Breaking Language Barriers How AI Voice Cloning Achieves Natural-Sounding Multilingual Speech in 2025
Breaking Language Barriers How AI Voice Cloning Achieves Natural-Sounding Multilingual Speech in 2025 - Voice Cloning Reshapes Anime Dubbing As Dragon Ball Series Retains Original Voices Across 17 Languages
Voice cloning technology is reshaping how anime is localized for international viewers. A prime example is the enduring Dragon Ball series, where this approach allows the original vocal performances, the very sound of the characters fans know, to be recreated and used across numerous languages, reportedly up to 17 different versions. This goes beyond just speaking the translated script; the aim is to mimic the specific tone, cadence, and emotional qualities of the initial performance, theoretically creating a more consistent and familiar character presence globally.
While the capability to retain these recognizable voice profiles offers a compelling way to bridge language gaps and connect audiences more intimately with beloved series, replicating the full, subtle range of human emotion and performance purely through AI remains a complex technical and artistic challenge. Variations in quality and nuance can still occur across different languages and characters. Looking ahead, this form of AI integration isn't limited to animation; it has significant implications for broader audio production, potentially allowing creators to generate consistent character voices for multilingual audiobooks or layer diverse vocal tracks seamlessly in complex podcast narratives, democratizing access to high-quality localized audio content.
The application of AI voice cloning technology is notably reshaping the field of anime dubbing, exemplified by the Dragon Ball series' capability to retain a degree of its original vocal identities across numerous languages. This moves beyond simple translation, aiming to capture and replicate the characteristic sound of specific characters, allowing for a form of vocal continuity as content crosses linguistic borders. Observing the results, the systems demonstrate an ability to reproduce nuances in tone and attempt to mirror emotional inflections from the source material, enabling localized versions to maintain a closer sonic fidelity to the original performances across multiple languages. This capability extends the reach of the content, making character voices more recognizable globally. While this represents a significant technical achievement in sound reproduction and localization, the question remains whether perfect sonic replication across disparate linguistic structures truly transfers the full spectrum of an actor's original performance and emotional intent, or if it primarily clones a vocal profile. This ongoing challenge is a focus point for continued development and research in refining the translation of performance beyond just pitch and timbre.
Breaking Language Barriers How AI Voice Cloning Achieves Natural-Sounding Multilingual Speech in 2025 - Audio Book Production Time Drops From 6 Weeks to 3 Days Through Neural Voice Models
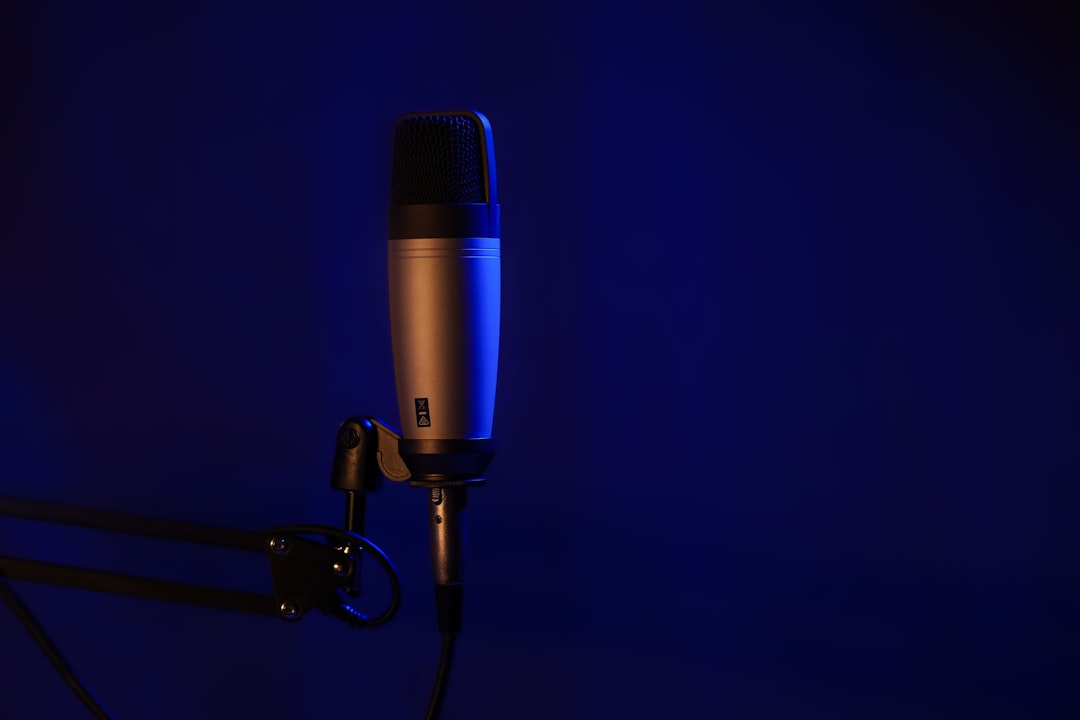
The timeline for producing audiobooks has shifted dramatically, now potentially taking just a few days instead of many weeks. This swift change is largely due to advancements in neural voice models capable of converting written text into spoken audio at scale. Beyond mere speed, these systems are increasingly producing narration that sounds natural and, importantly, can do so across various languages, significantly easing the process of reaching different audiences globally. The palette of available synthetic voices is also expanding considerably, offering creators numerous styles to choose from to fit their content. While the technology accelerates production and diversifies options, the challenge of perfectly replicating the subtle artistry and full emotional range a human narrator brings to a performance remains a key area for ongoing development and critical observation. Nevertheless, this shift holds significant potential for making audio content creation faster and more broadly achievable.
From an engineering standpoint, observing the trajectory of audiobook production timeframes presents a striking acceleration. What once typically demanded around six weeks to progress from finished manuscript to finalized audio is now, through the application of neural text-to-speech models, demonstrably achievable in as little as three days. This dramatic compression stems from the shift away from the traditional, largely manual process involving scheduled human narration sessions and subsequent painstaking audio editing and mastering.
The underlying technology here is the advancement of AI models trained to synthesize speech from text with increasing fidelity and naturalness. These systems, fed vast amounts of linguistic data, learn not just the pronunciation of words but also patterns of prosody – the rhythm, stress, and intonation that make speech sound human. Consequently, the generation of audio content becomes a significantly automated pipeline; once the text is prepared, the AI can process it far more rapidly than a human voice actor can record it.
Furthermore, these neural models are increasingly incorporating multilingual capabilities. Systems are being developed that can generate high-quality speech not just in one language, but across several, sometimes from a shared underlying representation. This capability inherently facilitates the creation of audio content for diverse linguistic markets, expanding the potential reach of an audiobook without requiring separate human narrators for each language. While the output is reaching impressive levels of naturalness and can mimic various voice characteristics, the technical challenge of truly replicating subtle emotional depth and nuance present in skilled human performance remains an active area of research and development. Nevertheless, this fundamental shift in production speed and multilingual capacity signifies a notable evolution in how audio content can be created and disseminated.
Breaking Language Barriers How AI Voice Cloning Achieves Natural-Sounding Multilingual Speech in 2025 - Podcast Networks Switch to AI Voice Translation After LibriVox Proves Success With 5000 Public Domain Books
Building on momentum seen in projects like LibriVox, which demonstrated the demand for widely accessible audio content by creating audio versions of numerous public domain works, podcasting platforms are increasingly integrating AI technology for multilingual delivery. A key development involves employing advanced voice generation techniques to translate podcast episodes while attempting to preserve the distinctive voice characteristics of the original host. This approach aims to connect creators with a much broader global audience by removing language barriers, offering content that feels more personal than conventional dubbed audio. While promising greater reach and a more authentic listening experience by retaining familiar voices across languages, faithfully replicating the full range of subtle vocal nuance and personality present in human-led conversational audio through AI remains a significant technical challenge and an area of ongoing refinement. This push towards AI translation marks a notable evolution in how audio content is localized and consumed worldwide.
A clear trend is materializing among podcast networks, pivoting towards harnessing AI for multilingual distribution of their content. This involves taking existing audio programs and rendering them accessible in various languages, not through traditional voice actors, but by employing sophisticated voice translation technology. The aim is to translate the spoken content while crucially preserving characteristics of the original speaker's voice – that specific tone, cadence, and identity listeners associate with a particular host or guest.
Observing early pilot implementations, such as initiatives by major audio platforms, reveals systems capable of generating translations that attempt to sound as though the original podcaster is speaking directly in another language. This relies on advanced AI models trained to understand and replicate subtle vocal patterns. The rationale behind this approach appears multifaceted: first, to significantly broaden a podcast's potential audience by removing linguistic barriers, and second, to maintain the personal connection and familiarity that listeners often develop with a specific voice, which can be lost in conventional dubbing processes.
This push towards AI-driven multilingual podcast delivery follows the demonstration of similar technologies' potential in other domains, such as expanding the accessibility of large collections of spoken-word content, like public domain literary works, to global audiences through automated translation and narration. It appears to serve as a compelling use case prompting wider adoption in the more dynamic format of podcasting.
From an engineering viewpoint, the task is complex. Beyond mere translation of words, the systems must analyze the prosody and vocal texture of the source audio and attempt to synthesize a translated version that mirrors these qualities convincingly in a different language. While impressive strides have been made in achieving natural-sounding output, the challenge of perfectly transferring nuance, subtle emotional states, or the spontaneity inherent in live or semi-scripted speech across languages remains a technical hurdle requiring ongoing refinement and careful evaluation of the resulting audio fidelity and emotional accuracy. The goal is not just understandable translation, but an authentic-feeling listening experience for diverse global listeners.
Breaking Language Barriers How AI Voice Cloning Achieves Natural-Sounding Multilingual Speech in 2025 - Language Learning Apps Adopt Personal Voice Mirroring To Help Perfect Native Pronunciations
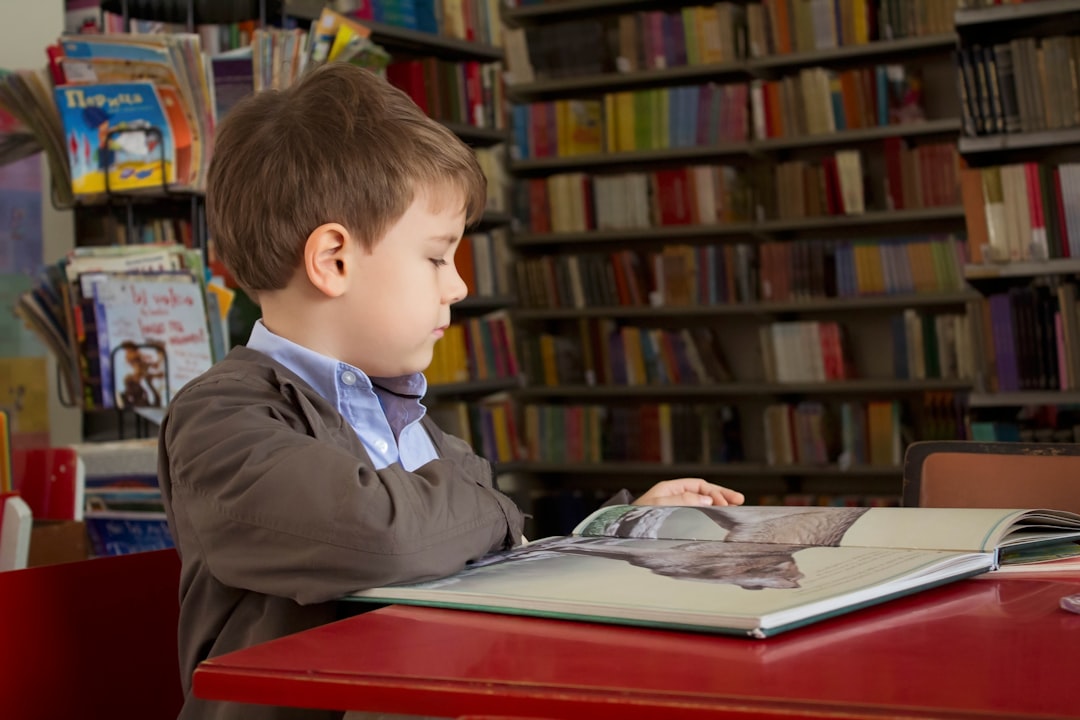
Language learning platforms are presently deploying sophisticated artificial intelligence to directly address the intricacies of pronunciation. A key innovation here involves integrating technologies capable of 'voice mirroring', allowing users to practice speaking and immediately receive analysis comparing their attempts to target native pronunciations. This feedback helps highlight subtle differences in accent, rhythm, and intonation. Leveraging the advancements in AI voice generation means these systems can produce natural-sounding examples of native speech for learners to emulate, moving beyond static recordings towards more dynamic practice scenarios. While these methods offer tailored feedback and opportunities for more immersive practice environments aiming for native-like sound, achieving the full, spontaneous character and emotional depth inherent in human conversation remains a complex technical frontier under active refinement.
Modern language learning platforms are increasingly integrating sophisticated AI capabilities specifically to target pronunciation, often a major hurdle for learners. The approach being developed moves beyond simple sound matching; it involves granular analysis of a user's spoken attempts. From an engineering standpoint, this means deploying advanced audio processing algorithms that dissect speech into its constituent parts, examining not just which phonemes are uttered but their acoustic properties like pitch contours, harmonic richness (timbre), and temporal pacing (rhythm). This detailed breakdown allows the system to build a profile of the user's specific vocal production for a given word or phrase.
These generated profiles are then held up against finely tuned models, typically derived from extensive datasets of native speaker recordings. The underlying neural networks are trained to identify the subtle differences and deviations in a learner's speech compared to the target language's standard pronunciations. This includes nuances in vowel qualities, consonant articulation, and crucially, the melodic structure of speech – intonation and stress patterns across sentences. The goal is to isolate precisely where the user's rendering diverges from a natural-sounding delivery.
The feedback provided is designed to be instantaneous. This real-time corrective mechanism appears to be a key component for learners tackling sounds or prosodic features that are alien to their native linguistic background. While the focus is primarily on phonetic and phonological accuracy, there is ongoing research and experimentation into getting the AI to analyze more complex aspects like overall expressiveness or emotional tone, although reliable interpretation and prompting in these areas remain technically challenging. The capacity to leverage insights from general speech synthesis and voice modeling research allows these systems to not only analyze but also provide examples of highly realistic, naturally intoned target speech for the user to mirror, moving beyond simplistic robotic voices. This development facilitates practicing not just isolated sounds but integrated phrases and sentences. The aim is to make the often-daunting task of perfecting pronunciation more interactive and less abstract, by providing immediate, specific comparison against a target sound model, thereby potentially reducing cognitive load during practice.
More Posts from clonemyvoice.io: