How Generative AI Voice Models Transform Call Center Training Through Automated Speech Analysis
How Generative AI Voice Models Transform Call Center Training Through Automated Speech Analysis - Voice Pattern Recognition Systems Cut Agent Training Time By 40% Through Real-Time Feedback
Voice pattern recognition systems are changing how call center agents are trained, allowing for a dramatic reduction in training times—up to 40%—by providing immediate feedback. This advancement utilizes generative AI voice models and automated speech analysis to dissect agent interactions in real-time, allowing for more effective coaching and improvements in performance. The use of AI brings valuable efficiency but also presents challenges like biases in the AI models and potential job displacement. The industry must cautiously manage these consequences as AI integrates deeper into operations. As voice technologies become even more refined, ensuring the accuracy of AI outputs will become increasingly crucial for preserving clear and helpful communication as well as high-quality service.
Voice pattern recognition systems are increasingly sophisticated, delving into the nuances of human speech, including subtle variations in tone, pitch, and rhythm. This allows the systems to identify emotional cues embedded within audio, potentially providing real-time feedback to agents on how to adjust their interactions. Imagine a system that can sense frustration in a customer's voice and prompt the agent to adopt a more empathetic tone – this is the kind of immediate guidance that these technologies can offer.
It's fascinating that studies suggest these systems can process sound data at a speed far exceeding human comprehension—up to 200 times faster, according to some estimates. This drastic speed advantage can significantly accelerate the training process and make feedback cycles incredibly efficient. The quicker turnaround time for feedback directly translates to faster development for agents and faster improvement cycles for training materials.
We also see this emerging in voice cloning technologies, which are utilizing deep learning to recreate individual vocal patterns with impressive accuracy. This means synthesized speech can remarkably mimic the original speaker, which is particularly useful in creating consistent training scenarios where replicating a certain vocal delivery is crucial. Though, there are concerns with ethical implications surrounding the accuracy of voice cloning and how these technologies can be misused.
This real-time feedback, made possible by advanced voice analysis, is not merely shortening training time, which has been shown to be as much as 40%, but also improving the effectiveness of agents and ultimately increasing customer satisfaction. While it remains to be seen if such claims of improvement are consistently valid, initial data is encouraging.
Furthermore, by analyzing vast amounts of voice recordings, these systems can progressively adapt and become more adept at discerning different accents and dialects. This ability reduces the need for extensive, individualized training to cater to diverse customer populations and make it easier for agents to effectively connect with a wider range of people.
While this technology is still developing, and some argue that these technological gains do not address the core problems faced by call centers, the ability to fine-tune training content to reflect specific interactions and contexts has a significant impact on the overall quality of service. Voice recognition is not limited to call centers. It is finding its way into audiobook production, where systems can identify mispronounced words during recording sessions and automate editing. Also in podcast production, the application of voice recognition technologies can lead to quicker and better editing and transcription.
It is worth noting that while human feedback can be influenced by personal biases, AI-driven voice recognition offers a more objective perspective. This eliminates some of the inconsistencies and variations inherent in subjective assessment.
The promise of customizing training to better reflect real-world situations by recording voice interactions in a variety of environments remains exciting. This aspect of training allows agents to anticipate and respond appropriately to the range of interactions they might face. While some argue that the focus should be on providing comprehensive and quality training to humans in the first place, there is a school of thought that believes AI-driven voice recognition can be beneficial in achieving the desired outcome.
The future of voice recognition technologies in areas such as podcast production is still being defined. However, improvements to voice transcription, editing, and production capabilities provide creators with powerful new tools to enhance their craft, ultimately producing higher quality work with less effort. While the adoption and utilization of such technology will no doubt evolve, its integration in audio production and beyond is quite remarkable.
How Generative AI Voice Models Transform Call Center Training Through Automated Speech Analysis - Speech Analytics Tools Transform Raw Call Data Into Training Modules For New Hires
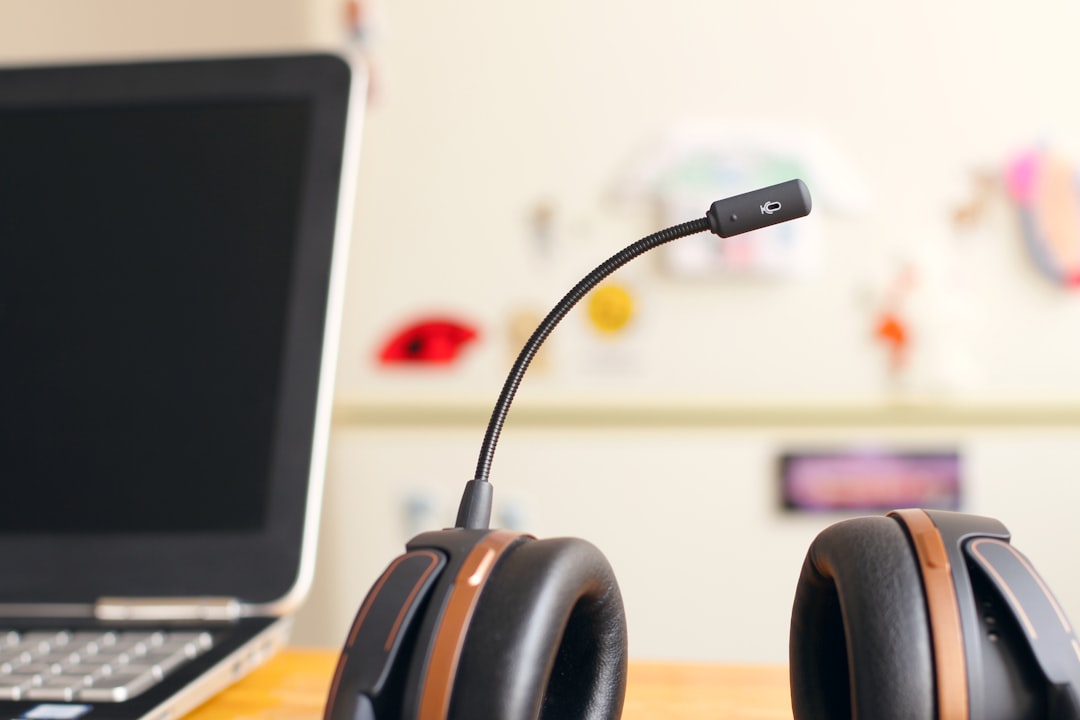
Speech analytics tools are revolutionizing how new call center agents are trained by transforming the raw audio of customer interactions into valuable training resources. These tools, powered by technologies like Automatic Speech Recognition (ASR) and Natural Language Processing (NLP), dissect the audio, converting spoken words into text and identifying patterns within conversations. By analyzing the transcribed data, training managers can create custom training modules that address specific customer issues and agent performance deficiencies. This capability allows them to pinpoint common problems or areas where agents struggle, helping tailor training for quicker improvements.
The effectiveness of training is enhanced by these analytics since trainers can pinpoint specific situations where agents need development. However, while there are significant benefits to this data-driven approach, we must be mindful of the potential downsides. AI algorithms that analyze these interactions can perpetuate biases that may exist in the original dataset, leading to a skewed understanding of the data. Oversimplifying nuanced human interactions is a risk, leading to training modules that fail to capture the complex reality of customer service. Moreover, there are valid questions about the future of human interaction in the realm of training and customer service, as AI-based voice recognition tools become more pervasive.
While speech analytics tools are still developing, their potential to reshape call center training is clear. It's a compelling development that could streamline onboarding, improve performance, and ultimately enhance customer satisfaction. This is particularly fascinating in the context of voice cloning, podcast creation, and audiobook production, where the application of AI-driven tools can streamline audio production and editing. However, the continued refinement of these technologies necessitates a thoughtful approach to address the ethical and practical considerations they introduce.
Speech analytics tools go beyond simply transcribing conversations. They delve into the nuances of speech, including "uhs," pauses, and other non-verbal cues, which can profoundly impact the meaning and context of a call. This provides a deeper understanding of the complex interplay between agents and customers, moving beyond simple word counts.
These tools can automatically pinpoint phrases or keywords crucial to a company's objectives, extracting valuable insights for refining training materials and customer service strategies. This targeted approach helps businesses hone in on the specific areas where agents need improvement, leading to more effective training.
Exciting advancements in audio signal processing are enabling systems to detect a wider range of emotions beyond just anger or joy. Tools are becoming more capable of recognizing subtle emotional cues like disappointment or confusion, providing a more nuanced understanding of customer interactions and informing training strategies that better address those complex emotional states.
By combining speech analytics with voice cloning technology, we can create hyper-personalized training scenarios. Synthetic voices could be used to simulate challenging customer interactions, allowing trainees to practice handling difficult situations before they encounter them in the real world. This ability to tailor practice to the specifics of certain situations is highly promising, but there are concerns about the ethical implications.
The speed of speech analytics is a game-changer for training. It allows for immediate adjustments in training content to incorporate evolving trends and address common customer questions without the lengthy revision processes of the past. This agility can keep training relevant and up-to-date, a critical feature in fast-paced industries.
Audiobook production, previously reliant on human narrators, is increasingly incorporating voice synthesis technologies that can replicate a specific narrator's style and vocal nuances. This approach enables faster, potentially more cost-effective production without necessarily compromising on quality. Yet, the use of artificial intelligence raises important questions about human artistic expression, the preservation of personal artistic styles, and the ethical implications of these methods.
Podcast producers are employing AI-driven voice recognition tools to refine audio quality and reduce the need for manual edits. Beyond basic mispronunciations, systems can now identify and eliminate background noise and balance sound levels with precision, enhancing audio clarity and polish. But, as with any technology, there is a potential for unintended consequences or flaws.
Speech analytics tools have created a new generation of metrics to assess agent communication styles. This move allows trainers to fine-tune instruction based on each individual's strengths and weaknesses, ensuring that training is tailored to the specific needs of each agent. This development builds upon existing knowledge in a more specific and targeted manner.
Voice cloning technology holds the potential to democratize audiobook narration. It could empower authors to utilize their own voices or explore cloned versions that reflect their preferred style, removing a major barrier to entry for aspiring authors and potentially allowing them to build a personal brand through their audio content. The ease of access is one side of the issue. The other side is the potential exploitation of such technology.
Research suggests that voice-based learning can significantly enhance memory and recall rates for trainees. If accurate, this means that speech analytics not only helps improve agent performance but also contributes to more long-lasting knowledge retention within the call center workforce. This is a noteworthy finding and it would be beneficial to study if the positive impact is as substantial as suggested, but also what are the risks of a over-reliance on voice-based learning in this area.
How Generative AI Voice Models Transform Call Center Training Through Automated Speech Analysis - AI Voice Simulations Allow Agents To Practice Difficult Customer Scenarios Safely
AI voice simulations offer a valuable training ground for call center agents, enabling them to practice handling challenging customer interactions in a safe, controlled setting. These simulations allow agents to tackle difficult scenarios, such as managing irate customers or navigating complex technical support requests, without the pressure of real-world consequences. By creating immersive role-playing scenarios with diverse customer personas and challenging situations, agents can develop their ability to confidently manage a wide range of customer interactions while staying within established procedures.
The potential of this technology is vast as AI voice simulations become more sophisticated, capable of replicating increasingly complex and nuanced conversations. This could lead to significant improvements in agent skills and, potentially, better overall customer satisfaction. Moreover, these simulations foster a learning environment that promotes ongoing skill development and adaptation, contributing to a culture of continuous improvement within the call center environment. While the benefits are promising, it's important to acknowledge the ongoing development and potential limitations of the technology, including its ability to accurately reflect the complexity of real-world scenarios. Despite this, the trajectory of AI-powered voice simulations in call center training appears to be leading towards a future where agents are better equipped and prepared to offer consistently excellent customer service.
AI voice simulations are increasingly used in training for a variety of applications, particularly in areas like voice cloning, podcast production, and audiobook creation, due to their ability to create safe and controlled environments for practice. These simulations allow agents, or even artists in the audio space, to safely navigate complex scenarios without real-world consequences.
For example, advanced voice cloning technologies are capable of recreating a speaker's voice with remarkable precision. This feature opens a door to diversity in training scenarios. Imagine a scenario where an agent can practice interacting with various vocal styles or tones – it provides a more adaptive method for developing communication skills.
Furthermore, some AI systems are becoming adept at detecting subtle emotional nuances in spoken dialogue, such as frustration or confusion. This capacity enables agents to receive real-time cues on how to adapt their responses based on a customer's emotional state. For example, an AI system might pick up on a frustrated customer’s tone and suggest the agent employ a more empathetic approach.
By leveraging synthesized voices, training scenarios can be designed with specific difficulties in mind. This hyper-personalized approach helps agents prepare for uncommon customer interactions in advance, refining their ability to respond to a wider range of interactions they might encounter.
These systems have a significant speed advantage over human comprehension, with the capacity to process sound data roughly 200 times faster. This incredible processing speed allows for immediate adjustments to training modules, helping ensure the content stays relevant in quickly changing environments. It's important to note that this rapid turnaround directly benefits the speed at which agents can be trained and improved.
Modern speech analytics tools not only examine the words spoken but also speech patterns, filler words (like "uh" or "um"), and pauses. These subtle aspects provide trainers with a comprehensive view of agent performance, going beyond a simple assessment of whether a script is followed.
AI is also enabling advances in audiobook production. Voice synthesis technology can recreate the author's voice with a high degree of accuracy, potentially eliminating the need for a separate narrator while maintaining the author's intended style. The process can be streamlined, allowing authors more creative control over the presentation of their works.
Podcast editing is also seeing significant improvements. AI-driven voice recognition tools can now easily identify background noise and other audio anomalies, streamlining the post-production process. This means podcast producers can allocate more time to developing quality content rather than spending countless hours on manual editing.
While it is an active area of research, voice-based learning may enhance memory and recall for learners. This suggests that AI-powered speech analysis not only improves agent performance but also contributes to longer-lasting knowledge retention within the workforce. It is worth noting that further research is needed to more definitively confirm the impact of voice-based learning and also understand any associated risks.
AI-driven feedback mechanisms can adapt training content in real-time based on trends in customer interactions. This personalized training, combined with content that directly addresses current customer challenges, enhances the overall training experience.
The expanding use of voice cloning tools in various applications also introduces ethical considerations. Questions about copyright, personal voice ownership, and the potential for misuse of synthesized voices will need to be addressed with thoughtful approaches to ensure that these technologies are used responsibly and fairly.
While these developments offer incredible possibilities, it is crucial to monitor and address the evolving ethical and practical implications they present. It's a reminder that the advancements of technology can have unintended or unforeseen consequences, and it is important to be mindful and prepare for such possibilities.
How Generative AI Voice Models Transform Call Center Training Through Automated Speech Analysis - Natural Language Processing Maps Customer Tone and Intent During Training Sessions
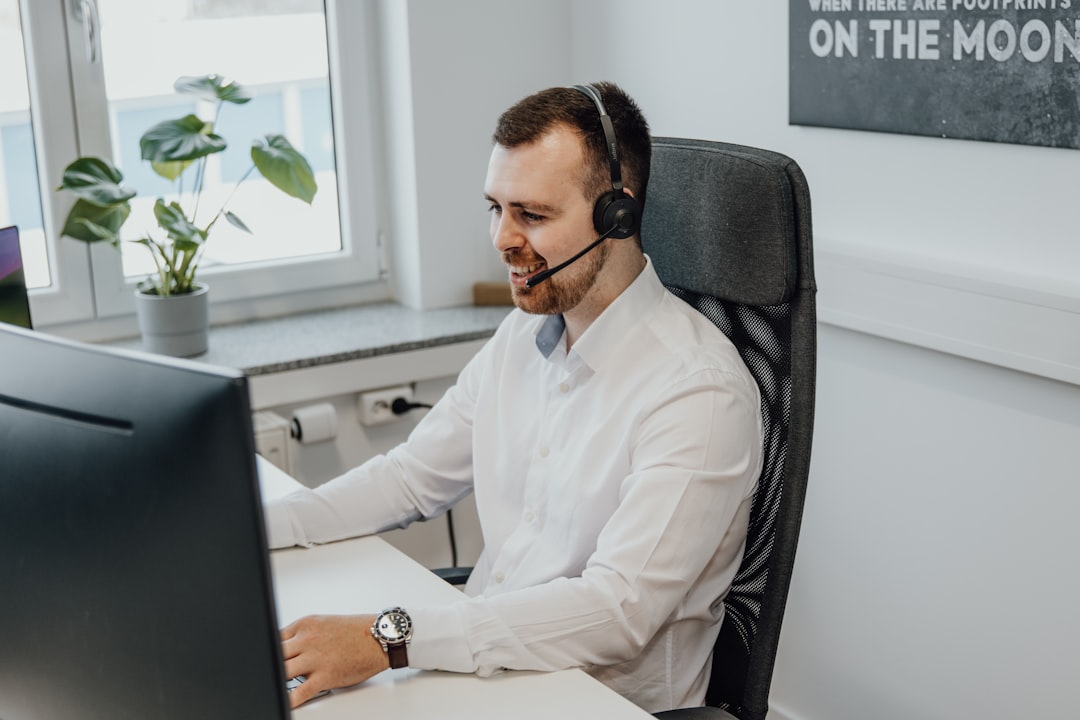
During training sessions, Natural Language Processing (NLP) has become increasingly important for understanding the subtleties of customer interactions. NLP systems can now analyze the emotional tone of conversations, classifying them as positive, negative, or neutral using sophisticated AI algorithms. This allows for a finer understanding of the customer experience, identifying the underlying intent behind what's being said. By leveraging these insights, training programs can be better designed to focus on specific areas where agents need to improve their communication and empathy.
This capability also leads to more individualized feedback for agents. As a conversation unfolds, the system can provide real-time insights into how the agent is perceived. Does the agent sound empathetic when a customer is frustrated? Or do they need to adjust their communication style to better match the customer's emotional state? This instant feedback fosters more refined and contextually aware responses.
The power of NLP is that it can tailor training in a way that more effectively addresses the unique nuances of customer interactions. This shift towards personalized training can result in better overall customer service, as agents become more adept at handling various situations with increased empathy and understanding. The end result of these tools can be a better overall experience for the customer and ultimately improvements in customer satisfaction. While there are challenges with biases in AI models and a need to ensure that the technology is used responsibly, the potential for NLP to transform call center training and other audio-related areas, like audiobook production or podcast creation, is clear.
Natural language processing (NLP) is becoming increasingly sophisticated in its ability to dissect the nuances of human speech. It goes beyond simply understanding the words spoken, delving into the subtle shifts in tone and pitch that reveal a speaker's emotional state. This capability is proving especially useful in areas like voice training for call center agents, where understanding the emotional context of a customer interaction is crucial. For example, NLP models can now discern subtle changes in vocal patterns that indicate a customer's anxiety or uncertainty, providing trainers with more specific feedback for agents.
The accuracy of these systems in detecting emotion is steadily improving. Some research suggests that NLP can identify complex emotional cues with an accuracy exceeding 80%. This heightened accuracy can transform vague customer sentiments into valuable actionable data, making training more effective and relevant. This data-driven approach can also help ease the cognitive burden on agents. By automatically analyzing customer tone and intent, NLP allows agents to focus more on problem-solving during calls rather than constantly trying to interpret a customer's emotional state in real-time, which can lead to a boost in productivity and customer satisfaction.
Furthermore, NLP tools enable dynamic adaptation in training. The ability to instantly analyze customer interactions and adapt training materials in real-time is a significant advancement. For instance, if a system detects that a certain type of customer question or emotional response is frequently encountered, the training modules can be instantly revised to address these trends. This kind of agility helps keep training fresh and relevant, which is critical in a fast-paced industry like call center operations.
Voice cloning, a technology based on deep learning, is being integrated into NLP-driven training. By replicating different vocal patterns with accuracy, it creates a wider array of customer persona simulations for agents to interact with. This added diversity in training environments enriches the learning experience and helps agents develop greater adaptability in communication. While promising, we must acknowledge the inherent bias present in AI training datasets. These datasets are a reflection of the data they're trained on and can perpetuate biases if not carefully curated, which could negatively influence the feedback and training outcomes.
The integration of NLP is revolutionizing audio production. In audiobook creation, NLP has automated the detection of mispronounced words and other errors, significantly reducing the time required for post-production editing. It's halved, some studies suggest. The technology is similarly transforming podcast production. NLP tools are now automating the removal of filler words, excessive pauses, and background noise, allowing podcasters to streamline the editing process and focus on content creation.
Authors themselves can also benefit from these tools. Some AI systems can evaluate an author's reading style during audiobook practice sessions, providing direct feedback on how to improve the vocal delivery. This targeted feedback allows authors to refine their performance before the official recording, ultimately enhancing the quality of the finished product.
This NLP-driven feedback can also help create simulated call scenarios that challenge agents' emotional intelligence. By recreating stressful or complex situations, training modules can teach agents strategies for responding to difficult customers, building a robust foundation for handling real-world challenges more effectively. These simulations prepare agents to practice calming techniques and responses for challenging situations, an increasingly crucial skill in today's customer-centric service industries.
While the potential of AI voice technology is remarkable, it’s vital to remain cautious. These innovations, while potentially groundbreaking, come with their own set of ethical and practical considerations, particularly when it comes to voice cloning. We need to carefully monitor the technology's development and usage to ensure fairness and responsibility, as the line between creative expression and exploitation becomes ever thinner.
How Generative AI Voice Models Transform Call Center Training Through Automated Speech Analysis - Voice Cloning Technology Creates Personalized Practice Sessions For Individual Agents
Voice cloning technology is transforming how call center agents are trained by creating customized practice sessions. Leveraging deep learning, these systems can generate synthetic voices that mimic real individuals, allowing agents to practice interactions with a variety of customer personalities and emotional tones. This tailored approach helps build confidence in managing challenging conversations and improves communication skills overall. While the potential for enhanced customer interactions is clear, ethical concerns regarding the potential misuse of voice cloning technology warrant consideration as this field continues to advance. The adoption of voice cloning in training marks a shift towards more refined and effective agent development, but responsible implementation is essential as we navigate the complexities this technology presents. Moreover, as the technology improves, there are concerns that AI-generated interactions, no matter how realistic, might not capture the full spectrum of human interaction. This is an area that needs more research and understanding. While this approach holds great promise for training, there is still the question of whether it can fully replicate the dynamic complexities of human-to-human interactions. It's also worth considering if this type of training reduces the need for strong foundational soft skills training for new agents. These questions are being investigated as the technology evolves.
Voice cloning technology, powered by techniques like WaveNet developed by DeepMind, has advanced significantly in its ability to generate incredibly natural-sounding speech. By predicting sound waves one sample at a time, these systems can create synthetic voices that are remarkably close to the original speaker, surpassing the quality of earlier methods. However, this high fidelity also raises important questions about the authenticity and potential misuse of these replicated voices, especially in fields like customer service where context and trust are critical.
Beyond simply mimicking vocal patterns, generative AI models used in voice cloning are increasingly adept at learning and replicating the emotional nuances present in a speaker's recordings. This means that synthesized voices can now convey emotions like happiness or frustration, enriching the dynamics of training scenarios and making interactions feel more lifelike.
This technology is already transforming the creation of audiobooks. Authors can now produce narrations using a cloned version of their own voice, offering a level of personalized storytelling that maintains their unique vocal characteristics throughout the audiobook. Interestingly, some studies suggest that listeners find audiobooks narrated by a synthetic clone of the author more engaging than those narrated by professional voice actors. This suggests that there's a perceived authenticity and connection that enhances the listening experience.
In the realm of call center training, the integration of NLP-driven systems with voice cloning allows for real-time feedback and greater adaptability during training exercises. This means agents can learn to react dynamically to specific conversational styles and emotional tones within simulated customer interactions. This dynamic learning environment, while potentially very useful, needs to be cautiously observed to see if it truly benefits agents in the long term.
Voice cloning has also streamlined the podcast editing process. AI systems can now automatically identify and remove filler words like "um" and "uh," significantly reducing the time required for manual editing. This automation frees up content creators to focus more on developing high-quality content, which is beneficial, but we also need to be aware of unintended consequences, such as a loss of creative nuance in audio.
Voice cloning systems are also increasingly able to adapt to different dialects and accents. This flexibility makes it possible to create training modules tailored for specific customer bases, enabling agents to prepare for a wider variety of communication styles they might encounter in real-world scenarios.
Furthermore, the bandwidth requirements for high-quality voice cloning are becoming increasingly efficient. Compression techniques are allowing for high-fidelity audio delivery even over limited bandwidth, expanding the accessibility of remote training and production. While promising, it is also important to remain vigilant in ensuring that accessibility does not come at the cost of audio quality or security.
Research suggests that incorporating voice cloning into training can not only boost agent confidence but also enhance overall learning outcomes. Some studies have indicated a doubling of recall rates when audio-based learning is involved. While these findings are promising, further investigation is needed to fully understand the long-term effects of relying heavily on synthetic audio in learning and training scenarios. It is crucial to understand the trade-offs involved in using this technology and be cautious of placing too much reliance on it.
How Generative AI Voice Models Transform Call Center Training Through Automated Speech Analysis - Automated Speech Analysis Detects And Corrects Common Agent Communication Mistakes
Automated speech analysis is increasingly being used to detect and correct common communication errors made by individuals in various audio-related fields. These systems employ sophisticated algorithms to analyze audio data, identifying patterns in speech that correlate with successful communication and pinpointing areas for improvement. This analysis provides real-time feedback, allowing trainers to guide individuals towards better communication and potentially boosting overall outcomes, like customer satisfaction in call centers. However, as these AI-driven tools become more prevalent, it is crucial to acknowledge the possibility of biases within the datasets and technology. These biases could impact the quality and fairness of the training and feedback. Nevertheless, the integration of automated speech analysis is a notable change in training techniques, particularly in areas involving voice interactions like voice cloning, audiobook narration, and podcasting, offering more efficient and effective pathways for skill development in these evolving media.
Automated speech analysis, capable of processing audio at speeds up to 200 times faster than humans, allows for rapid feedback during training. This accelerates learning cycles, enabling agents to swiftly refine their skills and enhance performance. It's quite remarkable how these systems can identify subtle emotional cues embedded within the voice, such as shifts in tone or pitch. This "emotional analysis" allows for a deeper understanding of customer interactions, helping agents tailor their responses more effectively.
Voice cloning has enabled the creation of training modules that provide personalized practice sessions. It allows trainees to interact with a variety of synthetic customer personalities, complete with unique vocal characteristics and emotional tones. This highly customized training approach can significantly improve communication skills and build confidence for dealing with challenging customer interactions. It's also fascinating to note that some studies suggest audiobooks narrated by AI-cloned versions of authors are more engaging to listeners. This could signify a shift in how we consume audiobooks and possibly the creation of a more personalized audio experience.
For podcast production, AI-driven voice recognition is streamlining the editing process, eliminating filler words, background noise, and enhancing audio quality. This time-saving innovation allows creators to focus on content rather than endless manual edits. Interestingly, these tools also have the potential to adapt training content dynamically to reflect changes in customer trends, allowing trainers to keep training material relevant. There is an obvious risk however. The high quality of these synthetic voices, coupled with the ability to imbue them with various emotional nuances, also raises concerns about potential for impersonation and misuse. It’s crucial that we stay aware of these risks and ensure that as voice cloning technology develops, it's employed responsibly.
Another interesting aspect is the objective nature of feedback provided by these systems. Voice analysis can remove biases that can be present in human assessments, enabling trainers to deliver more accurate and targeted feedback tailored to each agent’s strengths and weaknesses. Researchers are also exploring the potential impact of AI-driven voice-based learning on memory and recall. Studies hint that this method could lead to improved information retention compared to traditional training methods. This is a promising idea but requires further investigation to see if the benefits are as substantial as early studies suggest and what are the potential consequences of over reliance on AI for learning.
Finally, these technologies allow for the creation of a wider range of training scenarios, simulating diverse customer dialects and accents. Agents can be better prepared to interact with a wider range of people, potentially bridging communication gaps and improving service quality. It’s encouraging that these AI-driven tools are offering possibilities to make training more efficient, relevant, and personalized, though there are many complex questions still to be answered about responsible use and potential impact of this technology on our communication habits.
More Posts from clonemyvoice.io: