How IPA Phonetic Transcription Enhances Voice Clone Accuracy in 2024
How IPA Phonetic Transcription Enhances Voice Clone Accuracy in 2024 - Real-time IPA Integration with Neural Voice Models
The ability to seamlessly integrate the International Phonetic Alphabet (IPA) with neural voice models in real-time is a game-changer in the world of voice cloning. This direct connection between spoken words and IPA symbols drastically improves the accuracy of phonetic transcription. Think of it as a much more precise blueprint for the sounds we make. With this enhanced precision, we can leverage IPA for creating more nuanced and believable voices in diverse applications.
Audiobooks, podcasts, and even voice cloning for entertainment purposes can benefit immensely. Modern audio processing models, often with a real-time aspect, are allowing for more immediate and adaptive voice applications. It's about making sure the synthetic voice responds more naturally to its environment. As we increasingly rely on voice interactions, getting the sound of artificial voices "right" becomes even more crucial. This new approach using IPA helps to improve the overall experience with voice clones, making them sound more natural and easily understood, bridging the gap between human and computer-generated voices. There's still work to be done, but these improvements will likely refine the experience for creators and users of voice technologies.
OpenAI's recently released GPT-4o Realtime API, accessible to paying developers, presents an interesting opportunity for voice technology. Its ability to handle audio input and provide both text and audio responses makes it potentially valuable for building interactive voice systems. Crucially, this opens doors for real-time integration with the International Phonetic Alphabet (IPA). This is particularly relevant in voice cloning, where IPA's precision can significantly boost accuracy.
Imagine having a system that can instantly adjust a voice model's pronunciation to match different accents and dialects. That's the promise of real-time IPA. This means you wouldn't need extensive retraining for every subtle vocal variation. This type of adaptability could be a game changer in audiobook production, allowing for accurate vocal renditions across a range of characters or even personalized narrations based on user preferences.
However, it's not just about accents. The meticulous nature of IPA transcription, focused on capturing the intricate nuances of speech sounds, can significantly improve overall voice quality. We're talking about minimizing the dreaded "uncanny valley" effect—that eeriness that sometimes plagues synthetic speech. By grounding the voice in the precise mechanics of pronunciation, voice clones can sound more natural and relatable.
Furthermore, the benefits aren't confined to just voice cloning. Think about voice assistants. Current speech recognition often struggles with background noise. But a system that uses IPA in real-time might be able to isolate and prioritize core phonetic cues, improving responsiveness in noisy environments.
Azure's Speech SDK, with its real-time speech-to-text capabilities, provides a good illustration of this potential. But building these kinds of systems requires robust phonetic transcription models. Existing resources like CommonVoice could be leveraged, converting them to IPA prior to model training. While tools are emerging for IPA-based transcription, there's still room for improvement in this area.
Researchers are discovering that neural voice models trained on IPA data demonstrate a remarkable improvement in speech recognition. This is particularly evident with languages that have complex phonetics or limited training datasets. The ability to represent subtle articulation variations with IPA means voice models can capture unique speaker characteristics more accurately than relying on basic waveforms alone. This holds implications for future research into emotion and tone in generated speech.
While still experimental, there are hints that real-time IPA integration could allow for dynamic adjustments to tone and pitch, making synthetic speech even more adaptable and nuanced. Moreover, it has the potential to simplify the creation of multilingual content in areas like podcasting and audiobooks.
The journey towards truly natural and versatile voice technologies is ongoing. However, these ongoing advancements underscore that real-time IPA integration holds the key to unlocking a new era of enhanced accuracy, adaptability, and user experience in voice systems.
How IPA Phonetic Transcription Enhances Voice Clone Accuracy in 2024 - Multilingual Phoneme Detection Through Wavelength Analysis
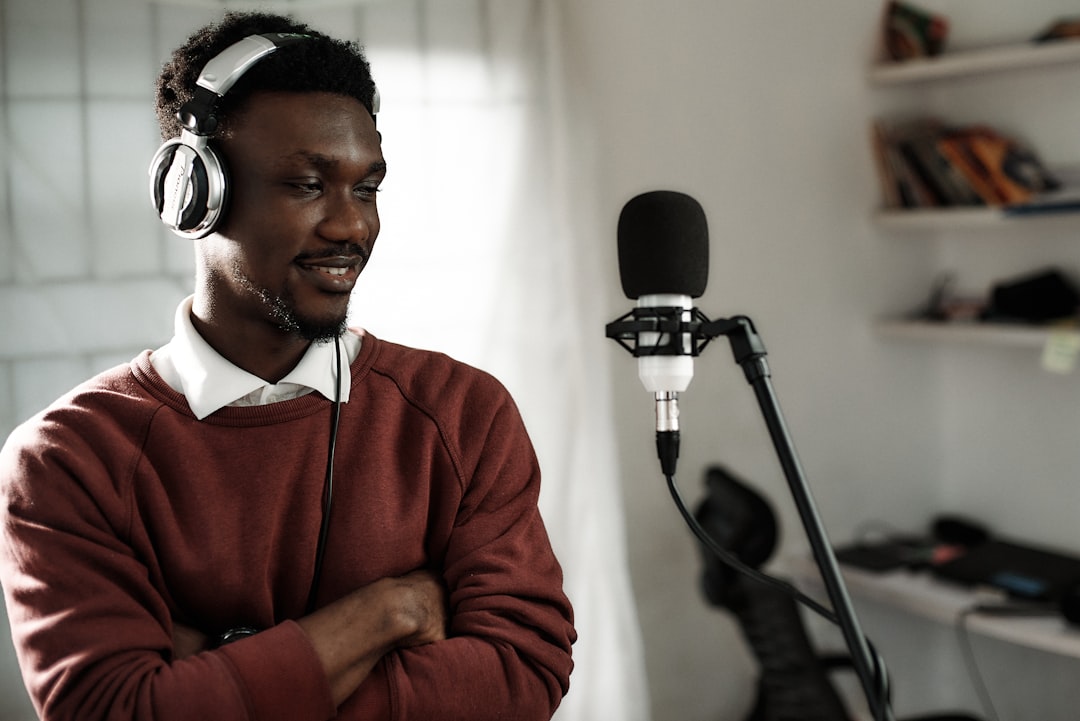
Analyzing sound waves to identify phonemes across multiple languages is a developing area that has important implications for voice technology, particularly in the realm of voice cloning and improving the accuracy of phonetic transcription. By examining the unique frequency patterns of different sounds, researchers are developing ways to automatically detect phonemes in a wide range of languages. This ability is crucial for projects like creating audiobooks or podcasts that feature multiple languages or accents, or for training more accurate voice clones.
Systems like MultIPA, designed to produce phonetic transcriptions across languages, depend on large training datasets and the ability to accurately recognize phonemes. While progress has been made, challenges remain in reliably identifying phonemes in diverse audio environments and handling languages with limited data available for training. Tools like Allosaurus, incorporating phonological knowledge into the analysis, show promise for enhancing the accuracy of these systems.
The ultimate goal is to improve the naturalness and clarity of synthesized speech, bridging the gap between human and artificial voices. While these methods still require refinement, the promise of more accurate multilingual phoneme detection is a significant step forward in crafting synthetic voices that are easier to understand and more enjoyable to listen to. The hope is that in time these technologies will provide better results for creating audio content in diverse environments.
Multilingual phoneme detection through wavelength analysis offers a powerful approach to enhancing voice cloning accuracy. By breaking down sound into its constituent frequencies, we gain a more precise understanding of how phonemes are formed, which is fundamental for creating realistic synthetic voices. This granular approach is especially crucial when dealing with multiple languages, as each language has its own unique set of phonetic features that must be captured accurately.
Interestingly, this method has the potential to significantly reduce the amount of training data needed for voice models. By using pre-existing IPA datasets and leveraging the precision of wavelength analysis, we can translate these phonetic representations into high-quality voice outputs without requiring extensive new recordings for every language or dialect. This is especially relevant for less-common languages with limited readily available data.
Furthermore, this approach can make voice cloning more resilient to noise. By focusing on the core phonetic cues embedded within the sound waves, models trained with this method can filter out background noise more effectively. This ability is invaluable for producing high-quality audio for applications like audiobooks and podcasts, where recordings might occur in less-than-ideal acoustic environments.
Real-time analysis of speech through wavelength detection has another compelling advantage: the ability to dynamically adjust a synthetic voice's pitch and tone. This could pave the way for more interactive voice assistants that adapt to different conversational contexts and user preferences. Imagine a voice assistant that can seamlessly switch between a formal and informal tone based on the nature of the interaction.
Another benefit of this technique is in tackling the “uncanny valley” effect – that unsettling feeling we get when encountering synthetic voices that are almost, but not quite, human-like. Detailed wavelength analysis allows for the capture of more nuanced speech patterns. This leads to a more natural-sounding voice and is particularly crucial in applications like audiobooks, where character voices need to be distinct and believable.
The ability to analyze phonemes through wavelength data can extend beyond individual languages, enabling the development of multilingual voice models. This adaptability is incredibly beneficial in applications like podcasting, where content needs to be delivered in various languages with appropriate accents and intonations.
It's worth noting that wavelength analysis goes beyond simple phoneme identification; it can also capture a wealth of acoustic features, including subtle aspects like emotional expression and emphasis. This rich data extraction is critical for enhancing the emotional expressiveness of synthetic speech, making it more relatable and engaging.
The combination of deep learning with wavelength analysis has yielded promising results in recent research. These hybrid models seem to surpass traditional methods in both accuracy and efficiency, which means more versatile and dynamic speech patterns for voice clones.
The ongoing research in integrating wavelength analysis into voice models holds tremendous promise. We can expect further improvements in speech recognition and synthesis, leading to more adaptive and accurate voice technologies with a wide range of future applications. This is an exciting field with the potential to revolutionize how we interact with computers and how we experience audio content.
How IPA Phonetic Transcription Enhances Voice Clone Accuracy in 2024 - Prosodic Features Enhancement Using IPA Mapping
**Prosodic Features Enhancement Using IPA Mapping**
Leveraging IPA mapping to enhance prosodic features is a key step forward in creating more realistic synthetic voices. Prosody, which encompasses aspects like intonation, stress, and rhythm, is crucial for conveying meaning and emotion in speech. By mapping these features onto the IPA system, we create a detailed phonetic roadmap for voice cloning models. This detailed approach is essential for generating voices that sound natural and expressive, particularly within applications like audiobook narrations or podcast production where conveying emotional nuance is paramount.
While the concept of using IPA for more accurate voice cloning is promising, the process of obtaining detailed prosodic annotations can be complex and time-consuming. Generating these annotations often requires specialized knowledge and substantial effort, making it a potential bottleneck in the development process. Further research into streamlined and more automated annotation techniques will be crucial to realizing the full potential of IPA in this context.
Despite these challenges, using IPA mapping to guide voice cloning models improves the overall naturalness and expressiveness of synthetic speech. The ability to capture finer phonetic details opens up opportunities for crafting voices that are more engaging and relatable, thus contributing to a more seamless experience for those interacting with artificial voice technology. As the field continues to develop more refined transcription methodologies, we can expect synthetic voices to become even more sophisticated and nuanced, bridging the divide between human and artificial speech.
The integration of IPA mapping into voice cloning systems offers a path towards more nuanced and accurate voice replication. For example, in audiobook production, IPA enables capturing subtle pronunciation variations across dialects, allowing for more authentic character voices that reflect regional speech patterns. This level of detail can significantly enhance the listener's immersion in the story.
Moreover, real-time integration of IPA allows for dynamic adjustments to the phonetic output of voice models. Imagine a podcast where the host interacts with a synthetic voice in a fluid conversation. The voice model, thanks to IPA, can adjust its pronunciation on the fly, seamlessly adapting to the conversation's flow. This kind of adaptability is vital for creating engaging and natural-sounding interactions.
Similarly, when crafting audiobooks with multiple characters, IPA enhances the distinction between character voices. Each character can have a unique vocal identity that is not only based on tone but also on the fine details of pronunciation. This nuanced characterization creates a richer listening experience, mirroring the complexities of human storytelling.
Beyond characterization, IPA enhances noise resilience in voice models. For podcasters recording in less-than-ideal environments, this capability is crucial for producing high-quality audio. IPA mapping allows voice models to better filter out unwanted background noise, ensuring that the core phonetic information remains clear and understandable.
Furthermore, IPA paves the way for supporting a broader range of languages. By leveraging IPA's universal phonetic representation, voice cloning systems can handle languages with limited available training data. This opens the door to creating content in less-common languages, thereby increasing accessibility and promoting inclusivity.
Interestingly, IPA's impact extends beyond the mere reproduction of sounds; it can also capture and convey emotional nuances through speech. This capacity is critical for applications where conveying feelings through speech is vital to the narrative, such as audiobooks or interactive storytelling. By integrating emotional intonations within IPA mapping, synthetic voices can express a wider spectrum of emotions, making them more engaging and relatable.
Another benefit lies in improving training efficiency. By using pre-existing IPA datasets, voice cloning systems can significantly reduce the need for extensive new voice recordings, streamlining the training process, especially for less-common languages. This accelerates development and reduces the resources required for model training.
This increased emotional expressiveness contributes to making synthetic voices more relatable and user-friendly. In interactive applications like virtual assistants or customer service bots, where a sense of warmth and empathy is important, this capability becomes increasingly crucial for positive user interactions.
Furthermore, IPA mapping provides the ability to thoroughly analyze articulation variations, allowing voice cloning models to replicate the unique characteristics of individual voices more faithfully. The resulting clones are not simply imitations of sounds; they also retain subtle vocal nuances, making them sound more authentic and natural.
The advancements in IPA mapping are pushing the boundaries of audio content creation. Whether for entertainment purposes, professional training, or interactive educational tools, the possibilities for synthesizing engaging and believable voice content seem to be limitless. These improvements are shaping a future where human-computer interactions are more seamless and where audio experiences are richer and more personalized.
How IPA Phonetic Transcription Enhances Voice Clone Accuracy in 2024 - Global Accent Recognition Through IPA Pattern Learning
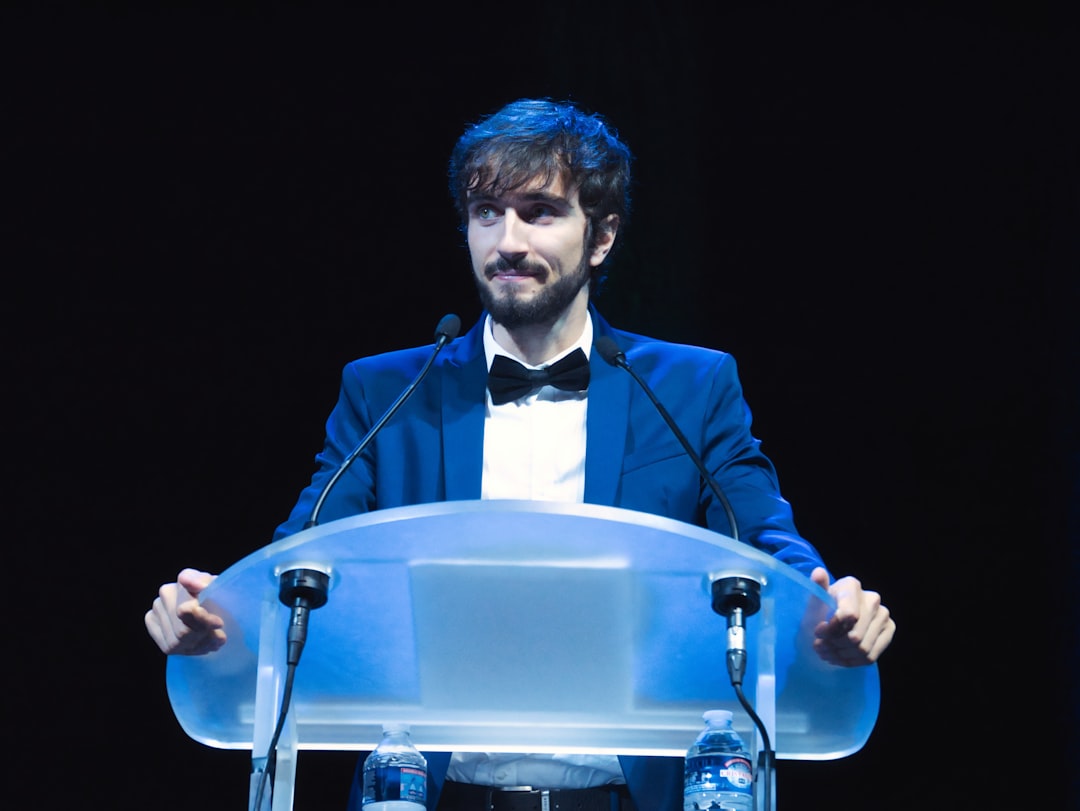
The ability to recognize accents globally through IPA pattern learning is a significant step forward in the field of voice technology. The International Phonetic Alphabet (IPA) provides a standardized way to represent sounds, which allows systems to better understand and replicate the specific nuances of different accents. This is particularly useful for applications like voice cloning, audiobook production, and podcasting, where capturing the subtleties of speech is essential for a high-quality and authentic user experience.
Traditional speech recognition systems often struggle when encountering non-native speakers or distinct accents. This is due to the complexity and variability of human speech. By relying on the consistent structure of IPA, researchers can design systems that are more adept at handling these variations. This approach is reflected in the development of Computer-Assisted Pronunciation Training (CAPT) tools that use IPA patterns to help individuals improve their pronunciation.
As we move towards a more interconnected world, the ability to create accurate voice clones that can seamlessly navigate the complexities of diverse accents becomes increasingly important. By integrating IPA into the core of voice cloning and accent recognition systems, we are moving closer to a future where synthetic voices sound more natural and understandable, no matter where the speaker originates. This is a key step in achieving more seamless human-computer interactions, particularly as we become increasingly reliant on voice-based interfaces for communication and entertainment. While the field still faces challenges, the potential for IPA to further improve voice technologies in 2024 and beyond is promising.
The International Phonetic Alphabet (IPA) has emerged as a powerful tool for enhancing the accuracy of voice cloning, particularly in recognizing and replicating diverse accents. Traditional voice cloning methods often struggle to capture the subtle nuances of pronunciation, relying primarily on sound wave analysis. However, by mapping speech sounds to IPA symbols, we gain a much more precise understanding of how sounds are produced. This detailed phonetic information enables voice clones to not only mimic accents with greater fidelity but also discern subtle differences in how people speak, even when those variations are difficult to distinguish for standard speech recognition systems.
For instance, training a voice model on a rich IPA-based dataset helps it better differentiate speakers with similar vocal qualities. This improved speaker recognition ability also extends to capturing emotional nuance through tone and inflection, which is essential for conveying character and narrative in audiobook productions. Imagine a voice clone that can accurately portray the subtle shifts in tone that convey sadness, joy, or anger. This capability, driven by the precise phonetic mappings, has led to substantial improvements in the realism of synthetic voices.
Moreover, the use of IPA has enabled the development of more adaptive voice cloning systems. Through real-time IPA recognition, voice models can adjust accents dynamically, tailoring the pronunciation to reflect different dialects or individual user preferences. This eliminates the need for extensive model retraining for every subtle change in vocal style, making voice cloning far more efficient and adaptable. This adaptability has implications for a wide range of applications, from audiobook production to interactive voice assistants.
One of the intriguing aspects of IPA-based voice cloning is its potential to address biases in existing voice models. Languages with limited training data often suffer from reduced accuracy in voice cloning. However, IPA, with its universal phonetic framework, can be used to bridge this gap. By translating existing IPA datasets into suitable formats for voice models, we can potentially achieve good results for these languages with a much smaller amount of training data.
Interestingly, this approach also enhances the ability of voice cloning systems to operate in noisy environments. By focusing on the core phonetic cues in a sound wave, even when masked by background noise, the models trained with IPA can filter out noise more effectively. This is critical for producing high-quality audio for podcasts, audiobooks, and other applications where recordings might not be taken under ideal conditions.
Furthermore, the use of IPA in voice cloning has opened the door to a new level of character voice differentiation. Now, when developing characters for audiobooks, voice designers can leverage not only tone but also the unique phonetic characteristics of each character. This adds a layer of authenticity and realism to the characters, allowing them to be much more easily differentiated by listeners.
However, developing these IPA-based voice cloning models isn't without its challenges. Obtaining detailed prosodic information about a speaker's vocal style can be complex and time-consuming. Building automated annotation systems to address this bottleneck would be a significant step forward for the field. This type of development could drastically reduce the time and effort needed to manually transcribe audio into IPA, thus accelerating progress.
Another exciting area of current development is personalized voice technology. Through real-time IPA processing, voice models could adapt to the pronunciation patterns and dialect of an individual listener. Imagine an audiobook that instantly modifies its pronunciation to match the listener's native dialect. This capability can personalize the listening experience and make it more accessible for a broader audience.
Finally, the integration of IPA into voice cloning is leading to the development of more sophisticated conversational AI systems. By adapting the tone and pitch of a voice clone in real-time to match conversational contexts, it’s possible to create interactions that are more dynamic and human-like. This development holds significant implications for building believable and engaging virtual assistants and interactive storytelling systems.
Though still a relatively new area of research, the potential of IPA for enhancing voice cloning is undeniable. Researchers continue to develop new methods and techniques for harnessing the power of this universal phonetic system. We can expect even more realistic and nuanced voice clones in the future, creating new and exciting possibilities for how we interact with computers and consume audio content.
How IPA Phonetic Transcription Enhances Voice Clone Accuracy in 2024 - Speech Pattern Consistency in Audiobook Productions
Maintaining consistent speech patterns is crucial for creating engaging and immersive audiobook experiences. The use of the International Phonetic Alphabet (IPA) in audiobook production, particularly in conjunction with voice cloning, significantly enhances this consistency. By providing a detailed, standardized representation of sounds, IPA helps voice actors achieve uniformity in their pronunciation, especially when portraying multiple characters or maintaining a consistent narrative tone. This translates to a more natural and believable listening experience. As voice cloning technology continues to develop, the integration of IPA allows for a deeper level of control over pronunciation, making it easier to capture the nuances of various dialects or create distinct character voices that sound authentic and relatable. Furthermore, the accuracy offered by IPA paves the way for synthetic voices that can express a wider range of emotions, thus adding depth and richness to audio stories and enhancing the listener's engagement. In an environment where audio content is increasingly prevalent, achieving consistent and expressive synthetic voices becomes vital for successful storytelling and communication across different audio platforms. The continued development of these techniques will likely result in more captivating and effective audiobook productions.
The use of the International Phonetic Alphabet (IPA) in audiobook productions is becoming increasingly important for enhancing voice clone accuracy and naturalness. IPA allows voice cloning systems to capture the unique phonetic characteristics of individual speakers, extending beyond simply replicating accents to encompass personal quirks in pronunciation. This detailed level of phonetic information makes synthetic voices sound more authentic and reflective of the original speaker's identity.
Further advancements in IPA mapping techniques have enabled dynamic prosody modeling, where synthetic voices can adjust intonation and emphasis depending on the context. This is particularly crucial for producing more realistic dialogues in audiobooks and podcasts, allowing for more natural emotional shifts and nuanced narrative delivery. The ability to capture these prosodic variations greatly enhances audience engagement with synthetic voices.
Another notable advantage of IPA is its ability to facilitate the integration of diverse linguistic data into voice training models. This is particularly helpful for languages that lack significant training datasets, allowing for the creation of clearer and more relatable synthetic voices in a wider range of languages. This is a step forward in breaking down barriers and increasing accessibility for users globally.
Furthermore, the IPA-based voice models exhibit greater resilience in noisy environments. This is due to the improved ability to isolate core phonetic information, helping to filter out background noise more effectively. This is a substantial advantage in real-world audiobook and podcast recording scenarios, where noise can be a significant factor.
Researchers are also investigating the potential of IPA to facilitate emotion detection in synthetic speech. This exciting area of research aims to allow voice clones to adapt their expressive qualities based on context. This would potentially mean that a voice clone could adjust its tone to reflect happiness, sadness, or any number of emotions, depending on the scenario. This is a key area for improving naturalness, especially for narrative-driven content.
With the use of IPA, producers can create diverse and distinctive vocal identities for multiple characters in audiobooks, making it easier for listeners to differentiate between them. Each character can be given unique phonetic and prosodic traits, which can greatly enhance the richness and complexity of the storytelling.
The integration of IPA allows for real-time pronunciation adjustments that accommodate diverse regional accents. This means that an audiobook could automatically adapt its pronunciation to align with the listener's native dialect, significantly enhancing the listening experience and increasing accessibility for a larger audience. This flexibility is a significant benefit for inclusivity and reach.
Computer-Assisted Pronunciation Training (CAPT) tools that leverage IPA patterns are increasingly available, giving users instant feedback on their accent and pronunciation. This can be used for educational purposes, but also opens the possibility for interactive elements in podcasts or audiobooks where users can practice their pronunciation skills alongside a story or educational program.
The universality of IPA aids in the development of voice cloning systems that can switch seamlessly between languages with accurate accents. This is crucial for listeners in multilingual contexts, especially when listening to podcasts or audiobooks that span multiple languages, as it maintains comprehensibility and audience engagement.
Finally, by leveraging IPA mapping, voice cloning systems are achieving more nuanced and context-dependent emotional expressions in synthetic speech. This allows for more dynamic voice models able to capture subtle emotional cues, such as sarcasm or disbelief, which were a major challenge for previous generations of voice cloning technology. These advancements help make synthetic voices sound more human-like, allowing for more natural and engaging conversational settings.
While the journey to perfect voice cloning continues, the advancements enabled by the International Phonetic Alphabet are undoubtedly significant. They're paving the way for a future where audiobooks, podcasts, and voice-based technologies are more engaging, accessible, and believable than ever before. The ongoing research and development in this field promise further breakthroughs and exciting new possibilities in how we experience and interact with audio content.
How IPA Phonetic Transcription Enhances Voice Clone Accuracy in 2024 - IPA Based Voice Clone Adaptation for Podcast Hosting
IPA, the International Phonetic Alphabet, has become increasingly vital for refining voice cloning technology in 2024. It's shown its value in capturing the subtleties of how we articulate sounds, which is crucial for making synthetic voices sound more authentic. This level of detail is a real boon for applications like audiobook narration or interactions with virtual assistants, where a more natural-sounding voice is preferred.
One notable advantage of IPA is its ability to give voice models a lot more flexibility when it comes to adapting to various languages and accents. The real-time integration of IPA lets these models seamlessly adjust their pronunciation without extensive re-training. This is a big win for podcasters and audiobook creators, who can save a lot of time and resources.
Furthermore, research has shown that voices trained on IPA data are much better at handling noisy environments. This is a huge plus for recording audiobooks and podcasts, where ideal recording conditions aren't always possible. By focusing on core phonetic sounds, these models can effectively filter out unwanted noise, ensuring the clarity of the audio output.
The benefits of IPA extend to capturing the nuances of prosody, which encompasses things like intonation and rhythm. The ability to incorporate these aspects with IPA allows for more nuanced emotional expressions in synthetic voices. This makes synthesized voices more relatable for listeners, especially in storytelling environments where expressing emotions is essential.
Similarly, voice models leveraging IPA can generate more consistent speech patterns throughout longer recordings. This is vital for audiobook producers handling multiple characters, ensuring a more cohesive narrative for the listener. By incorporating phonetic detail, it also gets easier to differentiate between characters, improving the listener's engagement with the story.
It's surprising, but using IPA can also significantly reduce the amount of data needed to train voice models, particularly for languages with smaller datasets. This is a huge advantage as it accelerates the development of high-quality synthetic voices for a wider variety of languages.
The universal nature of IPA makes it a powerful tool for crafting multilingual audio content. This is crucial for podcasters or audiobook producers who want to create content for global audiences. It ensures the clarity and coherence of the content across different languages, contributing to a more engaging experience.
IPA is also playing a key role in enhancing the capacity of voice clones to express emotions. It helps in detecting and replicating emotional cues within the audio, resulting in more human-like communication. This is a vital aspect in improving listener engagement and retention, especially for compelling audio content.
The rise of Computer-Assisted Pronunciation Training (CAPT) tools incorporating IPA is another promising area. These tools offer real-time feedback to users on their pronunciation, making them ideal for interactive audio content. Think podcasts or educational audiobooks that can include pronunciation practice segments.
Finally, the use of IPA has significantly improved the ability of synthetic voices to accurately recognize different accents. This is a substantial benefit for creators seeking to produce content that resonates with a broad range of listeners from different cultural backgrounds, enhancing user satisfaction.
While the field of voice technology is continuously evolving, the use of IPA has proven to be a cornerstone for enhancing the accuracy and naturalness of synthetic speech. It is a testament to how valuable phonetic detail is in shaping the future of how we interact with computers and experience audio content.
More Posts from clonemyvoice.io: