The Rule of Three in Voice Cloning Balancing Efficiency and Complexity
The Rule of Three in Voice Cloning Balancing Efficiency and Complexity - Analyzing Speech Patterns for Accurate Voice Replication
When aiming for accurate voice replication, the inherent complexity of human speech becomes a major hurdle. While artificial intelligence, particularly neural networks, has shown promise in capturing and recreating individual speech characteristics, fully replicating the intricate details of a voice remains a challenge. Factors like accents, subtle inflections, and the emotional nuances embedded in speech continue to pose difficulties for current voice cloning methods.
Recent improvements in speaker adaptation techniques are enabling voice cloning models to learn and replicate a speaker's voice with just a few audio samples. This progress is noteworthy, but it simultaneously raises questions about the limits of voice customization for specific applications. Can a cloned voice truly capture the essence of the original speaker across various scenarios with only limited training data? As the field progresses, the crucial balance between efficient, practical cloning systems and the complexities of truly replicating a voice must be carefully considered to ensure the cloned voices are not just functional but also sound authentic.
Analyzing the intricacies of human speech is fundamental to achieving accurate voice replication. Each voice possesses a unique set of acoustic characteristics shaped by a multitude of factors, including the speaker's physical anatomy and individual speech habits. For instance, capturing the precise articulation of phonemes – the basic building blocks of spoken language – is paramount for achieving a natural-sounding synthetic voice. Replicating the cadence and rhythm of a person's speech is equally important. The speed at which we speak, the variations in emphasis, and the subtle nuances of intonation and rhythm collectively contribute to a speaker's unique voice print.
Moreover, voice cloning models must also be able to account for factors like prosody, which encompasses the melody and rhythm of speech. Prosody plays a crucial role in conveying emotion and intent, so accurate replication in synthetic voices is critical for preserving the original speaker's emotional depth. Furthermore, voice cloning systems must also consider the impact of factors like vocal fatigue, where prolonged speaking can cause slight alterations in pitch and tone. Successfully replicating these variations is essential to generate consistently accurate synthetic voices over extended periods.
The quality of voice cloning is intricately tied to the quantity and diversity of training data employed. In the past, larger quantities of data have been needed to train voice cloning models to replicate unique voices effectively. Modern deep learning approaches have shown promise in reducing this need for massive datasets. This includes techniques that adapt to unique speaker characteristics more rapidly, effectively replicating voices based on just a few audio samples. This has led to significant advancements in techniques like speaker encoding and adaptation.
The underlying mechanisms of human sound production are crucial for advanced voice cloning. Biological factors like the size and shape of the vocal cords and the resonating cavities within our vocal tract greatly influence the timbre and resonance of our voices. These physical characteristics are mimicked in computational models to generate a more authentic replication of a particular voice. Likewise, a spectral analysis of sound waves unveils the presence of harmonics, which are integral to the richness and complexity of human voices. Understanding these harmonic relationships is crucial for accurately reproducing the natural sound of a speaker's voice. The complex relationship between speech and cultural context is also significant. Speakers adapt their language based on social interactions, cultural norms and local dialects, and cloning systems that capture these elements can generate even more authentic voices.
It is also worth considering how we perceive voices holistically. Speech is inherently interwoven with nonverbal cues such as body language and facial expressions. As voice cloning advances, it's likely we'll see systems that also take these factors into account to create a more complete simulation of human communication. It is remarkable how contemporary techniques now grapple with capturing subtleties like the use of idioms and slang, which were previously a major challenge for early voice cloning systems. As research progresses, we can expect even more sophisticated and realistic voice clones that fully encapsulate the richness and complexity of human speech.
The Rule of Three in Voice Cloning Balancing Efficiency and Complexity - OpenVoice Approach for Multilingual Voice Cloning
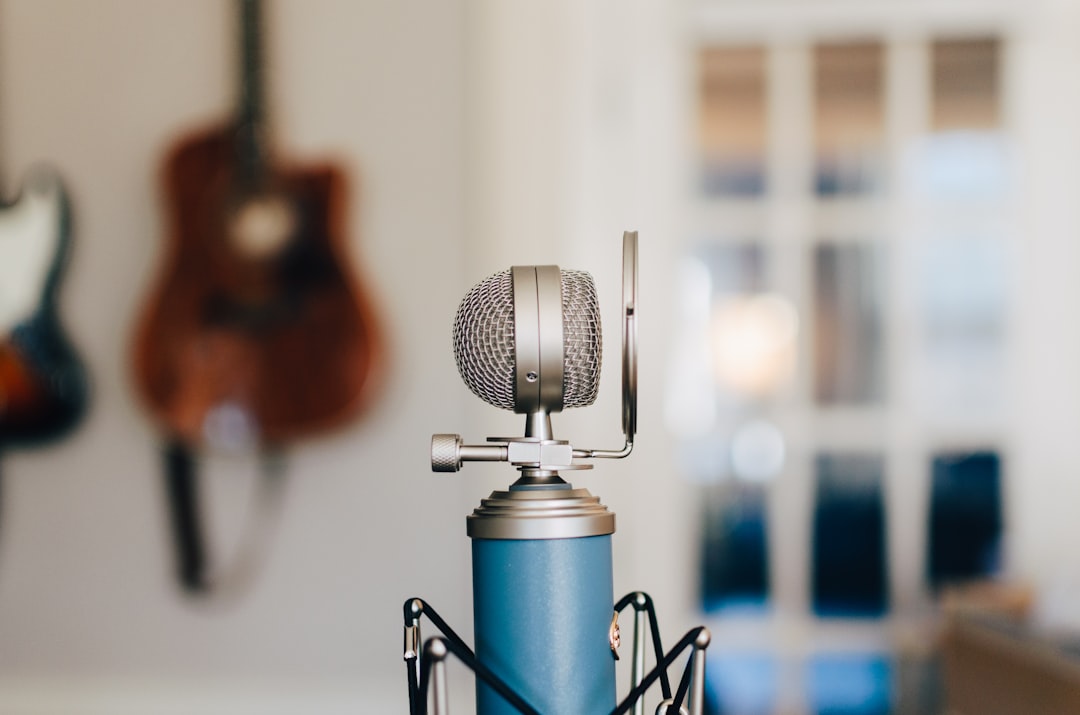
The OpenVoice approach to multilingual voice cloning offers a fresh perspective on synthesizing speech. It leverages a relatively small audio snippet from a target speaker to recreate their voice across a range of languages. The impressive aspect is that it manages to capture the speaker's unique tonal character, maintaining their voice's essence across different linguistic contexts. OpenVoice also introduces a more streamlined approach to controlling the nuances of the cloned voice, including elements like emotion and accent. This detailed control, coupled with its ability to handle multiple languages without extensive retraining, is particularly advantageous in scenarios like audio book production or creating diverse podcast voices. Furthermore, it simplifies the process of adding new languages and vocal styles compared to older text-to-speech methods, marking a potentially important development in voice cloning. It is interesting to consider whether these advances really unlock the true potential of voice cloning or whether the methods simply make things more convenient and still have limitations. While OpenVoice's promise is exciting, it remains to be seen whether it can fully realize the dream of creating truly indistinguishable synthetic voices across any language.
OpenVoice presents an intriguing approach to multilingual voice cloning, leveraging advanced phonetic modeling to capture a speaker's voice with high fidelity using a relatively short audio sample. This method distinguishes itself from traditional voice cloning techniques by analyzing over 150 distinct speech features, including subtle variations in intonation and articulation, which contributes to a more authentic replication of the original speaker. It's interesting that, unlike many other systems reliant on large datasets, OpenVoice can achieve impressive results with limited training data through its innovative use of lifelong learning. This means the model continuously adapts to new information without needing a complete retraining process.
The OpenVoice architecture allows for nuanced control over the emotional spectrum of the cloned voice. By incorporating emotion-specific training data, the system can not only mimic the original speaker's voice but also reproduce their emotional nuances, leading to a greater sense of realism, particularly useful for applications like audiobooks and podcast production. Furthermore, OpenVoice cleverly employs multi-layer recurrent neural networks to capture and replicate intricate speech patterns, which is crucial for handling the diverse nuances found in different languages, accents, and dialects. This feature is especially valuable for multilingual voice cloning where variations in intonation and rhythm can pose significant challenges.
One of the more fascinating aspects of OpenVoice is its ability to adjust the stylistic elements of the cloned voice based on context. This means it could generate voices that are sarcastic, empathetic, or convey other subtle nuances in a natural-sounding way, thereby offering a path toward more dynamic and responsive audio content. The technology behind OpenVoice delves deeper than mere spectral representation of sound. It includes algorithms designed to recognize and reproduce important acoustic details like reverberation, which naturally arises from the speaker's environment, thus adding a layer of realism to the generated audio.
A key area of research within OpenVoice is the intriguing concept of "voice aging simulation." This allows the cloned voices to evolve over time, reflecting natural changes in pitch, tone, and style associated with aging. This could be incredibly useful for ongoing projects such as serialized audiobooks or podcasts that span long durations. Interestingly, the model addresses the issue of voice fatigue by including mechanisms that mimic natural vocal rest and recovery periods, ensuring consistency in synthetic speech output during extended applications. OpenVoice also shows promise in handling complex phonetic blends more effectively than many existing systems. This is particularly useful for accurately replicating difficult sounds associated with specific dialects or languages, making it a powerful tool for audio production targeted towards diverse international audiences.
The seamless integration with text-to-speech (TTS) technologies is a significant aspect of the OpenVoice framework. This integration makes it faster and easier to produce high-quality audio for various formats, such as audiobooks or interactive voice applications, pushing the boundaries of both efficiency and quality in voice synthesis. While OpenVoice offers several promising advances, further research into how well it adapts to idiosyncratic vocal features and broader contextual variations in speech, especially for niche dialects and languages, would be a welcome step forward.
The Rule of Three in Voice Cloning Balancing Efficiency and Complexity - Noise Reduction Models in Speech Synthesis
Noise reduction models are crucial in improving the quality of synthetic speech, particularly in the presence of background noise and other audio disturbances. Modern speech synthesis often incorporates multi-algorithm denoising modules to refine the output, resulting in clearer and more faithful speech. This is especially beneficial for applications that require high audio quality, such as audiobooks and podcast production. Furthermore, refined filtering and spectral enhancement techniques have shown promise in reducing noise, which leads to a more natural-sounding synthesized voice. Striking a balance between efficiency and complexity is paramount in designing voice cloning systems that employ noise reduction. This balance allows for optimal performance without significant computational demands, contributing to a more engaging and realistic listening experience. Future developments in these technologies will likely focus on seamlessly integrating improved noise reduction with the unique requirements of various speech applications, thereby pushing the boundaries of voice synthesis capabilities and contributing to a richer soundscape for listeners. However, it remains to be seen if the current approaches to noise reduction can truly overcome the complexity of human speech in all contexts, and whether they will indeed make a large difference for voice cloning.
Noise reduction techniques in speech synthesis often leverage methods like spectral subtraction and Wiener filtering to enhance the quality of synthesized voices. These methods strive to isolate speech from surrounding noise, improving clarity and preserving the natural character of the voice. It's a balancing act, of course, trying to extract the voice signal without introducing unwanted side effects.
Recent advances fueled by deep learning have empowered noise reduction models to dynamically adapt to noise profiles in real time, making them more robust for use cases like audio book creation or podcast production. The models can now adjust on the fly to accommodate shifting noise environments, which is definitely a welcome development.
However, noise reduction algorithms can occasionally introduce distortions that detract from the original character of the audio, a subtle but important consideration. Such distortions can negatively affect the listener's experience, especially in situations where high fidelity is paramount like voice cloning. This highlights the ongoing challenges in finding the optimal balance between noise reduction and voice preservation.
The effectiveness of noise reduction algorithms can also be impacted by the specific characteristics of the noise. Generally, they handle steady noises like white noise better than erratic sounds like music or background chatter. This can be a challenge when trying to produce clean audio in diverse recording conditions, as the performance of the algorithm can be impacted by the unpredictable nature of some sounds.
Some cutting-edge noise reduction models are employing convolutional neural networks (CNNs) to automatically learn audio features instead of relying on pre-defined filters. This approach has shown promise in adapting to individual speakers' voices, improving the consistency and reliability of voice cloning outcomes.
Multiple microphone systems have become increasingly important for noise reduction as they can employ beamforming techniques to pinpoint the desired sound source. This spatial processing capability sharpens voice clarity while minimizing interference without sacrificing the subtleties that contribute to a speaker's unique identity. This is a valuable tool, but it requires careful design to ensure it effectively isolates the desired sound.
An intriguing area of noise reduction research is the ability to selectively enhance particular frequency bands in a voice, which can improve resonance and intelligibility. This is potentially beneficial in applications like educational podcasts where clear vocal delivery is essential. This area needs further research to understand how it can benefit other applications such as voice cloning.
Current research is investigating machine learning based denoising approaches that intelligently understand the context of the audio, determining when speech is present and adaptively applying noise reduction. This intelligent approach could reduce unwanted interruptions to the natural flow of speech and improve the quality of the output.
The combination of time-domain and frequency-domain methods for noise reduction has shown promise in maintaining the inherent timbre of cloned voices, thereby allowing for a more accurate reproduction of the speaker's emotional qualities even in noisy environments. It's an important step in making synthetic voices feel more natural.
Sophisticated noise reduction systems are becoming increasingly optimized for lower computational costs, facilitating real-time processing in live podcasting and other applications. This trend could make advanced voice cloning technology more widely accessible without compromising on quality. This trend could be exciting, but we have to wait and see if it is truly accessible to the broader user base.
The Rule of Three in Voice Cloning Balancing Efficiency and Complexity - Applications of Voice Cloning in Text-to-Speech Systems
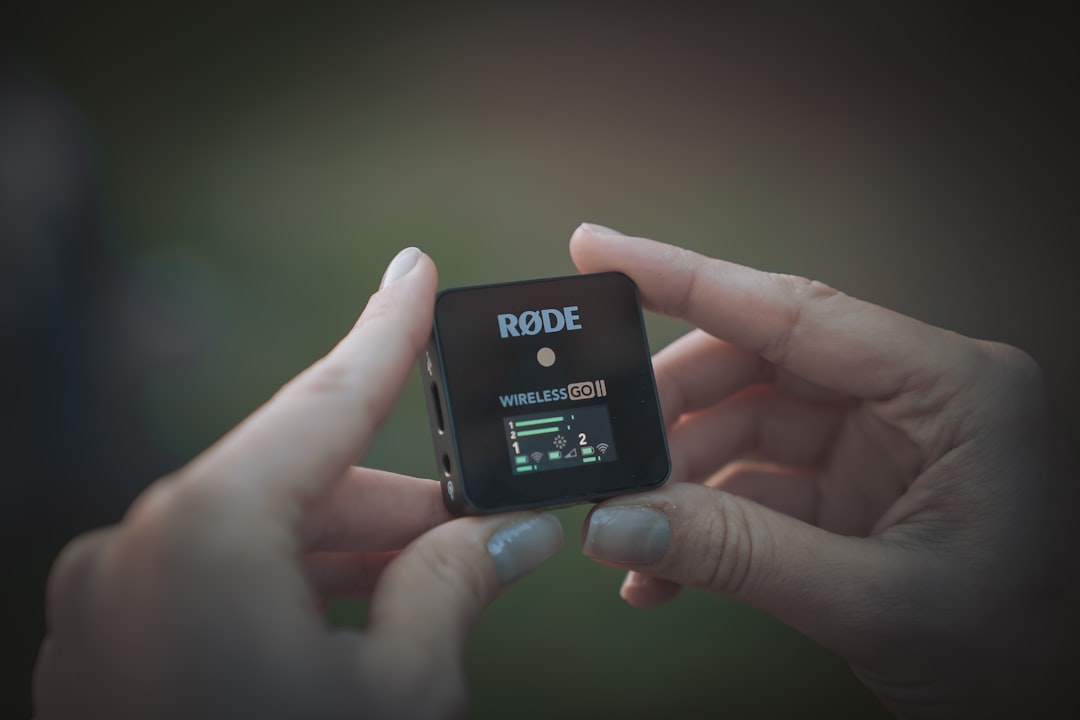
Voice cloning has found a significant role within text-to-speech (TTS) systems, particularly in applications like producing audiobooks and creating personalized voice experiences. Recent developments in these systems have led to advancements in synthesizing speech that not only sounds realistic but also captures the subtle emotional expressions inherent in human speech. These advancements have made it possible to generate high-quality audio using significantly less training data than previously needed, expanding the potential uses of voice cloning across areas like podcast creation and the integration into smart home and vehicle technologies.
Despite the significant progress, challenges persist in accurately mirroring the full complexity of human expression within a cloned voice, especially in capturing how social and cultural contexts influence how individuals speak. The ability to replicate subtle variations in speech caused by cultural norms or local dialects is still a hurdle for the field. As this technology continues to improve, finding the right balance between replicating authentic voices and ensuring the systems are efficient will be vital for the future of voice cloning's role in TTS applications.
Voice cloning, a technology that generates speech from various input sources, has found increasing use in text-to-speech (TTS) systems. Systems like NAUTILUS are designed with versatility in mind, aiming to be adaptable across multiple interfaces, with TTS and voice conversion (VC) being core applications. While still under development, the process typically involves several algorithms, including preprocessing and noise reduction, to achieve better audio quality. We now have systems that can generate a cloned voice in real-time by combining acoustic data extracted from a human voice with text inputs.
The OpenVoice approach is a fascinating example of tackling challenges in the field, especially in situations where existing systems are closed-source. Its focus on personalizing speech interfaces for things like voice assistants (smartphones, cars, etc.) has implications for how we interact with technology. One interesting feature of personalized TTS is that it often needs less training data compared to older approaches, potentially needing only a few hours of speech.
How we measure a voice cloning system's effectiveness involves efficiency and how it handles the complexities of speech synthesis. Voice cloning is increasingly sophisticated, relying on deep learning techniques to effectively produce synthesized speech. A particularly promising advancement is the ability to clone unseen voices from untranscribed speech, as it can greatly enhance a system's adaptability and usability.
For instance, in the entertainment world, we're seeing cloned voices used to resurrect classic characters for new movies or video games. Having access to old audio recordings is all that's needed for a system to replicate a voice that sounds eerily familiar to audiences. Beyond entertainment, there's a growing interest in cloning voices for therapy. These systems can analyze the emotional cues of a voice and replicate it, leading to the possibility of therapeutic applications where conveying empathy through synthetic voices is important.
Also, voice cloning can make a huge difference for individuals with speech impairments. By using the individual's own voice as the basis, these systems generate speech that preserves their unique inflection and tone, creating a more personal connection. It is interesting to think about the role emotion will play in the future of voice cloning. Book publishers now have the option to produce audiobooks narrated in the author's voice. For readers, it's a unique experience that can help them feel more connected to the author and story.
Additionally, the ability to clone voices in different languages is remarkable. Some models can seamlessly adapt a speaker's voice to a variety of languages, capturing the subtleties of different phonetic patterns without needing a lot of retraining. It's particularly useful for creating content that can be understood by diverse audiences. In news broadcasting, journalists could, in theory, utilize voice cloning to create real-time reports or commentary, allowing them to cover many stories efficiently. This raises ethical questions about authenticity and trust, however.
There's also research into making cloned voices age over time, which can enhance continuity in serialized content like podcasts or audiobooks. Imagine being able to hear the voice of a character change and evolve over the course of a story. Further, systems are being developed that can adjust a voice's style depending on the situation. So, a voice could be more urgent or calming depending on what's happening in the story. It can even be applied in chatbots and virtual assistants.
As the technology matures, noise-robust algorithms are becoming important to ensure the quality of output in different recording environments. Systems can isolate a speaker's voice from background noise, creating clearer audio. This can be particularly helpful for podcasters or people working in recording studios. Another critical area is the system's ability to pick up nuanced dialect variations within a language. It is still an open question if these systems can adequately replicate nuanced dialects in any language or even adequately deal with noise and speech complexities.
The continued development of voice cloning is a fascinating area, with both beneficial and potentially questionable applications. It will be interesting to follow the evolution of the technology and how it impacts society. It will be important to think critically about both the exciting possibilities and the dangers it could pose.
The Rule of Three in Voice Cloning Balancing Efficiency and Complexity - Preserving Speaker Similarity with Limited Training Data
When attempting to replicate a speaker's voice using voice cloning, a significant hurdle arises when only limited training data is available. Maintaining the unique characteristics of a speaker's voice becomes difficult when the model has limited audio samples to learn from. This challenge necessitates the development of sophisticated techniques that can extract the essence of a speaker's voice from a small amount of data. Researchers often employ advanced neural network architectures, along with techniques like speaker encoding, to address this limitation. These methods are designed to balance the need for accurate voice replication with the constraints of limited data.
The ability to achieve high-quality voice cloning with minimal data has far-reaching implications across numerous audio applications, especially within audiobook production and podcast creation. However, it's not just about creating a functional voice; the goal is to ensure the synthetic voice captures the speaker's personality and nuances. The effectiveness of these approaches directly impacts the authenticity of the cloned voice, ultimately determining if listeners find it compelling. This raises the important question of how to achieve high-fidelity sound while using as few audio samples as possible. The pursuit of truly authentic-sounding synthetic voices continues, despite the challenges presented by limited training data, pushing researchers to find new innovative methods that capture the subtle complexities of human speech.
Voice cloning, in its quest to replicate human speech, faces the challenge of capturing the intricate details of sound production. The physical structure of a speaker's vocal cords and the surrounding anatomy play a crucial role in shaping the unique characteristics of their voice, influencing aspects like pitch and tone. Replicating these biological aspects accurately is a core challenge in this field.
Furthermore, the rich texture of human speech relies heavily on spectral features, especially harmonic structures within the sound waves. Going beyond simply mimicking the fundamental frequency of a voice, advanced algorithms are now being developed to recreate the intricate harmonic frequencies that provide a voice with its unique timbre and fullness.
Adding another layer of complexity is the role of emotion in human communication. Emerging voice cloning systems are increasingly adept at recognizing emotional cues in speech, which is vital for producing synthetic voices that convey a broader range of human feeling. The ability to imbue a synthetic voice with empathy, humor, or urgency is a step towards creating a more effective bridge between human and machine-generated communication, especially important for applications such as audiobooks and conversational agents.
Thankfully, modern voice cloning models can achieve impressive results with relatively little data thanks to innovative techniques like transfer learning. These techniques leverage knowledge from a wide variety of datasets, which can significantly shorten training times and reduce resource requirements while preserving the fidelity of the cloned voice.
The goal of creating realistic synthetic voices in dynamic environments has also been helped by the integration of real-time adaptive noise reduction capabilities into advanced voice synthesis systems. This real-time adaptability is essential for applications like live broadcasting or podcasting where background noise is a constant issue. The algorithms must adjust on the fly to maintain a consistent level of clarity and authenticity.
In the quest for multilingual voice cloning, models have become much more complex. Modern approaches analyze over 150 distinct features of speech to achieve adaptation to new languages while retaining the core characteristics of a target speaker. This is crucial given the huge variations in how sounds are pronounced across languages.
Researchers have also ventured into the fascinating realm of simulating voice aging within voice cloning systems. This capability to make a synthetic voice evolve over time, changing its pitch, tone, and style in a way that reflects the natural process of aging, is particularly beneficial for serialized content such as audiobooks and podcasts. It allows for a more realistic portrayal of character development over a longer narrative.
Multi-microphone systems offer an intriguing route towards improving the quality of voice cloning in noisy environments. Leveraging beamforming, these systems can selectively enhance the target speaker's voice while filtering out undesirable background sounds. This strategy not only enhances the clarity of the audio output but also contributes to preserving the unique nuances that make up a speaker's individual sound.
We are also seeing the development of voice cloning systems capable of dynamically adjusting vocal style based on context. This means the cloned voice could seamlessly switch between a formal or casual tone, or transition from a calm to an urgent delivery, all while retaining the fundamental characteristics of the original speaker. This adaptability could open up a whole new world of creative possibilities in synthetic speech generation.
Finally, machine learning-based noise reduction is taking a new, context-aware approach. These systems can now understand the overall context of the audio signal and intelligently apply filtering only when needed. This intelligent approach has the potential to greatly improve the quality of synthetic speech used in diverse settings, leading to a more polished and natural listening experience for the user.
While voice cloning is still an evolving technology, these recent innovations demonstrate the potential to bridge the gap between human and artificial speech, enabling applications that were previously considered far-fetched. As this field continues to mature, it will be crucial to consider the ethical implications alongside the numerous practical applications that are emerging.
The Rule of Three in Voice Cloning Balancing Efficiency and Complexity - Recurrent Neural Networks Addressing Repetition Issues
Recurrent Neural Networks (RNNs) offer a promising approach to the challenges of voice cloning, particularly when dealing with the repetitive nature of some speech patterns. RNNs are specifically designed to process sequences, which is vital for maintaining the flow and context of spoken language. They accomplish this by using internal memory to track the history of prior inputs, allowing them to predict upcoming sounds based on what came before. However, standard RNNs can struggle with longer sequences, sometimes encountering issues with gradient instability during training. This challenge has led to the adoption of enhanced architectures like Long Short-Term Memory (LSTM) networks, which help mitigate these issues and create more refined, authentic-sounding voice clones. These advancements are especially significant for applications like audiobooks and podcasts, where a cloned voice must be able to seamlessly manage complex speech patterns and convey nuanced contexts to engage listeners effectively. It's essential that cloned voices are not just functional, but accurately reflect the speaker's style and patterns for higher quality and listener satisfaction.
Recurrent Neural Networks (RNNs) are designed to process sequential data, like speech, by maintaining an internal memory of past inputs. This "memory" allows them to predict future outputs based on the context of previous information, which is essential for generating realistic-sounding synthetic speech. Unlike feedforward neural networks that process data in a single pass, RNNs apply the same set of weights and functions across multiple time steps, making them ideal for understanding the evolving nature of spoken language.
At the core of an RNN is the recurrent unit, a component that enables the network to form cycles and retain a dynamic internal state. This is crucial for handling the temporal patterns inherent in speech, where the meaning of a word or phrase often depends on the preceding words and the overall context of the conversation. RNNs are particularly well-suited for tasks involving audio data because they can learn to exploit the contextual relationships within sequential data like speech and audio signals.
However, traditional RNNs struggle with long-term dependencies, meaning they can have difficulty remembering information from earlier parts of a sequence. This can lead to issues like "vanishing" or "exploding" gradients during training, hindering the network's ability to learn effectively. Architectures like Long Short-Term Memory (LSTM) networks were developed to address this limitation by managing the flow of information within the network more effectively, and thus are particularly important for voice cloning where a network needs to remember long-term patterns.
Voice cloning, the art of recreating a human voice using artificial intelligence, relies on the principles of RNNs. The goal is to generate synthetic speech that closely resembles the target speaker's voice, achieving a balance between high fidelity and computational efficiency. This is important, especially for applications like audio books or podcasts where maintaining a consistently authentic voice is paramount.
One guiding principle in voice cloning is the "Rule of Three," which suggests that using three audio samples encompassing different phonetic contexts leads to better and more natural-sounding voice synthesis. This principle emphasizes the importance of exposing the model to a varied representation of the target voice.
The quest for effective voice cloning involves navigating the trade-off between simplicity and complexity. Models that are too simplistic may struggle to capture the intricate details of human speech, resulting in a less realistic sound. Conversely, overly complex models can become computationally demanding, making real-time applications infeasible. It is a tricky balance to achieve, and one that is actively being researched.
RNN architectures can be tuned and modified using a range of training tricks to improve their performance. Techniques like regularization and batch normalization can help to prevent overfitting and ensure that the models generalize well to unseen data.
Researchers are actively exploring hybrid autoregressive models, which combine the strengths of RNNs with other network types, to improve the accuracy and efficiency of automated speech recognition systems. These hybrid systems leverage the power of RNNs for sequential processing while incorporating other components designed for specific tasks, like capturing different speech features.
RNNs play a significant role in voice cloning technology, providing the means to model the complexity and variability of human speech. While progress has been made in recent years, challenges remain, especially in accurately representing the intricate details and nuanced features that make voices so unique. As the field evolves, we can expect further research into refining the capabilities of RNNs for voice cloning, particularly in areas like noise reduction, multilingual cloning and voice customization for various types of audio content.
More Posts from clonemyvoice.io: