Get amazing AI audio voiceovers made for long-form content such as podcasts, presentations and social media. (Get started for free)
Exploring Voice Cloning Applications in XAI for Deep Reinforcement Learning at AAAI2024
Exploring Voice Cloning Applications in XAI for Deep Reinforcement Learning at AAAI2024 - Voice Cloning Techniques for Enhancing AI Explanations
Voice cloning is experiencing a surge in sophistication, particularly in how it can improve the way AI systems communicate their reasoning, especially within deep reinforcement learning. Modern approaches use deep learning to effectively capture the subtle features of human speech, allowing for the creation of very natural-sounding voices, even when only a small amount of audio is available. This has opened doors for richer and more individualized experiences for users in various settings, including education and interactive media like audiobooks or podcasts. The advancements in voice cloning technology are important as they can help improve communication and understanding when an AI explains its actions.
However, as this technology develops, we must be mindful of the ethical concerns that emerge. Issues of permission and the authenticity of the voices being created become increasingly critical. These considerations will significantly influence how voice cloning technologies are used in the field of AI in the coming years. The capability to replicate not only speech but also the emotional tone of a voice can radically change the way AI systems interact with humans, potentially leading to more transparent and easily understood AI explanations.
1. Voice cloning hinges on deep learning, with techniques like GANs proving especially effective in mimicking human speech. Surprisingly, even with limited voice samples, these systems can produce remarkably accurate imitations.
2. The field has seen advancements in synthesizing voice with rich emotional nuance. This ability to manipulate the emotional tone of a voice has interesting applications, like adding layers of expressiveness in audiobooks to enhance the narrative.
3. Significant strides have been made in refining the alignment of phonetic and prosodic features within synthetic speech, thanks to clever signal processing. The result is increasingly natural-sounding cloned voices, bridging the gap between artificial and human speech.
4. Podcast creation could greatly benefit from voice cloning. Imagine automating the process of generating content in various languages or dialects without the need for a vast network of voice actors.
5. The ethical dimension of voice cloning is a major concern. The possibility of unauthorized voice replication can fuel misinformation and identity theft, highlighting the urgent need for robust guidelines and regulations in the tech world to prevent misuse.
6. Modern voice cloning systems are exploring context-awareness. This means the generated speech could adapt to the topic or audience, enhancing the pertinence of the audio material.
7. "Voice morphing" is an intriguing area of research that aims to blend the qualities of different voices. This could result in new, unique voices that maintain a connection to the original speakers while simultaneously sounding completely fresh.
8. The emergence of real-time voice cloning systems is exciting. They enable the generation of synthetic voice in response to live interactions, potentially reshaping domains like customer service or enhancing interactive gaming experiences.
9. Personalized content is a burgeoning application of voice cloning. Audiobooks narrated in a familiar voice, for instance, can foster a stronger connection with the listener, potentially increasing engagement and enjoyment.
10. The capacity to recreate specific accents or speech impediments with voice cloning sparks discussions on accessibility. It prompts us to think about how adaptive technologies can better cater to individuals with speech challenges, fostering inclusivity in audio media.
Exploring Voice Cloning Applications in XAI for Deep Reinforcement Learning at AAAI2024 - Audio Visualizations in Deep Reinforcement Learning Models
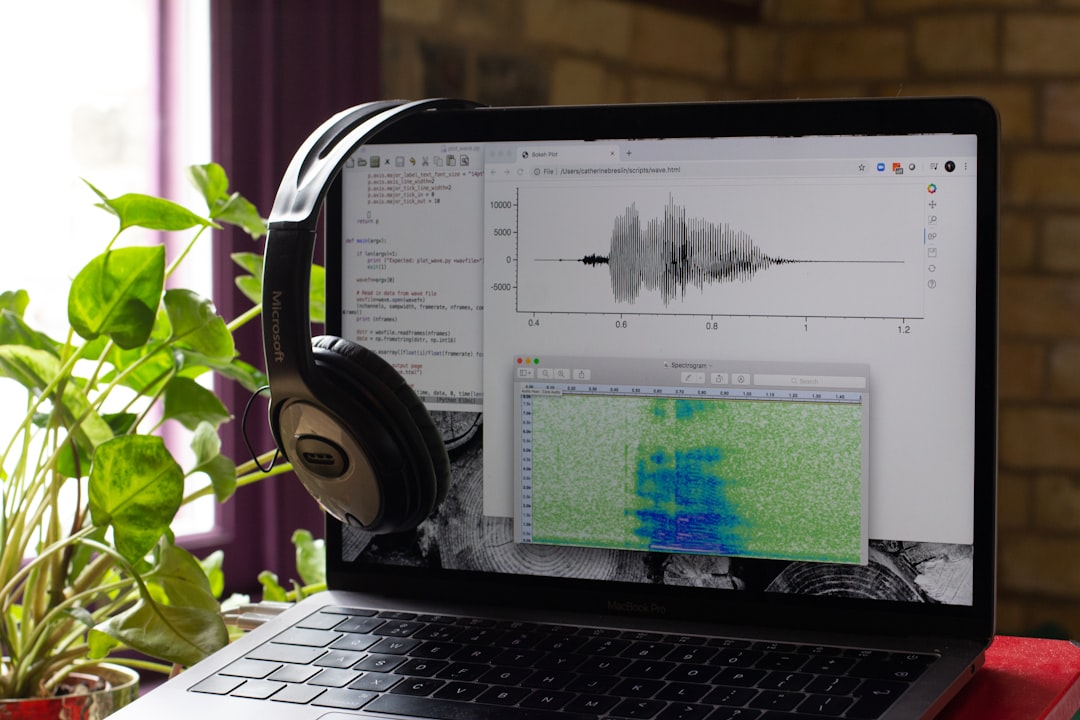
Audio visualizations are becoming increasingly important within deep reinforcement learning (DRL) models, especially in applications related to sound production, like voice cloning and audiobook creation. By combining audio and visual information, these models can learn more effectively and create richer experiences for users. For example, imagine audiobooks where visualizations enhance the story or podcasts where visuals provide additional context for listeners. This merging of modalities could lead to a more immersive and engaging experience.
However, integrating audio and visual data also presents significant hurdles. One challenge is that the datasets used to train these models may be unbalanced, potentially affecting the overall accuracy and reliability of the audio visualizations. Additionally, as voice cloning technology advances, there's a growing need to ensure the authenticity of cloned voices and address the potential for misuse.
The synergy between voice cloning and explainable AI within DRL is a particularly promising area of research. By visualizing the internal decision-making processes of AI systems alongside their generated speech, we can potentially create more transparent AI agents that effectively communicate their reasoning. This could pave the way for new applications, such as more engaging interactive experiences with podcasts or the development of audiobook technologies that seamlessly integrate both audio and visual components, resulting in more accessible and enriched content for a wider audience. While the possibilities are exciting, addressing these technological and ethical challenges is crucial to ensure that audio visualization within DRL is developed responsibly and benefits everyone.
Deep reinforcement learning (DRL) is having a profound impact on many AI fields, including areas like robotics and healthcare, by enabling autonomous systems to effectively interact with the world around them. This includes audio processing, where DRL models are being used to learn from sound input, leading to a wider range of applications. Deep learning techniques are being used to refine voice cloning tech, especially in text-to-speech (TTS) systems. However, there are challenges like uneven training data when identifying cloned voices which can affect things like voice authentication.
The intersection of audio and visual data in deep learning is becoming increasingly important. Research in deep audiovisual learning aims to combine the strengths of both audio and video to make tasks that were previously seen as single-modality challenges more effective. Audiovisual speech recognition (AVSR) is an area seeing lots of work and continues to face hurdles. Research on DRL applications related to audio has highlighted some areas where more investigation is needed, underscoring the potential for DRL to transform the field.
Voice cloning usually needs a lot of recordings from a single person, making it tricky to imitate voices outside of the trained data set. Audiovisual speech decoding is a major challenge researchers are trying to solve when building speech recognition systems. The combination of voice cloning and explainable AI (XAI) is emerging as an important research topic within the larger area of DRL and audiovisual applications.
It's quite interesting that visualizations using audio can give researchers a new way to understand how DRL models make decisions, especially when the data is complex. By using audio waveforms or spectrograms, researchers can get insights into model behavior. Audio visualizations are not just for pretty pictures; they can also pinpoint problems within a reinforcement learning model. By looking at specific audio patterns that might correspond to poor decisions, engineers can get a better grasp of how to refine model behavior.
In voice cloning, these visualizations could be key to improving how users perceive synthesized speech. They can expose the changing features of a synthetic voice, which could improve the listening experience overall. Unlike traditional visualizations, audio can engage users in a way that's quite unique. When you turn data into sounds, you're communicating information in a different way. This could be especially helpful when trying to reach people who might learn better through sound rather than pictures.
The exciting potential of integrating sound and reinforcement learning is beginning to be explored. For example, imagine developing audiobooks that can change based on the listener's input. DRL could potentially use data to adjust the narrative in real-time, leading to a more personalized experience. Soundscapes could be a part of DRL environments too, creating more immersive simulations and helping models learn about context within a sound-rich world. There is early evidence that adding audio feedback in the training process from cloned voices may actually help the model learn more efficiently because it's more engaging.
It seems that sounds can act as a feedback loop: they give information to the AI system and also communicate the AI's performance back to developers. This could revolutionize the way that models are trained and improved. We're seeing the beginnings of interactive media that's responsive to audio. Imagine podcasts that can alter their speed or tone in real-time based on a listener's emotional responses. With the capability of voice cloning techniques to capture subtle nuances, including aspects like speech rhythm, audio visualizations could significantly improve the quality of storytelling. It's exciting to envision a future where audiobooks can be crafted in a way that is more relatable to a wider range of listeners.
Exploring Voice Cloning Applications in XAI for Deep Reinforcement Learning at AAAI2024 - Podcast Creation Tools Integrating XAI Principles
Podcast creation tools are increasingly incorporating explainable AI (XAI) principles, marking a shift towards greater transparency and user control in audio production. These tools are aiming to not just generate audio content, but also provide insight into the processes driving the output, leading to improved understanding by the user. This is particularly notable in the context of voice cloning technologies, which allow podcasters to customize and personalize content for various audiences, preferences, and accessibility needs. While the ability to fine-tune audio through XAI principles is promising, it also highlights a need for careful consideration of ethical implications. Questions of voice authenticity and the potential for misuse of cloned voices remain central concerns. Maintaining user trust and ensuring responsible AI applications will be vital as podcast creation tools further integrate XAI features and evolve to leverage the growing capabilities of voice cloning technology in the creation of high-quality and engaging audio stories.
Explainable AI (XAI) is gaining momentum, moving beyond a specialized research topic to a widely explored area with a constant flow of new theories, practical tests, and analyses. The aim of XAI is to make the inner workings of sophisticated machine learning models clear, particularly those that were traditionally considered "black boxes." This transparency is essential for fostering trust in AI systems, allowing people to better understand how AI decisions are made, especially in sensitive situations like medical diagnoses. However, there's still a need for a shared framework for XAI since there's no universal agreement on what exactly constitutes an "explanation."
Voice cloning technology utilizes deep learning to reproduce a person's unique voice by imitating their speaking style, creating audio that sounds remarkably like the original speaker. Podcast production tools are starting to incorporate XAI principles to make their content more appealing and easy to understand for everyone. Researchers are exploring how integrating voice cloning with XAI could create flexible and individualized user experiences. XAI is critical for making sure that AI systems based on deep reinforcement learning aren't just good at tasks but can also provide easy-to-understand feedback on how they're learning. The AAAI 2024 conference is expected to include discussions on the latest developments in XAI, including the impact of voice cloning technologies and their uses in both podcasting and deep learning.
Voice cloning is getting very good at capturing the unique characteristics of a person's voice, including their emotional tone. This "voice style transfer" allows for exciting new possibilities, such as the ability to transform one voice into another, while keeping the original speaker's emotional expression. This opens doors for podcast producers to get creative with voice blending.
Another remarkable feature of voice cloning is the potential for producing multi-character audiobooks. Using this technology, different characters can have unique voices, generated by the same core technology. This offers listeners a more complete audio experience without the need for multiple voice actors. Recent improvements in the noise-reduction algorithms embedded within voice cloning software result in high-quality audio even when recording conditions are less than ideal. This means that podcast producers can create high-quality audio content using readily available devices like smartphones or home recording setups.
Deep learning models are now capable of understanding speech patterns that go beyond simple phonetic features. They capture the distinct prosodic features of an individual's voice. This creates a more nuanced and true-to-life replication of speech, crucial for keeping podcast listeners engaged. Some voice cloning methods are now equipped with real-time editing features. Podcast creators can make changes to the speech during the production phase, a capability particularly valuable for live podcasting, where presenters can adapt their speech in real-time based on audience response.
Voice cloning systems can also be trained to reproduce specific vocal patterns that are specific to cultural or regional dialects. This enables content localization while preserving the voice's essential characteristics. This approach to global content distribution maintains authenticity without losing the essence of the original voice. One particularly fascinating application of voice cloning involves seamlessly blending edited voice samples. Podcast creators can combine multiple takes or sentences without sacrificing a natural speech flow. This ability significantly reduces the overall editing time.
The emergence of personalized voice avatars —essentially customized digital versions of a user's voice —has the power to transform podcast listening. Users can select their preferred voice style for narration, fostering a deeper connection to the content. The recent development of "directionality" in voice synthesis is a noteworthy innovation. Cloned voices can now be generated to mimic spatial audio effects. This improves the listener experience and creates immersive auditory environments within podcast production.
Voice cloning is also finding a niche in feedback systems, where synthesized voices provide real-time commentary on content, similar to a virtual co-host. This innovation can help boost audience engagement through interactive and relatable audio experiences. While these advances are exciting, they do come with the need to address ethical considerations like potential misuse of the technology. It remains to be seen how these advancements will evolve in the years to come.
Exploring Voice Cloning Applications in XAI for Deep Reinforcement Learning at AAAI2024 - Synthetic Voice Applications in AAAI 2024 Presentations
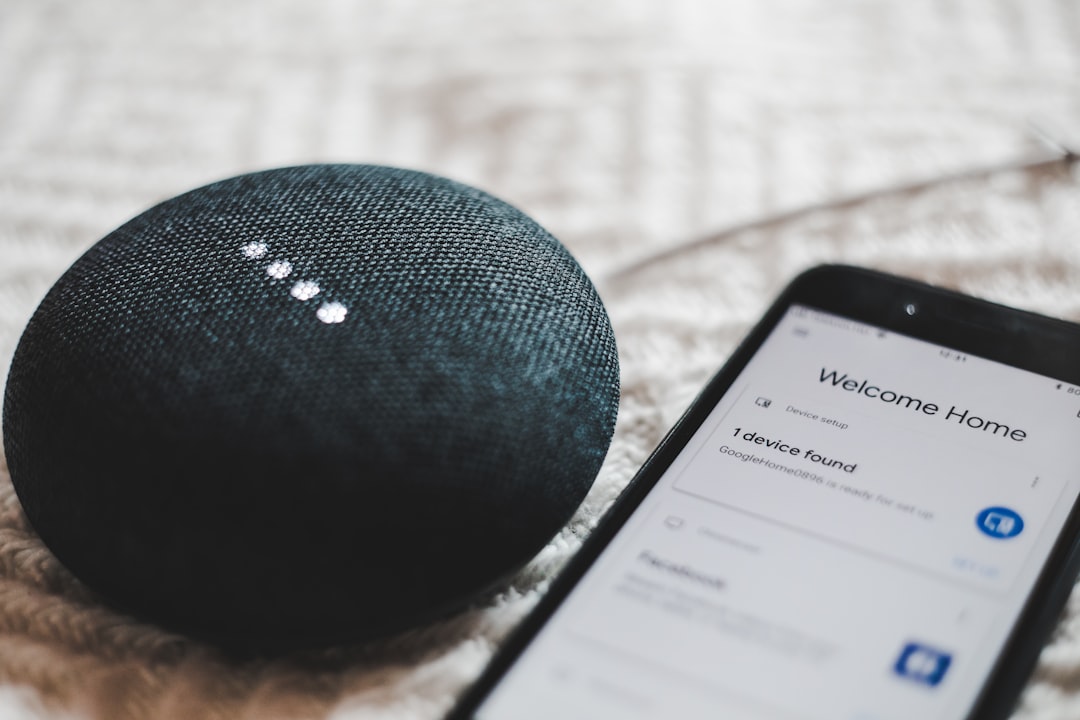
The AAAI 2024 Conference will likely feature prominent presentations on synthetic voice applications, highlighting the continued progress in voice cloning. These technologies are revolutionizing the audio landscape, particularly in audiobook and podcast production, where they provide tools to create more compelling and personalized content. However, ethical questions surrounding the authenticity of cloned voices and the potential for misuse will be central to the discussions, underscoring the vital need for careful deployment of these capabilities. The conference is expected to facilitate discussions on how synthetic voices can enhance user experience while navigating the complex implications of their integration into various audio media. As voice cloning matures, the combination with explainable AI opens new avenues for creating more transparent and user-friendly AI interactions, especially within content creation, potentially improving engagement and accessibility.
The ability of voice cloning to not only mimic speech but also capture subtle vocal quirks like pauses or filler words is making conversational AI, like those used in customer service interactions, sound more natural. This can improve the way users perceive interactions with AI.
Researchers are finding ways to adapt the speed of a synthesized voice to the emotional tone of the text being read, making cloned voices more expressive and relatable for listeners. Imagine listening to an audiobook where the character's voice naturally speeds up or slows down as the story's emotional intensity changes.
Developments in neural networks are enabling voice cloning to occur almost instantly, which has opened up possibilities for live streaming and interactive media. This is pretty exciting as it could reshape how we interact with things like live podcasts or even video games.
The potential for synthetic voices to be fluent in multiple languages is creating a path towards more accessible global communication and cultural exchange. Imagine how this could benefit online learning platforms or language learning apps.
By enabling a single voice actor to create multiple character voices in audiobooks, voice cloning is deepening the richness of audio narratives without the need for a large cast. This could lead to more cost-effective and creative storytelling in audio formats.
There's a movement towards crafting synthetic voices with distinct cultural accents or dialects, which can tailor audio content for specific audiences. It'll be interesting to see how well these voices can capture the nuances of regional accents.
There's work being done to apply voice cloning to create individualized meditation or relaxation audios, allowing users to choose the voice they find most soothing. This could have interesting implications for mental wellness and personalized audio therapy.
Interactive storytelling powered by voice cloning could let listeners influence the story by using voice commands. This could create new kinds of experiences for audiobooks and podcasts, making them feel more like interactive games.
Voice cloning developers are using techniques called adversarial training. The idea is that the system learns by being presented with examples of real and synthetic speech and is constantly trying to improve itself until it's very difficult to distinguish between the two. This could lead to very high-quality synthetic speech.
There's a growing need for tools that can identify synthetic voices. The reason is that as the technology improves, it becomes important to have a way to make sure that people are aware of when they're interacting with AI-generated voices, particularly in contexts where authenticity is important. This is a necessary step to ensure that voice cloning is used in responsible ways.
Exploring Voice Cloning Applications in XAI for Deep Reinforcement Learning at AAAI2024 - Audiobook Production Leveraging Deep Learning Insights
Deep learning is revolutionizing audiobook production, primarily through advancements in voice cloning. These technologies enable the creation of synthetic voices capable of mimicking not only speech patterns but also the subtle emotional qualities of a human narrator, making audiobooks more immersive and engaging. Despite these advancements, replicating the full complexity of human speech remains a hurdle, potentially hindering listener engagement. Additionally, ethical concerns surrounding voice authenticity and the possibility of misuse are prompting increased scrutiny and a call for responsible development within the audiobook industry. Future directions in this field suggest that incorporating emotional cues and contextual awareness into voice cloning can lead to more personalized and relatable audio experiences, further enhancing the audiobook landscape.
1. Voice cloning techniques are getting increasingly sophisticated, now able to reproduce not just the basic sounds of speech but also the more subtle aspects like breathing patterns and moments of hesitation, which makes the synthetic voices sound much more human and relatable.
2. Researchers are employing machine learning methods to generate voices that capture the unique characteristics of regional dialects and informal language patterns, enabling audiobooks and podcasts to resonate more deeply with audiences from specific areas.
3. Some advanced voice cloning tools can now imitate the distinct ways people emphasize certain words and phrases, creating synthesized speech that carries a sense of personality and emotional variation in a way that feels very natural.
4. The data used to train voice cloning models often combines both scripted and unscripted speech recordings. This helps these systems learn how to handle spontaneous, conversational speech, thereby overcoming the limitations of artificial or overly polished speech styles.
5. We are seeing the development of real-time voice cloning systems that can adjust the generated speech on the fly based on how a user is interacting with it. This capability has implications for live audio events and interactive media platforms.
6. Deep learning methods are being designed to interpret the emotional content of written text. This lets voice cloning systems alter their vocal delivery based on the emotional nuances of the content, making audiobooks capable of effectively expressing a wide range of emotions.
7. The idea of "voice blending" is an active area of research, where the goal is to combine the features of multiple voices into a single, hybrid voice. This approach might lead to entirely new kinds of audio experiences that capture unique and diverse vocal characteristics.
8. Integrating voice cloning with augmented reality (AR) holds a lot of promise for educational and training applications. Learners might be able to hear instructions and lectures in a familiar, cloned voice during virtual or AR simulations, potentially enhancing the experience.
9. Researchers are exploring how cloned voices might affect the mental effort required to understand and process information in educational settings. It's suspected that less cognitively demanding voices could improve comprehension and memory in listeners.
10. Alongside the development of tools to create synthesized voices, there's a push to create tools that can analyze those voices. These tools could enable real-time assessments of the authenticity and emotional content of a synthetic voice, which would be useful for quality control in voice production.
Exploring Voice Cloning Applications in XAI for Deep Reinforcement Learning at AAAI2024 - Sound Design Innovations for AI Model Interpretability
Within the expanding field of AI-driven audio production, particularly in areas like voice cloning and audiobook creation, sound design is taking on a new role—that of enhancing model interpretability. This means that the ways sounds are designed and manipulated can help us understand how AI systems are making decisions and generating audio content. This is especially vital within voice cloning, where the integration of explainable AI (XAI) can make it clearer how the system is creating synthetic voices. By employing sophisticated sound design, AI can generate synthetic voices that are not just accurate, but also nuanced, emotionally expressive, and interactive within platforms like audiobooks or podcasts.
While this development is promising, the ethical concerns related to voice authenticity and potential misuse remain a major challenge. As these sound design innovations progress, the need to consider the broader implications of their application becomes more urgent. The way we use sound in AI-driven audio has the potential to fundamentally change how audiences experience and engage with voice-based content, and it's important to ensure that this happens in a way that's both responsible and beneficial. The challenge is to find a balance between the excitement of new possibilities and the serious responsibilities that come with the power to control and manipulate sound.
1. **Capturing the Nuances of Human Speech**: Voice cloning has progressed beyond simply replicating basic sounds, now incorporating intricate details like vocal fry and subtle changes in pitch. This leads to synthetic voices that sound more natural and engaging, bridging the gap between artificial and human-like speech.
2. **Infusing Emotion into Narratives**: Researchers are exploring ways to train voice cloning models to recognize emotional cues within text. This could revolutionize audiobooks, allowing for a dynamic delivery that adapts the tone and pacing based on the story's emotional arc, leading to a more immersive and powerful listening experience.
3. **Voice Cloning for Diverse Audiences**: Efforts are underway to make voice cloning capable of adapting to regional accents and dialects. This opens exciting possibilities for audiobooks and podcasts to resonate more deeply with diverse listeners, enhancing cultural understanding and relatability.
4. **Creating Unique Synthetic Voices**: "Voice blending," a concept that involves combining the attributes of different voices, is gaining attention. This method could generate entirely new, synthetic characters for audiobooks, providing rich and dynamic narratives without requiring numerous human voice actors.
5. **Adaptive Audio for Interactive Experiences**: Recent breakthroughs in real-time voice cloning allow for synthetic speech to dynamically adapt to user interaction. This could transform live podcasting and interactive audio experiences, offering more tailored content and enhancing listener engagement.
6. **Learning to Understand Natural Conversation**: Incorporating a mix of scripted and spontaneous speech into the training data for voice cloning models is helping them better capture the nuances of natural conversations. This allows for a more authentic listening experience that feels less mechanical and repetitive.
7. **Feedback Loops for Optimized Storytelling**: The idea of integrating feedback mechanisms, such as analyzing listeners' emotional responses, is gaining traction. This could allow voice cloning systems to adapt their narration dynamically based on listener engagement, potentially improving comprehension and enjoyment of audio content.
8. **Tailoring Audio for Accessibility**: Voice cloning systems are being developed to adjust vocal characteristics to meet various accessibility needs. This creates possibilities for a broader range of audiobook experiences, accommodating listeners with different auditory preferences and needs.
9. **Integrating Voice with Immersive Learning**: The merging of voice cloning with educational technologies, like virtual and augmented reality environments, is promising. Customized audio resources that adapt to individual learners could create a richer and more personalized educational experience through tailored vocal delivery.
10. **Ensuring High-Quality Synthetic Voices**: The need for tools to objectively evaluate the quality of synthetic voices is becoming increasingly evident. Such tools could assess the emotional authenticity and overall quality of cloned voices, ensuring higher production standards and more engaging experiences for listeners.
Get amazing AI audio voiceovers made for long-form content such as podcasts, presentations and social media. (Get started for free)
More Posts from clonemyvoice.io: