging and satisfying user experience.
Exploring Dynamic Assessment A Holistic Approach to Speech-Language Intervention Beyond Subtest Results - Applying DA Principles to Podcast Production Workflows
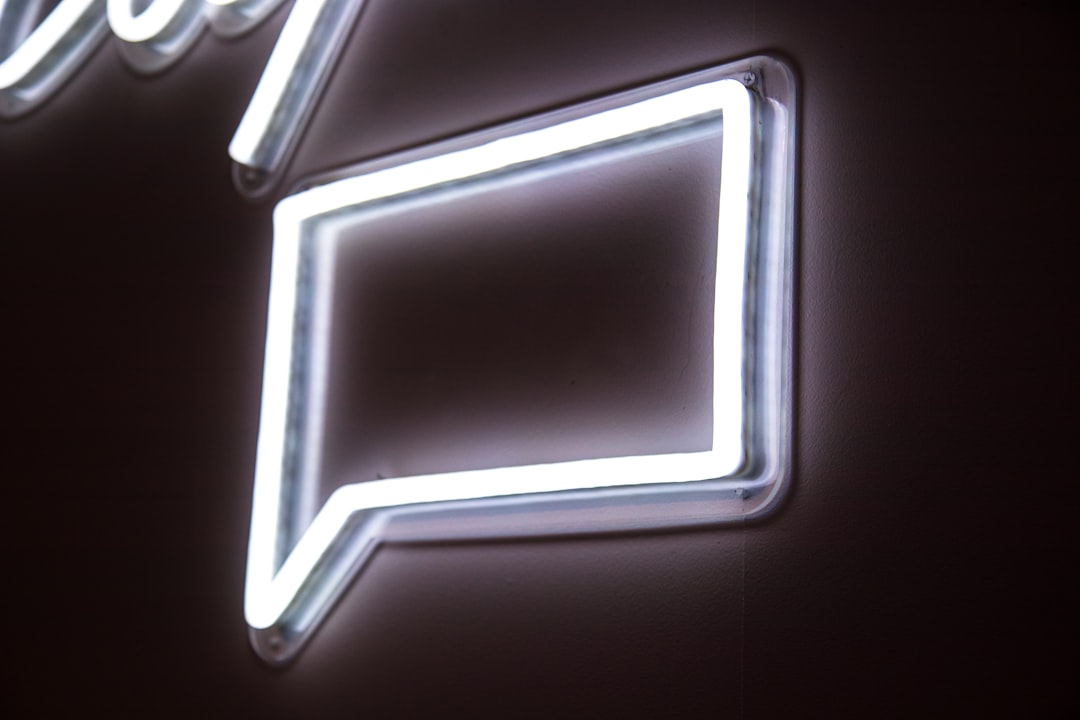
Applying the principles of dynamic assessment (DA) to podcast production workflows offers a path towards creating richer and more authentic audio experiences. Instead of solely focusing on the final product, DA emphasizes the interactive process of sound creation. This shift allows podcasters to gain a deeper understanding of how individuals produce sounds, adapt their voices, and express emotions.
By focusing on the learning process, DA can reveal subtle vocal patterns and nuances that traditional methods often miss. This is particularly valuable when trying to capture a speaker's unique style, or recreate a natural tone and conversational flow in audio book productions. Podcasters can use the interactive nature of DA to provide real-time feedback and guide adjustments, resulting in a more collaborative and effective creative process.
This approach could also reveal potential vocal limitations or strengths, particularly useful when dealing with voice cloning, potentially improving the authenticity of the resulting synthetic voice. This process can lead to a deeper understanding of the speaker's voice, leading to improved sound quality, authenticity, and greater connection between the content and the audience. Ultimately, integrating DA into podcast production workflows could contribute to a more humanized soundscape and potentially enhance the impact and resonance of the content. However, the applicability of DA in audio production is still an area ripe for further exploration.
Dynamic assessment (DA) offers a promising avenue for enhancing podcast production, voice cloning, and audiobook creation, by focusing on the learning process rather than just a static measurement of vocal skills. DA's interactive nature emphasizes the learner's ability to adjust and adapt their vocal production, aligning well with the need for natural-sounding synthetic voices. Research shows a strong link between cognitive load and voice modulation, suggesting that audio environments can be tailored to promote clear communication and more engaging audio.
Listeners demonstrate remarkable skill in discerning emotion from voice alone, highlighting the importance of capturing emotional nuances in voice cloning. This ability can enhance the realism of synthesized voices, especially crucial for tasks like audiobook narration where emotional delivery can greatly improve listener experience. DA also illuminates the inherent variability in human speech patterns based on social context and emotional state. Understanding this can lead to podcast production techniques that ensure cloned voices adapt naturally to various narrative styles, resonating with diverse audiences.
The human brain’s neuroplasticity underscores the potential for voice cloning to adapt and evolve in real time. DA, applied to voice cloning technology, could facilitate the development of synthetic voices that learn from interaction and modify their production accordingly. Traditional voice assessments often overlook subtle acoustic features like breathiness or nasality, while DA can capture these intricacies. This detail could elevate cloned voices' realism and expand their suitability for sensitive applications such as therapeutic tools.
Engaging speakers in real-time feedback during DA could significantly enhance the quality of data for voice cloning. The adjustments and reactions captured during these sessions provide valuable information about nuanced sound adjustments. This adds a layer of complexity to the resulting voice profile and moves beyond a basic snapshot of vocal abilities. Further, it's vital to acknowledge vocal fatigue, an often overlooked issue in podcast production. DA can aid in monitoring vocal fatigue and informing session structure for high-quality audio while prioritizing vocal health.
The intelligibility of speech hinges not only on articulation but also on the emotional tone delivered. Podcast production employing DA can ensure that synthetic voices are both intelligible and emotionally expressive, thereby bolstering listener comprehension and engagement. Individuals adjust their speech patterns in response to various dialects and accents. DA reveals these adaptations, proving crucial for developing voice cloning algorithms that generate synthetic voices capable of reflecting regional variations, thereby resonating with diverse listener groups.
Podcast productions often include multiple speakers, creating potential issues with crosstalk. DA can aid in understanding how speakers naturally adapt their vocal production to avoid overlap. This knowledge can, in turn, inform engineering solutions for cleaner audio recording in these environments. While DA has not yet been widely embraced in voice cloning practices, the potential benefits to refining voice cloning methods and tailoring them to individual vocal characteristics appear promising. This exploration should be prioritized to improve voice cloning algorithms, achieve more authentic-sounding voices, and potentially expand the accessibility of voice technologies for wider use.
Exploring Dynamic Assessment A Holistic Approach to Speech-Language Intervention Beyond Subtest Results - Enhancing Audiobook Narration through Interactive Assessment
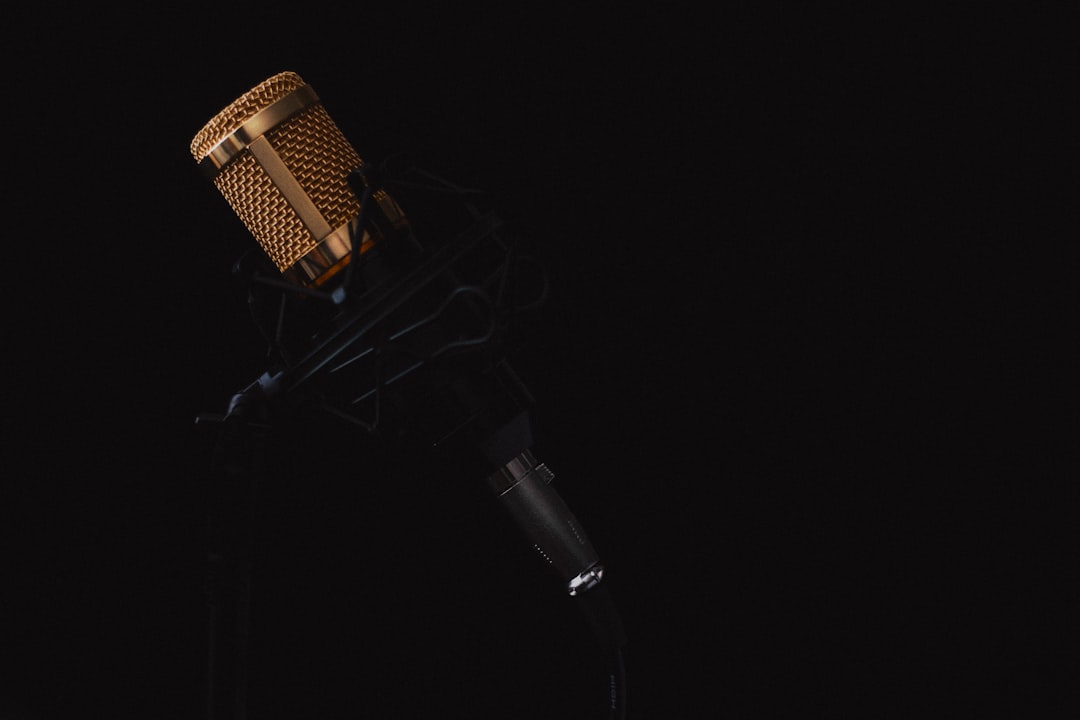
Enhancing audiobook narration through interactive assessment shifts the focus from simply evaluating a narrator's vocal abilities to understanding how they learn and adapt their sound production in real-time. This approach emphasizes the dynamic interplay between the narrator and the material, allowing for a more nuanced understanding of how they convey emotion and meaning through their voice. By incorporating elements of dynamic assessment, audiobook narration can become more engaging and authentic. Narrators can gain a deeper awareness of their own vocal nuances and learn to fine-tune their delivery to match the emotional landscape of the story, resulting in a richer and more immersive experience for listeners. Furthermore, this interactive assessment process helps researchers and developers of voice cloning technologies better grasp the subtleties of human voice production, which is essential for creating more natural and expressive synthetic voices. This holistic approach not only refines the technical aspects of audiobook narration but also creates a deeper connection between the narrator and the listener, fostering a more profound and enriching audio experience. While promising, this field requires further exploration to fully integrate the benefits of interactive assessment into the production of audiobooks and voice cloning technology.
In the realm of audio production, especially within the context of podcasting and audiobook narration, dynamic assessment (DA) presents an intriguing approach to refining voice cloning technologies. The concept of real-time feedback, central to DA, allows for a more nuanced understanding of how a speaker adapts their vocal delivery based on immediate reactions and guidance. This could be incredibly beneficial for podcast production, potentially leading to enhanced vocal clarity and a richer emotional expression, ultimately resulting in a more immersive listening experience.
Research suggests a compelling link between the subtle emotional nuances embedded in speech and a listener's ability to perceive them. This highlights the importance of voice cloning technologies being able to accurately mimic these subtleties, particularly in audiobook narration where emotional delivery can deeply impact the listener's experience. DA's interactive nature seems well-suited to capture these emotional nuances, potentially moving beyond the limitations of traditional assessments.
Additionally, a growing body of research indicates a clear connection between cognitive load and how individuals modulate their voices. This implies that carefully adjusting audio environments, such as pacing and intonation, can potentially optimize a speaker's output and enhance listener comprehension. Integrating this understanding into podcast workflows could lead to a more effective and accessible audio experience.
Furthermore, DA provides a framework for monitoring vocal fatigue during recording sessions. This could be extremely useful for podcasters who aim to produce high-quality audio while preserving their vocal health. By incorporating DA principles into production, podcasters can build in necessary breaks and potentially manage vocal strain more effectively.
DA also offers a window into the inherent variability of human speech patterns across different social contexts and emotional states. By understanding these variations, voice cloning technologies could be developed to adapt to diverse narrative styles and resonate with wider audiences. This capability is especially pertinent for developing voice cloning systems that can replicate regional dialects and accents, potentially opening up new markets for audio content.
Traditional vocal assessments frequently overlook subtle acoustic characteristics such as breathiness or nasality. DA, in contrast, appears to be able to capture these intricacies, potentially enhancing the realism of cloned voices and enabling them to deliver a greater range of emotional impact.
The brain's natural capacity for adaptation and learning, known as neuroplasticity, suggests that interactive voice cloning systems could evolve and learn over time based on user interactions. This could lead to the development of systems that are capable of refining their vocal outputs based on feedback, resulting in a more personalized and engaging experience for the listener.
Understanding how multiple speakers adjust their vocal productions to avoid crosstalk during conversations could lead to improved audio engineering practices for multi-speaker podcast formats. The insights from DA could be integrated into the recording process, ultimately resulting in clearer and more polished audio.
Dynamic assessment can lead to the development of personalized voice profiles that incorporate not just a speaker's inherent vocal characteristics but also their unique learning journey. These enriched profiles offer a valuable dataset for training voice cloning models, potentially resulting in more authentic and natural-sounding synthetic voices across a range of applications.
While DA remains a relatively novel approach in voice cloning applications, the potential benefits in refining these technologies appear substantial. It holds the potential to lead to a new generation of more refined voice cloning methods tailored to individual characteristics, potentially increasing the accessibility and adoption of these technologies in diverse settings. This line of research warrants further exploration to optimize the precision and versatility of voice cloning algorithms and expand the capabilities of voice technologies.
Exploring Dynamic Assessment A Holistic Approach to Speech-Language Intervention Beyond Subtest Results - DA's Impact on Accent Modification in Voice Acting
Dynamic assessment (DA) is increasingly relevant for accent modification within voice acting. It emphasizes the learning process rather than simply measuring current abilities, enabling voice actors to explore their sound production in a more interactive and adaptive way. DA allows actors to refine their accents while maintaining authenticity and achieving desired vocal changes. This focus on the process of change is particularly valuable when aiming for a natural and nuanced delivery, especially for voiceover work in audiobooks or podcasts, where emotional conveyance plays a crucial role. Through DA's emphasis on real-time feedback, voice actors can become more aware of the subtle vocal adjustments that impact how their voices are perceived by audiences. This can lead to more tailored strategies that allow voice actors to connect with diverse listener groups, ultimately enriching the overall listening experience. While conversations regarding accent modification continue to evolve, DA offers a path forward that is more respectful of cultural and linguistic diversity, potentially transforming how accent modification is understood and implemented within voice acting.
DA's principles, particularly its emphasis on real-time learning and adaptation, could significantly impact accent modification within voice acting. By focusing on how individuals adjust and modify their sound production, DA provides a pathway to capture nuanced changes in speech patterns that might otherwise be missed by static assessments. This dynamic approach is particularly relevant for voice cloning, where replicating a speaker's natural voice with subtle variations like accents is crucial for creating realistic and emotionally rich synthetic voices.
There's a growing body of research suggesting a close connection between cognitive load and how individuals modulate their voices. Understanding this link could be essential for voice actors aiming to refine their accent work. When actors are under cognitive strain, their vocal production can change, impacting the overall quality of the accent. This knowledge could help voice actors tailor their training and practice to reduce cognitive load and enhance control over vocal modulations for specific accent modifications.
The ability of listeners to perceive emotional nuances from voice alone underscores the importance of DA's application in accent modification. The subtleties of emotional expression can significantly influence the perception of an accent. If a voice actor is trying to convincingly portray a character with a certain emotional state while maintaining an altered accent, DA's interactive approach can be crucial in capturing those nuances and tailoring the training to achieve the desired result.
Furthermore, the brain's remarkable ability to adapt and rewire itself, known as neuroplasticity, provides a promising avenue for accent modification. Using DA's principles, accent training could be structured to promote these adaptive processes within the voice actor's brain, resulting in more long-lasting and natural-sounding modifications. DA could allow trainers to identify areas where the voice actor's neural pathways need to be reshaped for effective accent modification.
DA methods have the potential to pinpoint subtle acoustic features, such as breathiness or nasality, often missed in traditional assessments. These subtle features can significantly impact the perception of an accent, and being able to precisely control and manipulate them is essential for voice actors looking to refine their abilities in accent modification. This finer-grained analysis can guide training to create a more nuanced and accurate portrayal of the target accent.
People naturally adapt their speech patterns when interacting with those who speak with different dialects and accents. Understanding how these adaptations occur can provide valuable insights for voice actors who are working on accent modification. Voice cloning technologies could incorporate this understanding, leading to more realistic synthetic voices capable of replicating a range of accents and dialects.
When multiple voice actors collaborate, issues like crosstalk can arise. DA could help illuminate the ways in which individuals naturally adjust their speech to avoid this problem. This knowledge can inform better microphone placement and recording techniques in voice-over studios to optimize the recording process and create clearer, more professional audio recordings.
Voice actors can experience fatigue during long recording sessions, particularly when dealing with accent work. DA provides a means to monitor this fatigue and incorporate necessary breaks into the training or recording schedule. By integrating insights from DA into the practice of voice acting, actors can ensure vocal health and maintain consistent high-quality output.
In the realm of microphone techniques, DA's emphasis on interactive assessment provides the chance to gain a deeper understanding of how sound is produced in relation to microphone positioning. Understanding the subtle adjustments made during dynamic assessment could potentially refine microphone placement and technique for optimized voice recordings during accent work. This could result in superior audio clarity and more nuanced accent reproduction.
Lastly, applying DA principles in accent training and voice cloning can lead to the development of more individualized and comprehensive vocal profiles. These detailed profiles would go beyond a mere snapshot of an actor's vocal abilities and include data about the actor's unique learning journey. This richer data set would then serve as a foundation for creating more precise and effective training methods, leading to a higher level of accuracy and authenticity in accent work. This approach has the potential to revolutionize the way voice actors learn and refine accents, potentially leading to a new level of realism and finesse in audio productions.
Exploring Dynamic Assessment A Holistic Approach to Speech-Language Intervention Beyond Subtest Results - Integrating Dynamic Assessment in AI-Driven Speech Synthesis
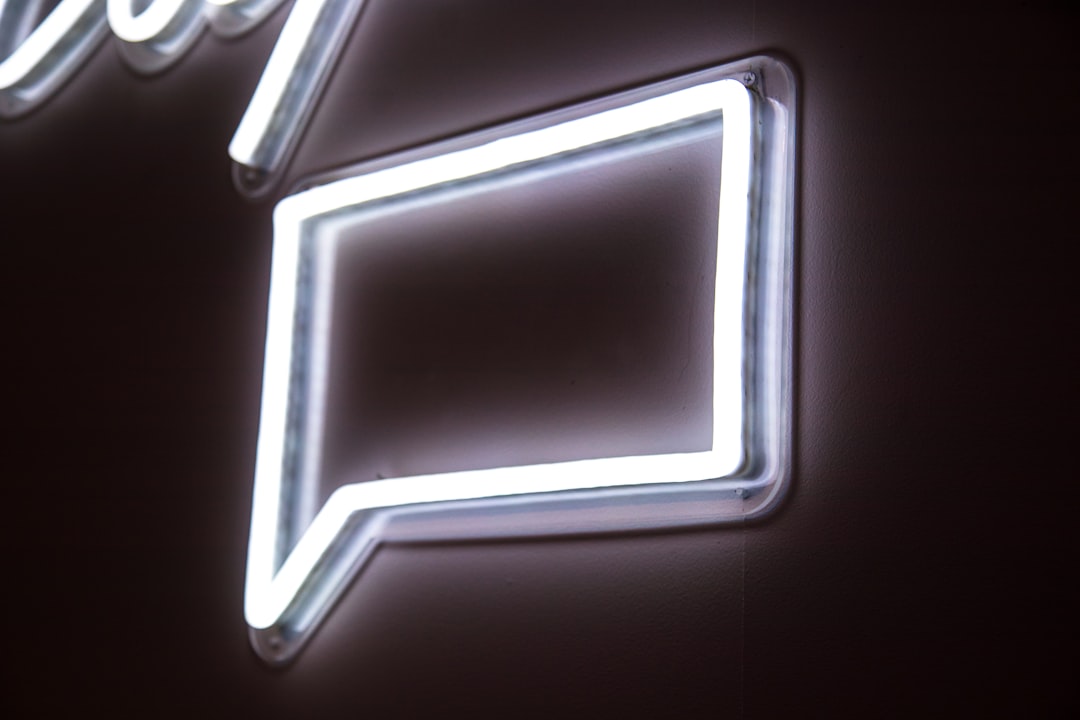
Integrating dynamic assessment (DA) into AI-powered speech synthesis offers a promising path towards creating more lifelike and effective synthetic voices. This approach emphasizes understanding how individuals produce sounds, focusing on the subtleties of real-time vocal changes and responses. Through interactive feedback, DA captures nuances in tone, emotion, and subtle vocal patterns that might otherwise be missed in static assessments, resulting in synthetic voices that sound more authentic and expressive. This is especially valuable in applications like audiobooks or podcast creation where emotional delivery is crucial. Additionally, the potential of DA to create individualized voice profiles that incorporate a speaker's unique learning process could significantly enhance voice cloning technology. However, fully realizing the potential of DA in AI-driven speech synthesis requires further investigation and refinement of existing techniques within the audio production realm.
Integrating dynamic assessment (DA) into AI-driven speech synthesis, specifically within the context of voice cloning for applications like audiobook production or podcasting, offers a compelling avenue for enhancing the naturalness and expressiveness of synthetic voices. DA's interactive nature, focusing on how individuals learn and adapt their sound production rather than simply measuring static vocal abilities, is particularly beneficial. It provides a way to capture subtle vocal nuances that traditional assessments often miss, leading to a more nuanced understanding of how individuals modulate their voices in response to feedback and changing contexts.
For instance, the real-time feedback loops inherent in DA allow for interactive learning cycles where speakers receive immediate auditory cues regarding their vocal production. This interactive process is crucial for musicians, podcasters, and audiobook narrators looking to refine their vocal techniques and achieve greater control over their sound production. This approach can lead to enhanced vocal clarity, expression, and an increase in the emotional range of synthetic voices, as subtle intonations and variations can be captured and replicated more accurately.
Further, the concept of neuroplasticity, the brain's ability to change and adapt, becomes particularly relevant when considering how DA can lead to lasting vocal changes. By encouraging adaptation through structured practice, DA can help voice actors permanently integrate desired vocal changes into their repertoire, whether it be accent modification or specific vocal styles.
DA also reveals subtle acoustic features like breathiness or nasality, often missed by traditional assessments. These seemingly small details can significantly enhance the realism and human-like quality of cloned voices. Moreover, DA allows for real-time monitoring of vocal fatigue, enabling breaks and management strategies to be incorporated into recording sessions, which is crucial for maintaining vocal health and consistency in lengthy audio projects like podcasts or audiobooks.
Recognizing that people adjust their speech when interacting with those who speak differently, DA can aid in creating more adaptable voice cloning models. By understanding how individuals modify their speech to accommodate dialects and accents, AI algorithms can be refined to mimic these variations with more accuracy, enhancing the authenticity and global appeal of audio content.
DA also provides insights into how speakers naturally adapt their vocal production to avoid crosstalk in multi-speaker environments. This understanding can guide improved audio recording setups and techniques, improving overall sound quality and ensuring better communication in collaborative audio projects.
The interplay between cognitive load and voice modulation is another area where DA provides valuable insights. By analyzing how cognitive strain affects vocal production, researchers can better understand how to optimize speakers' outputs when facing stressful environments, resulting in clearer and more focused audio.
Finally, DA provides a framework for developing personalized voice profiles for each individual. These profiles go beyond static measurements and encompass the individual's unique learning journey regarding their sound production. These comprehensive profiles provide a richer dataset for training voice cloning models, ultimately leading to more authentic and natural-sounding synthetic voices across a range of applications. While still in its early stages of implementation within AI-driven speech synthesis, DA appears to be a promising technique with the potential to revolutionize voice cloning and contribute to a new generation of more refined, expressive, and adaptable voice technologies.
Exploring Dynamic Assessment A Holistic Approach to Speech-Language Intervention Beyond Subtest Results - Using DA to Improve Vocal Range in Sound Production
Dynamic Assessment (DA) offers a unique way to enhance vocal range within sound production, moving beyond a simple snapshot of current abilities. This method emphasizes the learning process, providing individuals with immediate feedback as they explore their vocal capabilities. This interactive approach allows for real-time adjustments and refinements, leading to a more nuanced and authentic vocal performance. DA's ability to capture subtle variations in sound, including emotional expression and pitch control, makes it valuable for applications like audiobook production, podcasts, and the creation of cloned voices.
DA also reveals the impact of external influences on vocal output, including emotional state and mental focus. Understanding these factors can lead to more effective techniques in sound production. By integrating these insights into the workflow, we can potentially achieve not only higher-quality synthetic voices but also more engaging listening experiences. Despite being relatively new within speech-language practices, DA shows great potential for improving voice technologies, creating a compelling avenue for future research and development.
Dynamic assessment (DA) presents a promising approach to understanding and enhancing vocal range, particularly within the context of sound production for audio applications like voice cloning and audiobook creation. The core idea of DA is to focus on the process of learning and adaptation rather than just taking a static snapshot of a person's vocal abilities. This shift in perspective is crucial as it highlights how individuals can modify their vocal tone, pitch, and emotional delivery in real-time, which is fundamental for creating lifelike voices.
The concept of neuroplasticity suggests that vocal training methods, facilitated by DA, can create lasting changes in vocal patterns. This is significant because it implies that the application of DA principles can potentially impact both the development of human vocal range and the refinement of AI-generated voices over time. Furthermore, the benefits of real-time feedback during vocal training are well-documented. This immediate interaction can contribute to notable improvements in vocal clarity and emotional expression, both of which are vital for immersive storytelling in audio formats.
Interestingly, research indicates that the cognitive demands placed on a speaker can influence their vocal modulation. It's a reminder that managing cognitive stressors is essential for consistently delivering high-quality vocal performances within audio production, particularly in situations where the production environment is demanding.
DA also offers a unique advantage in capturing those subtle vocal aspects that traditional assessments often overlook. Features like breathiness or nasality, which can subtly alter how a voice is perceived, are easily identified with DA, enhancing the naturalness and emotional depth of synthesized voices, for instance, in voice cloning.
Beyond the individual, it's important to acknowledge that individuals naturally adapt their speech patterns when interacting with others who speak with different accents. DA exploits this natural adaptability, allowing voice cloning algorithms to replicate regional dialects with improved accuracy, which is vital for synthesizing voices that resonate with a wider audience.
Similarly, vocal fatigue is a common issue in lengthy recording sessions, and DA offers a way to monitor and manage vocal strain. DA allows for scheduled breaks and better management of vocal health during recording, thereby ensuring the maintenance of high-quality audio throughout the session, an issue especially critical for podcast and audiobook production.
The influence of social context and emotional state on human speech is another area where DA proves useful. Understanding how these factors affect vocal production is critical for training voice cloning systems to reflect more naturally the intimacy and expressiveness that characterize human conversations, thereby improving listener engagement.
DA also results in the creation of highly individualized vocal profiles that don't just capture innate vocal characteristics but also detail the speaker's unique learning journey. These rich datasets are valuable tools when training voice cloning models because they ensure the synthesized voices are more accurately personalized.
Finally, researchers are also learning how individuals naturally adjust their vocal production in order to avoid crosstalk during conversations, a common challenge when multiple individuals are recording audio. This knowledge gleaned from DA can lead to enhanced audio recording practices in multi-speaker audio projects, ultimately improving the clarity and focus of the recorded dialogue. While still a developing field, DA shows strong potential to refine vocal techniques and enhance voice cloning systems in numerous ways.
More Posts from clonemyvoice.io: