Fact-Checking Claims in Voice AI Advancements
Fact-Checking Claims in Voice AI Advancements - Spotting an Artificial Voice in Audiobook Production
Detecting whether an audiobook narrator is artificial or human remains a nuanced task, one that becomes trickier as voice synthesis technology advances. Gone are many of the obvious robotic tells of years past; today's AI voices often achieve a remarkable level of fluency. However, subtle differences can still exist, sometimes manifesting as an unnatural pacing in complex sentences, an inconsistent emotional arc over longer passages, or an inability to imbue words with the specific, layered meaning a human performer instinctively applies. The discussion isn't solely about sonic fidelity; it also involves questioning whether these highly realistic voices truly capture the interpretative artistry of narration, and if listeners are consciously or unconsciously aware of these distinctions, or simply prioritize clarity and ease of listening.
Okay, digging into the technical aspects of spotting artificial narration in audiobooks presents some interesting challenges and reveals the current limitations researchers are grappling with. From an engineering perspective, several tell-tale characteristics often stand out:
A prevalent issue is the tendency for AI voices to exhibit a rigid, almost metronomic speaking rate. Unlike a human narrator who naturally varies pace for emphasis, dramatic effect, or simple conversational rhythm, synthetic voices can maintain an unnervingly consistent tempo. This uniformity in syllable duration and pause timing is a key area for analysis when trying to identify non-human performance.
Furthermore, the nuanced use of pitch to convey subtle emotion remains a difficult hurdle for AI. While models can hit basic intonation patterns, replicating the fine-grained frequency shifts and vocal colorations that a skilled human narrator uses to express complex feelings over a lengthy narrative often falls short. The result can sometimes sound technically correct but lacking in genuine warmth or emotional depth.
Curiously, the audio post-production applied to AI-narrated books is often quite sophisticated, employing heavy dynamic processing and mastering. While partly intended to polish the output and mask imperfections, this can sometimes result in an audio signal that sounds almost *too* perfect or artificial in its lack of natural variation – or, conversely, it drives efforts to deliberately introduce synthetic 'imperfections' to mimic human recordings.
Pronunciation of words outside the AI's core training set, particularly specialized or fictional terms, can also be a giveaway. Narrating a complex text often requires handling proper nouns, technical jargon, or unique vocabulary that a human would research. Current AI models can stumble over these, producing inconsistent or incorrect pronunciations that interrupt the listening flow in a way less likely with a professional human voice actor.
Lastly, the frontier is shifting beyond just the voice waveform. Engineers are exploring methods for AI to synthesize not just the voice but also the acoustic environment – imitating real-world room reverb or subtle background ambiance instead of just recording in a controlled environment. Distinguishing this synthetically generated environmental realism from actual recording conditions adds another layer of complexity to the detection problem.
Fact-Checking Claims in Voice AI Advancements - Evaluating AI Voice Quality for Podcasting Purposes
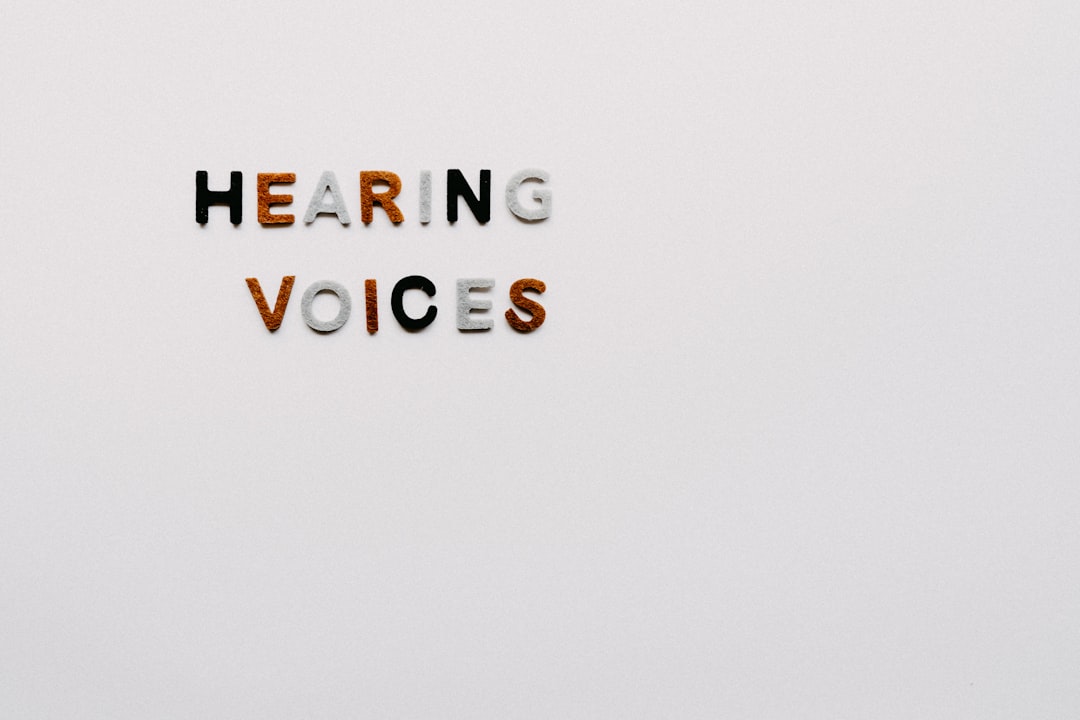
As AI voices become more prevalent in podcast production by May 2025, assessing their actual quality for this medium is increasingly necessary. It's not just about whether the voice sounds clear or understandable; true evaluation requires considering how effectively it serves the specific purpose of a podcast. This involves looking at factors beyond simple fidelity, such as the ability to convey genuine enthusiasm or navigate the unpredictable flow of conversation typical in many formats.
While synthetic voices are remarkably capable of delivering scripts with accuracy, replicating the subtle cues that human hosts use to connect with an audience remains a significant hurdle. Can an AI voice truly build rapport, maintain listener attention through shifts in tone and energy, or handle the organic imperfections and spontaneity that lend authenticity to a podcast? Often, while the sound itself might be technically clean, there can still be a perceived lack of human presence or the natural variation that prevents monotony over extended listening. The tools are advancing rapidly, yet the qualitative leap to consistently deliver compelling, nuanced performance suitable for diverse podcasting styles is still a point of critical consideration.
Shifting focus specifically to AI voice output intended for podcast distribution introduces a distinct set of considerations for evaluation researchers. Unlike the often demanding fidelity expectations of audiobook consumers, preliminary observations suggest a higher degree of listener tolerance for certain sonic artifacts or minor performance irregularities within the more conversational and immediate context of podcasts. It appears the primary driver in podcast consumption is content delivery and connection, potentially lowering the perceptual threshold for "unnaturalness" compared to immersive narrative formats.
Furthermore, the notion that a single "best" AI voice exists for podcasting seems fundamentally flawed from an engineering perspective. Effective evaluation necessitates considering the intended genre and audience. The characteristics required for a synthetic voice to be perceived as credible or engaging in a documentary podcast differ significantly from those needed for a comedic or interview-based format. Metrics must therefore evolve to assess a model's capacity for stylistic variance and appropriateness across this diverse landscape.
Interestingly, the perceived naturalness of a synthetic podcast voice can be significantly influenced not just by the underlying model's capabilities, but by the strategic design of the input text itself. Incorporating elements typically edited out of formal narration, such as carefully placed micro-pauses, hesitations, or even non-lexical sounds like subtle 'ums' or 'ahs', seems to boost the sense of a genuine, spontaneous human delivery to listeners. This highlights the complex interplay between voice synthesis engine quality and upstream text-processing or script-engineering choices in achieving a desired perceptual outcome.
Another intriguing area is the exploration of integrating sections utilizing voice cloning or distinct synthetic profiles within a single podcast episode. Early anecdotal feedback suggests that introducing vocal variety by, for instance, cloning a host's voice for specific segments like introductions or advertisements, or using different synthetic voices for interview questions versus answers, can effectively break up monotony and potentially enhance listener engagement by providing textural and prosodic shifts throughout the audio experience.
Finally, a peculiar challenge arises in post-production: aggressive application of common audio processing techniques, particularly noise reduction algorithms. While aiming for clarity, overzealous noise gating or spectral subtraction on synthetic voices can paradoxically strip away subtle nuances or even intentionally synthesized environmental cues, resulting in an audio signal that sounds unnaturally clean, sterile, and ultimately *less* like a voice recorded in a real acoustic space. Evaluating this trade-off between perceived clarity and the loss of natural ambient realism becomes critical.
Fact-Checking Claims in Voice AI Advancements - Difficulties in Verifying AI Generated Sound
The ongoing evolution of artificial intelligence in creating sound presents considerable hurdles when trying to confirm its genuine nature. The speed at which voice synthesis technology is advancing means the output frequently becomes indistinguishable from human speech, posing a significant challenge for listeners attempting to identify its origin. While faint characteristics might theoretically hint at its artificiality, the overall high fidelity now achievable often serves to obscure these potential indicators. Adding to this complexity are the layers introduced by audio production processes, which can further confound efforts to distinguish between synthetic and authentically captured sound. Navigating this environment highlights a growing necessity for robust procedures to evaluate and confirm the provenance of AI-produced audio.
Delving deeper into the specifics of discerning AI-generated sound reveals that the challenge has moved far beyond merely spotting obviously robotic voices. For a researcher sifting through audio, particularly as synthesis models grow more sophisticated by May 2025, the complexities lie in identifying artificiality that mimics highly granular or even undesirable human characteristics. Here are some points illustrating this difficulty:
Engineers are finding that modern generative models have become unnervingly adept at synthesizing sounds traditionally associated with human vocal tracts and their use in speech, often perceived as imperfections. This includes incredibly realistic replications of throat clearing, lip smacking, and other subtle mouth noises. Previously, their absence or unnatural uniformity might signal synthetic origin; now, their convincing inclusion makes it difficult to rely on these natural sounds alone for identification. Their synthetic addition is sometimes intended, curiously, to increase perceived engagement or realism, blurring the lines further.
Beyond just controlling pitch and volume, the sophisticated models now incorporate granular manipulation of the audio spectrum. This means they can simulate the very subtle shifts in frequency content – the timbre or 'color' of the voice – that a human speaker naturally uses to convey nuanced emotions or emphasis. Detecting whether these specific spectral changes are organic or computationally generated is becoming a significant analytical hurdle, as the output often convinces the ear that a 'feeling' is present in the audio.
Furthermore, the frontier has expanded to replicating not just standard speech, but highly idiosyncratic vocal behaviors. Certain advanced models are demonstrating the capability to learn and convincingly emulate mild speech impediments, such as subtle stutters or lisps, from reference audio. This development complicates traditional detection approaches that might flag 'perfect' or 'uniform' speech as artificial, as synthetic voices can now deliberately incorporate what would once have been considered human speech flaws.
The synthetic creation of acoustic environments alongside the voice is no longer limited to applying generic reverb in post-production. We're seeing models capable of generating and overlaying highly specific and customizable background sounds – anything from a bustling crowd to the distinct acoustics of a specific room – that are then made to acoustically interact with the synthetic voice in a plausible way. The difficulty is heightened by algorithms that attempt to predict or produce ambient situations that *perceptually match* the synthesized voice's characteristics, making it exceedingly hard to verify if the voice was genuinely captured in that specific simulated space.
Perhaps counterintuitively, a growing difficulty is the ability of current advanced algorithms to intentionally synthesize the characteristics of a "bad" recording. This isn't about polishing poor human audio; it's about generating audio that *sounds* like it was captured on a cheap microphone, in a resonant or noisy room, or with signal distortion. These models can reproduce microphone artifacts, poor gain staging effects, or room acoustics from scratch. Identifying whether such perceived low quality is genuine human recording imperfection or deliberate synthetic degradation poses a significant challenge to verification methods that often look for pristine or unnaturally clean signals as indicators of AI.
Fact-Checking Claims in Voice AI Advancements - Assessing Claims About Emotion and Nuance in Synthetic Speech
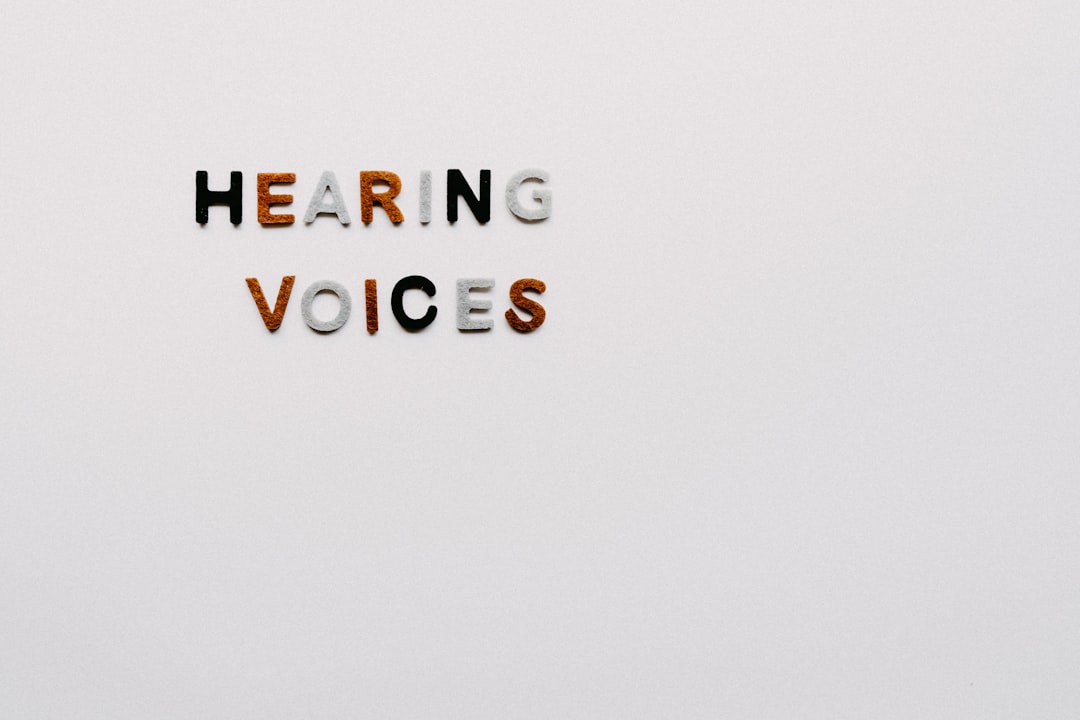
Assessing the genuine conveyance of emotion and subtlety in synthetic speech remains a significant area of focus and challenge as voice AI technology continues its rapid evolution towards May 2025. While synthetic voices have achieved impressive levels of clarity and naturalness in delivering text, the capacity to imbue language with nuanced emotional depth – the kind a human speaker uses to convey layers of meaning or feeling – is still subject to critical examination. Evaluating claims about an AI voice's ability to perform expressively goes beyond simply measuring intelligibility; it requires assessing its capability to embody different emotional states convincingly and consistently across varying contexts and lengths of speech. Researchers grapple with developing robust methods to measure this perceived emotional quality, acknowledging the inherent difficulty in objectively quantifying subjective expressiveness. The gap isn't merely in hitting the right intonation patterns, but in delivering performances that listeners genuinely perceive as emotionally resonant or appropriately nuanced for specific applications like compelling audiobook narration or engaging podcast hosting. Claims of 'emotional AI' voices warrant careful scrutiny; while technical proficiency is undeniable, replicating the full spectrum of human vocal expressiveness and its impact on listener perception is a hurdle yet to be definitively cleared.
Exploring claims about how well synthetic voices capture human emotion and subtle nuances is a particularly complex part of evaluating AI voice advancements. It goes well beyond simply assessing if the voice sounds clear or understandable; it delves into the very fabric of human communication, where much meaning is conveyed through how something is said, not just the words themselves. From an engineering perspective, the challenge lies in recreating not just the sound, but the *system* of paralinguistic cues humans instinctively use.
It's fascinating to observe the directions research is taking by May 2025. Engineers are tackling increasingly subtle vocal phenomena. For instance, some advanced models appear capable of reproducing things like vocal fry – that low, creaky sound often heard at the end of sentences. While perhaps undesirable in some formal contexts, its convincing inclusion in synthesized speech is sometimes explored as a technique to enhance perceived naturalness, making the boundary between human and machine production harder to discern for a casual listener in, say, a conversational podcast or audiobook narration.
Similarly, there's significant effort being put into synthesizing believable breathing patterns. This isn't just adding a generic inhale sound; the work involves attempting to correlate breath timing and intensity with the simulated emotional state or phrasing of the speech. The idea is that these subtle respiratory sounds, often unconsciously perceived by humans, contribute to the overall sense of a live, feeling presence, making generated audio feel more like a genuine human performance in a studio or conversational setting.
Beyond simply adjusting overall speed, engineers are now designing models that try to tie speaking rate dynamically to the expressed emotion. We're seeing attempts to synthesize voices that naturally accelerate delivery during moments designed to sound exciting or anxious, or slow down for somber or reflective passages. This goes beyond basic prosody control and aims for a more organic rhythm, attempting to mimic the natural ebb and flow of human emotional cadence, a key element in captivating narration or compelling podcasting.
A perhaps counterintuitive area of development is the intentional incorporation of minor speech disfluencies or "imperfections." Models are being developed that can deliberately inject features like subtle "ums," "ahs," or brief hesitations into the output. While this might seem counterproductive, the thinking seems to be that these very human elements, often edited out of professional recordings but common in spontaneous speech, can ironically boost the perception of authenticity and conversational naturalness for listeners, particularly in podcast or interview formats.
Moreover, there's intriguing research into models that attempt to learn the *expression* of emotion in one language and apply that understanding to synthesize emotional speech in another. This suggests a theoretical decoupling of emotional prosody from specific linguistic structures, implying that models are beginning to grasp more abstract concepts of how emotion manifests acoustically across different human communication systems. It raises interesting questions about how universal or culturally specific our expressions of emotion truly are, and how AI might navigate that complexity.
More Posts from clonemyvoice.io: