How Voice Cloning Technology Adapts to Regional American Accents A Technical Deep-Dive
How Voice Cloning Technology Adapts to Regional American Accents A Technical Deep-Dive - Machine Learning Adapts to Southern Drawl Using 500 Hours of Mississippi Audio Samples
Machine learning algorithms are being refined to capture and reproduce the nuances of regional accents, with a particular focus on the Southern drawl. Researchers have leveraged a vast audio dataset—500 hours of recordings from Mississippi—to train these algorithms. This ambitious undertaking demonstrates the growing ability of artificial intelligence to generate synthetic speech that is not only understandable but also captures the distinctive characteristics of a specific dialect. By leveraging deep learning techniques, these technologies aim to produce voices that are not merely generic but possess a strong connection to their regional origins. This advancement, however, underscores the rising concern surrounding potential misuse of this technology. The possibility of voice impersonation and the ethical implications of creating AI-generated speech demand close attention. As voice cloning progresses, it's critical to address these concerns and ensure responsible application of these innovations in various domains like audio book creation, podcasting, and voice cloning for entertainment and artistic purposes.
Researchers are exploring how machine learning can accurately capture and replicate the nuances of the Southern drawl, specifically focusing on the Mississippi dialect. This endeavor necessitates a large dataset—in this case, 500 hours of audio—to train models that can effectively learn the unique phonetic features of the region. Southern accents present a unique challenge due to their distinct vowel shifts and intonation patterns, deviating from the standard speech patterns often used in machine learning training. It's not just about pronunciation, though; the rhythm and pace of Southern speech add another layer of complexity.
Voice cloning models, while improving, need adaptation for dialects. For example, replicating the drawl might require additional training layers within the neural network architecture, focusing on how the accent impacts vowel sounds. The goal is to reduce common artifacts in synthetic speech, which is why pre-processing these audio samples to capture subtleties in pitch and tone is crucial. Furthermore, simply copying sounds isn't enough. Understanding the sociolinguistic context of the Southern drawl is key, as it influences not only the sounds but also how meaning is conveyed within conversations.
Imagine the potential applications of this research for audiobook production. Imagine a story narrated in a genuine Mississippi accent, increasing relatability for listeners in that region. Similarly, podcasters could benefit from the ability to generate voices in different regional accents to better reach targeted audiences without the need for extensive recordings. However, achieving a truly natural-sounding Southern drawl requires careful consideration of phonetic transcriptions to ensure the synthetic voice aligns with authentic regional speech patterns.
This work emphasizes the continuous interaction between technological advances in machine learning and the complexities of human language, especially in localized dialects. The challenge is to design systems that can capture these nuances effectively while upholding ethical considerations around authenticity and misuse of voice cloning technology. We need to strike a balance between pushing the boundaries of artificial speech and respecting the intricate tapestry of human language and its diverse regional expressions.
How Voice Cloning Technology Adapts to Regional American Accents A Technical Deep-Dive - Breaking Down Voice Pattern Recognition in New England Dialects
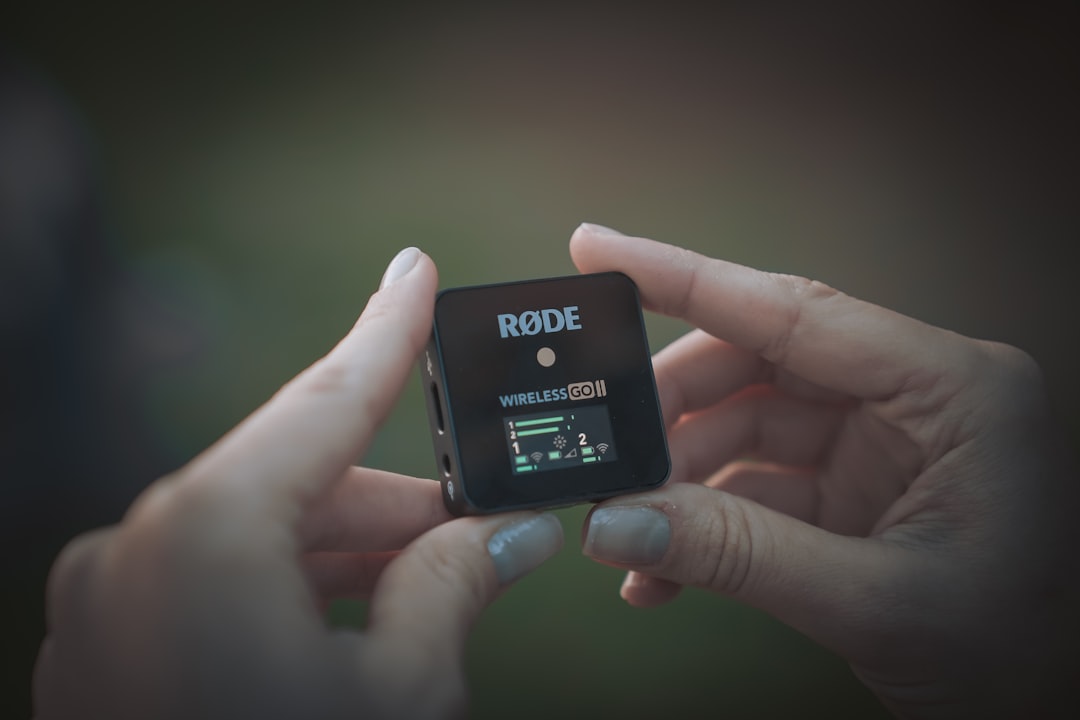
Understanding how voice pattern recognition tackles New England dialects reveals a fascinating and complex landscape. New England speech, historically known for features like "R-dropping," actually encompasses a variety of accents across Eastern and Western regions. These dialects, shaped by historical settlement patterns, present unique challenges for voice cloning technology. Researchers are making headway in this area by meticulously documenting these soundscapes through field recordings and statistical modeling. This includes linking dialectal features to factors like age, gender, and social background. Interestingly, studies suggest that certain hallmark features of the "Yankee" dialect, particularly R-dropping, are becoming less prevalent among younger generations. This constant evolution underscores the need for voice cloning technologies to remain adaptable, if they are to effectively mimic the true sound of these ever-changing dialects. While the progress in capturing these specific acoustic features is promising, ethical considerations about the potential misuse of such technology for impersonation or other nefarious purposes cannot be ignored. The path forward requires a careful balance between pushing the boundaries of technological capabilities and respecting the intricate and diverse fabric of spoken language in New England.
Analyzing New England dialects presents a unique challenge for voice cloning technologies. The distinctive phonetic features, like the prominent "r-dropping" associated with the historical "Yankee" dialect, require specialized training data and algorithms to replicate accurately. While the "r-dropping" feature is a hallmark of Eastern New England speech, it's important to note that younger generations are adopting the standard "r" pronunciation more frequently. This generational shift highlights the dynamic nature of dialect evolution, demanding a constant refinement of the voice cloning models to stay current with changing speech patterns.
Furthermore, the intonation patterns of New England speech, characterized by rising pitch at the end of sentences, pose a challenge for standard machine learning models. These models might struggle to accurately reproduce the melodic contours of the accent, resulting in artificial-sounding speech. The diverse linguistic influences, including indigenous languages and immigrant communities, contribute to the region's complex dialectal landscape. Voice cloning models need to be trained on data that incorporates this diversity to achieve a faithful representation of New England speech.
Currently, the datasets used to train voice cloning models for New England dialects are smaller compared to the resources available for accents like the Southern drawl. This smaller dataset limits the range of expressions and pronunciations that can be captured, resulting in potentially less nuanced and diverse synthetic voices. The process of segmenting phonemes in New England dialects also differs from the standard segmentation used in American English, requiring sophisticated audio processing techniques to capture the unique sonic characteristics of the region.
Beyond just the sounds, understanding the context in which words are spoken is critical. Some words within New England dialects can have multiple meanings depending on the subtle nuances of pronunciation, making context-aware modeling crucial. Additionally, capturing the unique emotional inflections of New England speech is essential. Simply replicating the sounds without understanding the emotional context can lead to a flat and unnatural-sounding synthetic voice. Moreover, it is crucial to recognize the sociolinguistic context of these dialects, including regional identity and perceptions, to ensure the cloned voices do not sound artificial or insensitive.
We see that dialect change is a continuous process, and voice cloning models for New England English must constantly adapt. The changes over time are fueled by cultural interactions, ultimately necessitating frequent retraining of the AI to maintain accuracy. Lastly, researchers are still focused on perfecting the art of voice cloning to reduce artificial-sounding speech. This involves advanced filtering techniques to eliminate any artifacts or irregularities that would make the synthetic voice sound unnatural or misrepresent the subtle features of regional speech patterns.
This research ultimately provides a pathway to preserving regional linguistic diversity and demonstrating how technology can be used to study language. Yet, we must be mindful of potential ethical concerns surrounding the responsible development and application of voice cloning technology for preserving and understanding human communication in its many beautiful, diverse forms.
How Voice Cloning Technology Adapts to Regional American Accents A Technical Deep-Dive - California Valley Girl to Midwest Transformation Through Neural Networks
The ability to shift a voice from a California Valley Girl accent to a Midwestern one using neural networks showcases the evolving capabilities of voice cloning technology. Deep learning methods, combined with voice style transfer, allow for the creation of highly personalized synthetic voices using just a small amount of audio samples. This process is quite complex, involving the intricate mapping of acoustic features like intonation and pitch, as well as the often subtle social and contextual cues embedded in speech. The goal is to generate a synthetic voice that not only sounds authentic to a particular accent but also conveys the emotional depth present in human speech. As this technology matures, researchers must consider the ethical ramifications of voice cloning, such as potential misuse for impersonation and the need to respect the cultural weight carried by regional dialects. Voice cloning offers creative potential for things like audiobook narration and podcasting but also reminds us of the profound connection between language and individual or group identities.
The shift from a California Valley Girl accent to a Midwestern one presents a fascinating challenge for voice cloning, requiring a deep understanding of the distinct phonetic features that separate these accents. For example, the vowel sounds in words like "cat" can sound significantly different. A Valley Girl's pronunciation might lean towards a more forward 'a' sound, while a Midwesterner typically uses a flatter sound.
Regional accents impact intonation patterns, which are crucial elements in training voice cloning systems. A characteristic Valley Girl might often use a rising intonation at the end of sentences, creating a questioning tone. Midwestern speech tends to have a more monotonous intonation, influencing how a synthesized voice is ultimately perceived.
One exciting possibility is using voice cloning within audiobook productions. By employing voice models specifically trained on regional dialects, audiobook creators can create narrations that resonate more authentically with listeners' cultural backgrounds. This increased relatability could lead to a more engaging and immersive listening experience.
To transition a voice from one accent to another, neural networks need specialized training layers dedicated to parsing out phonetic features from various regions. This adds considerable complexity to the design process, and developing models capable of generating convincingly accurate target accents requires substantial computational resources.
Neural network techniques like acoustic attention mechanisms prove essential for refining synthesized voices. These mechanisms enable the model to focus on the most relevant sound features, improving the accuracy of regional accent replication. This helps capture subtle nuances that standard training might miss.
Subtle aspects of speech like pace and rhythm are highly variable between accents but often receive insufficient attention in basic voice cloning algorithms. For instance, a Midwestern accent might display a more even pacing compared to the more rhythmically diverse Valley Girl accent.
Preparing audio samples for training requires meticulous effort to isolate and eliminate background noise that could skew the accent features. To produce truly accurate voice cloning models, high-quality datasets are essential, demanding careful control over the recording environment and conditions.
Recent improvements in voice conversion allow real-time accent transformations, holding great promise for communication tools. This has the potential to revolutionize how people communicate by bridging regional barriers and fostering smoother conversations across different dialects.
The ethical dimensions of voice cloning become particularly acute when dealing with accents. Misrepresenting an accent can lead to misunderstandings and reinforce harmful stereotypes. Accurately representing accents is not only important for the quality of the technology, but also for respecting the cultural identity of each regional speech community.
Finally, leveraging gradient-based optimization techniques allows for fine-tuning voice cloning programs to closely adhere to the specific acoustic features of a Midwestern accent. This kind of meticulous adjustment is necessary to minimize the typical artifacts present in synthetic voices. The ultimate goal is to achieve a more natural sound that listeners find engaging and acceptable.
How Voice Cloning Technology Adapts to Regional American Accents A Technical Deep-Dive - Voice Cloning Tackles Complex Brooklyn and Bronx Accent Patterns
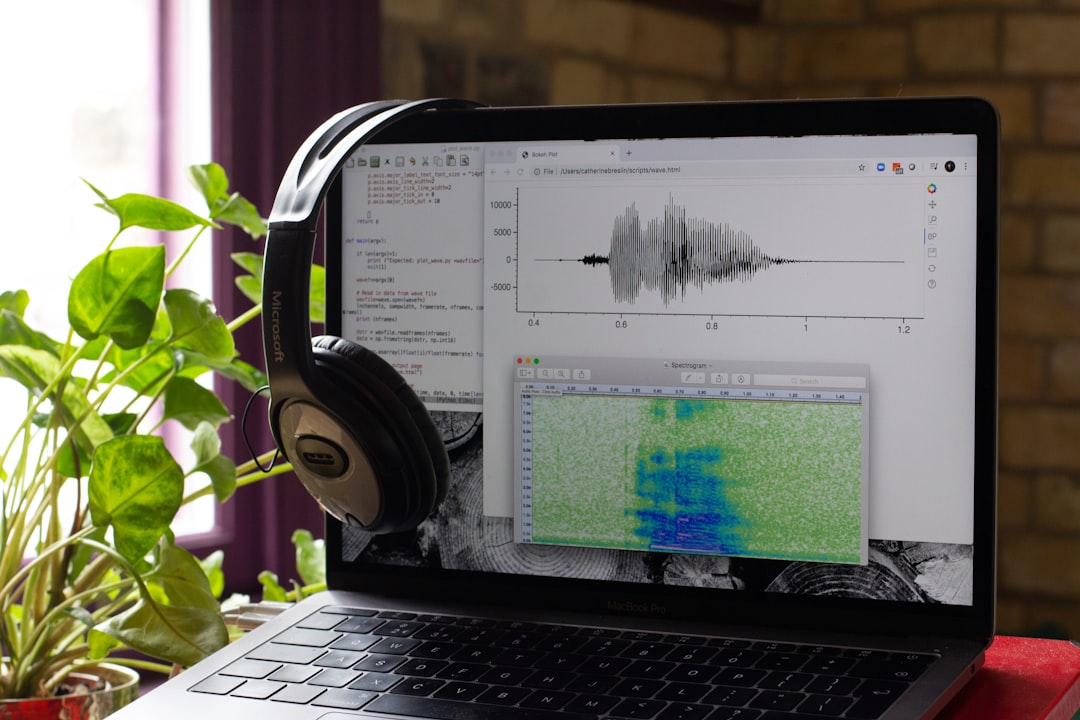
Voice cloning technology is demonstrating its capability to accurately replicate the complex sound patterns of accents, specifically those found in the Brooklyn and Bronx areas. Machine learning models are trained using large amounts of audio data representative of these regions. The goal is to capture the unique phonetic characteristics and intonation patterns that distinguish these urban accents. This is no small feat, as human speech is incredibly nuanced. Achieving a genuinely authentic sound necessitates continuous refinements to these models. The challenges include not only accurately representing the sounds but also navigating the potential ethical issues that arise when such powerful technology is used to mimic human voices. There are concerns about misuse, and the need to respect regional identities is paramount. Despite these complexities, voice cloning holds promise in fields such as audiobook creation, podcast production, and entertainment. As this technology advances, it's crucial to strike a balance between innovation and responsible use to ensure that the technology benefits society while protecting individual and community identities.
Voice cloning technology is facing a new set of challenges when it comes to capturing the intricacies of accents found in neighborhoods like Brooklyn and the Bronx. The unique phonetic nuances of these dialects, characterized by specific vowel shifts and the frequent use of nasal sounds, require advanced machine learning techniques. Specifically, neural networks are being augmented with specialized layers to adapt to these complex sound patterns, which is a challenging endeavor in acoustic modeling.
Beyond the core phonetic features, there's a growing interest in analyzing vocal tremor variations that exist in these accents. Subtle tremors can subtly convey emotional depth, adding a layer of authenticity to synthetic speech that standard models often fail to replicate. However, a key hurdle is the strong cultural and social connection woven into these dialects. Simply capturing audio samples isn't enough for truly authentic representation. Voice cloning needs to account for the intricate way that language and social identity intertwine in these urban areas, often expressed through specific patterns of speech that are hard to pick up through basic recording methods.
Researchers have noted that these accents tend to have a high density of sounds, squeezing more vowels and consonants into a given phrase compared to standard American English. This density requires much larger and more robust datasets for training, leading to potential efficiency issues within the models themselves. Also, the rhythm and tempo of speech varies significantly between Brooklyn and the Bronx dialects. Replicating these distinct pacing patterns is crucial for preventing the synthetic voice from sounding robotic or unnatural.
The rise of voice cloning has spurred concerns about authenticity and cultural representation. Misrepresenting these accents could potentially reinforce negative stereotypes or lead to cultural insensitivity. Researchers recognize the importance of carefully curating training data and designing models that respect the unique linguistic and social identity associated with these accents.
However, the scarcity of publicly available audio data related to these accents is a major roadblock. Compared to more widely studied accents, the available data is limited, potentially hindering the ability of voice cloning systems to accurately represent the full diversity of speakers using these accents.
Another layer of complexity is how the meaning of certain words can change depending on the nuanced pronunciation and intonation used in these dialects. This necessitates the integration of reinforcement learning within the voice cloning models, allowing them to adapt to contextual modulations that dictate these meaning shifts.
Furthermore, the voice cloning process itself can introduce artificial-sounding artifacts, which can be particularly problematic when dealing with the subtle nuances of regional speech. Advanced filtering techniques are essential to clean up the output and prevent the synthesized voice from sounding artificial or failing to faithfully capture the characteristics of the dialect.
The acoustic conditions found in these urban environments can also affect how accents are recorded, introducing factors like background noise and ambient sounds that voice cloning systems must consider during training to avoid inaccurate outputs. Successfully adapting to these acoustic factors is crucial for ensuring that the resulting synthetic voices resemble real-life speech interactions in these dynamic settings.
The continuous refinement of voice cloning technology has the potential to offer fascinating new tools for research, entertainment, and education. Yet, the challenge of capturing and representing complex accents like those of Brooklyn and the Bronx emphasizes the need for a delicate balance between innovation and responsibility. We must ensure that technological advancements do not come at the cost of misrepresentation or cultural insensitivity in the quest to perfect voice cloning.
How Voice Cloning Technology Adapts to Regional American Accents A Technical Deep-Dive - Teaching AI to Recognize and Replicate Texas Speech Markers
Teaching AI to recognize and replicate the distinct speech markers found in Texas presents a unique challenge within voice cloning. Texas's diverse linguistic landscape, shaped by its history and cultural influences, results in a wide range of accents and speech patterns. To successfully capture these nuances, voice cloning models need access to substantial high-quality audio data specifically representative of Texas speech. This includes mapping the unique phonetic features, such as vowel sounds and intonation patterns, that define the Texan dialect. However, it's not just about the sounds themselves; understanding how Texan speech conveys meaning and emotion within a social context is equally crucial, as it often reflects a strong sense of regional identity. Researchers face a balancing act in this area, needing to explore the innovative potential of voice cloning while being acutely aware of the ethical implications. They must ensure that any technology developed not only accurately reflects the sonic characteristics of Texan speech but also avoids any potential misuse or misrepresentation of the voices and dialects it seeks to replicate.
Teaching AI to recognize and replicate Texas speech markers is a complex endeavor due to the unique characteristics of Texan accents. The blend of English, Spanish, and indigenous language influences creates a distinctive phonetic landscape, where certain vowel sounds merge, leading to challenges for AI models in differentiating sounds like "pen" and "pin." This merging can cause ambiguity in speech recognition, requiring sophisticated algorithms to correctly capture these regional pronunciations.
Furthermore, the melodic rise in intonation common at the end of Texan statements presents a unique obstacle for synthesizing natural-sounding dialogue. Voice cloning systems must be trained to accurately capture this melodic quality if they are to sound authentic. It's important to consider that Texan speech patterns vary greatly across different social and ethnic groups, reflecting cultural identity. AI models need to be sensitive to these differences to avoid overly simplified representations that overlook the diverse range of speech within Texan communities.
Gathering the necessary audio data for Texas accents poses a substantial hurdle. The vast and diverse landscape, coupled with a large and varied population, creates the need for extensive and representative audio corpuses for effective model training. The computational cost of this endeavor is significant, demanding substantial resources to handle the vast amounts of data needed to capture the nuances of Texan speech. Moreover, the context in which words are spoken can heavily influence meaning in the Texan vernacular. This means AI systems must be trained to understand not just individual words, but the intricate interplay of language and context to accurately synthesize nuanced dialogue, rather than merely mimicking sounds.
The spontaneous and casual nature of Texan conversations, often filled with pauses and filler words, adds another dimension of difficulty for voice cloning systems. Achieving fluidity and authenticity in synthesized speech requires capturing this inherent spontaneity. Additionally, the distinctive Texan lexicon, filled with colloquialisms that might not be found in standard English datasets, needs to be integrated into training resources. Specialized vocabularies are crucial for enhancing accuracy and facilitating better model training.
Importantly, it's crucial to remember that Texan accents are not static. They are in a constant state of flux, influenced by shifting demographics and evolving cultural interactions. This dynamism requires that AI models be continually retrained to remain relevant and accurate for voice cloning applications. The acoustic environments across the state also introduce complexity. Texas features both urban and rural settings, each contributing distinct acoustic characteristics to speech. This variability can make audio sample collection challenging, as different environments introduce background noise or alter speech recording conditions, impacting data quality and training efficacy for voice cloning systems.
Overall, the research challenges presented by Texan speech are complex, requiring a nuanced approach that combines phonetic analysis, intonation studies, and social and cultural awareness. If achieved, this research could create innovative voice cloning applications for entertainment, audiobook creation, and other purposes, while simultaneously providing new avenues for exploring the intricate relationship between technology, language, and regional identity.
How Voice Cloning Technology Adapts to Regional American Accents A Technical Deep-Dive - How Hawaii Pidgin Challenges Current Voice Synthesis Models
Hawaii Pidgin poses a unique challenge for existing voice synthesis models due to its distinct sound patterns, rhythm, and cultural context. Unlike more standardized American English accents, Pidgin is closely connected to Hawaiian culture and blends elements of various languages, resulting in a complex linguistic structure that's difficult to replicate accurately. Voice cloning tools need specialized training datasets that capture the wide range of speech patterns and intonational variations specific to Pidgin. Currently, the majority of voice cloning systems are optimized for common English accents, which may struggle to capture the richness and emotional expression that are characteristic of Pidgin. This suggests a need for ongoing advancements in adaptable voice synthesis technologies. Moving forward, the field needs to consider not only the technical aspects of achieving accurate replication but also the ethical implications of cultural representation and authentic expression.
Hawaii Pidgin, also known as Hawaiian Creole English, presents a unique linguistic landscape that poses considerable challenges for current voice synthesis models. It's a fascinating blend of English with influences from various local and immigrant languages, creating a distinct phonetic system that deviates significantly from standard American English. The rhythm and structure of the language differ, making it difficult for AI systems to learn and reproduce accurately.
One of the key difficulties lies in the variations in vowel sounds. Certain diphthongs, common in standard English, are often merged or simplified in Hawaii Pidgin. This can cause confusion for voice cloning models trained primarily on standard dialects, leading to inaccuracies when attempting to generate truly authentic-sounding speech.
Beyond just the sounds, Hawaii Pidgin relies heavily on pitch variations and expressiveness to convey meaning, often conveying emotional nuances that extend beyond the literal words themselves. This requires advanced algorithms that not only replicate sounds but also understand and interpret the emotional context embedded within the speech. Replicating this is no easy task for current AI models.
Adding another layer of complexity are the numerous local terms and idiomatic expressions unique to Hawaii Pidgin. Synthesized voices need to not only correctly pronounce these but also understand the cultural significance behind them to ensure appropriate contextual usage. Simply mimicking the sounds isn't sufficient for genuine authenticity.
The multicultural environment of Hawaii plays a major role in shaping the language, with Hawaiian, Japanese, Filipino, and other language influences evident in the Pidgin. This requires considerably diverse audio datasets to train voice cloning systems, unlike regions with more homogenous dialectal characteristics. The need for robust and varied training data presents a significant technical obstacle.
Further complicating the matter are the inherent sociolinguistic nuances of Hawaii Pidgin. The language plays a crucial social role, and its usage varies among speakers from different backgrounds. AI models must be designed to not only understand the phonetics but also the social context that shapes the accent and how it signifies identity within the community.
The often lively and dynamic environments of Hawaii, filled with diverse sounds, also impact the quality of audio samples used to train models. Background noise can significantly impact recordings, making it difficult to isolate and capture the distinct acoustic features of the speech. This creates a hurdle when trying to develop accurate AI models.
Current voice synthesis systems struggle to accurately reflect the natural rhythm and pacing of Hawaii Pidgin. The language often features a slower tempo with strategic pauses that are integral to its communication style. Mimicking this in a synthetic voice is challenging, as standard models tend to rush through speech, potentially creating a jarring or unnatural sound.
Within the Hawaiian Creole community, there's a remarkable variety of accents and usage across the islands, requiring very nuanced training datasets to ensure that voice cloning models can adapt to the specific communities they aim to represent. This requires a higher degree of flexibility and adaptability compared to other dialect-focused cloning initiatives.
Finally, ethical concerns surrounding the representation of Hawaii Pidgin in voice cloning technology cannot be ignored. The potential for misrepresentation can reinforce harmful stereotypes and display cultural insensitivity. The responsible development of voice cloning technology in this context must be carefully considered to avoid perpetuating potentially problematic or damaging representations. It's crucial that the technology be developed with careful attention to capturing the nuances of Hawaii Pidgin while avoiding the potential pitfalls of misrepresentation.
More Posts from clonemyvoice.io: