Get amazing AI audio voiceovers made for long-form content such as podcasts, presentations and social media. (Get started for free)
Decoding Digital Audio How Binary Data Shapes Modern Sound Production
Decoding Digital Audio How Binary Data Shapes Modern Sound Production - From Waves to Bits The Basics of Analog-to-Digital Conversion
The foundation of digital audio lies in the conversion of analog sound waves into a digital format, a process known as Analog-to-Digital Conversion (ADC). This fundamental step is crucial for capturing and manipulating audio in modern digital workflows, be it in voice cloning, podcasting, or any form of audio production. Microphones, acting as the initial bridge, translate acoustic vibrations into fluctuating electrical signals, a vital step in the conversion process. The essence of digital audio lies in the ability to represent continuous sound as a sequence of discrete samples. These samples, captured at a specific rate (often 44,100 samples per second for CD quality), are then encoded into binary data – the language of computers. While this digitization revolutionized audio production, offering accessibility and affordability, it introduces a potential trade-off in audio quality. The inherent limitations of the conversion process can result in some loss of fidelity compared to the original analog signal, which naturally contains an infinite number of possible values within its waveforms. The evolution of ADC continues to refine these limitations, impacting how audio creators manage, shape, and deliver their productions in a constantly evolving digital environment.
The choice of sampling rate, a fundamental aspect of ADC, significantly influences the perceived quality of the resulting digital audio. While a standard CD utilizes a 44.1 kHz sampling rate, this rate surpasses the human ear's ability to distinguish subtle differences at the higher frequency range.
The very act of conversion from analog to digital introduces errors known as quantization errors. This stems from the process of rounding continuous analog values to discrete digital ones, potentially leading to perceptible distortions in the audio output. Careful management of this rounding step during the recording process is crucial.
The Nyquist-Shannon sampling theorem dictates that to accurately capture a signal without introducing aliasing artifacts, the sampling rate must be at least twice the highest frequency component in that signal. This fundamental concept sparks ongoing discussions in professional audio circles about the necessity of employing ever-higher sampling rates.
The bit depth, which represents the number of bits used to store each audio sample, plays a crucial role in shaping the overall dynamic range of the audio. A 16-bit representation allows for a dynamic range of about 96 dB, while 24-bit pushes the limits to approximately 144 dB, enabling recordings with finer levels of detail.
During the process of returning from the digital realm to analog using a DAC, digital filters are frequently employed to modify the audio's frequency response. This crucial step helps to minimize artifacts and refine the audio signal, preserving the desired sound quality.
Modern audio compression techniques, like MP3, harness psychoacoustic models to understand the limitations of human hearing. These models analyze audio signals to identify inaudible frequencies which are then discarded, leading to substantially smaller file sizes without sacrificing the perceived audio quality.
Real-time audio processing in DAWs relies heavily on buffering mechanisms. These mechanisms manage the flow of audio data, and a poorly managed buffer can introduce latency, which negatively affects both recording and playback performance.
The burgeoning field of voice cloning faces challenges related to capturing and accurately replicating subtle aspects of a speaker's voice. While large quantities of voice data are needed for accurate training, slight changes in pitch or intonation can contribute to a loss of the original voice's unique characteristics.
The rise of podcasts has shifted the expectations of audio production in the digital age. With an increased emphasis on listener experience, a clear, high-fidelity audio output has become critical for audience engagement and retention, highlighting the importance of mastering the technical aspects of audio capture.
The comparison between the inherent warmth attributed to analog audio systems (often stemming from saturation technologies like tape recording or tube-based equipment) and the precise control offered by digital systems is a consistent topic of debate. Audio engineers are constantly seeking new ways to blend these characteristics to optimize modern recordings, creating a truly unique and dynamic sound.
Decoding Digital Audio How Binary Data Shapes Modern Sound Production - 24-Bit Resolution The Current Standard in Audio Fidelity
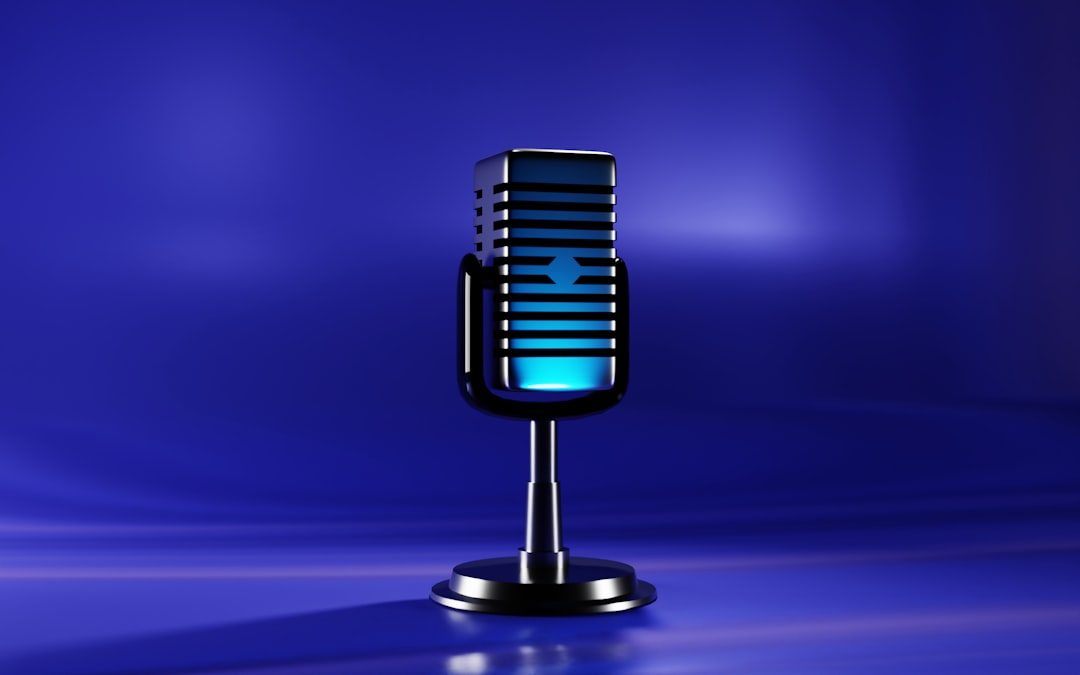
In the realm of digital audio, 24-bit resolution has become the prevailing standard for achieving high fidelity. This means each audio sample can be represented by one of 16,777,216 possible values, a significant leap from the 65,536 values available in 16-bit audio. This increase in bit depth directly translates to a wider dynamic range, reaching roughly 144 dB, allowing for much finer detail and nuance in the captured sound. This level of detail is especially important in applications like voice cloning, where subtle variations in a speaker's tone need to be precisely captured and replicated, and in producing professional podcasts that require clarity and richness.
The availability of high-resolution audio has, however, introduced a trade-off: larger file sizes. Managing these substantial files becomes increasingly important in audio production workflows. While the move to 24-bit is frequently promoted as a pathway to superior sound quality, the tangible advantages over lower bit-depth formats are still a subject of debate within the audio engineering community. The ongoing evolution of digital audio formats necessitates a deeper understanding of the technical intricacies of different resolutions, bit depths, and sampling rates. This knowledge is vital for audio engineers who aim to optimize their recording and production techniques within the evolving landscape of digital audio.
24-bit resolution has become the prevailing standard in audio fidelity, primarily due to its ability to capture a much wider range of sound levels compared to the older 16-bit standard. With 24-bit, each individual sound sample can be represented by over 16 million distinct values, a stark contrast to the 16-bit system's 65,000. This level of precision is crucial in various audio applications, particularly where subtle nuances of sound are important.
This higher precision directly translates to a greater dynamic range of around 144 decibels (dB) in 24-bit audio, in contrast to 16-bit's 96 dB. This expanded range allows for capturing even the faintest sounds without them getting lost in the system noise. This characteristic is particularly valuable in scenarios like recording orchestral music or capturing detailed voice recordings, where capturing a vast range of sound levels is critical. It also inherently leads to a reduction in noise within recordings, a welcome attribute for situations where ambient noise could compromise the audio, such as in podcasts or film production.
Moreover, 24-bit audio offers a valuable amount of 'headroom' for sound editing and manipulation. Sound engineers can apply various effects, gain adjustments, or mixing processes without the risk of distorting the audio because of clipping—a problem prevalent in 16-bit recordings. This makes 24-bit a natural choice in post-production environments. As a result, many modern recording technologies have adopted 24-bit as the preferred standard for capturing high-definition audio, a necessity for high-fidelity playback systems and online streaming services that seek to deliver the best audio experience.
However, the benefits of 24-bit come at a cost—it demands more storage space and processing power. This can create workflow challenges, particularly for smaller productions with more limited resources. Additionally, while it's the preferred choice for a lot of professional applications, 16-bit audio still holds relevance in many contexts because it often provides sufficient quality for general consumer applications.
The improved quality from 24-bit comes partly from managing the inherent quantization noise through a technique called dithering, smoothing out any irregularities in the digital representation of the sound. It's particularly valuable in areas like voice cloning and audiobook production. Using 24-bit in such applications helps capture the subtle nuances of human speech, resulting in a more lifelike and engaging audio output.
But, even with its established position, 24-bit is not necessarily the final word in audio fidelity. A gradual transition towards 32-bit floating-point systems is gaining momentum, promising further improvements in dynamic range and audio fidelity. This pushes the boundaries of what's possible in sound production and prompts continuous discussions regarding future audio technologies and their potential to reshape the sound experience.
Decoding Digital Audio How Binary Data Shapes Modern Sound Production - Sampling Rates and the Nyquist Theorem in Digital Audio
Sampling rates and the Nyquist-Shannon sampling theorem are fundamental concepts in the realm of digital audio, significantly impacting sound quality across applications like voice cloning and podcasting. The Nyquist-Shannon theorem states that to faithfully reproduce an audio signal without introducing distortions, the sampling rate—the number of times per second a signal is measured—must be at least twice the highest frequency present in that signal. If this isn't the case, aliasing can occur, resulting in higher frequencies being incorrectly represented as lower ones, creating a distorted sound. This theorem highlights the crucial role of sampling rate selection in digital audio production. Higher sampling rates allow for more precise capturing and reconstruction of sound, leading to greater fidelity, especially when dealing with complex recordings demanding intricate detail. While the conventional CD-quality rate of 44.1 kHz might suffice for many purposes, the evolving world of digital audio necessitates that sound engineers constantly evaluate the need for even higher sampling rates to achieve optimal results. Balancing sampling rate with factors like bit depth—which determines the number of bits used to represent each sample—is key to optimizing audio quality and achieving clarity and richness in modern digital workflows. Understanding this interplay is essential for capturing, manipulating, and ultimately delivering a high-quality audio experience.
The Nyquist theorem lays out a fundamental principle: to accurately capture audio, the sampling rate—the number of times a sound wave is measured per second—must be more than twice the highest frequency present in that sound. While humans can potentially hear up to 20kHz, the standard 44.1kHz sampling rate used for CDs captures frequencies well beyond the practical range of human hearing. This highlights the constant interplay between achieving perfect fidelity and dealing with the realities of practical audio applications.
When the sampling rate falls short, a distortion known as aliasing creeps in. This results in higher frequencies being wrongly interpreted as lower ones, leading to undesirable artifacts in recordings. This emphasizes the importance of choosing the right sampling strategy for any particular recording scenario.
While higher sampling rates like 96kHz or 192kHz are often presented as the key to ultimate sound quality, numerous audio engineers argue they provide diminishing returns for the majority of listeners. Subjective listening tests regularly reveal that many people struggle to distinguish between 44.1kHz and higher sampling rates, particularly in casual listening scenarios.
In voice cloning, the faithful replication of speech nuances is of paramount importance. The Nyquist theorem comes into play here, because applying an appropriate sampling rate ensures that subtle variations in intonation and pitch are captured precisely. This leads to a more lifelike and convincingly cloned voice.
The Nyquist theorem focuses on frequency response, but the bit depth, or the number of bits used to represent each sample, impacts dynamic range. A 24-bit audio file, for example, possesses a theoretical dynamic range of 144dB, making it capable of representing both very quiet and incredibly loud sounds without distortion. This is crucial in contexts such as audiobook production, where the subtle details of the human voice are critical.
The act of digitizing audio introduces errors, known as quantization errors, that arise from converting continuous analog values into discrete digital ones. Understanding how to control these errors, through techniques like dithering, is crucial in situations where high audio fidelity is required.
Innovative audio compression techniques like MP3 rely on psychoacoustic models which take into account the limitations of human hearing. This means the system can discard audio components that are unlikely to be noticed, enabling greatly reduced file sizes without sacrificing the perceived sound quality.
In digital audio workstations (DAWs), audio data is handled using buffering mechanisms. If these buffers are not managed properly, it introduces latency, which negatively impacts recording performance and the ability to seamlessly apply real-time audio effects—crucial aspects of podcasting or live audio.
While 32-bit floating-point audio has the potential for unparalleled dynamic range and fidelity, many audio engineers remain unconvinced of its overall benefits in typical production workflows. The majority of consumer audio playback systems lack the capacity to take full advantage of the expanded dynamic range, and the practical benefits may not justify the increase in processing demands for numerous applications.
As the field of audio technology constantly evolves, the concepts underlying sampling rates and the Nyquist theorem will undoubtedly shape the development of future audio formats. Understanding these core ideas will be vital for audio engineers, allowing them to make well-informed choices when it comes to capturing and producing sound for years to come, navigating the ever-changing landscape of audio.
Decoding Digital Audio How Binary Data Shapes Modern Sound Production - Quantization and Dithering Techniques in Sound Encoding
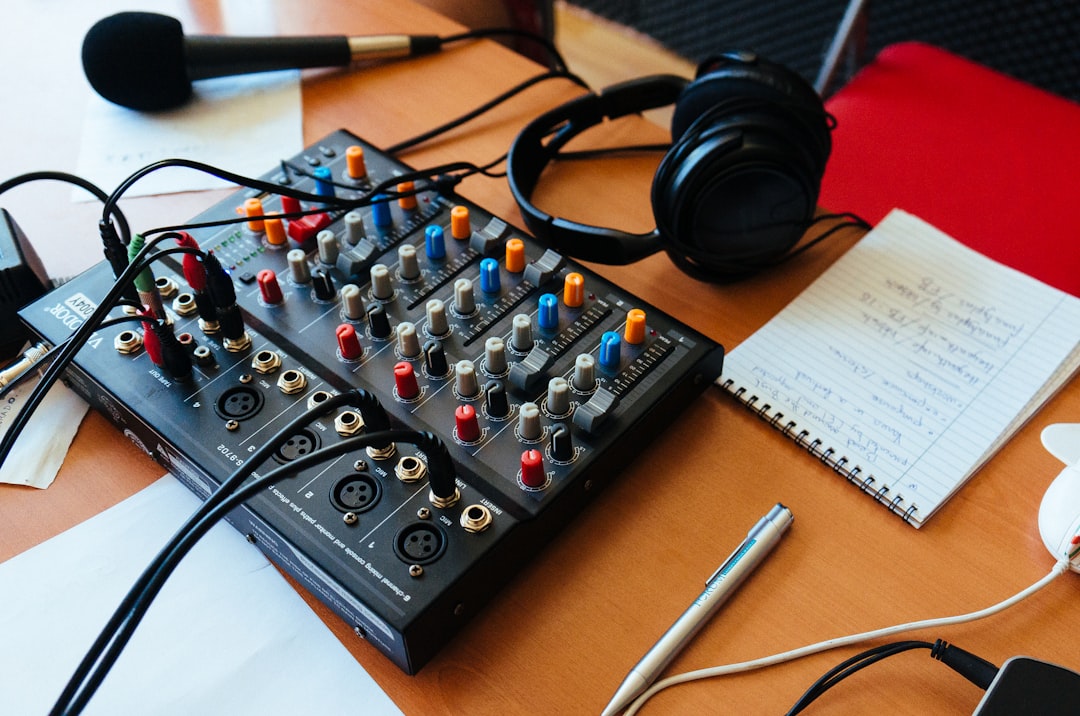
Quantization and dithering are fundamental techniques in the digital encoding of audio, playing a vital role in shaping the resulting sound quality. Quantization involves taking measurements of the audio signal's amplitude at specific points in time, converting this continuous analog signal into a discrete digital representation using binary numbers. This process is critical, as accurate sampling and quantization are essential to faithfully recreate the original audio.
Dithering, on the other hand, is the intentional addition of a very subtle noise signal during the quantization process. This added noise acts to mask quantization errors, which are inherent in the conversion from analog to digital. These errors can lead to audible distortions, particularly in quiet sections of recordings. Dithering helps to minimize these distortions, preserving the intended audio quality. Different dithering methods exist, and skilled use can improve the linearity of the quantization process for lower amplitude audio, enhancing the overall sonic fidelity.
The impact of these techniques is felt across various aspects of audio production. Whether working on a voice clone, meticulously crafting an audiobook, or refining a podcast, the choices made during quantization and dithering have a direct impact on the final listener experience. The ability to manipulate and control these aspects of the audio signal is central to the artistry of sound engineering in today's digital landscape.
When converting continuous analog audio into its digital equivalent, a process called quantization introduces rounding errors, leading to potential distortions. This quantization error can be a noticeable artifact in the sound. Dithering is a clever technique that attempts to mitigate this problem by deliberately adding a low-level noise signal before the quantization process. This added noise effectively masks the quantization error, creating a more natural and pleasing sound, especially in quieter parts of a recording.
While a jump from 16-bit to 24-bit audio representation delivers a dramatic increase in the range of possible sound intensities, or dynamic range, it's not a universal upgrade. In many listening situations, the subtlety captured in 16-bit is more than adequate, especially in environments with background noise where the finer details of a higher dynamic range might be lost. One could argue that it’s an unnecessary jump in certain situations and leads to larger files.
The introduction of dithering, though beneficial in masking quantization noise, does increase the overall noise level of the digital audio. This might seem counterintuitive, but it leads to a perceived enhancement of sound quality as it makes the quantization error less noticeable. This especially matters when handling soft or quieter passages in music or spoken word audio.
While CD-quality audio at 44.1 kHz is a well-established standard, humans can perceive frequencies up to 20 kHz. This suggests that the need for extremely high sampling rates might be overstated in some scenarios, as many listeners won't discern a significant difference in sound quality when comparing high-resolution recordings to standard recordings.
Finding the sweet spot between sampling rate and bit depth is key to optimizing the quality of audio. A high sampling rate captures greater detail in sound, but a lower bit depth can compromise the overall dynamic range of the recording. Balancing the two is critical, particularly when the audio is to be used for nuanced purposes, like in voice cloning or for ensuring high-quality podcast production.
Audio recordings captured at higher bit depths provide a wider range of possibilities for editing and mixing without incurring distortion or clipping issues. Having this added "headroom" is extremely helpful when applying audio effects during post-processing, minimizing the risk of losing valuable detail. This is especially beneficial for spoken-word audio and the kind of detailed audio modifications seen in voice cloning or audiobook production.
When the sampling rate of a recording is too low, higher frequencies can be misrepresented as lower ones, leading to distortion in the audio. This problem, known as aliasing, can create persistent audio issues when working with complex sounds. Therefore, choosing the appropriate sampling rate to avoid this type of distortion is crucial in maintaining audio quality.
Advanced compression technologies like MP3 take advantage of human perception by identifying and discarding inaudible frequencies masked by louder sounds. This enables large reductions in file size while preserving the perceptual qualities of the sound. This is a critical concept for delivering and streaming audio in applications like podcasting and music streaming.
The size of the audio buffer in a digital audio workstation (DAW) directly affects latency. A smaller buffer helps reduce delays in real-time monitoring but increases the risk of audio glitches or dropouts. Finding the right balance between real-time feedback and stability remains a constant challenge for audio engineers in the production process.
The use of 32-bit floating-point audio has the potential to deliver an incredible dynamic range, potentially resulting in exceptionally high-fidelity recordings. However, its broader adoption in typical audio production workflows is often debated. Many consumer playback devices lack the ability to utilize its full potential, leading some engineers to question if the perceived increase in fidelity justifies the increase in complexity.
Decoding Digital Audio How Binary Data Shapes Modern Sound Production - Lossy vs Lossless Compression Methods for Audio Files
In the realm of digital audio, particularly within applications like voice cloning, podcast production, and audiobook creation, the choice between lossy and lossless compression methods plays a vital role in shaping the audio's quality and usability. Lossless compression techniques, exemplified by formats like FLAC and Apple Lossless (ALAC), preserve all the original audio data, ensuring that the decoded audio is identical to the source. This approach prioritizes audio fidelity and is often the preferred choice for applications where preserving every detail of the recording is crucial, for example, when working with voice samples for cloning or in archiving recordings.
Lossy compression, on the other hand, focuses on reducing file size by eliminating data considered inaudible to the human ear. MP3, the most well-known example, employs psychoacoustic models to selectively remove this information, resulting in files significantly smaller than their uncompressed counterparts. This makes lossy compression a practical solution for situations where storage space or data transfer rates are critical, such as streaming audio or using portable devices. However, this approach inherently leads to a reduction in audio quality, with the degree of compromise depending on the chosen compression ratio. Overly aggressive compression can severely impact the audio's clarity and richness, a drawback that audio professionals must consider when choosing the appropriate format.
While lossy compression offers benefits like smaller files and greater efficiency for applications like online distribution, it is generally less desirable in contexts where high fidelity and the finest audio details are paramount. In professional studio settings, for example, lossless compression is often chosen for archiving valuable audio assets and ensuring the integrity of the original recordings. Similarly, voice cloning applications greatly benefit from the high-quality audio that lossless compression provides as even the most subtle nuances in the original voice are important. Ultimately, the decision between lossy and lossless compression is contingent upon the specific requirements of the audio production process and the intended purpose of the resulting audio files.
### Lossy vs Lossless Compression Methods for Audio Files
Audio compression techniques, whether lossy or lossless, aim to decrease the number of bits needed to accurately represent analog sound in the digital realm. Lossless methods, like FLAC and ALAC, preserve the entirety of the original audio information. Decoding a losslessly compressed file results in an exact replica of the original recording. While they do offer a more compact storage size compared to raw audio, these formats do not achieve the same compression rates as lossy methods.
On the other hand, lossy compression, most famously exemplified by MP3, prioritizes significantly smaller file sizes by selectively discarding parts of the audio data. These algorithms, utilizing psychoacoustic modeling, identify and remove audio information that the human ear is less likely to perceive. MP3s, for example, typically result in files that are ten times smaller than their uncompressed or losslessly compressed counterparts. This makes them exceptionally well-suited for scenarios where efficient storage or transmission is critical, such as internet streaming and mobile music players. However, the trade-off comes at a cost of reduced audio fidelity.
Interestingly, many casual listeners are often unable to distinguish between high-quality lossy formats and lossless formats during informal listening sessions. This begs the question if lossless is truly necessary for casual listening or if it mainly benefits certain niche areas, such as audio engineers, who greatly appreciate the transparency of lossless formats during editing stages. However, the more a lossy file is compressed, the more significant the perceived drop in audio quality, including a noticeable decline in dynamic range.
The application of compression methodologies heavily depends on the intended use and the specific requirements of the audio production. For professional audio production, where the integrity of the sound is of utmost importance, and during the archiving of invaluable recordings, lossless is favored for maintaining pristine audio fidelity. But for everyday audio consumption, like the use in audio books, the subtle impact of lossy compression might not detract significantly from the listening experience for the vast majority of listeners. Lossy audio's smaller file size is also often a necessity for situations with restricted bandwidth and network capabilities, as seen in the case of many online streaming platforms that primarily utilize lossy formats.
Nonetheless, a trend towards higher-resolution lossless formats, though not yet widely used in typical listening scenarios, is developing. This development emphasizes the need to consider future audio delivery methods, especially concerning the archival of precious audio recordings. Lossless compression provides a vital tool for preserving original audio quality, assuring that future generations can experience these sounds in their most authentic form. Moreover, lossless provides a considerable amount of flexibility when processing audio during post-production because of the elimination of distortions from compression.
The selection between lossy and lossless audio formats involves striking a delicate balance between file size, audio fidelity, and the context of audio application. This selection can have a significant impact on listeners' perception of the sonic qualities and needs to be carefully considered during every stage of production and dissemination, be it in the creation of podcasts, voice cloning, or audio books.
Decoding Digital Audio How Binary Data Shapes Modern Sound Production - AI-Driven Sound Classification in Modern Audio Production
AI-driven sound classification is revolutionizing audio production by automating the process of categorizing audio. These systems, powered by machine learning, can automatically sort audio into predefined categories like musical genres, environmental sounds (think train whistles or birdsong), or even specific sound effects. This automation relies heavily on deep learning techniques and, in cases where training data is scarce, data augmentation methods can help improve accuracy.
These AI tools are finding practical applications in areas such as voice cloning, podcasting, and audiobook creation where discerning specific audio characteristics is paramount. Convolutional neural networks (CNNs) have proven particularly adept at identifying complex features within audio, making them a valuable tool in these contexts. However, there's a limitation. CNNs can sometimes struggle with capturing the extended temporal relationships within longer audio segments, a feature that is critical when analyzing musical pieces. This presents an ongoing challenge in leveraging these networks for tasks that involve detailed musical analysis.
The future of AI-driven sound classification is bright, promising further enhancements in audio processing and potentially redefining how we categorize and manipulate sound. Its growing integration into audio production workflows has the potential to optimize production processes, while simultaneously leading to significant changes in the ways we perceive and interact with audio.
AI's ability to categorize sounds is becoming increasingly important in modern audio production, particularly in areas like voice cloning and podcasting. Machine learning algorithms, especially those based on deep learning, can analyze audio clips and classify them into various categories, such as musical genres, specific sound effects, or even human emotional states. Tools like the MediaPipe Audio Classifier demonstrate how this technology can be applied to both individual audio clips and continuous streams.
Deep learning models, particularly Convolutional Neural Networks (CNNs), have shown considerable promise in these applications. CNNs are especially well-suited to identifying intricate spatial characteristics within audio data, which is essential for tasks like genre classification. However, their ability to capture long-term temporal information, crucial for understanding musical structure or the flow of speech, is somewhat limited.
The process of classifying sounds usually involves preprocessing the audio data—which could involve segmenting the audio into "windows" or extracting key features. This preparation is vital for training deep learning models. Because deep learning often requires a substantial amount of data for effective training, researchers often employ data augmentation techniques. This involves creating variations of the existing audio data, like changing pitch or adding noise, to artificially increase the size of the training dataset.
Researchers are also trying to get these AI-based systems to understand the context of the sounds they're analyzing. For instance, a system could be trained to differentiate between laughter recorded in a studio and laughter captured from a live audience.
It's worth noting that the development of robust AI-driven sound classification is still an ongoing process. Challenges remain, particularly in areas with limited training data. Some sounds, especially those that are less common, may not be readily classified with high accuracy.
Another current research direction is improving the transparency of AI classification decisions. Understanding how AI arrives at a classification is crucial, especially when the applications are sensitive—think voice cloning, where recreating a specific voice requires understanding the very essence of the speaker's unique characteristics. This drive towards "explainable AI" will likely play a crucial role in the future development of these technologies.
The evolution of deep learning has indeed led to advancements in music genre classification, where AI can automatically learn features that humans might find difficult to define. This automated feature learning paves the way for more accurate and nuanced classification systems. Such technologies have the potential to revolutionize how we work with audio, enhancing audio generation techniques, assisting in source separation, and fundamentally influencing the future of audio production.
Get amazing AI audio voiceovers made for long-form content such as podcasts, presentations and social media. (Get started for free)
More Posts from clonemyvoice.io: