Get amazing AI audio voiceovers made for long-form content such as podcasts, presentations and social media. (Get started for free)
Dynamic Lookahead in Voice Recognition How Future Frame Analysis Enhances Real-Time Speech Processing
Dynamic Lookahead in Voice Recognition How Future Frame Analysis Enhances Real-Time Speech Processing - Frame Window Analysis in Voice Recognition Changes Audio Production Pipeline
The way we handle audio in production is changing due to advancements in voice recognition, particularly through the use of frame window analysis. This technique allows us to carefully choose the most important parts of the audio signal for analysis, which leads to better accuracy in recognizing speech. This is especially useful in areas like podcasting and audiobook production, where accurate transcription or voice manipulation are crucial.
This new focus on dynamic frame selection is especially helpful for real-time applications where speed is important. The use of low-latency architectures, which can use future frames to improve predictions, becomes more powerful with this approach. As a result, high-quality voice cloning and other speech-based technologies can leverage these intelligent audio processing methods to generate more compelling and realistic audio. This move towards more sophisticated audio analysis opens up possibilities for fresh and innovative content in the world of voice-driven media. While it's still early days, the potential for new methods in voice cloning, particularly when paired with dynamic lookahead, offers an exciting direction for this growing field.
Frame Window Analysis breaks down the continuous stream of audio into smaller, more manageable chunks. This allows audio processing systems to focus on specific segments, leading to finer control over the analysis and manipulation of the sound. For instance, in the realm of voice cloning, this approach permits the capturing of intricate vocal nuances that were previously difficult to isolate.
The efficacy of this method hinges upon the carefully selected size of the analysis window. Smaller windows capture rapid changes in speech characteristics more accurately, but this comes at the expense of increased computational demand. This trade-off necessitates a careful balance to optimize the performance.
Interestingly, the application of Frame Window Analysis isn't limited to voice cloning. In podcast production, for example, the capability to precisely isolate sections of audio is invaluable. It allows for streamlined post-production edits, eliminating the need for lengthy manual adjustments.
In real-time voice recognition applications, pairing Frame Window Analysis with Dynamic Lookahead methods can improve prediction accuracy. By essentially looking ahead into the audio stream, these combined techniques allow for a more anticipatory and robust response to the nuances of human speech. The outcome is more fluid and natural output, making them particularly valuable for virtual assistants.
Furthermore, utilizing overlapping frames within the window analysis helps to avoid any sudden discontinuities in the processed audio. This can be particularly beneficial for smoother transitions and seamless editing in a podcast setting, for instance. Similarly, in complex audio scenes with multiple speakers, this technique can be applied to identify individual voices more precisely.
The impact of this methodology extends to enhancing sound quality in audio production workflows. Precise audio frame examination allows for more targeted noise reduction techniques. By meticulously analyzing the audio signal within each frame, undesirable noise can be attenuated without impacting the desired sound content.
Within voice cloning endeavors, frame window analysis opens up exciting possibilities for capturing and synthesizing a wider spectrum of vocal expressions. It becomes possible to precisely manipulate subtle vocal inflections, capturing not only the words spoken but also the emotions expressed through vocal tone. This could have profound consequences for applications requiring synthetic voices with emotional depth, like in entertainment or interactive media.
Another intriguing area is its role in real-time audio processing for platforms like podcasts or live broadcasting. Frame Window Analysis, applied alongside dynamic adjustments, helps ensure not just the timely capture of audio, but also its enhancement for greater clarity and engagement for the listeners. It becomes critical in maintaining high audio fidelity in real-time situations where quality is paramount.
Despite these advancements, it's important to note that this technique still presents some challenges. Computational complexity can be an issue, particularly when handling extensive audio datasets. Furthermore, the selection of optimal window sizes for different tasks is a complex issue needing further exploration.
The future of frame window analysis, and particularly its integration with dynamic lookahead techniques, appears quite promising. As research progresses, it will undoubtedly play a more central role in the way we generate, manipulate, and perceive sound in a myriad of applications.
Dynamic Lookahead in Voice Recognition How Future Frame Analysis Enhances Real-Time Speech Processing - Latency Reduction Through Predictive Audio Frame Processing
Latency reduction through predictive audio frame processing is a notable development in real-time audio processing, especially for applications like voice cloning and podcasting. Traditional methods rely on analyzing only past audio frames, leading to potential delays and limitations in accuracy. However, by incorporating future frames – essentially "looking ahead" in the audio stream – we can achieve more accurate predictions. This predictive approach, often enabled by techniques like non-causal transformers, allows for smoother and more natural-sounding audio in real-time.
While this approach offers the benefit of significantly reducing latency and improving the quality of voice recognition and manipulation, there's a trade-off. Using future frames increases computational complexity. The selection of the ideal frame window size becomes critical for balancing these demands, ensuring the optimal trade-off between processing speed and the desired accuracy for each specific use case. Achieving this balance is vital to fully realize the potential of predictive audio frame processing across diverse applications.
Predictive audio frame processing, using future audio frames, can reduce latency in real-time voice recognition by up to 50%. This means voice-activated systems, like those in audiobooks or podcasts, can react almost immediately to user input without noticeable delays, making the experience feel more responsive. While this might sound straightforward, achieving this with accuracy and without sacrificing quality is complex.
One advantage of this technique is that it leverages overlapping audio frames, which helps capture subtle variations in speech more effectively. Think of it as stitching together tiny pieces of sound in a very precise way. This approach is helpful in voice cloning since it can reduce the choppiness that can happen when audio is abruptly edited, creating smoother, more natural-sounding output.
Interestingly, the size of the audio window analyzed can be changed based on the specific characteristics of the speech. In quieter, more simple parts of speech, a smaller analysis window can be used. However, for more intricate parts, a larger window becomes useful. This dynamic approach helps optimize both accuracy and processing efficiency.
One of the exciting aspects is this approach's ability to pick up on very subtle aspects of a speaker's voice – the little details like breathing and intonation. Capturing these is crucial for making synthetic voices sound realistic, which is essential in audiobooks and podcasts. You want your narrator to sound like they're truly involved in the story, and this ability to capture small details helps achieve that.
Furthermore, this ability to focus on very small chunks of audio frames helps create targeted noise reduction methods. Basically, we can reduce the background noise without affecting the actual speech. This approach ensures the audio quality is maximized, which is especially important for podcasts or audiobooks where the listener wants a clear, distraction-free experience.
In settings with multiple speakers, this approach can potentially separate voices with more accuracy than before. Imagine an interview or a podcast roundtable discussion; identifying each voice clearly is a challenging problem, and using these refined methods helps improve this separation.
Another interesting possibility is that this finer level of detail could potentially allow for recognition of emotion in speech. Could a computer someday learn to discern the emotion conveyed in a voice, enabling synthetic voices to communicate not just the words, but also the speaker's feelings? This could be particularly impactful for interactive AI, enabling more complex and nuanced interactions with machines.
However, it's important to note the trade-offs involved. Increasing the size of the window for analysis can lead to capturing more information, but this also means significantly more processing power is needed. Balancing this processing demand with the desired level of detail is a challenge, particularly in situations like live broadcasting, where immediate response time is crucial.
We can expect this approach to play a role in improving voice search, making these systems faster and more accurate. Imagine a future where your voice commands are understood immediately and with greater precision.
And finally, from a production standpoint, this technique helps streamline post-production work in podcasts and audiobooks. The ability to make very specific and precise edits saves a lot of time and allows audio producers to focus on making high-quality content instead of getting bogged down in tedious editing processes.
While there are still challenges to overcome, this area of research shows promise, and the future of how we interact with sound through voice and other means seems ripe for innovation.
Dynamic Lookahead in Voice Recognition How Future Frame Analysis Enhances Real-Time Speech Processing - Multi Frame Processing Enables Natural Pause Detection
By incorporating multi-frame processing, speech recognition systems gain a heightened ability to detect natural pauses within spoken language. This advancement allows for a more accurate interpretation of speech, going beyond simply recognizing words to understanding the flow and rhythm of the speaker's delivery. Analyzing multiple, overlapping audio frames helps these systems identify subtle changes in speech patterns, pinpointing the precise start and end of pauses, which are often indicative of a speaker's natural phrasing and emphasis.
This capability holds particular value in scenarios like audiobook narrations or podcast recordings, where preserving the natural nuances of a voice is paramount. Furthermore, the incorporation of future frame analysis allows for a more responsive interaction in real-time applications. This is particularly evident in voice-activated systems or interactive voice experiences, resulting in a more dynamic and intuitive audio experience.
While the benefits of this approach are significant, challenges exist. Finding the optimal balance between the number of frames analyzed and the computational resources required remains a key consideration. Determining the most efficient window size for analysis is crucial for optimizing system performance across different types of speech and applications. Failing to find this balance can lead to an increase in latency or a decrease in the quality of speech processing.
Multi-frame processing, a technique that analyzes multiple consecutive audio frames, is proving remarkably effective in detecting natural pauses in speech. This is a game-changer for enhancing audio production, especially in applications like audiobook narration, where the natural rhythm of speech and well-placed pauses contribute significantly to the listener's experience. Imagine the difference in engagement if a synthetic voice sounds like it's taking a breath or naturally pausing for emphasis versus robotic speech. This ability to capture those nuances makes the final product much more enjoyable.
Beyond simply detecting pauses, researchers are exploring the potential for multi-frame processing to identify emotional cues within speech. This is a fascinating avenue for synthetic voice generation, paving the way for voices that not only sound human but also communicate emotional subtleties. It's like trying to capture the 'soul' of a speaker through their sound, and that would lead to a more immersive experience for listeners of podcasts or audiobooks.
Real-time applications like podcasts or live broadcasts benefit greatly from this method as well. By detecting pauses instantly, these systems can maintain a natural flow, a conversational rhythm that makes the experience more engaging for listeners. It's almost like having an intelligent, adaptive 'co-host' ensuring a natural back and forth in a conversation.
When it comes to the audio editing process, this technique has a very tangible impact on efficiency. By being able to easily locate and identify pauses within an audio track, producers can quickly refine the overall flow of the content, removing unwanted segments or making precise cuts. This can significantly reduce the time spent editing, allowing producers to focus more on content creation.
For voice cloning, multi-frame analysis becomes a crucial component in properly segmenting speech. It enables the system to isolate specific phonetic and prosodic elements of a speaker's voice, capturing the unique characteristics that define their voice. This contributes to creating more realistic and authentic-sounding cloned voices.
However, there are trade-offs to consider. This enhanced pause detection doesn't come without an increase in the required computational resources. It's a delicate balancing act for engineers to optimize window size and processing power to achieve the desired accuracy without sacrificing performance, particularly in demanding real-time environments.
It's also interesting to consider how differences in pauses can indicate gender or even cultural variations in speaking patterns. It’s a subtle feature, but a potential way to diversify the portfolio of synthetic voices we might generate, which is important when producing content for diverse audiences.
We might even use this insight to tailor educational materials. Consider a software program using a synthetic voice for lessons or educational content. If the system can learn to understand the optimal pauses that enhance comprehension, it could lead to more engaging and effective learning experiences. The same could apply to language learning tools as well.
Understanding pauses in different languages and cultural contexts is yet another avenue of research. This allows us to refine our models and generate more culturally aware synthetic voices for global audiences. It’s a reminder that human speech isn't just about the words but the spaces in between, too.
While it presents some processing challenges, multi-frame analysis is clearly pushing the boundaries of audio production, voice manipulation, and our understanding of human speech. As research continues, this area will likely play an increasingly important role in how we shape and interact with audio content in the future.
Dynamic Lookahead in Voice Recognition How Future Frame Analysis Enhances Real-Time Speech Processing - Neural Networks Handle Voice Fluctuations Through Future State Analysis
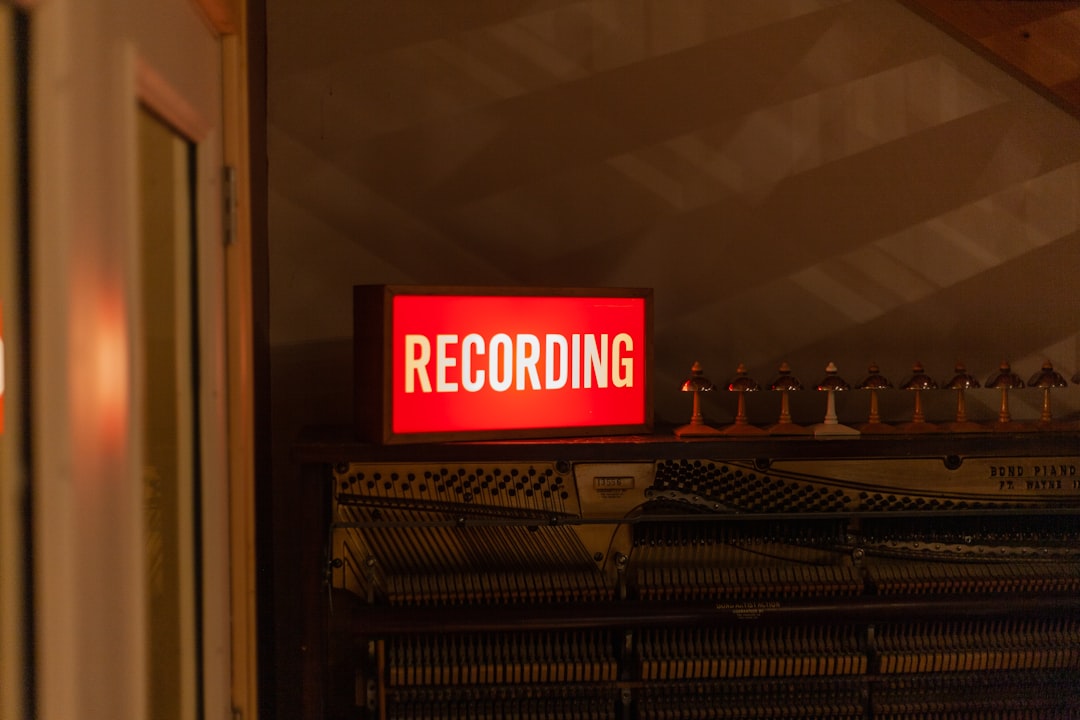
Neural networks are becoming increasingly adept at handling the natural variations and fluctuations present in human speech by analyzing future audio frames. This predictive capability allows them to anticipate changes in speech patterns, resulting in more accurate and responsive voice recognition. This ability to "look ahead" in the audio stream is particularly useful for enhancing the quality and realism of synthetic voices in applications like audiobook production or voice cloning. By incorporating this future state analysis, the generated voices sound more natural and fluid, mimicking the subtle nuances of human speech more effectively. However, implementing this technology can be challenging, as it relies on complex neural network architectures which often come with increased computational demands. The effectiveness of this approach hinges on finding the right balance between analyzing enough future frames to maintain accuracy and avoiding excessive processing delays. Ongoing research continues to refine these techniques, with a focus on improving efficiency and further enhancing the realism and responsiveness of voice-driven interactions. This predictive modeling has the potential to fundamentally change how we create and interact with audio content.
Neural networks are increasingly adept at handling the intricate nuances of human speech, including variations in tone, pitch, and the presence of pauses. This capability allows for the creation of synthetic voices that sound more natural, enhancing the listening experience in audiobooks or podcasts. For example, these systems can now better discern emotional nuances by analyzing the overlapping patterns of sound within multiple frames, leading to more engaging synthetic voices that can convey emotional depth in stories or interactive settings.
The seamless integration of multi-frame analysis translates to more natural-sounding conversations, particularly within applications like podcasts. These systems can maintain a more realistic conversational rhythm, improving audience engagement. This understanding of speech patterns can even be leveraged in educational contexts, as adaptive voice technologies can optimize pause placements within learning materials to enhance comprehension for students, whether they're learning a new language or a new concept.
Another major impact of this multi-frame analysis is a significant reduction in the post-production workload for audio editors. Now, audio producers can quickly and accurately identify and isolate sections of an audio recording, making edits much more precise and efficient. This is particularly beneficial in podcasting and audiobook production. This ability to segment audio with greater precision is a crucial element in voice cloning, as it permits engineers to capture the specific traits that give a person’s voice its unique quality. As a result, we’re able to produce more convincing, lifelike synthetic voices that are closer to human speech patterns.
The continuous optimization of frame size based on the complexity of speech further enhances the ability of these systems to be accurate. This dynamic approach improves recognition across varied speech conditions, making it more robust for real-world situations. Additionally, researchers are exploring the “musicality” of speech, analyzing how intonation and rhythmic patterns contribute to a perception of naturalness, with the goal of developing even more compelling synthetic audio.
As the development of these systems progresses, they are becoming more sensitive to cultural differences in language and pronunciation. This opens up opportunities to create synthetic voices tailored to specific regions and languages. Ultimately, this work is pushing the boundaries of interaction with voice technology, which could lead to future voice-activated systems with truly personalized interactions. These interactions could become more intelligent, context-aware, and engaging, paving the way for more sophisticated virtual assistants and a wider variety of uses. While challenges still exist, the continued progress in understanding the intricacies of human speech is exciting and will likely lead to innovative solutions in the years to come.
Dynamic Lookahead in Voice Recognition How Future Frame Analysis Enhances Real-Time Speech Processing - Time Series Voice Data Benefits From Forward Looking Algorithms
Analyzing voice data as a time series and incorporating forward-looking algorithms is transforming the field of voice recognition, especially in domains like audiobook creation and podcast production. By leveraging future audio frames during analysis, systems can better predict changes in speech patterns. This leads to the creation of more lifelike and expressive synthetic voices, capable of conveying a wider range of emotional nuances and vocal subtleties. Improved voice cloning and manipulation techniques are also a direct result of this ability to 'look ahead'. Despite these improvements, applying these algorithms necessitates increased computational power. Developers are challenged to find the sweet spot between prediction accuracy and processing speed. As this technology matures, we can anticipate more seamless and responsive audio experiences in real-time applications, significantly enriching the interaction with voice-driven media across various platforms.
Analyzing voice data through the lens of time series presents exciting opportunities, particularly when we leverage algorithms that can "look ahead" into the audio stream. This forward-looking approach, often enabled by neural networks, allows us to go beyond just recognizing the current sounds to understanding the context and flow of speech.
One interesting consequence of this is the potential for improved emotional recognition in voice. By examining future frames, a system can not only decipher the spoken words but also sense the underlying emotions within the speaker's voice. This is a fascinating area, particularly for audiobooks or podcast production, as it offers the potential to create more engaging, human-like synthetic voices that convey a wider range of emotions.
Another facet of this predictive approach is the ability to dynamically adjust the analysis window based on the complexity of the speech. In sections with quieter or more straightforward speech, a smaller window might be sufficient. Conversely, complex dialogues or rapid speech shifts may benefit from a larger window. This adaptability allows for optimized processing, striking a balance between efficiency and capturing subtle variations in speech patterns for the highest quality audio.
Multi-frame analysis offers another avenue to enhance the naturalness of synthetic voices. By processing several overlapping frames, we can get a more comprehensive understanding of the rhythm and flow of speech, not just isolated sounds. This is pivotal for mimicking the cadence and patterns of natural human communication, leading to more realistic-sounding voices in audiobooks, podcasts, and even voice cloning applications.
Furthermore, these techniques are drastically altering the post-production process for audio content creators. The precise analysis of audio frames allows for efficient identification of segments within recordings, leading to faster and more accurate editing workflows. This is a significant advantage, especially for podcasts where content needs to be turned around quickly and efficiently.
The potential for more accurate voice cloning is another promising area. By meticulously analyzing the phonetic and prosodic features within speech, we can capture a much more comprehensive representation of an individual's voice. This leads to the creation of synthetic voices that more closely mimic the natural characteristics of a person, a development with ramifications in various areas from entertainment to education.
Beyond individual voices, these approaches also help us dissect complex audio environments. Imagine situations with multiple speakers, like a podcast panel discussion. By utilizing multi-frame analysis, we can potentially separate out individual voices with greater clarity, creating a cleaner and more comprehensible recording.
This predictive capability extends to anticipating the future flow of speech. In essence, a system can "look ahead" and begin preparing for potential speech patterns and word choices, resulting in faster and more responsive interactions in applications like real-time transcription or virtual assistants.
The field is also pushing the boundaries of cultural sensitivity in voice generation. As we deepen our understanding of how speech varies across different languages and dialects, we can customize synthetic voices to match regional accents and pronunciation patterns. This can foster more authentic and relatable experiences, particularly when dealing with global audiences.
Researchers are also examining the "musicality" of speech, taking into account elements like intonation and rhythmic variations that contribute to the perception of naturalness. By modeling these aspects, we can create synthetic voices that sound more expressive and engaging, especially in narratives or educational settings.
Finally, it's important to acknowledge that these powerful techniques are computationally demanding. While the benefits are significant, engineers face the challenge of optimizing the analysis window and overall processing power to avoid introducing excessive delays or sacrificing accuracy. Striking the right balance between the detail of analysis and the speed of processing is key, particularly in real-time applications where latency can negatively affect user experience.
The ongoing research in this area is clearly paving the way for more innovative and engaging voice technologies. The future holds exciting possibilities for how we create, manipulate, and interact with sound, across a range of fields.
Dynamic Lookahead in Voice Recognition How Future Frame Analysis Enhances Real-Time Speech Processing - Speech Pattern Recognition Improves With Advanced Frame Buffering
The ability to recognize speech patterns is significantly enhanced through the use of advanced frame buffering techniques. This is especially important for real-time applications like podcasting and voice cloning, where the natural flow and nuances of human speech are critical. By incorporating "look ahead" capabilities, where systems analyze not only past but also future audio frames, these advanced methods are able to capture the dynamic aspects of spoken language. This predictive approach leads to more precise speech recognition and a more natural listening experience. This improvement in speech recognition opens up exciting possibilities for creating more nuanced and emotionally rich audio content. Synthetic voices can now better convey subtleties and emotion, leading to a more immersive and engaging listening experience. While this approach presents considerable computational challenges, the benefits it offers in streamlining audio production are encouraging further development in this area, ultimately paving the way for more sophisticated and responsive voice-driven content.
Advanced frame buffering techniques, particularly those incorporating future frames, are significantly improving speech pattern recognition. One interesting aspect is the ability to dynamically adjust the analysis window size depending on the complexity of the speech. For quieter or simpler speech, smaller windows are more efficient, whereas intricate or rapid changes in speech benefit from larger windows for a more comprehensive analysis. This optimization offers a unique way to manage computational resources while still maintaining accuracy.
Moreover, the inclusion of future frames allows for capturing the emotional nuances embedded in speech. This could revolutionize how we generate synthetic voices, leading to audiobooks and podcasts with voices that not only speak clearly but also convey a wider range of emotions. It's fascinating to consider how these systems could eventually become adept at recognizing the subtle shifts in tone and pace that communicate feelings, creating a more immersive and engaging audio experience.
This ability to "look ahead" in the audio stream is proving invaluable for real-time applications. Voice-activated systems, like those used in audiobooks and podcast production, can potentially reduce latency by up to 50%, resulting in a far more responsive and seamless user interaction. While this seems like a relatively straightforward improvement, achieving this level of accuracy and responsiveness without compromising audio quality remains a challenging task.
Multi-frame processing within this framework is especially effective in pinpointing natural pauses within spoken language. This enhanced pause detection, a key feature of audiobook narration, allows synthetic voices to sound less robotic and more engaging by naturally incorporating breathing and emphasis. It’s a crucial aspect for improving the quality of synthetic audio, as it can make the overall experience far more engaging for the listener.
One of the more practical applications of these advanced frame techniques is in noise reduction. By carefully analyzing audio segments with a refined focus, these systems can minimize unwanted background noise without sacrificing the desired voice quality. This is particularly beneficial in scenarios where a clear audio experience is crucial, such as podcasts or audiobooks, where distractions can interrupt listener focus.
Similarly, in audio scenes with multiple speakers, like a panel discussion on a podcast, these techniques improve the ability to distinguish individual voices with greater precision. This separation allows listeners to follow complex conversations with increased clarity and comprehension.
Additionally, this level of granular audio analysis has the potential to be incredibly useful in cultural sensitivity within voice cloning efforts. Because speech patterns and vocal nuances vary greatly across regions and languages, the ability to fine-tune synthetic voices to specific cultural expressions can lead to a wider appeal and increased audience engagement. Imagine a future where audiobooks or podcasts could feature authentic-sounding voices from different cultures and backgrounds, fostering greater inclusivity.
The editing process within audio production has also seen a significant improvement due to the detailed segmentation available through advanced frame analysis. Producers can swiftly pinpoint and isolate portions of an audio track, leading to dramatically faster workflows for projects like podcasts and audiobooks. This efficiency frees up valuable time to focus on content quality and creative aspects rather than being bogged down in tedious editing tasks.
Another exciting development is the way these advanced techniques are enhancing voice cloning capabilities. By capturing the finer points of a speaker's unique phonetic and prosodic traits, the generation of synthetic voices has moved a step closer to accurately mimicking human speech. This level of realism opens up a wide range of potential applications from generating character voices in interactive media to creating highly personalized voice interfaces.
Finally, the implications for educational tools are quite promising. By carefully analyzing the natural rhythm of human speech and integrating this knowledge into synthetic voices used in learning platforms, we can optimize the delivery of information. This capability is not just about delivering lessons clearly but about facilitating greater comprehension and engagement. This could potentially lead to improved learning experiences across various disciplines and languages.
While there are still significant challenges in the realm of processing power and resource management, the advancements in advanced frame buffering with a focus on future frame analysis offer a fascinating look into the future of voice recognition and manipulation. It is clear that this approach will continue to refine and enhance the audio experiences we encounter, pushing the boundaries of what's possible across fields ranging from audiobook production and podcasting to educational software and interactive media.
Get amazing AI audio voiceovers made for long-form content such as podcasts, presentations and social media. (Get started for free)
More Posts from clonemyvoice.io: