Get amazing AI audio voiceovers made for long-form content such as podcasts, presentations and social media. (Get started for free)
How Azure AI Services Are Revolutionizing Voice Clone Consistency in Audio Production Studios
How Azure AI Services Are Revolutionizing Voice Clone Consistency in Audio Production Studios - Azure Voice Studio Updates Deep Learning Configuration for DragonHDv1Neural Model
Azure Voice Studio has recently tweaked the deep learning settings within the DragonHDv1Neural model. This update is meant to improve the model's capabilities, especially in the realm of high-definition audio synthesis. The focus is on generating audio that sounds more natural and contextually appropriate, potentially benefiting applications like audiobook narration or podcast creation where a consistent voice is crucial.
Interestingly, using these new HD voices doesn't require learning a new set of tools. Developers can continue leveraging the same speech synthesis SDKs and REST APIs as before, making the transition smoother for existing projects. The capability to fine-tune the neural voice model allows for a greater variety of speaking styles and broader language support. This adds more flexibility when creating synthetic voices that can better mimic different accents, emotions, and even personalities.
The ramifications of these updates for voice cloning, particularly when it comes to creating believable voice bots, are still unfolding. While it's still early days, these enhancements could very well transform how realistic synthetic voices are used in various audio productions, changing the way we interact with digital interfaces and consume audio content. There are still limitations and challenges, particularly in getting the nuances of human speech entirely right, but this update represents a notable step forward in improving the capabilities of this technology.
Azure Voice Studio has recently tweaked the inner workings of their DragonHDv1Neural model, focusing on its deep learning setup. This fine-tuning is aimed at pushing the boundaries of what's possible with synthetic speech. Essentially, they're trying to make the generated audio sound even more like a real person, which is vital for things like audiobooks and podcasts.
While the underlying foundation of the model remains the DragonHDv1Neural, the updates are tracked through version control, offering some insight into the development process. The good news is that if you're already using the Azure Speech SDK or REST APIs, these HD voices are integrated seamlessly. No need to learn a new system. They've managed to create a relatively uniform experience across HD and non-HD voices.
It's worth noting that the 'Custom Neural Voice' feature allows for crafting synthetic voices with varied speaking styles and language support, all driven by neural networks. If you want to create your own voice clone, you can use Azure's Speech Studio, ensuring you pick the 'Neural' training method. The model seems geared towards producing universal voices that work across multiple languages and speakers, a testament to Azure's efforts in building robust, versatile voice synthesis systems.
One thing that I find intriguing is the integration of Azure OpenAI services. Specifically, a new lineup of models (O1 Series) appears to be playing a role. How that translates to audio production is something that deserves further exploration. It also appears that they are employing techniques like VoiceRAG (Voice Retrieval Augmented Generation) by utilizing Azure AI Search to enrich the generated voice responses with relevant knowledge.
While this combination of AI features shows promise, it's important to cautiously monitor their impact on voice cloning and how these tools might evolve. The potential for generating extremely convincing and perhaps even indistinguishable synthetic voices warrants a thoughtful approach. It feels like we are at a turning point in voice synthesis. These recent Azure advancements suggest that we're moving towards a future with even more sophisticated voice bots and voice technology, though, it will be interesting to see how it unfolds.
How Azure AI Services Are Revolutionizing Voice Clone Consistency in Audio Production Studios - Professional Recording Studios Adapt to 300 Utterance Training Requirements
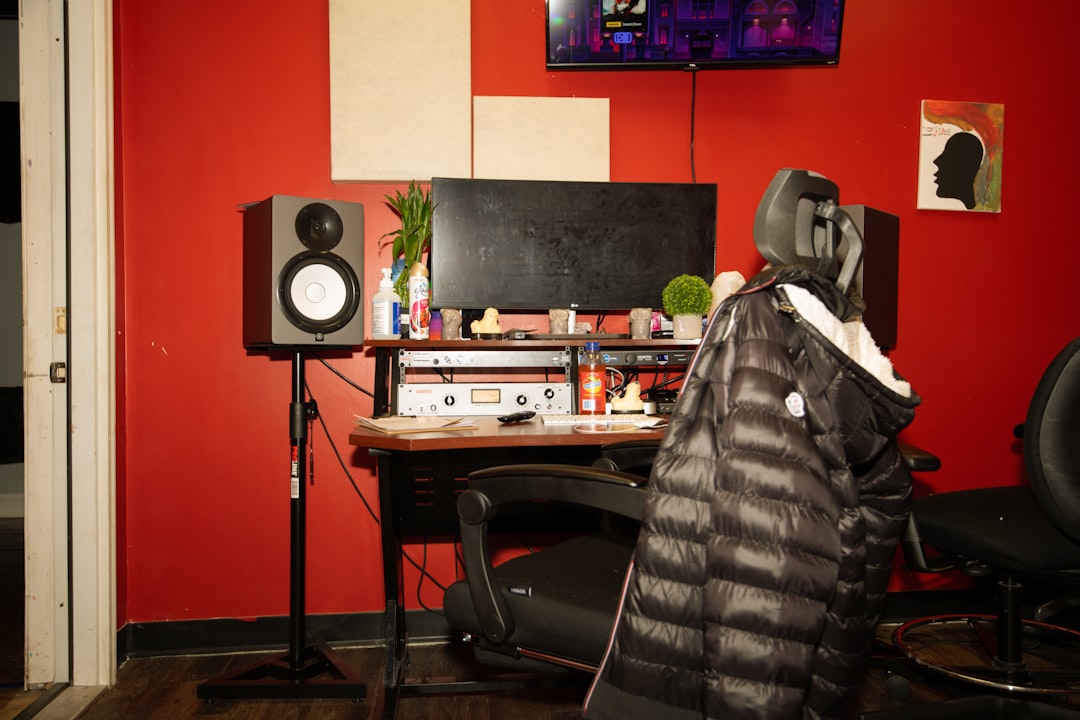
The rise of AI voice synthesis, especially systems like Azure's Custom Neural Voice, has introduced a new standard for professional recording studios: the 300 utterance training requirement. This means studios must now capture a larger volume of voice samples to train these AI models effectively. Achieving high-quality synthetic voice outputs necessitates a higher focus on capturing audio with a superior signal-to-noise ratio, pushing studios to refine their recording techniques.
Meeting these new requirements presents both opportunities and obstacles. While Azure's neural multi-style training opens up possibilities to customize voice characteristics—potentially allowing for unique brand voices or nuanced audiobook narration—studios face the challenge of capturing the full range of human speech and translating those complexities into the AI models. The quest to replicate natural human speech in AI-generated voices is an ongoing pursuit, requiring a delicate balance between customization and maintaining a semblance of authenticity. Studios are forced to reassess how they design and implement voice projects to best leverage these new AI capabilities while striving to maintain a level of realism.
Professional recording studios, when working with voice cloning technologies like Azure's Custom Neural Voice, are encountering a new hurdle: the need for 300 distinct spoken phrases for training a truly convincing synthetic voice. This requirement stems from the complexity of human speech, which involves a wide range of phonetic and rhythmic nuances. To capture these nuances accurately, models need a substantial dataset.
Interestingly, these 300 utterances typically amount to about 10-20 minutes of recorded audio. This duration is crucial, as it allows the model to grasp the speaker's individual vocal characteristics, including tonal qualities and subtle inflections, rather than just relying on broad statistical patterns. The quality of these recordings is paramount. If the audio is unclear or suffers from background noise, the synthesized voice may exhibit undesirable distortions or inconsistencies. This highlights the importance of a controlled, professional recording environment.
While capturing a speaker's voice is a significant step, true voice cloning goes beyond replicating vocal qualities. It involves capturing the nuances of prosody—the rhythm and intonation of speech. Emotional variation adds richness and realism, but models that solely focus on pitch and tone may struggle to convey authentic emotional depth in the synthesized speech.
Fortunately, advancements in voice cloning allow for capturing diverse accents and dialects, provided the training data includes a representative sample of these variations. This adaptability makes it possible for a single model to generate voices that sound authentic across different linguistic backgrounds. However, this requires careful attention during data preparation.
One critical aspect is the coverage of phonemes—the smallest units of sound that distinguish words. For natural-sounding synthetic voices, the training dataset should encompass a broad range of these phonemes. If certain phonemes are underrepresented, the model might struggle to accurately recreate less common words or phrases, leading to potentially awkward or unnatural-sounding speech.
Deep learning has enabled the development of models that can better understand the context of speech, meaning the synthetic voice can adapt its delivery based on the conversation or narrative. This feature has significant benefits for applications like voice assistants and audiobooks, where natural-sounding interactions are vital.
Transfer learning techniques are also gaining prominence. These methods can leverage existing models and adapt them to new voices with less data, potentially speeding up the voice cloning process. Studios can potentially create compelling voice clones with limited utterance datasets.
It's intriguing to consider that synthetic voices, once trained, aren't static. Their quality can deteriorate over time, especially if not periodically updated with fresh training data. This is due to the ever-changing nature of language and speech patterns, with new words and pronunciations constantly emerging.
Real-time voice synthesis is another exciting area of development, enabling applications where synthetic speech can be generated instantly. This capability is highly relevant for interactive gaming and real-time translation but puts new demands on the processing power and capabilities required in the recording studio environment. It's clear that the field of voice cloning is evolving rapidly, and professional recording studios will need to continue to adapt to the new technical requirements of these advancements.
How Azure AI Services Are Revolutionizing Voice Clone Consistency in Audio Production Studios - Quality Control Methods in Custom Neural Voice Audio Production
The creation of high-quality custom neural voices relies heavily on robust quality control methods. The effectiveness of the Azure Custom Neural Voice (CNV) service is strongly tied to the quality and consistency of the training data used to build the voice model. This training data needs to capture a wide array of vocal characteristics, including subtle phonetic differences and a spectrum of emotional expression. To avoid issues with distorted or unnatural-sounding audio, it's crucial to incorporate thorough quality checks during the voice design and the model deployment phases. This is particularly critical for audio applications such as audiobook production and podcasts where listeners demand a natural and enjoyable experience.
Furthermore, a persona brief document can serve as a valuable guide, helping to ensure the final synthetic voice closely aligns with the desired brand identity or character traits required for the specific audio project. This careful alignment further enhances audience engagement by providing a more tailored listening experience. As advancements in AI voice cloning continue, ongoing quality control will become even more vital. This commitment to meticulous quality assurance will ensure that future voice cloning technologies achieve the highest possible levels of authenticity and relatability, creating a seamless and natural listening experience.
Custom Neural Voice (CNV) within Azure's Speech service has revolutionized voice cloning and synthetic voice generation by leveraging neural text-to-speech technology. However, the quality of these synthetic voices hinges on the quality and consistency of the training data. It's become increasingly evident that simply recording a few voice samples is not enough; achieving truly natural-sounding speech requires a more rigorous approach.
For instance, achieving a high-quality synthetic voice necessitates professional audio recordings with exceptional signal-to-noise ratios. Anything less can significantly degrade the voice's clarity, making the use of high-quality microphones and controlled recording environments essential. To ensure the generated voice encompasses a broad range of sounds, the training data needs to be diverse enough to cover a language's complete set of phonemes. This helps prevent the synthetic voice from sounding odd or unnatural when attempting to articulate less common words or sounds.
Furthermore, we've learned that capturing the nuances of human speech – like prosody, or the rhythm and intonation of speech—is critical. Neural voice models that are not adept at understanding prosodic variations may fall short, generating voices that sound overly robotic or fail to convey natural human emotions. Fortunately, speaker normalization techniques are employed during the training process to address inconsistencies across different audio recordings. These techniques ensure that the synthetic voice maintains consistency in volume and other factors, irrespective of the recording conditions.
The beauty of neural networks, however, is that they allow for a dynamic approach. They can adapt their output based on the surrounding context. For example, in applications like conversational AI or narrating stories, this capability gives the synthetic voice the ability to change its style and delivery. This enhances the listener's experience, making the conversation or narrative more engaging.
But even the most advanced models require ongoing maintenance. Without regular updates to the training data, the synthetic voice can gradually degrade in quality. Language, after all, is dynamic, and new words and pronunciations emerge over time. Periodically revisiting and updating the dataset is a crucial part of keeping a synthetic voice current and relevant.
Interestingly, CNV technology opens doors for brands to develop unique synthetic voices that are tailored to their specific identity. Organizations are now empowered to craft a memorable audio experience across a wide variety of media formats by forging their own recognizable voice using Azure's tools. The potential for creating unique brand voices and personalized customer experiences is particularly noteworthy.
Moreover, neural networks have made it possible to build synthetic voices that can seamlessly switch between different languages. Imagine a single voice narrator effortlessly switching between English and Spanish in an audiobook or podcast. This capability is invaluable for reaching global audiences.
Recent advancements in CNV have also brought about the capability for real-time voice generation. Applications like live translation and interactive gaming are now within reach. However, this advancement demands greater computational resources and advanced algorithms to ensure that the dynamically generated voice remains fluid and coherent.
Finally, the introduction of data layering in certain training processes is a promising technique. The approach involves training models on basic voice characteristics before fine-tuning them with additional utterances. This method reduces the amount of training data needed to create a compelling voice while allowing for more adaptable voice outputs.
The advancements in custom neural voice synthesis are undeniably remarkable. As the technology continues to evolve, we can anticipate a future with increasingly sophisticated and realistic synthetic voices, opening up new and exciting possibilities in the realms of entertainment, education, and beyond.
How Azure AI Services Are Revolutionizing Voice Clone Consistency in Audio Production Studios - Voice Pattern Recognition Analysis in Modern Studio Settings
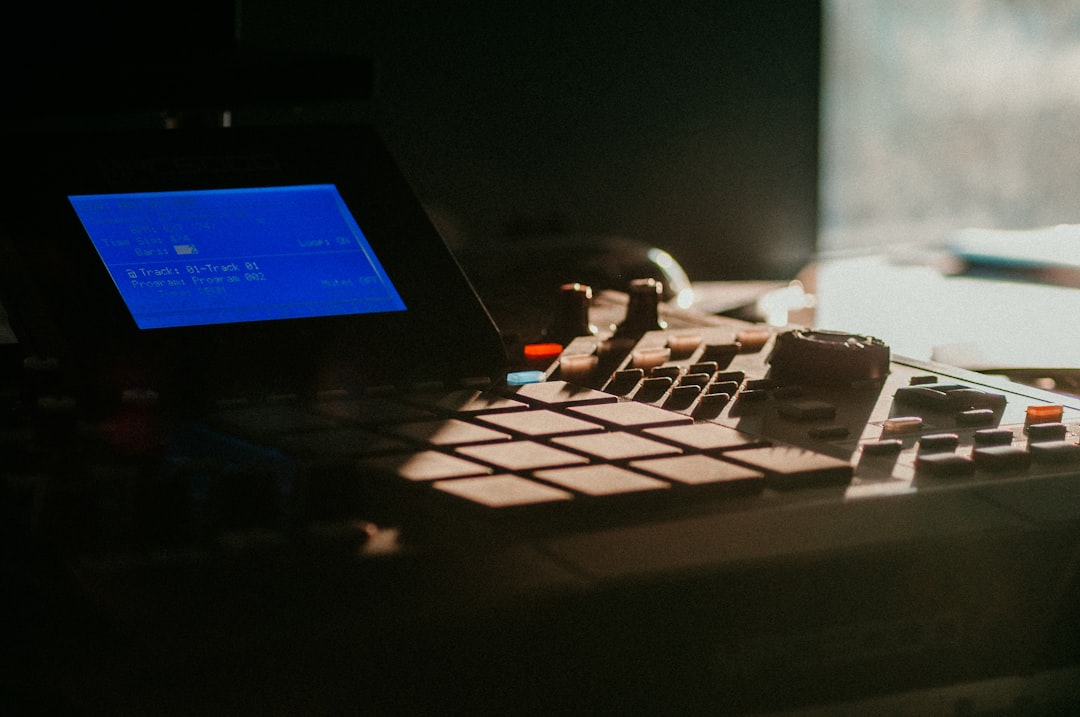
Within the contemporary audio production landscape, the analysis of voice patterns is gaining prominence, especially in the context of voice cloning and related applications. Azure AI's advancements in this area offer studios powerful tools to analyze and replicate vocal patterns with precision. This capability allows for a deeper understanding of subtle nuances within a speaker's voice, leading to more natural-sounding synthetic voices. The ability to generate voices that sound authentic is critical for diverse applications, such as podcast creation and audiobook narration.
While the potential for generating highly realistic synthetic voices is exciting, challenges remain. Capturing the full spectrum of human vocal expression in a way that is both accurate and emotionally nuanced is still a work in progress. Striking the right balance between pushing the boundaries of what's technically possible and maintaining a level of sonic authenticity is a continuous challenge for those working in this field.
As the tools and technologies in this realm continue to evolve, the need for close scrutiny of the voice data used to train these AI systems is also increasing. The quality of the input data and the effectiveness of the processing methods employed are critical factors in achieving high-quality synthetic speech outputs. Professional recording studios and audio engineers will need to continue adapting their workflows to fully realize the benefits of these evolving tools while simultaneously preserving the integrity and richness of human vocal expression.
In the realm of modern audio production, Azure AI's capabilities are reshaping how we approach voice cloning and generating synthetic speech. One of the most fascinating aspects of this evolution is the focus on refining voice pattern recognition. For instance, capturing a wide array of phonemes – the fundamental building blocks of speech – is crucial. If a model's training data lacks a diverse range of phonemes, the resulting synthetic voice may struggle to accurately pronounce less common words, leading to unnatural or awkward speech patterns. It highlights the importance of carefully curating the training data to ensure the synthesized voice sounds as natural as possible.
Furthermore, the quality of the audio recordings themselves plays a significant role. Achieving a high signal-to-noise ratio is paramount, as even slight background noise can hinder the clarity of the synthetic voice. This requirement puts a premium on using high-quality microphones and recording environments optimized to reduce unwanted sounds. Professional studios are increasingly realizing the importance of achieving this high level of audio fidelity for consistent and convincing results.
Beyond simply replicating a speaker's voice tone, AI models are being designed to emulate emotions. This is a key factor for creating more immersive and compelling audio content, such as audiobooks and podcasts. However, models that haven't been trained on the nuances of prosody – the rhythm and intonation of speech – may struggle to deliver the emotional richness of human speech authentically. It's a challenge to bridge the gap between replicating tone and effectively communicating human feelings through synthesized speech.
Interestingly, contemporary voice clones are not static; they can adapt their delivery style depending on the surrounding context. This ability to dynamically tailor the output based on the specific application – like conversational AI or narration – enhances the listener's engagement. Imagine a synthetic voice adapting its delivery to better match the tone of a story being told. It's a capability that makes synthesized speech feel more natural and dynamic.
The emergence of real-time voice synthesis expands the possibilities of voice cloning even further. Imagine applications like instant translation or interactive gaming relying on synthetic voices generated on the fly. While a promising development, it presents new hurdles. Advanced algorithms and considerable processing power are needed to maintain fluidity and coherence in the synthetic speech as it's dynamically generated. It's an area pushing the boundaries of what's possible.
It's also clear that the models need continuous maintenance. As language evolves, new words and pronunciations emerge. This necessitates regular updates to the model's training data to prevent the synthetic voice from becoming outdated and sounding unnatural. It's an ongoing process that ensures the voice stays fresh and consistent.
Tools like speaker normalization techniques help maintain a uniform output across recordings. By compensating for inconsistencies in volume and tone, they ensure that the resulting voice sounds the same regardless of the original recording conditions.
Some recent work has focused on layering the training data. By training a model on basic voice characteristics and then refining it with additional, more specific utterances, it can create more versatile voices with less training data. It signifies a move towards faster and more adaptable model development.
It's also exciting to see how voice cloning technology is empowering brands to cultivate their own unique synthetic voices, forging a memorable audio identity. It's a way to tailor the audio experience across a range of media, allowing for more personalized and effective audio branding strategies. The ability to represent a variety of accents and dialects also makes the technology more useful when trying to reach a wider audience with a global presence.
The field of voice cloning, through the lens of Azure AI, is constantly evolving. As the technology matures, we'll likely see more refined and realistic synthetic voices, with the potential to transform how we interact with audio content in entertainment, education, and countless other areas. It's an exciting space to be watching.
How Azure AI Services Are Revolutionizing Voice Clone Consistency in Audio Production Studios - Cross Platform Integration Between Azure Speech SDK and DAW Software
The ability to integrate Azure's Speech SDK with various Digital Audio Workstation (DAW) software marks a notable step forward for audio production, especially when it comes to voice cloning. This cross-platform compatibility allows for smooth integration of Azure's speech-related features into established workflows, ultimately improving the quality and consistency of synthetic voices used in fields like podcasting and audiobook creation. The SDK's flexibility, including support for several programming languages and real-time audio transcription, empowers developers to design adaptable and high-performance applications that cater to the changing demands of audio production. As recording studios adjust to these developments, they can leverage Azure's AI tools to refine their practices and craft more lifelike synthetic voices. While this is promising, the process of capturing the subtleties of human speech remains challenging. This highlights the importance of carefully handling data and rigorously maintaining quality control throughout the process.
The Azure Speech SDK offers interesting possibilities for integrating with various Digital Audio Workstation (DAW) software, potentially opening doors for enhanced audio production capabilities, including voice cloning. It's available across a range of programming languages and platforms, which makes it a flexible tool for developers working on speech-related applications. While Azure's speech-to-text feature enables real-time transcription, which is handy for voice command recognition in voice assistant applications, it also raises questions about the accuracy and potential for errors in those transcriptions.
Azure's collection of AI services, such as Azure Speech and Azure OpenAI, provides adaptable APIs and machine learning models, paving the way for the development of sophisticated voice bots and other audio-centric applications. When it comes to voice assistants, Azure relies on server-side verification to enhance activation accuracy. While this approach is different from device-only solutions, there are trade-offs to consider regarding internet connectivity and responsiveness.
Azure AI Speech encompasses some impressive features, such as real-time translation and transcription for various languages. This is useful for audio production that involves multilingual teams or projects that need to cater to global audiences. One of the nice things about the SDK is that it can handle audio from different sources – local devices, files, Azure storage, and a variety of input/output streams – making it suited for a wide range of scenarios.
Recent advancements in Azure AI Speech include text-to-speech (TTS) avatars and a feature called Personal Voice. These additions provide more options for personalization in audio production and allow creators to refine the sounds of their synthesized voices. Azure AI Studio provides a collaborative hub for managing projects and deploying AI models, which could prove helpful in smoothing out integration with audio production applications.
The role of Azure AI services in improving the consistency of voice clones is a critical aspect, particularly for professional audio studios aiming for high-quality outputs. These studios must aim to maintain the intended voice characteristics of the audio they create. It's crucial for building trust with listeners and creating a unified auditory experience. While the pursuit of incredibly realistic-sounding voices has shown some progress, the current technology still faces the challenge of capturing the subtleties of human speech, including emotional nuances and various speech patterns. It's a space to keep a close eye on, to see if the advances keep pace with the demands of diverse audio production uses.
However, challenges remain, particularly when it comes to latency in real-time audio processing. If the processing isn't fast enough, there can be lags during recording or playback. The compatibility of different audio formats used in DAWs and the output from the Azure Speech SDK can also require careful management and potential conversions. There's a potential for memory and processing bottlenecks when using the Azure services, especially if the hardware isn't sufficient. This trade-off must be factored into the workflow.
The tools within the SDK allow engineers to adjust features like pitch, speed, and modulation in real time, which is handy when producing audio with varied vocal performance. Developers can create their own custom plugins that expand the functionality of the SDK within DAWs. The ability to maintain consistent features and voice quality across multiple DAWs and platforms is an asset.
Further, speech analysis features integrated into the SDK can help with quality control and provide real-time feedback on synthesized audio. Creating custom voice instances tailored to individual projects or brands is another possibility. Azure's AI also allows for creating context-aware synthesis, where the voice adapts to the overall narrative or content, potentially improving engagement. Furthermore, the localization of content is potentially easier because the tools support multiple languages and accents. These functionalities are useful for creators looking to expand their reach into international markets. Overall, as the Azure Speech SDK continues to evolve and develop, it could play a more important role in diverse audio production contexts.
How Azure AI Services Are Revolutionizing Voice Clone Consistency in Audio Production Studios - Studio Recording Techniques for Multilingual Voice Model Training
Modern audio production increasingly relies on sophisticated studio techniques to train effective multilingual voice models. Building a strong custom neural voice necessitates capturing high-quality audio with minimal background noise, demanding precise recording practices. A minimum of 300 unique spoken phrases is typically required to train these models effectively, ensuring they can capture the subtleties of human speech, such as consistent volume, pitch variations, and the subtle expression conveyed through our voices. Azure AI services play a key role in this process by allowing models to learn multiple languages and dialects with automatic language detection capabilities, adding further complexity while expanding the scope of what can be achieved with voice cloning. Adapting to these evolving requirements is critical for professional recording studios as they seek to create realistic and flexible synthetic voices for uses like audiobook creation or podcasting, a testament to the ongoing challenges and possibilities in the realm of AI-powered voice generation.
Developing truly effective multilingual voice models for AI applications like audiobook production or podcast creation requires careful consideration of several factors, starting with the training data itself. A diverse range of sounds, or phonemes, needs to be captured across the languages the model will support. Otherwise, the generated voice may struggle with less common words or sounds, resulting in an unnatural, almost robotic delivery.
Furthermore, replicating the natural rhythm, stress, and intonation of human speech – what we call prosody – is key. If the AI model hasn't been trained on these subtle aspects of speech, the generated voice can sound flat and emotionless, a far cry from the rich, expressive voice needed for many compelling audio narratives.
The quality of the recordings used to train these AI models is also crucial. High-quality audio, with a good signal-to-noise ratio (ideally 20 dB or higher), is essential. Otherwise, any background noise or inconsistencies in the recordings can directly affect the clarity and naturalness of the synthesized voice. Listeners tend to be quite sensitive to these imperfections, so the recording quality needs to be on point.
Fortunately, we're seeing progress in allowing synthetic voices to adapt their delivery based on the surrounding context. This ability to change tone and style, as needed, is particularly beneficial for dynamic content like audiobooks and conversational AI applications. It gives the synthetic voice more natural flow and makes it feel more integrated into the narrative.
However, the integration of real-time voice synthesis presents challenges. For applications requiring instantaneous speech generation, like live translation, even minor delays (latency) can significantly impact the user experience, disrupting the natural flow of interactions. It underscores the need for robust hardware and efficient algorithms for optimal performance.
Researchers are experimenting with innovative data layering techniques to improve training efficiency. The idea is to start with a model trained on fundamental voice characteristics and then refine it with more specific examples. This approach potentially reduces the amount of training data required to create high-quality and adaptive synthetic voices.
Maintaining a voice model over time can also be tricky. Just as human language is constantly changing, so are the patterns of human speech. If we don't continuously update the model with new training data, the synthetic voice can start to sound stale and out of touch with contemporary speech patterns. This highlights the ongoing maintenance and adaptation required for long-term quality.
Techniques like speaker normalization are valuable for ensuring consistency across different recordings. These techniques can compensate for variations in volume and tone, ensuring that the synthetic voice retains a uniform character regardless of the specific conditions in which the original recordings were made.
One of the most exciting developments in this field is the ability to effortlessly switch between languages within a single synthetic voice. This cross-language capability expands the potential audience for content created with AI-generated voices, making it possible to engage with diverse groups of listeners without sacrificing the consistency of the voice itself.
The Azure Speech SDK, meanwhile, provides valuable feedback mechanisms, including speech analysis features. These tools are incredibly useful for quality control, allowing engineers to fine-tune their voice models in real time. It emphasizes the importance of ongoing monitoring and refinement to achieve truly high-quality synthetic voices.
In conclusion, as this technology continues to mature, we can anticipate increasingly sophisticated and lifelike synthetic voices. This has major implications for a wide array of applications in audio production, entertainment, education, and beyond. It's a dynamic area to watch, and the potential for advancement in this field is vast.
Get amazing AI audio voiceovers made for long-form content such as podcasts, presentations and social media. (Get started for free)
More Posts from clonemyvoice.io: