Get amazing AI audio voiceovers made for long-form content such as podcasts, presentations and social media. (Get started for free)
Mondegreens in Audio Production How Misheard Lyrics Shape Voice Recognition Technology
Mondegreens in Audio Production How Misheard Lyrics Shape Voice Recognition Technology - Misheard Lyrics in Studio Recording Sessions and How to Prevent Audio Confusion
During the recording process, misinterpretations of lyrics can cause considerable audio confusion, highlighting the importance of vocal clarity and precision in the production stage. Rapid tempos and intricate lyrics can amplify the likelihood of mishearing, resulting in interpretations far removed from the artist's intended message. To combat this, a primary focus should be placed on employing meticulous mixing techniques and ensuring vocalists deliver articulate pronunciations. These misheard lyrics aren't just about listener perception; they play a role in the evolution of voice recognition technology, exposing the difficulties in accurately interpreting spoken language across diverse contexts. This is particularly relevant to voice cloning technology as accurately recreating a human voice depends on the ability to process the nuanced details in the language and pronunciation. Recognizing and tackling these challenges is fundamental to developing audio experiences that are clear and effective, regardless of whether the output is music, a podcast, or a cloned voice.
The intricacies of how we perceive sound can lead to unexpected outcomes, especially when it comes to understanding lyrics in audio productions. Misinterpretations, or mondegreens, can be a byproduct of how our brains process auditory information, often filling in gaps with what we anticipate or have experienced previously. This can manifest in various ways during the recording and production phases, creating a need for meticulous attention to detail.
For instance, if a microphone isn't optimally positioned, capturing vocal clarity becomes difficult, potentially obscuring the intended lyrics. Understanding this link between microphone placement and audio quality is crucial. Similarly, the frequency balance within a mix can impact how certain words or phrases are perceived. Certain frequencies can be masked by instruments or other audio elements, causing a listener to misinterpret what they hear.
Emotional context also plays a part. If the intended emotion of the vocals isn't effectively conveyed during a recording, listeners might form an interpretation that doesn't align with the original intent, leading to misunderstandings of the lyrics. Further complicating things, the use of audio effects and arrangement can generate auditory illusions—similar to optical illusions—potentially warping how the listener perceives the clarity and context of the vocal performance.
These challenges are particularly apparent in audiobook productions, where a slight misinterpretation of a phrase can change the listener's understanding of the entire story. Voice actors need to articulate clearly, and engineers need to ensure that the audio is presented in a way that allows for seamless comprehension.
Furthermore, the development of voice cloning technologies has introduced an opportunity to reduce misinterpretations by utilizing context-aware algorithms in voice synthesis. While this technology is promising, its ability to perfectly replicate human speech and nuanced expression remains a challenge.
Additionally, certain phonetic similarities between words can create a breeding ground for mishearings, especially when working with layered vocal tracks. Engineers must pay close attention, utilizing spectral analysis and other tools to pinpoint potential problem areas within vocal recordings.
The existence of regional accents and dialects further complicates the situation, creating variations in how people understand lyrics. This necessitates a strong awareness of the target audience during the production process. Ultimately, audio production, particularly in the realm of voice-based content, requires a delicate balance of technical knowledge and a keen understanding of human auditory perception, to help reduce the possibility of mondegreens altering the intended messages in our audio worlds.
Mondegreens in Audio Production How Misheard Lyrics Shape Voice Recognition Technology - Voice Recognition AI Training With Common Song Lyric Mix-ups
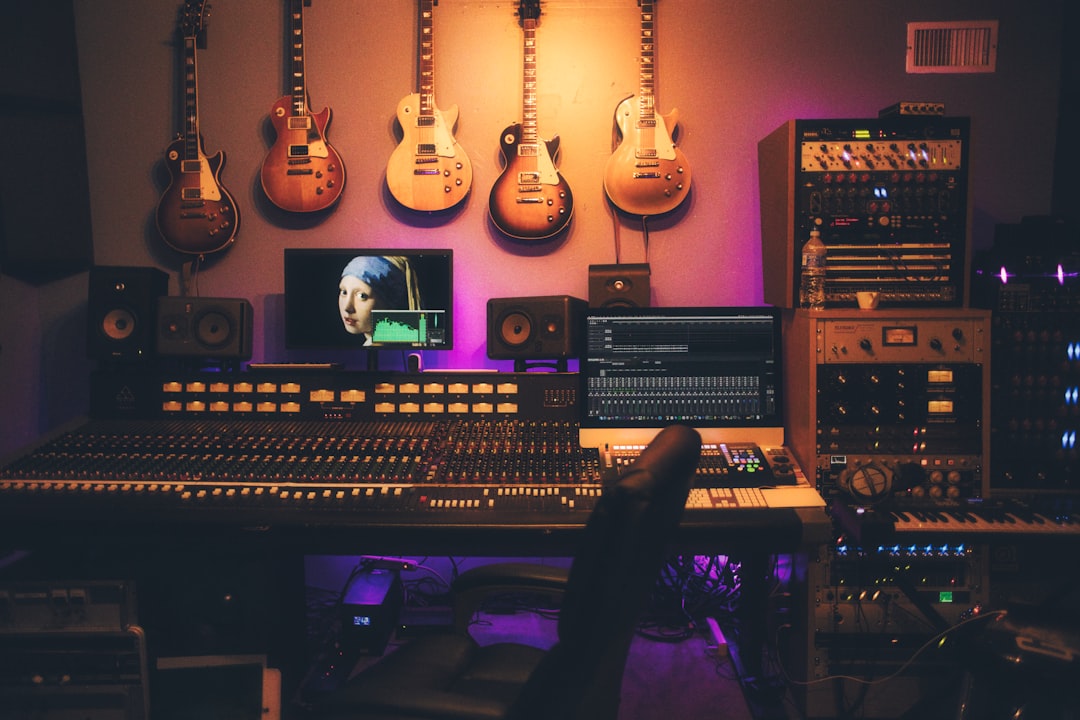
Voice recognition AI systems are constantly learning, and a big part of that learning process involves dealing with how people often mishear song lyrics, a phenomenon known as mondegreens. These misheard lyrics pose a significant challenge to AI, making it difficult for the technology to accurately transcribe songs. This is due to the fact that music presents a complex audio environment with elements like varied tempos, unique vocal styles, and the layering of instruments, all of which can influence how the human ear interprets the words being sung. While AI has made strides with deep learning techniques to improve the accuracy of speech transcription in general, music remains a tricky domain.
Voice recognition systems still find it tough to accurately capture the subtleties in a vocal performance, especially when it comes to discerning the intended lyrics. These issues highlight a key area of research within voice AI, as the ability to understand and transcribe song lyrics with accuracy is critical for diverse applications. As technologies like voice cloning become more sophisticated, accurately understanding the nuances of spoken language, including mispronounced words and regional accents, becomes even more crucial. Ultimately, these challenges are intertwined with the human experience of listening to music, and researchers continue to refine algorithms that leverage a better understanding of auditory perception to deliver improved results in music, podcasting, audiobook narration, and voice cloning technology. Developing AI that can truly mimic the complexities of human speech requires addressing the limitations posed by mondegreens and enhancing the ability to discern intended meaning amidst the richness and intricacy of spoken audio.
The quirks of human auditory perception, particularly the phenomenon of mondegreens, present fascinating challenges for voice recognition AI training, especially when dealing with song lyrics. Many misheard lyrics stem from the fact that our brains are adept at recognizing patterns in sound, sometimes leading us to hear similar-sounding words or phrases even when they're not there. For example, words with comparable vowel structures can lead to drastically different lyrical interpretations, emphasizing the role of pattern recognition in our hearing process.
Beyond phonetic similarities, our cognitive expectations play a crucial part. We tend to anticipate lyrical content based on familiarity and thematic context, sometimes subconsciously filling in gaps with what we think *should* be there. This tendency can lead us astray, resulting in hearing words that aren't actually present in the audio.
Audio mixing also introduces complexity. The frequencies of different instruments or sounds can mask other audio, leading to certain words being masked or misheard. This effect is particularly noticeable in tracks with multiple vocal layers, where certain words or phrases become obscured by the sonic landscape. Regional accents and dialects further muddy the waters, as lyrics interpreted through one accent can sound vastly different through another.
Furthermore, the emotional context in which a song is performed can greatly influence how we perceive the lyrics. If the vocal delivery creates a mood that clashes with the intended lyrical meaning, listeners may interpret the lyrics through the lens of the vocal performance rather than their literal wording, leading to unintended interpretations.
In the pursuit of a clearer audio experience, engineers use tools like spectral analysis to visualize the frequency content and highlight which parts of the audio hold critical linguistic cues. This can help pinpoint problematic areas prone to misinterpretation and lead to better mix decisions. Additionally, the recording environment itself plays a part. Even low-level background noise can introduce small distortions that become more impactful at the listener's end, affecting how lyrics are perceived in the mix.
Voice cloning technologies present a unique set of challenges in this realm. While promising in their ability to recreate human voices, they are still prone to difficulties understanding context within audio recordings. As such, they can end up replicating misinterpretations from the original audio source, carrying these errors forward in their output.
Once a mondegreen takes root, it can be challenging to correct. Listeners may cling to the misheard lyric even when presented with the correct one, highlighting the stubborn nature of cognitive dissonance within our auditory experiences. However, this persistence of misinterpretations also offers a pathway for improving AI systems. By incorporating a deliberate study of common mondegreens into training datasets, AI models can become better equipped to recognize and process the variations inherent in human speech, ultimately achieving a more robust understanding of spoken language in various contexts. This continuous process of refinement based on human errors will be essential in continuing to improve voice recognition systems to the point where they can nearly mirror human comprehension of language in audio.
Mondegreens in Audio Production How Misheard Lyrics Shape Voice Recognition Technology - Audio Engineers Share Their Most Notable Mondegreen Moments of 2024
Throughout 2024, audio engineers have observed a continued trend of listeners mishearing song lyrics, a phenomenon known as mondegreens. Popular artists like Lady Gaga, Taylor Swift, and Kelly Clarkson were among those whose songs were most frequently misinterpreted, underscoring the ongoing challenge of vocal clarity in music production. These misheard lyrics, while often amusing, highlight how complex human auditory perception can be, particularly when dealing with the intricacies of musical arrangements and vocal styles.
The audio industry remains focused on tackling the challenges posed by these misinterpretations, working to enhance mixing techniques and improve the overall quality of audio experiences. As voice recognition and AI-powered technologies continue to advance, understanding how listeners commonly mishear lyrics is crucial for further refinement. The ability of AI to accurately transcribe music and understand the nuances of human speech, including regional accents and variations in vocal delivery, is becoming increasingly critical, especially within the field of voice cloning.
The pursuit of better voice recognition technology requires a deeper understanding of the human experience of listening to audio. By studying these common mistakes and incorporating this data into the development of AI, engineers are striving to minimize the discrepancies between intended meanings and perceived interpretations. Mondegreens serve as a reminder of the delicate balance needed in audio production—balancing technical precision with an acute understanding of how humans hear and interpret the sounds around them. This delicate dance between technology and human perception will continue to shape the future of voice cloning, audiobook creation, podcast production, and more.
Audio engineers in 2024 are increasingly encountering the phenomenon of "mondegreens"—misheard lyrics—highlighting the intricacies of human auditory perception within sound production. These misheard lyrics stem from a variety of factors, posing challenges for both audio engineers and the development of voice recognition technology.
One common culprit is the existence of similar-sounding words (phonemic similarities). Our brains, naturally adept at pattern recognition, can sometimes latch onto auditory patterns that lead to misinterpretations, emphasizing the need for vocal clarity during recording and mixing.
Another challenge is the masking effect in audio mixes, where certain frequencies obscure other sonic elements, including vocal clarity. To counter this, engineers rely on techniques like equalization (EQ) to enhance critical frequencies within the vocal range, ensuring the intended lyrics are easier to perceive amidst a dense sonic environment.
The rise of voice recognition AI is further impacted by these misheard lyrics. These systems are trained on massive datasets, but those datasets often contain mondegreens, making it a challenge for the AI to differentiate between actual lyrics and listener interpretations. Researchers are working to improve this situation, developing algorithms that account for the diverse ways humans interpret sound.
Additionally, our cognitive processes often play a role. The brain anticipates and fills in gaps based on context, leading to "cognitive fillers" that can introduce errors in lyric comprehension. This highlights the importance of vocalists enunciating clearly during recordings, minimizing the potential for misunderstandings.
Regional dialects and accents are also critical factors, influencing how people interpret lyrics. This underscores the need for audio producers to consider the intended listener audience when creating audio content to avoid mondegreens stemming from accent-related variations in pronunciation.
Furthermore, the emotional delivery of a vocal performance can significantly shape how lyrics are perceived. If the vocal delivery contradicts the lyrical message, it can lead to a distorted interpretation of the lyrics. This reinforces the importance of vocalists aligning their performance with the song's intended mood and meaning.
To mitigate these issues, engineers are increasingly utilizing spectral analysis tools, visually representing the frequency content of audio and pinpointing potential areas for misinterpretation within vocal tracks. This visualization aids in making informed mixing decisions that prioritize vocal clarity and reduce mondegreens.
Even with advances in voice cloning, these misheard lyrics can be a problem. Voice cloning technologies can inadvertently replicate mondegreens present in the original audio source, propagating these misinterpretations into cloned voices.
Mondegreens, once ingrained in a listener's mind, can be remarkably persistent. This stubbornness highlights the role of cognitive dissonance, where listeners may resist accepting the correct lyrics even after being made aware of them.
Finally, multi-layered vocal tracks present unique challenges, as some phrases can get masked within a complex mix. Engineers must carefully balance these layers and utilize techniques that maintain lyrical clarity to prevent listeners from mishearing intended lyrics.
In summary, the challenges posed by mondegreens necessitate a keen awareness of the complexities of human auditory perception within audio production. By understanding these challenges, engineers can utilize appropriate techniques to improve vocal clarity and minimize misinterpretations, contributing to a more robust and effective audio experience for listeners across music, podcasts, audiobooks, and other audio formats, including the developing field of voice cloning technology.
Mondegreens in Audio Production How Misheard Lyrics Shape Voice Recognition Technology - Pattern Recognition in Podcast Production The Role of Natural Speech Flow
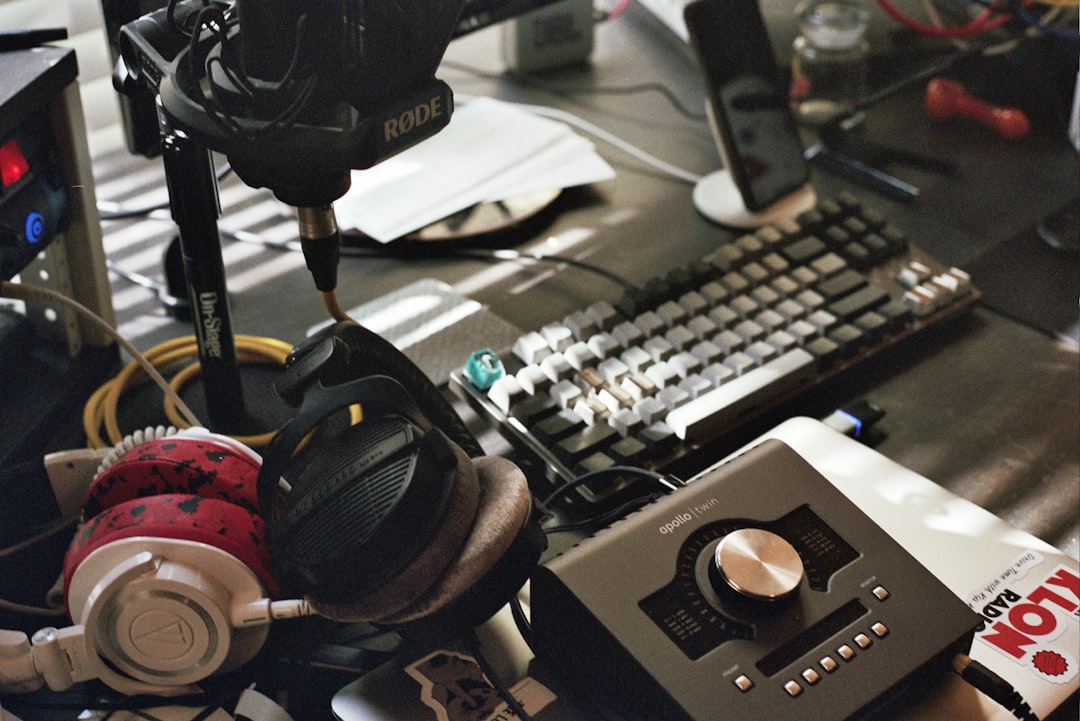
Podcast production hinges on the natural flow of speech, which profoundly influences how listeners perceive and process information. Pattern recognition, both in the human brain and within the algorithms of artificial intelligence, is fundamental to how we decipher spoken words. When podcasters master the subtleties of natural speech—like the rhythm, pitch, and speed of a speaker's delivery—listeners experience greater clarity and engagement. However, this intricate interplay can lead to problems, such as "mondegreens," where listeners misinterpret words or phrases, potentially distorting the intended meaning.
As technologies like voice cloning refine their ability to mimic human speech, a deeper awareness of the patterns and nuances of natural language becomes critical. The capacity to capture and replicate these elements faithfully is crucial for podcast production and other applications where accurate speech interpretation is paramount. Understanding how listeners process speech, and the challenges that can occur, can help ensure the success of various audio formats, from podcasts and audiobooks to the newer, evolving field of voice cloning. The future of successful audio production is intrinsically tied to our understanding and implementation of speech's natural rhythms and structures.
In the realm of podcast production and voice-related technologies, the natural flow of speech plays a crucial role in listener comprehension. A smooth and natural delivery generally leads to better understanding than a more rigid or overly stylized vocal presentation. This insight is particularly important when considering how vocal style choices affect audience engagement and comprehension in podcasts.
Voice cloning, a rapidly evolving field, faces challenges in replicating the intricacies of human speech, specifically with regards to phonetic similarities. When similar-sounding words occur, the cloning technology might misinterpret the intended word, potentially leading to inaccuracies in the synthesized voice. Audio engineers need to meticulously scrutinize the speech patterns in the source audio to prevent the propagation of these errors into the cloned voice.
Humans naturally employ cognitive shortcuts when processing audio, often relying on prior experiences to fill in gaps or anticipate what they are about to hear. This inherent bias can create situations where listeners misinterpret lyrics or spoken words, a phenomenon termed "mondegreens." This tendency creates challenges for AI development, as the training datasets need to represent these common misinterpretations to achieve greater accuracy in voice recognition.
The acoustic characteristics of a recording environment have a noticeable impact on speech intelligibility. Poor acoustics can mask critical speech details, leading to misinterpretations. Therefore, engineers must pay close attention to the acoustics during podcast recording, taking steps to address any issues that might obscure speech.
Regional accents and dialects can significantly impact how listeners understand spoken content. This necessitates careful consideration during podcast recording and production to ensure clarity for a broad audience. Engineers might need to use techniques to emphasize certain phonetic aspects or make adjustments to improve intelligibility.
Modern voice recognition systems frequently leverage artificial neural networks (ANNs) trained on expansive audio datasets. The phenomenon of mondegreens represents a significant area of research for improving these networks, as their ability to accurately interpret and reproduce spoken language requires understanding and adapting to common auditory errors.
The emotional tone of a voice plays a substantial role in how a listener perceives the spoken message. This is particularly evident in audiobooks, where the emotional connection between the voice actor and the narrative content heavily influences a listener's comprehension of the story.
Spectral analysis has become a vital tool for audio engineers who aim to ensure the clarity of audio content. By visualizing the frequency components of an audio recording, engineers can readily identify frequencies that might be masking or obscuring vocals. This allows them to make informed decisions about equalization or other mixing techniques to enhance the intelligibility of spoken words.
Mondegreens, once they become entrenched in a listener's mind, can be incredibly stubborn and resistant to correction. Listeners may cling to the incorrect interpretation, even when confronted with the accurate version. This psychological phenomenon of cognitive dissonance is a significant hurdle for voice cloning technology, which might end up propagating these errors.
The complexity of multi-layered vocal tracks adds another layer of challenge for audio engineers. When multiple vocal or instrumental layers are present in an audio recording, there is an increased likelihood that some parts of the vocals might become obscured, creating opportunities for mondegreens. Engineers need to strategically manage the balance of these layers to prioritize vocal clarity.
In conclusion, the presence of mondegreens reveals the intricacies of human auditory perception in audio production and presents significant challenges for voice recognition and cloning technology. By understanding these challenges and applying suitable recording and mixing techniques, audio engineers can strive to enhance vocal clarity and mitigate potential listener misinterpretations, contributing to more effective and impactful audio experiences in various formats, including podcasts, audiobooks, and voice cloning.
Mondegreens in Audio Production How Misheard Lyrics Shape Voice Recognition Technology - Audiobook Narration Techniques That Minimize Word Boundary Confusion
In the realm of audiobook production, ensuring listeners don't misinterpret words due to unclear pronunciations is paramount. Narrators need to be mindful of how they pace their delivery, how they shift their tone of voice (intonation), and how precisely they pronounce each word. This meticulous approach to word articulation helps ensure the listener gets the intended meaning of the story. The quality of the recording is also a critical factor. A quiet recording space free of distractions and good recording equipment are needed to capture clear audio. Additionally, effectively conveying the various characters within a story can be complex, and it relies on the narrator's ability to subtly shift their vocal style, tone, and even accent to create distinct and memorable characters. By thoughtfully integrating these strategies, audiobook producers can significantly minimize potential confusion stemming from poorly articulated words and improve the overall listener experience, ensuring the story's message is communicated effectively.
Audiobooks, podcasts, and even voice cloning hinge on the clarity of spoken language. However, the way our brains process sound can lead to misinterpretations, especially at word boundaries, a phenomenon that's a challenge for both narrators and AI systems. To combat this, audiobook narrators have developed a range of techniques to make their words crystal clear.
One strategy involves emphasizing certain sounds. By slightly exaggerating vowel sounds and making consonants crisp and distinct, narrators can help listeners distinguish between similar-sounding words. This is particularly useful when multiple words share a similar beginning or ending sound. They might also adjust the pace of their delivery, slowing down at crucial points in a sentence or phrase to give listeners a moment to process the information. This is especially important in narratives where complex ideas or intricate details are being conveyed.
Further enhancing comprehension is the conscious manipulation of pitch and intonation to differentiate between words that sound alike. A skilled narrator will use these tools to emphasize the intended meaning of the words, minimizing any ambiguity that can lead to misinterpretations.
Beyond the narrator's skill, the technical aspects of the recording process also play a vital role. The use of highly directional microphones, for instance, isolates the narrator's voice and minimizes distracting background noise. Proper positioning is paramount as even minor background sounds can cloud a listener's perception. And in scenarios where multiple vocal tracks are layered, like with music or sound effects, recording engineers often employ "vocal stacking" to help separate these elements in the mix.
Managing the dynamics of the recording is also important. Using dynamic range compression, engineers can ensure that the quieter portions of the narration aren't overwhelmed by the louder sections, maintaining a consistently clear audio level throughout. And, just as important is the recording space itself. Sound engineers strive to create spaces with balanced acoustics to help prevent sound waves from interfering with the clarity of the narration. This is where elements like sound-absorbing materials come into play, ensuring a clean soundstage for recording.
Another tool in the engineer's arsenal is spectral analysis. This technique allows engineers to see the frequency makeup of the audio signal, enabling them to spot areas where frequencies might be masking the desired elements, like the narrator's voice. It's like looking at the building blocks of sound, revealing areas that can be manipulated during the mixing stage to maximize vocal clarity.
Beyond the technical, even understanding how our own brains work can help in minimizing misinterpretations. Listeners tend to anticipate what they hear, filling in gaps with preconceived notions. By strategically employing repetition and emphasizing specific thematic elements, audiobook producers can help align listener expectations with the intended meaning of the story.
AI is also playing a role in minimizing the issues related to word boundary confusion. Algorithms are being developed to recognize and process common errors that lead to mondegreens. By integrating context-aware systems and analyzing common misheard words, voice cloning technology and speech recognition in general are beginning to show promise in improving accuracy.
In essence, minimizing confusion at word boundaries is a collaborative effort between the narrator, the recording engineer, and increasingly, artificial intelligence. Each contributes to delivering a more robust listening experience that prioritizes clarity and reduces the possibility of those pesky misinterpretations.
Mondegreens in Audio Production How Misheard Lyrics Shape Voice Recognition Technology - Text to Speech Systems and the Challenge of Phonetic Interpretation
Text-to-speech (TTS) systems strive to convert written text into spoken audio, aiming for a natural and understandable output. However, accurately interpreting the phonetic nuances of language remains a challenge. These systems face hurdles in handling various factors, including user phrasing errors, the influence of regional accents, and the intricacies of audio mixing. The result can be misinterpretations, where listeners or AI might perceive the audio differently than intended. The concept of mondegreens, often referencing misheard song lyrics, vividly illustrates how our brain's complex auditory processing can introduce errors in both the creation and comprehension of audio content.
As voice recognition technology evolves, the importance of accurately interpreting phonetic details and considering the context of speech grows. The ability to translate the subtleties of human language, including subtle pronunciations, regional accents, and emotional tone, is vital to enhancing accuracy in voice recognition and synthesis. This is particularly crucial in applications like voice cloning where achieving a truly natural-sounding voice hinges on an advanced understanding of how humans speak. Ultimately, improving the way TTS systems decipher the complexities of speech can significantly improve the user experience across various platforms and applications, especially in areas like voice cloning where clear and accurate communication is critical.
Text-to-speech (TTS) systems, while increasingly sophisticated, still face hurdles in accurately converting text to speech due to the complexities of phonetic interpretation. The challenge often stems from the subtle differences in how humans pronounce words, especially in the presence of background noise or layered audio. For example, words with similar sounds can easily be misconstrued by listeners, especially in musical contexts where multiple instruments and vocal tracks can mask certain frequencies.
Our own cognitive processes further complicate matters. When confronted with unclear or ambiguous audio, our brains frequently fill in the gaps with what we anticipate or expect to hear, leading to "cognitive fillers" that can result in mondegreens—misheard lyrics or phrases. This tendency to impose meaning onto unclear audio highlights how our perceptual biases can influence how we interpret what we hear.
Furthermore, the recording environment itself can play a significant role. Poor room acoustics can introduce reverberation and echoes, creating a sonic environment where subtle phonetic differences become obscured. This issue is especially relevant in voice cloning, where accurately replicating the nuance of a speaker's voice requires not only capturing their unique pronunciation patterns but also the acoustic characteristics of the original recording space.
Additionally, the natural variability of human speech presents a challenge for machine learning algorithms that power voice recognition systems. Differences in speaking style, pacing, and intonation between individuals can confuse the systems that attempt to decipher and transcribe human speech. The emotional context of speech also plays a part. If the speaker's tone conflicts with the semantic content of their words, listeners can be misled, resulting in misinterpretations.
Voice cloning technology attempts to address these challenges by learning patterns from massive datasets of speech. However, these datasets often include mondegreens, which can lead to inconsistencies in the cloned voice. To address these inconsistencies, researchers are working on refining training data and using techniques like spectral analysis to analyze audio and identify areas where phonetic nuances are obscured or distorted. By visually representing frequencies in the audio, engineers can manipulate elements during mixing to optimize vocal clarity and reduce the potential for misinterpretations.
Furthermore, the prevalence of various dialects and regional accents presents additional complexities. What might be clear to one listener may be unintelligible to another. As a result, developers of voice recognition and TTS systems must consider the diverse linguistic landscapes within their intended audiences when designing and optimizing their technologies.
Despite these ongoing challenges, the continued evolution of machine learning and TTS systems holds great potential for improving the accuracy of synthetic speech. As the algorithms become more sophisticated, and training datasets become more robust and representative of diverse linguistic backgrounds, we can anticipate that voice cloning, TTS, and voice recognition will overcome many of the hurdles posed by the intricate and varied nature of human speech.
Get amazing AI audio voiceovers made for long-form content such as podcasts, presentations and social media. (Get started for free)
More Posts from clonemyvoice.io: