Get amazing AI audio voiceovers made for long-form content such as podcasts, presentations and social media. (Get started for free)
Real-time Voice Quality Monitoring How AI Detects Anomalies in Live Audio Streaming
Real-time Voice Quality Monitoring How AI Detects Anomalies in Live Audio Streaming - Voice Pattern Analysis Through Machine Learning Detects Speech Interruptions
Machine learning is refining how we detect interruptions in speech, a critical aspect of improving audio quality for diverse applications like audiobooks and podcast creation. The recently developed WavLM SI model showcases encouraging results in pinpointing disruptions in spoken content, enhancing the potential of real-time audio monitoring. This model's streamlined architecture simplifies implementation and addresses the rising emphasis on eco-friendly technology within audio production. Moreover, the incorporation of Voice Activity Detection within Automatic Speech Recognition systems helps to minimize energy consumption, signifying the vital role of noise control in varied settings. As voice analysis continues to mature, we anticipate a deeper comprehension of speech-related emotions and stress levels, promising to elevate user experiences in all audio formats, including voice cloning and sound design. This can lead to a better understanding of voice performance, allowing for the creation of more realistic, emotive, and engaging audio content. The ability to quantify aspects like phoneme degradation through metrics like entropy-based Gini also suggests that we are only at the beginning of an era where very fine grained analysis of audio will be possible, eventually leading to more robust AI driven solutions in areas like voice cloning and sound editing.
Researchers are exploring how machine learning can dissect the intricacies of speech, not just for recognizing what's being said but also for understanding *how* it's said. A recent development, WavLM SI, demonstrates the capability to pinpoint when speech interruptions occur, a task crucial in various audio applications. Interestingly, this model's design prioritizes efficiency, making it suitable for a wider range of use cases by reducing its computational burden and size, which is environmentally beneficial as well.
The ability to identify speech interruptions is particularly relevant for voice activity detection (VAD), often used in Automatic Speech Recognition (ASR) systems to filter out unwanted noise and improve efficiency. However, researchers have discovered that extended pauses can skew quality assessment in real-time voice analysis. Insights from studies like P563 suggest that a balanced input, somewhere between 25-75% speech activity, might be optimal for these systems.
Historically, voice comparison relied on traditional classification models. Now, the application of machine learning in this area is revolutionizing our understanding of speaker characteristics. Moving beyond mere identification, AI can be leveraged to delve into nuances like speech emotions, stress levels, and even speech quality. This is particularly intriguing when we think about its potential in audiobook or podcast production.
Furthermore, researchers are investigating deep learning methods to refine the evaluation of speech quality. Concepts like entropy-based Gini are being explored to better quantify the degradation of phonemes in a spoken utterance. The broader scope of voice analysis, encompassing the application of AI technologies to identify meaningful patterns in spoken language, is continuously evolving.
Deep learning's potential extends to domains such as virtual reality and public speaking where recognizing emotions or stress in a speaker's voice could revolutionize audience engagement. Through the sophisticated application of neural networks, deep learning promises to improve voice quality estimation based on the performance of phonemes. This kind of real-time evaluation is a powerful tool for creators of audio content, enabling them to fine-tune a speaker's delivery or adjust content for optimal listener engagement. However, achieving universal solutions in voice analysis, especially in the realm of speech interruption detection, remains a challenge due to the diverse nature of spoken languages and dialects across the world.
Real-time Voice Quality Monitoring How AI Detects Anomalies in Live Audio Streaming - Neural Networks Track Audio Quality Fluctuations in Podcast Production
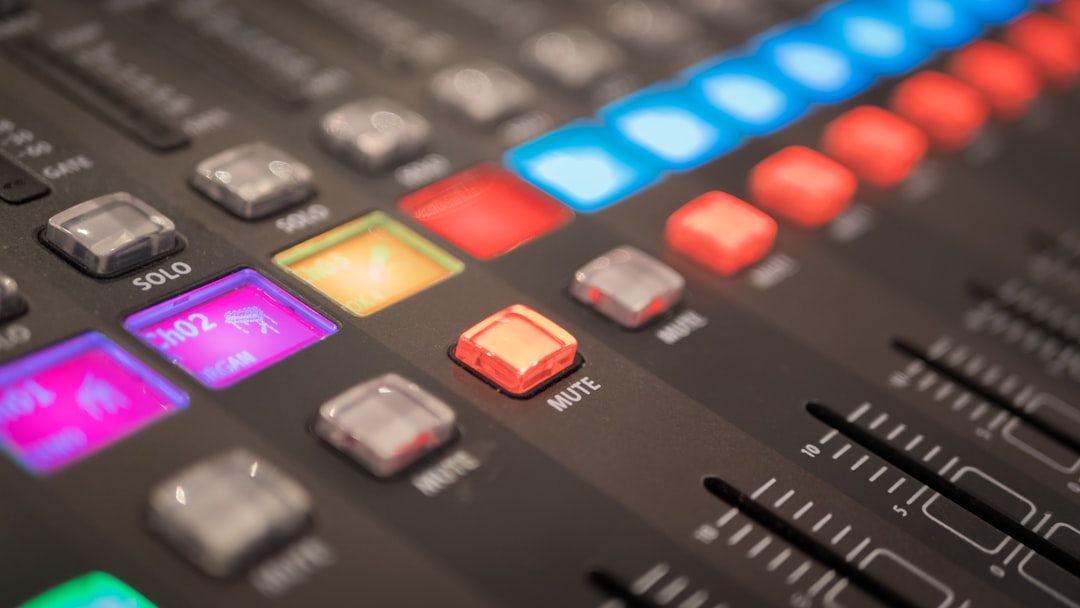
Neural networks are becoming increasingly important tools in audio production, particularly in the realm of podcasts, where maintaining consistent audio quality is paramount. Real-time monitoring of audio quality, driven by these networks, is allowing producers to identify and address issues that might otherwise go unnoticed. Techniques like audio super-resolution, powered by neural networks, are capable of improving audio clarity, even when the initial source material has a low bitrate. The ability of architectures like LSTM and TCN networks to track fluctuations in audio quality in real-time is crucial for detecting sudden changes or anomalies that could negatively impact the listener's experience. The application of neural networks to audio production holds immense promise for enhancing both the technical and artistic aspects of creating audio content. This can lead to audio that is crisper, more engaging and allows creators to fine tune a speaker's performance in new and inventive ways. A persistent issue, though, lies in guaranteeing consistent audio quality across various recording environments and audio sources. As this technology advances, it will be vital to address these challenges to ensure the consistent quality that listeners expect.
Neural networks are becoming increasingly sophisticated in their ability to analyze audio quality in real-time, a critical aspect for applications like podcast and audiobook production, even voice cloning. For instance, WaveNet, a model initially developed by DeepMind, offers a promising avenue for analyzing raw audio data, allowing for fine-grained quality monitoring. While initially conceived for audio generation, its potential for real-time analysis is gaining traction.
One area where neural networks excel is in audio super-resolution. By employing these networks, we can reconstruct higher quality audio from lower bitrate sources. Imagine enhancing the clarity of a podcast recorded with a low-quality microphone – these networks are able to fill in the missing audio information to some degree, enhancing the overall listening experience.
RNNs, particularly LSTM and GRU variants, are well-suited for modeling audio signals as they can maintain a persistent internal state, making them ideal for real-time scenarios. These models are even being explored for the creation of real-time audio effects, opening up exciting new possibilities for sound design and manipulation. However, the computational load of these models can be a challenge in many cases.
To address the limitations of RNNs, researchers are also looking at Temporal Convolutional Networks (TCNs). TCNs offer an alternative approach to analyzing audio, often achieving comparable performance with lower latency, which is vital for real-time applications. Libraries like RTNeural, a C library specifically designed for real-time inferencing, are making it easier to run small neural networks with high accuracy and efficiency for tasks such as audio quality assessment.
Anomalies in audio streams, such as sudden drops in volume or unexpected noises, can be readily identified through neural network analysis. This capability is particularly valuable for podcasters and audiobook producers who need to ensure a consistent and professional sound.
Interestingly, the concept of end-to-end neural audio codecs is pushing the boundaries of audio compression. Models like SoundStream, which utilize an encoder-decoder structure, can effectively compress and transmit audio while preserving high quality. This could lead to more efficient and bandwidth-friendly solutions for streaming audio content, potentially even in the future for voice cloning technologies.
Although these advancements are encouraging, we're still in the early stages of understanding the full potential of neural networks for audio production. Furthermore, factors like the specific neural network architecture and the nature of the audio being analyzed greatly impact the effectiveness of these tools. The diversity of human voices and audio environments introduces inherent complexities for universal solutions. The ongoing challenge is to develop more robust and adaptable neural networks that can effectively handle the wide range of audio encountered in practical applications.
As the field continues to evolve, we can expect to see more advanced models developed that better capture the subtle nuances of sound. This includes not only detecting interruptions but also capturing emotion and even speaker fatigue through voice analysis. These capabilities could revolutionize the way audiobooks, podcasts, and voice-cloned materials are created, potentially allowing creators to tailor their output for maximum impact.
Real-time Voice Quality Monitoring How AI Detects Anomalies in Live Audio Streaming - Speech Recognition Models Filter Background Noise During Live Broadcasts
Modern speech recognition models are becoming increasingly adept at filtering out unwanted background noise during live audio streams. This capability is crucial for applications that prioritize clear audio, including podcasting, audiobook production, and even voice cloning. These models leverage deep learning approaches, often employing algorithms like Butterworth filters specifically designed to isolate human speech frequencies (300 Hz to 1500 Hz), effectively minimizing the impact of ambient noise while retaining the essence of the spoken words. Further enhancing the clarity, real-time voice activity detection (RTVAD) systems can differentiate between speech and silence, optimizing the recognition process. While substantial progress has been made, the challenge of creating compact and resource-efficient models remains, particularly for devices with limited processing power. This highlights the need for ongoing development in audio processing technology, aiming for better efficiency and accessibility. As these technologies evolve, the potential for intricate audio analysis could profoundly alter how we produce and experience audio content.
Recent advancements in artificial intelligence, particularly deep learning, have made significant strides in enhancing the quality of speech recognition during live broadcasts. One of the key challenges has been the development of efficient models that can filter out background noise without sacrificing processing speed, especially when deployed in environments with limited computational resources.
Techniques like spectral gating help isolate human speech from background noise by scrutinizing the frequency components of the audio. This allows the model to emphasize the important speech signals while effectively minimizing distractions such as crowd noise or static. Meanwhile, algorithms for Voice Activity Detection (VAD) play a crucial role in identifying spoken portions within an audio stream. By leveraging machine learning, these algorithms not only detect when someone is speaking but also aid in enhancing clarity by removing unwanted silences or background noise – vital for a smooth broadcasting experience.
Many speech recognition models utilize spectrograms, which are visual representations of sound frequency changes over time. This detailed analysis provides a deeper understanding of how sound frequencies evolve, which in turn helps the models distinguish between speech and various environmental sounds. This capability helps ensure robustness in demanding real-time audio applications.
Convolutional Neural Networks (CNNs), and Recurrent Neural Networks (RNNs) like LSTMs, are frequently combined within these models to boost the accuracy of noise reduction. The CNNs are especially effective at recognizing spatial aspects of the audio, while the RNNs, thanks to their internal memory, excel at handling the temporal nature of speech. This dual approach contributes to a more comprehensive model, suitable for handling the dynamic nature of live audio analysis.
In multi-speaker environments, some systems utilize localization algorithms. These algorithms help pinpoint the origin of sound sources, which is useful in scenarios where several speakers might be present. This helps to focus on the primary speaker's voice and reduce interference from other sources.
The use of adaptive filtering in speech recognition is becoming more prominent, as it allows the model to continuously adjust its response to new types of background noise. This is a crucial feature for live broadcasts, where unpredictable audio conditions can occur, ensuring consistent and high-quality voice clarity throughout a performance.
Furthermore, to objectively evaluate the performance of a speech recognition system, metrics such as PESQ and STOI are incorporated. These metrics offer a quantifiable representation of the audio's quality, allowing developers to automate adjustments to optimize the audio signal for the best possible clarity and listening experience during streaming events.
Increasingly, researchers are striving to develop speech recognition models that can function across a broader range of languages and dialects. This flexibility is important for global audiences in a world that is becoming more interconnected through live broadcasting.
Integrating short-term and long-term memory within neural models enhances the retention of speech context, further improving the accuracy of noise reduction. Maintaining a relevant context for an ongoing dialogue is critical for dynamic conversations where the speech pattern and background noise could rapidly shift, a trait very common in a live broadcast.
In some instances, speech recognition systems are built with real-time feedback mechanisms, allowing users to receive instantaneous cues on the clarity of their voice. This kind of feedback, based on the detected quality of speech, empowers speakers to make instant changes in their volume or pronunciation, optimizing their audio for a better overall listener experience.
While impressive strides are being made in speech recognition, especially in the area of noise reduction for live broadcasts, there is still much work to be done in refining these techniques. There are still limitations and areas for improvement, particularly when handling a variety of audio environments and the diverse nature of human voices. Nonetheless, the progress to date is encouraging, highlighting the potential for AI to continue revolutionizing the realm of audio production and communication.
Real-time Voice Quality Monitoring How AI Detects Anomalies in Live Audio Streaming - Automated Quality Control Systems Alert Audio Engineers to Distortions
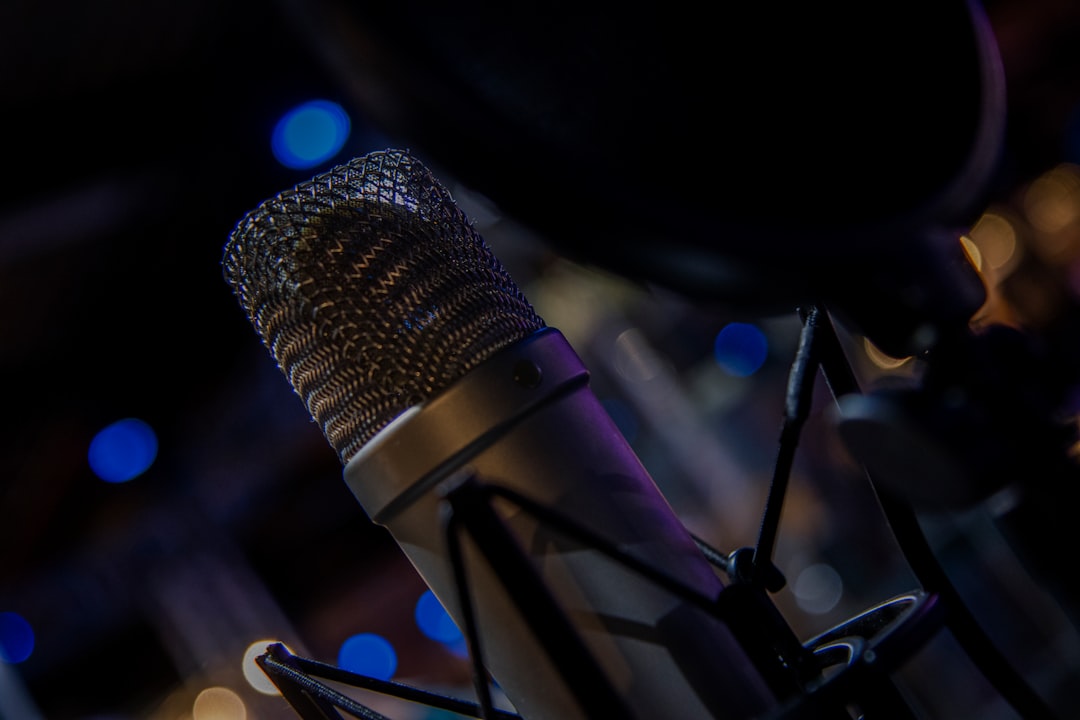
Automated quality control systems are increasingly important for audio engineers, providing real-time alerts whenever audio distortions or other issues arise. These systems rely on sophisticated signal processing and machine learning techniques to constantly monitor audio streams, ensuring audio quality remains consistently high. By identifying distortions immediately, they improve the listening experience and safeguard a producer's reputation, preventing potential issues arising from low-quality audio. As these technologies improve, they're expected to minimize the need for manual checks, allowing engineers to dedicate more time to creative aspects while upholding rigorous audio standards. However, hurdles remain in making these systems adaptable to various audio environments and the diversity of human voices, highlighting the necessity for ongoing development and refinement.
Automated quality control systems are becoming increasingly important in audio production, especially in areas like audiobook production, podcast creation, and even voice cloning, where maintaining a high standard of audio is crucial. These systems can detect distortions in audio right at the source, allowing engineers to address problems before they become embedded in the final product. This proactive approach helps to minimize the risk of audio quality degradation, ensuring a smoother listening experience for the audience.
Typically, these systems are designed to be most effective within the frequency range of 300 Hz to 1500 Hz, which encompasses the primary range of human speech. This focus on the human voice helps in effectively filtering out background noise while preserving the clarity of spoken words. Engineers can also receive real-time alerts from these systems, notifying them not only of interruptions but also of any subtle tonal distortions in the audio. This instant feedback allows for rapid adjustments in live scenarios, leading to a consistently high-quality audio stream for listeners.
Interestingly, the insights gleaned from these automated systems aren't limited to just speech. These analyses can be applied to sound design and audio mixing for a broader range of multimedia projects, fostering a higher degree of production quality and consistency across various audio elements. One intriguing aspect of this technology is its ability to decipher different speakers in a single audio recording, allowing engineers to closely track audio quality in more complex scenarios like panel discussions or interviews.
These systems also exhibit a degree of machine learning adaptability. They can learn from past audio data, tailoring their algorithms to improve the accuracy of distortion detection over time. This is helpful as it allows the system to better identify and respond to distortion patterns that might be specific to particular recording environments or equipment.
Furthermore, engineers can quantify the level of phoneme degradation using entropy-based metrics. This allows for very fine-grained analysis, which is particularly important in advanced applications like voice cloning, as it can help enhance the overall realism of the cloned voice. Spectrograms are often utilized by automated systems to visually represent audio frequencies over time, providing deeper insights into audio quality issues and aiding in corrective adjustments.
Background noise poses a constant challenge to audio quality assessment. Studies consistently demonstrate that ambient noise can significantly reduce speech intelligibility, which can further complicate the task of maintaining a consistent sound experience. The ability of these systems to effectively filter out background noise is therefore essential for maintaining audio clarity, especially in scenarios like podcasts and live events.
Real-time adjustments to audio encoding parameters based on ongoing quality monitoring are another capability of these systems. This adaptability can significantly contribute to maintaining a more consistent audio quality across different parts of a recording, particularly in dynamic contexts such as podcasts where the content and audio sources might change frequently. However, achieving perfect consistency across diverse audio environments and devices still remains a challenge. The development of more robust, adaptable algorithms that can effectively manage a wide array of acoustic conditions is an ongoing area of research and a necessary step for widespread adoption of these technologies.
Real-time Voice Quality Monitoring How AI Detects Anomalies in Live Audio Streaming - Voice Synthesis Monitoring Identifies Cloned Voice Authentication Issues
The increasing sophistication of voice cloning technology, particularly zero-shot multi-speaker text-to-speech systems, has heightened concerns about voice authentication. These systems can readily generate highly realistic synthetic voices, raising the specter of identity theft and fraud. While voice cloning offers exciting possibilities in creative industries like audiobook production and podcasting, it also presents serious risks to security and privacy.
The ability to easily mimic someone's voice using limited audio samples makes traditional voice-based authentication methods less reliable. Consequently, there's a growing need for real-time detection systems capable of differentiating genuine voices from synthesized ones. Deep learning, particularly deep neural networks, is showing promise in this area, providing a more robust approach to voice quality monitoring.
These monitoring systems aim to detect anomalies and identify potentially synthesized voices, thereby preventing the misuse of cloned voices in various applications. This ongoing development of voice synthesis monitoring is crucial, given the potential for harm stemming from deepfake voice conversions in the increasingly audio-centric world of content creation. The challenge remains to create solutions that are effective across diverse voices and audio environments.
The increasing sophistication of voice synthesis, particularly voice cloning, has made it easier to replicate human voices, raising concerns about the reliability of voice authentication systems. Even subtle shifts in voice characteristics like pitch or tone during the cloning process can potentially expose weaknesses in systems that rely on voice as a verification method. This highlights the need for improved techniques that can discern between genuine and synthetic voices.
Capturing the nuances of human speech, specifically phoneme variability, is crucial for creating realistic cloned voices. Techniques like prosody modeling strive to not only replicate words but also to capture the emotional expression and intonation patterns of the original speaker, significantly increasing the complexity of voice authentication.
Researchers are utilizing tools like entropy measures to quantify any degradations in phonemes during the voice cloning process. By analyzing these metrics, they aim to identify glitches that might creep in during synthesis, providing insights for improving the quality and authenticity of cloned voices.
Interestingly, deep learning models have demonstrated a promising capability to predict potential authentication vulnerabilities by analyzing the intricate details of sound waves. This precision in analysis allows for the early detection of possibly fabricated or misleading audio, potentially aiding in preventing malicious use of voice cloning.
Furthermore, advancements in voice synthesis are pushing the boundaries of realism by incorporating emotion recognition features. These features allow systems to assess a speaker's stress level or emotional state, enabling even more lifelike cloned voices. However, this very sophistication also poses new challenges for authentication processes.
Within the audio production workflow, automated systems are crucial for continuously monitoring the performance of microphones. This vigilance ensures that disturbances or distortions that could impact the quality of cloned voices are immediately recognized, ensuring the reliability and integrity of voice cloning projects.
The acoustic environment plays a critical role in voice cloning. Factors like ambient noise and reverberation can significantly affect the quality of the synthesized voice. While advanced algorithms are being developed to filter out these disturbances, achieving a truly universal solution remains a challenge due to the vast diversity of recording environments.
Speaker diarization, the technique of automatically separating voices within a recording, is being integrated into voice quality systems to more precisely monitor volume fluctuations and distortions that might arise during conversations. This enhanced capability is particularly useful in scenarios where several voices, including cloned voices, are involved in the same audio.
AI techniques like audio super-resolution can improve the perceived quality of cloned voices derived from lower-quality audio files. This maintains a consistent listening experience for audiences, even when the original recordings are less than ideal. However, it simultaneously adds complexity to accurate voice cloning detection.
Looking towards the future of voice cloning, a compelling question emerges regarding ethical guidelines. Creating protocols that flag unauthorized voice clones or identify deviations from expected voice patterns could provide important safeguards in a world where audio content is increasingly created and manipulated by AI. This delicate balance between innovation and responsible use will be a defining factor in how voice cloning technology evolves.
Real-time Voice Quality Monitoring How AI Detects Anomalies in Live Audio Streaming - Real Time Audio Processing Measures Voice Clarity in Audiobook Recording
Real-time audio processing is increasingly vital in audiobook production, playing a key role in refining the clarity of the narrator's voice. This involves applying digital effects to the audio stream, usually at sample rates of 44.1 kHz or higher, to achieve a richer and more defined sound. Additionally, real-time systems use voice activity detection (VAD) to identify when a narrator is speaking versus periods of silence, streamlining audio processing. This is especially beneficial when recording in varied or less-than-ideal acoustic environments. Furthermore, artificial intelligence algorithms are being integrated to enhance recordings, particularly those with less than ideal initial quality, making them sound more polished and studio-ready. While some concerns may exist about the intrusiveness of the technologies involved, the potential for real-time adjustments offers significant advantages to creators. As this technology continues to mature, its role in audiobook production will undoubtedly continue to grow, leading to more compelling and high-quality listening experiences for the audience.
Real-time audio processing is transforming how we create and experience audio, particularly in areas like audiobook production and voice cloning. One key aspect is enhancing voice clarity. Achieving a high signal-to-noise ratio (SNR), often targeted at 60 dB or higher, is a critical step in ensuring that the speaker's voice is dominant over background noise. Listeners need to clearly understand the speaker's words without distractions. Techniques like dynamic range compression help level out the volume variations in recordings, making the audio more consistent and, frankly, more palatable.
Beyond simple volume adjustments, we're seeing a deeper level of analysis that explores the subtleties of speech. For example, algorithms can dissect audio into phonemes and detect even minor shifts in pitch – a 1% change can sometimes signify a change in speaker intent or emotion. This offers exciting possibilities for gauging listener engagement and understanding the nuanced delivery of a speaker. Incorporating real-time feedback systems allows producers to guide a speaker towards optimal vocal performance, especially in dynamic settings like a live podcast where instant adjustments can be critical.
AI is also starting to uncover insights into emotion recognition, moving beyond just what is said to understanding how it's said. By evaluating the intonation and stress patterns in a voice, it may be possible to create more authentic and impactful audio content – perhaps enhancing the ability of an audiobook narrator to sound more compassionate or emphatic, for instance. In addition, we can now quantify the clarity of audio using metrics like the speech intelligibility index (SII). This allows producers to take a more systematic approach to improving the understandability of audio in complex listening environments.
However, this push towards sophisticated audio processing also comes with challenges. Real-time processing often introduces latency, and delays of even 20 milliseconds can sometimes disrupt a natural conversational flow, reminding us that audio processing must be carefully balanced with the needs of listeners who expect live interactions.
Another fascinating aspect is the expansion of vocal ranges in AI-powered voice cloning. Models are becoming more capable of capturing the full spectrum of human voices, including those with deep basses or high trebles, creating a greater sense of realism in generated voices. And adaptive algorithms are becoming increasingly important for handling varying acoustic environments. By learning to react to changes in background noise in real-time, they can ensure that the foreground audio is always heard with optimal clarity, regardless of the environment.
It's worth considering that human perception of audio quality isn't always straightforward. Clarity, for listeners, isn't solely determined by the volume. Factors like the frequency response and the dynamic range play a significant role, showcasing the complexity of ensuring an enjoyable listening experience, especially within media like podcasts or audiobooks, which are designed to be highly engaging.
This intersection of advanced algorithms, human perception, and creative content production is exciting and continually evolving. As we see more research into these areas, it’s clear that we’ll gain more granular control over the audio experience, paving the way for more impactful and enjoyable listening experiences in the future.
Get amazing AI audio voiceovers made for long-form content such as podcasts, presentations and social media. (Get started for free)
More Posts from clonemyvoice.io: