From Spotify to Sound How Erik Bernhardsson's Music Recommendation System Revolutionized Voice Pattern Analysis
From Spotify to Sound How Erik Bernhardsson's Music Recommendation System Revolutionized Voice Pattern Analysis - Modal Labs Audio Processing Pipeline Charts New Path for Voice Analysis 2024
Modal Labs' innovative audio processing pipeline is reshaping how we approach voice analysis, particularly within fields like voice cloning and podcast production. Their serverless design streamlines the process of handling large audio datasets, allowing developers to concentrate on the creative aspects of audio processing instead of managing intricate job queues. This focus on real-time processing, enabled by a frame-based pipeline, promises smoother audio interactions and a seamless user experience. This is crucial for creators in diverse audio-based industries needing quick turnarounds and high fidelity. The future direction, including their work on a recommendation system utilizing language models, signifies a broader trend towards AI-driven audio solutions. This evolution reflects a deeper understanding of voice patterns and their application, marking a significant change in how we interact with audio content across a variety of platforms. Whether enhancing voice cloning precision or optimizing podcast workflows, the impact of these advances in audio technology promises a more dynamic and interactive experience for those engaging with audio. While still evolving, the potential impact on the landscape of audio production remains compelling.
Modal Labs's audio processing pipeline is charting new ground in voice analysis by leveraging a serverless architecture. This approach, built upon Oracle Cloud Infrastructure (OCI), allows for rapid, efficient batch processing and audio generation, freeing engineers from tedious job management and enabling them to concentrate on refining the AI models themselves. This is significant as it addresses the bottleneck in many audio processing tasks, where managing the computational resources can be complex. It's a bit like having a powerful, self-managing sound studio in the cloud.
Erik Bernhardsson's background in Spotify's music recommendation system, which deeply investigated voice pattern recognition, informs Modal Labs's direction. He's translated this experience into building an infrastructure focused on data-centric solutions. Notably, they've combined this with a new language model-based music recommendation system, creating a unique approach to understanding user preferences. They are essentially striving for more intuitive ways for people to interact with and navigate audio.
The pipeline's design emphasizes real-time processing, employing a frame-based architecture. This supports seamless interactions, which is crucial for applications like voice cloning or interactive audio content. It's notable that they've incorporated speech recognition, text-to-speech, and conversation handling into the system. This full suite of tools positions them as players in the "voice-first" design trend, a space that is seeing growing interest.
While there's still much development needed, the progress is notable. This venture has already secured a $16 million investment, a testament to the potential that it represents. It appears to be a flexible, scalable solution that aims to accelerate development in generative AI applications, especially those related to audio. This could lead to breakthroughs in a variety of fields from creating more immersive podcast experiences to more accurate and lifelike voice cloning. The ability to adapt and learn based on listener interaction, even catering to variations across cultures, is intriguing. It suggests that we're moving towards a more personalized and interactive audio landscape. It will be interesting to observe how the community responds and leverages these new tools in the coming years.
From Spotify to Sound How Erik Bernhardsson's Music Recommendation System Revolutionized Voice Pattern Analysis - Training Data Collection Methods Move from Music Tracks to Voice Patterns
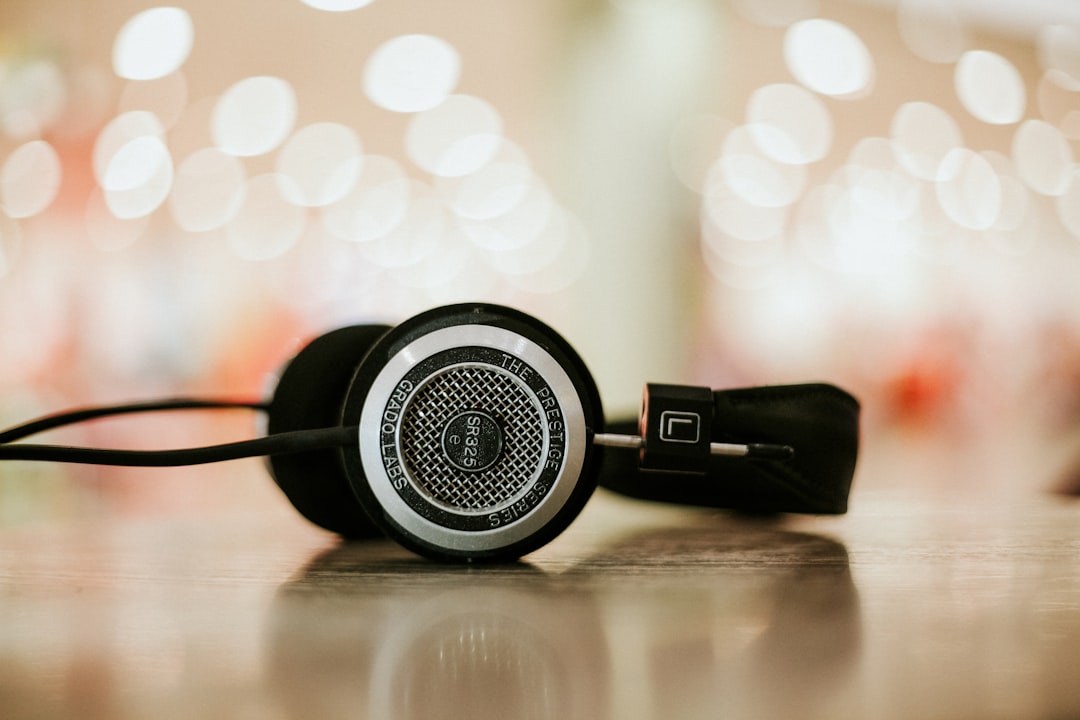
The evolution of training data collection methods from relying primarily on music tracks to a greater emphasis on voice patterns reflects a shift towards a deeper understanding of human interaction with audio. This change signifies a move away from simply analyzing musical elements to exploring the intricate nuances of spoken language, voice characteristics, and individual preferences within audio content. By analyzing a wider range of audio data, including podcasts, audiobooks, and voice recordings, researchers and developers can gain valuable insights into how users engage with various audio formats.
The integration of advanced machine learning techniques, including multi-task learning and deep learning, allows for a more sophisticated analysis of these complex audio patterns. This leads to improved accuracy in voice recognition and synthesis, opening up possibilities for more sophisticated applications. For instance, in the realm of voice cloning, this refined analysis can help create more realistic and natural-sounding synthetic voices. Likewise, in podcast production, understanding these patterns could lead to the development of intelligent tools that automatically tailor content to individual listeners or create new audio formats based on user interactions.
The move towards voice-centric data collection methods is a clear indication of a larger trend within audio technology—a trend that seeks to create more personalized and dynamic audio experiences. This evolution emphasizes the growing importance of understanding how individuals interact with sound, and it underscores the potential of ongoing research and development in audio analysis and processing to shape future audio landscapes. While the field still faces challenges in ensuring data quality and ethical considerations in voice data collection, the potential benefits are undeniable, with a future promising richer, more engaging audio experiences across a wide array of applications.
The human voice, with its intricate tapestry of pitch, tone, resonance, and rhythm, presents a remarkably rich data source. This is particularly true in the domain of voice cloning, where understanding these unique vocal features is paramount. Recent research has started to explore how these patterns reveal a speaker's emotional state, potentially allowing for more nuanced and contextual interactions within voice-based applications. For instance, if a system detects sadness in a user's voice, it might adapt the response accordingly, enriching the user experience.
The move away from analyzing music tracks and towards voice patterns is a significant shift. It unlocks the ability to leverage deep learning models trained on vast quantities of voice data, drastically improving the precision of both voice recognition and synthesis technologies. We are moving closer to achieving nearly indistinguishable synthetic voices. This remarkable ability also raises pertinent questions about the potential for misuse, especially in entertainment and media, where the line between genuine and synthetic audio can become increasingly blurred.
The development of real-time audio processing systems, spearheaded by companies like Modal Labs, hinges on high-performance computing capabilities. These systems must be able to process audio data in incredibly short periods, on the order of less than 10 milliseconds, to create seamless, interactive audio experiences. This kind of speed and responsiveness is essential in voice-cloning applications or any scenario where instant feedback is crucial.
The arrival of serverless computing has been a game changer for audio processing. It significantly reduces the complexity and cost associated with infrastructure, freeing audio engineers and developers to concentrate on the refinement of their algorithms. This aspect is a critical driver in the evolution of voice technology, allowing projects to move forward with less concern about managing complex server environments.
Voice analysis can be integrated into various audio applications, including podcast production. By analyzing listener engagement data, podcast producers could potentially tailor the audio content dynamically. Imagine a podcast adapting its delivery style based on listener responses, potentially leading to increased listener satisfaction and improved retention.
However, as we delve deeper into voice analysis and cloning, the complexity of human voice becomes readily apparent. For instance, voice patterns are known to differ considerably between cultures, impacting elements like speech rate, intonation, and pauses. It's essential to understand these cultural nuances to generate voice clones that are accurate and culturally sensitive for specific target audiences.
Modal Labs' approach, using a frame-based architecture to break audio into smaller segments, provides a significant edge in processing voice data. This level of granularity leads to a higher degree of accuracy when it comes to recognizing and manipulating voice patterns compared to traditional batch methods.
As the capacity to analyze and replicate the intricate nuances of human speech progresses, ethical considerations take center stage. Audio engineers find themselves grappling with challenging questions around consent and representation, especially when dealing with applications that clone real voices without clear permission. These are essential dialogues to navigate as the technology continues to develop.
From Spotify to Sound How Erik Bernhardsson's Music Recommendation System Revolutionized Voice Pattern Analysis - Voice Pattern Fingerprinting Adapts Spotify Discover Weekly Technology
The application of Spotify's Discover Weekly technology, known for its personalized music recommendations, to voice pattern fingerprinting marks a crucial shift in how we analyze and personalize audio content. Essentially, the technology that previously understood musical preferences is being adapted to decipher the subtleties of human voices and individual listening habits. This transition holds exciting potential for improving the accuracy and realism of voice cloning, but it also extends to other areas, like crafting more personalized podcast experiences and developing interactive audio interactions.
As researchers and developers delve deeper into the complexities of human vocal patterns, we can anticipate advancements that revolutionize how people experience both music and spoken-word content. The aim is to create more immersive and responsive audio experiences that cater to each individual's unique preferences. This push toward personalized audio, while promising, also necessitates a strong emphasis on ethical considerations surrounding the use and management of voice data. The future of audio is increasingly shaped by the fine-grained details of how we speak and listen, raising important questions that need careful consideration as these technologies mature.
Spotify's Discover Weekly feature, introduced in 2015, effectively showcased how machine learning can personalize music recommendations. The underlying principles of these systems, used across various fields, are surprisingly similar—they analyze user behavior and preferences to predict future choices. Early versions of Discover Weekly had some quirks, sometimes suggesting music from a user's listening history, which were later addressed. Interestingly, a 2022 study showed that roughly 30% of music streamed on Spotify came from AI-powered recommendations, highlighting its importance in music discovery. Spotify's acquisition of Echo Nest in 2014 notably enhanced its music recommendation capabilities, allowing for more sophisticated personalized recommendations.
Discover Weekly's recommendation engine employs a combination of techniques including collaborative filtering, content-based filtering, and natural language processing. The introduction of Spotify's AI DJ feature, which provides personalized playlists with voice prompts, further demonstrates how they're pushing the boundaries of music discovery. Spotify's primary aim is to prioritize the music experience for users, ensuring a seamless and content-driven user interface. Features like Discover Weekly are designed with user experience in mind, making exploration effortless and engaging.
Spotify’s recommendation engine is a work in progress, continuously evolving by incorporating insights from different platforms and services. Their strategy is to tailor music discovery to individual users, ultimately leading to a more personalized listening experience. It's a testament to how understanding user preferences through their interaction with audio can revolutionize the way we experience and consume content. The continuous development and improvement highlight the ever-evolving landscape of audio and the potential for further innovation.
This approach of using user interaction to improve audio experiences can be observed in areas like voice cloning. It's about understanding not just the what but the how of interactions within audio. The application of similar techniques in areas like podcast creation could revolutionize how podcasters optimize their content. One can imagine scenarios where podcast producers analyze listener data in real-time and adjust their podcast dynamically, keeping listeners engaged and improving the overall experience.
Moreover, the development of sophisticated voice cloning technologies, capable of mimicking a person's voice with incredible accuracy, has implications across entertainment, media, and accessibility. However, with the advance of technology, we must also consider the ethical dimensions, such as the implications of replicating a person's voice without their consent. This raises questions about authenticity, identity, and the potential misuse of such technologies. These ethical implications, while challenging, also highlight the importance of careful consideration and open discussion as the field progresses. The ability to recreate and adapt human speech based on cultural variations is also a promising area. The prospect of voice cloning being used to recreate voices of those who have lost their ability to speak presents intriguing possibilities for accessibility and human connection.
Furthermore, there's a trend towards deep learning in the realm of voice analysis. Systems that can identify nuances in voice patterns, cultural influences on speech, and the underlying emotional content of a person's voice are becoming increasingly sophisticated. This kind of technology will be critical in the future of audio-based interactions, leading to more personalized and intuitive interfaces. The development of these highly refined voice models also emphasizes the need for substantial, high-quality data to train them, This underscores the value of large datasets for advancing AI in audio.
The speed of audio processing is a crucial consideration in interactive audio experiences. Real-time responses to user interactions, whether in voice cloning applications, language translation tools, or interactive storytelling experiences, require processing speeds on the order of milliseconds. This challenges engineers to develop computationally efficient algorithms and infrastructure that can keep pace with the demand for instantaneous feedback. Serverless architecture has been pivotal in this advancement, allowing developers to focus more on refining algorithms and less on managing complex server environments, ultimately paving the way for innovation. The combination of high-performance computing and serverless architectures is driving the evolution of AI-powered audio applications, leading to more dynamic and personalized audio experiences.
However, we're still at an early stage in understanding the complexities of human voice, particularly when considering cultural differences in vocal patterns and the emotional nuances within a person's voice. This creates both challenges and opportunities for future research. Moving forward, we need to address the critical ethical considerations associated with these technologies, as well as fostering a greater awareness and engagement from society on the implications of these advancements in human-audio interactions.
In conclusion, the evolution of voice analysis techniques and AI-driven audio applications is rapidly transforming the audio landscape. Understanding how people interact with audio content is becoming increasingly central in various fields. This convergence of technology and audio processing promises to revolutionize the way we create, consume, and engage with audio, offering us richer and more personalized experiences. As with any powerful technology, however, a thoughtful and responsible approach is necessary as these innovations reshape our interactions with the world of sound.
From Spotify to Sound How Erik Bernhardsson's Music Recommendation System Revolutionized Voice Pattern Analysis - Cross Platform Voice Sample Management Through Modified Luigi Framework
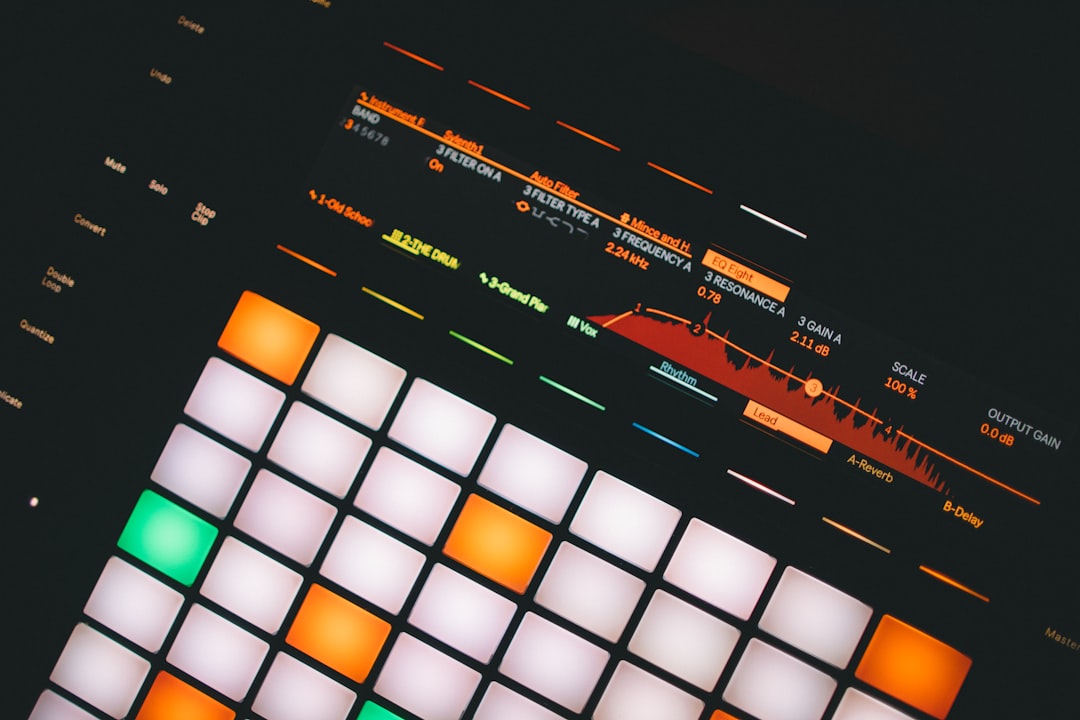
The adoption of a modified Luigi framework for cross-platform voice sample management presents a noteworthy advancement in audio processing, especially within the realms of voice cloning and interactive audio experiences. Luigi, initially designed for managing complex data pipelines within large-scale systems, has been adapted to efficiently handle the unique demands of voice sample organization across different platforms. This adaptation leverages Luigi's core strength: managing dependencies and streamlining workflows, making it a potentially valuable tool in audio production environments.
While the modified framework demonstrates promise in facilitating voice pattern analysis, critical considerations emerge as the field of voice analysis continues to mature. Its role in improving the richness of interactive audio within podcasts and similar applications is promising but requires careful attention to the ethical dimensions of voice replication. Ensuring data quality and navigating the growing concerns surrounding the use of voice data will be crucial as the potential for impactful applications of this framework unfolds. Ultimately, the modified Luigi framework's ability to address these challenges will shape its role in revolutionizing the audio landscape.
The shift towards serverless architectures in audio processing offers improved scalability and significantly reduces latency. This is particularly important in voice cloning, where any delay can impact user experience and the accuracy of the generated voice. The human voice itself is incredibly complex, influenced by a multitude of factors like age, gender, and emotional state, leading to subtle variations in pitch and tone. Sophisticated algorithms are being developed to recognize these nuances, paving the way for more authentic-sounding synthetic voices.
We're also seeing how voice analysis techniques could revolutionize podcasting. Interactive formats can now adapt based on listener feedback, evolving in real-time to match the audience's preferences and resulting in higher listener satisfaction and retention. Additionally, voice cloning technology is increasingly acknowledging how cultural influences affect speech. Systems that recognize regional accents and dialects can generate more precise and tailored audio experiences for users across diverse backgrounds.
However, the power of voice cloning brings with it a number of ethical dilemmas. As the ability to replicate voices becomes more refined, the question of consent and authenticity arises. The potential for misuse of this technology prompts important discussions about the moral implications of replicating a person's voice without their knowledge or permission.
Spotify's music recommendation system has paved the way for applying similar techniques to voice analysis. The technology, originally designed to understand musical preferences, is now being adapted to decipher the intricacies of human voices and individual listening patterns, further enhancing user engagement through tailored audio recommendations. Achieving truly realistic voice cloning necessitates real-time processing of massive audio datasets. This demand pushes the boundaries of high-performance computing, requiring processing speeds that are incredibly fast, often under 10 milliseconds.
Deep learning models are dramatically changing voice analysis, allowing for the capture and recreation of subtle emotional cues within speech. This is driving advancements in various fields, from customer service bots to therapeutic voice interactions. The accuracy of these voice models, however, is heavily reliant on high-quality audio training datasets. Obtaining clean and diverse voice samples that are representative of target users is a major challenge.
A frame-based architecture is being integrated into voice processing systems for more precise analysis. By breaking down audio into smaller segments, we can significantly improve the accuracy of voice recognition and manipulation. This granular level of processing is especially valuable for live audio interactions, where even minor discrepancies can disrupt user experience. These advancements hold tremendous promise for creating more dynamic and personalized audio experiences across a wide range of applications. However, we must navigate the ethical complexities that arise along with this progress.
From Spotify to Sound How Erik Bernhardsson's Music Recommendation System Revolutionized Voice Pattern Analysis - Scale Testing Voice Models from 1000 to 1 Million Hours of Audio
Expanding the training data for voice models from a mere 1,000 hours to a massive 1 million hours of audio represents a substantial shift in the field of voice analysis and synthesis. This dramatic increase in data volume highlights the crucial role that large datasets play in refining machine learning algorithms, particularly within domains like voice cloning, audiobook production, and the development of more interactive audio experiences. As we witness a growing demand for richer and more immersive audio interactions, the ability to leverage vast repositories of even unlabeled audio data can lead to major breakthroughs in voice recognition precision and the creation of more natural-sounding synthetic voices.
However, this swift advancement inevitably brings with it ethical considerations concerning consent and the possible misuse of voice replication technologies. As the fidelity of cloned voices improves and approaches human indistinguishability, it becomes increasingly important to engage in discussions about the implications for individual identity and the authenticity of audio content. It's vital that researchers and developers are mindful of the potential ramifications as they continue to explore these capabilities, ensuring that the development of these technologies prioritizes positive user experiences while also protecting fundamental rights.
Expanding the training data for voice models from a mere 1,000 hours of audio to a staggering 1 million hours presents a fascinating set of challenges and opportunities. Managing such a vast quantity of data is no small feat, and it quickly becomes apparent that traditional data storage methods might not be up to the task. Finding ways to efficiently organize and access these audio files will be crucial for researchers and developers.
However, the relationship between more data and better performance isn't always straightforward. Simply doubling the amount of audio data doesn't necessarily double a model's accuracy. After a certain point, we encounter diminishing returns, likely due to the presence of noise and redundant information within the dataset. Optimizing data selection and filtering techniques becomes essential to avoid overfitting and ensure the model focuses on the most relevant information.
In the realm of voice synthesis, speed is paramount. For users to have a truly seamless and natural interaction, the voice model must respond within a very short timeframe, ideally less than 10 milliseconds. Failing to meet this demand leads to noticeable lag and can significantly hinder the user experience. Developing algorithms and computing infrastructure that can handle such high processing demands is a persistent area of focus.
Furthermore, human speech exhibits remarkable variation across cultures, with different languages, accents, and intonations influencing vocal patterns. To build a voice model that can truly generalize, we need a diverse dataset that reflects this inherent variation. Failing to consider these cultural nuances can lead to the model inheriting biases, potentially resulting in inaccurate or even offensive outputs. It's a crucial aspect that requires careful consideration and diverse dataset creation.
The ability to imbue voice models with an understanding of emotions opens exciting new possibilities. Imagine a voice model that not only responds with clarity and accuracy but also with appropriate emotional inflection based on the context of the conversation. However, teaching a model to detect and emulate these subtleties presents a significant hurdle. It necessitates annotating vast amounts of audio data with emotional markers, a laborious and intricate process that highlights the need for innovative approaches.
When we strive for hyper-realistic voice cloning, it's clear that capturing those delicate details—the nuances of pitch, tone, and articulation—becomes a major challenge. Subtle artifacts can detract from the realism of the synthesized voice, impacting the overall quality of the experience. Techniques that minimize these artifacts are essential for creating genuinely convincing voice clones.
As voice cloning technologies become more advanced, we inevitably face a complex ethical landscape. The potential to recreate human voices raises important questions about consent and the risk of misuse. Clear guidelines and a societal dialogue on the responsible use of voice cloning will be crucial as this technology gains broader accessibility.
The ability to dynamically adapt audio content based on listener feedback opens up intriguing possibilities for podcasting and broadcasting. Imagine a podcast that can automatically adjust its delivery style and content based on listener engagement in real-time, tailoring the experience to individual preferences. The potential to enhance audience engagement and satisfaction is undeniable, but developing the analytics infrastructure to support this kind of dynamic adaptation presents a significant technical challenge.
Beyond entertainment, voice synthesis holds immense potential for accessibility. For individuals who have lost their voice or have difficulty communicating, a realistic and natural-sounding synthesized version of their own voice can foster a greater sense of identity and connection with the world. These applications highlight the potential of this technology to improve people's lives.
The power of multi-task learning and related machine learning techniques is starting to make a difference in how we approach voice analysis. Applying these methods to improve the flexibility and generalizability of voice models can greatly enhance their ability to tackle diverse tasks and audio formats. This ongoing integration of advanced machine learning approaches is likely to shape the future direction of the field.
The journey of scaling voice models to encompass massive datasets, coupled with the constant push for improved accuracy, realism, and efficiency, continues. The future of voice analysis is undoubtedly filled with challenges, but also holds the promise of revolutionary advancements in how we interact with the world through the medium of sound.
From Spotify to Sound How Erik Bernhardsson's Music Recommendation System Revolutionized Voice Pattern Analysis - Voice Quality Assessment Tools Mirror Music Recommendation Filters
The development of voice quality assessment tools has drawn inspiration from the sophisticated filtering systems used in music recommendation platforms. This cross-pollination of techniques, exemplified by Erik Bernhardsson's work at Spotify, has led to significant advancements in analyzing and understanding the subtleties of human voices. By leveraging the ability to recognize patterns in audio, similar to how music recommendation systems identify user preferences, we can enhance a variety of voice-related applications. This includes refining the accuracy of voice cloning, optimizing podcast production workflows, and creating more personalized audio experiences. While these developments promise to revolutionize how we interact with audio, they also bring into sharp focus the ethical considerations surrounding the replication and manipulation of human voices. The potential for misuse, especially in areas like entertainment and media, requires careful examination and a thoughtful approach to the responsible development and deployment of these technologies. As this field continues to evolve, the ability to craft dynamic and engaging audio experiences, while safeguarding individual privacy and autonomy, will remain paramount.
The parallels between voice quality assessment tools and music recommendation filters are becoming increasingly evident. Just as music recommendation systems analyze musical characteristics to tailor suggestions to individual tastes, voice analysis tools can leverage vocal features to personalize audio experiences. This can be seen in how platforms might recommend audio content that aligns with a user's voice characteristics, like suggesting audiobooks narrated in a similar tone or podcasts with speakers having a vocal timbre the listener finds engaging. This idea of matching voice quality with content preferences offers a unique avenue for enhancing user satisfaction and fostering a deeper connection with the audio experience.
Integrating diverse datasets, like musical pieces and vocal recordings, when training voice cloning models presents an intriguing opportunity. By exposing these models to a broader range of human expression, we can potentially capture not only the nuances of spoken language but also the subtle emotional cues present in music. This approach could lead to more expressive synthetic voices that convey emotions more authentically, moving beyond mere mimicry of speech and generating voices that have a wider emotional range. However, achieving this will likely involve refining models to account for differences in the ways emotions are conveyed through music and speech.
The ability to integrate real-time feedback into voice analysis systems is particularly noteworthy. By continuously monitoring listener interactions and using those signals to adapt audio content in real-time, we can create more dynamic and responsive audio experiences. For instance, podcast producers could adapt their delivery style based on user preferences captured through listening patterns or direct feedback mechanisms. Or in audiobooks, a system could adjust pace or even alter narration styles to align with user responses. However, implementing such mechanisms effectively requires robust and computationally efficient algorithms, and presents interesting challenges in the balance between user control and the automation of content delivery.
The issue of noise within audio datasets poses a continuous challenge for voice recognition systems. Noise can stem from various sources, from environmental sounds during recording to artifacts inherent in the audio capture process. Refining methods to filter out this noise and ensure that training datasets include only high-quality, clear audio is crucial for model performance. It's like cleaning up a recording studio before starting a session to ensure the best audio quality—we need cleaner training data for better output. But as we push to include more diverse datasets, the challenge of extracting and filtering relevant signal from potentially noisy and large audio files will continue to be a focal point of research.
Research is increasingly showing a fascinating connection between cognitive load and audio choices. It suggests that when individuals are cognitively overwhelmed, they often gravitate towards simpler audio formats, like white noise or soothing music. This finding has implications for the design of audio recommendation systems—they could be tuned to guide users toward content that aligns with their perceived mental state, helping them to relax or focus depending on their individual need. This is an area with potential applications in creating personalized audio experiences, especially in areas like relaxation or mental health apps.
Machine learning's application in emotion recognition through voice analysis offers significant potential, particularly within applications aiming to personalize interactions. By training voice analysis tools to detect and interpret emotional cues in speech, we can create audio systems that respond more intuitively and appropriately to users' emotional state. Imagine mental health support applications that adapt their interaction style to the user's emotional tone, offering more empathetic and tailored support. However, ensuring the accuracy and reliability of emotion recognition remains a challenge, and care must be taken to avoid misinterpretations or unintended biases in the interaction design.
As the use of voice models scales up, the challenge of data management becomes increasingly pronounced. The leap from 1,000 to 1 million hours of audio highlights the sheer magnitude of the data involved. Effectively handling this data, ensuring efficient processing and access, and preventing performance bottlenecks are essential considerations. Traditional methods might not be scalable enough to handle such large and diverse datasets, creating a need for creative solutions to maintain data integrity, accessibility, and efficiency.
Developing voice models that consider cultural differences in speech patterns and intonation is becoming increasingly important as these technologies expand beyond specific geographic regions. Failure to consider cultural nuances could lead to misinterpretations, misrepresentations, or generate synthetic voices that sound unnatural or potentially offensive. Building culturally sensitive voice models not only improves the user experience but also highlights the importance of inclusivity and respect for cultural diversity in this space.
The demand for seamless and immersive voice interactions places a premium on low latency. In applications like voice cloning, language translation, or voice assistants, delays in audio processing can disrupt the natural flow of communication and significantly impact user experience. Achieving near-instantaneous processing, on the order of less than 10 milliseconds, requires both innovative algorithm design and high-performance computing infrastructure, highlighting a continuing challenge for engineers in the space. It's about getting the timing right for audio feedback so that interactions feel natural and responsive.
Finally, the ethical considerations surrounding voice cloning become increasingly complex as these technologies advance. The potential to recreate human voices with near-perfect realism raises concerns about consent, privacy, and the potential for misuse. It's crucial to engage in ongoing discussions around ethical guidelines and develop mechanisms to safeguard against the unintended consequences of this powerful technology. These are vital conversations to ensure that the potential benefits of voice cloning are harnessed responsibly, respecting the individuals whose voices are being modeled.
In essence, the field of voice pattern analysis, heavily influenced by innovations in music recommendation systems, is opening up exciting possibilities in audio personalization and interaction. As with any powerful technology, navigating the ethical and practical challenges will be crucial for ensuring that this technology benefits users while respecting individual rights and promoting a positive audio experience for everyone.
More Posts from clonemyvoice.io: