Get amazing AI audio voiceovers made for long-form content such as podcasts, presentations and social media. (Get started for free)
Voice Cloning Techniques in Electronic Music Production Analyzing Jon Gurd's Glass from Space Between
Voice Cloning Techniques in Electronic Music Production Analyzing Jon Gurd's Glass from Space Between - Neural Networks in Voice Cloning for Electronic Music
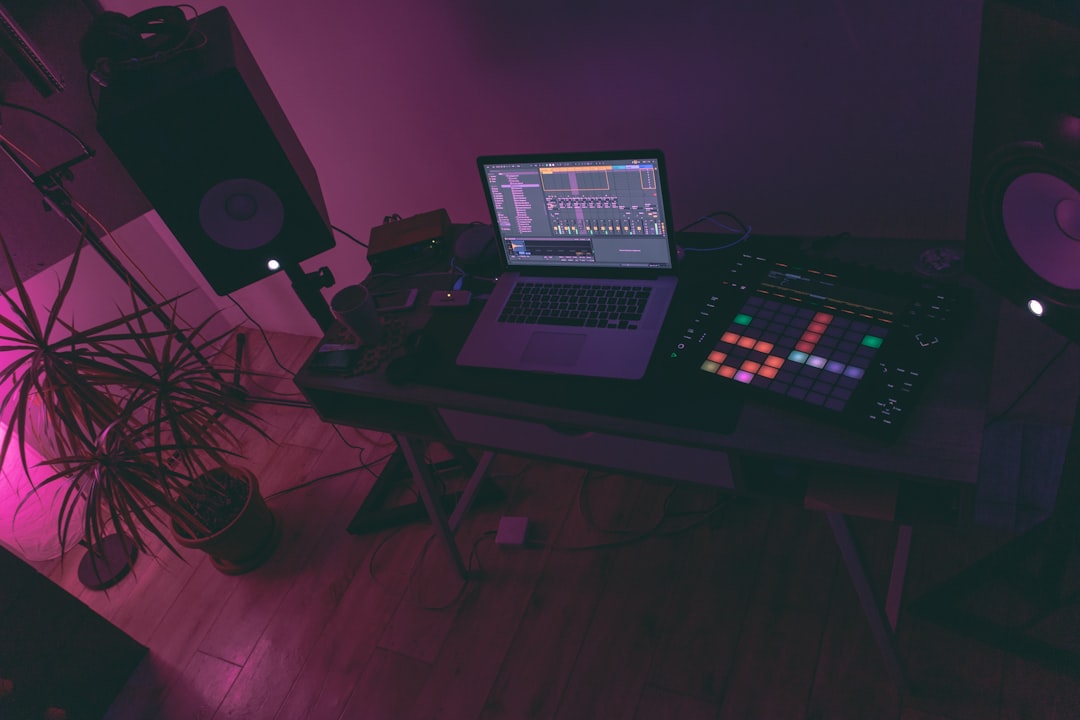
Neural networks are central to the emerging field of voice cloning for electronic music, allowing creators to generate remarkably realistic vocal performances using surprisingly small audio snippets. These networks can be trained to adapt to specific speakers, capturing their unique vocal characteristics while preserving the emotional depth in their voice. This has opened up exciting possibilities for creating personalized audio experiences, particularly in fields like electronic music production where innovative soundscapes are always sought.
Despite these advancements, voice cloning still faces challenges. Current techniques often struggle to accurately replicate the full range of human vocal expression, including subtle changes in tone and emotional nuances that enrich the listening experience. This limitation raises concerns about how well these technologies can contribute to genuinely engaging musical or spoken-word content.
Furthermore, the integration of voice cloning with music production workflows is constantly evolving. The field intersects with the development of new ways to create and manipulate sounds in electronic music. This suggests the potential for future innovations in interactive audio and personalized speech interfaces that may prove impactful for interactive music experiences, podcast production, or audio storytelling. As the technology matures, ongoing research needs to focus on improving the quality and versatility of cloned voices, ensuring they can adapt effectively across different audio environments and create truly expressive and immersive auditory content.
1. Neural networks, especially recurrent types like RNNs, have become central to voice cloning due to their aptitude for handling the sequential nature of speech. They excel at capturing the intricate patterns and nuances of human voice, which is crucial for generating realistic cloned voices.
2. The application of neural networks in electronic music allows for the creation of distinctive, synthetic vocal textures that can be further shaped through digital manipulation. This opens up a new sonic landscape for producers, allowing them to craft vocal elements that are entirely different from traditional sample-based approaches.
3. Cutting-edge voice cloning systems often leverage techniques like WaveNet, which generate raw audio waveforms directly. This method tends to produce higher-fidelity and more natural-sounding synthetic voices compared to the more conventional text-to-speech methods.
4. Voice cloning technology has the potential to generate convincingly realistic "deepfake" audio, capable of imitating famous voices or even historical figures. This raises legitimate ethical questions in fields like audiobook and podcast production, where authenticity is crucial for building trust with the audience.
5. The quality of the training data plays a critical role in the efficacy of a voice cloning model. Using high-quality recordings with a wide range of phonetic elements generally leads to a more versatile and convincing cloned voice.
6. Advanced voice cloning algorithms are increasingly capable of incorporating emotional nuance into the synthesized speech. Producers can now generate vocal performances that convey specific emotions, vastly expanding the creative possibilities within electronic music composition.
7. Neural networks can be employed to pinpoint and analyze the unique spectral signatures of a voice. This allows us not only to clone voices but also to seamlessly apply sound effects that complement the aesthetic of different music genres, adding another layer of complexity to electronic music production.
8. Within the context of electronic music production, voice cloning offers a powerful tool for building entirely synthetic vocal ensembles. This can reduce the need for live vocalists and enable a streamlined production process, allowing for programmable and precisely controlled vocal lines.
9. In cases where a neural network isn't adequately trained, the resulting cloned voice can contain undesirable artifacts like glitches or artificial-sounding pitch fluctuations. This underscores the critical need for meticulously tuning these models to ensure the produced audio maintains a high level of quality.
10. Ongoing research in voice cloning is focusing on developing cross-lingual capabilities. The goal is to create voice models that can be trained on one language and then generate realistic vocalizations in another. This development has the potential to greatly increase the accessibility and global reach of electronic music production.
Voice Cloning Techniques in Electronic Music Production Analyzing Jon Gurd's Glass from Space Between - Isolating Vocal Tracks from Commercial Productions
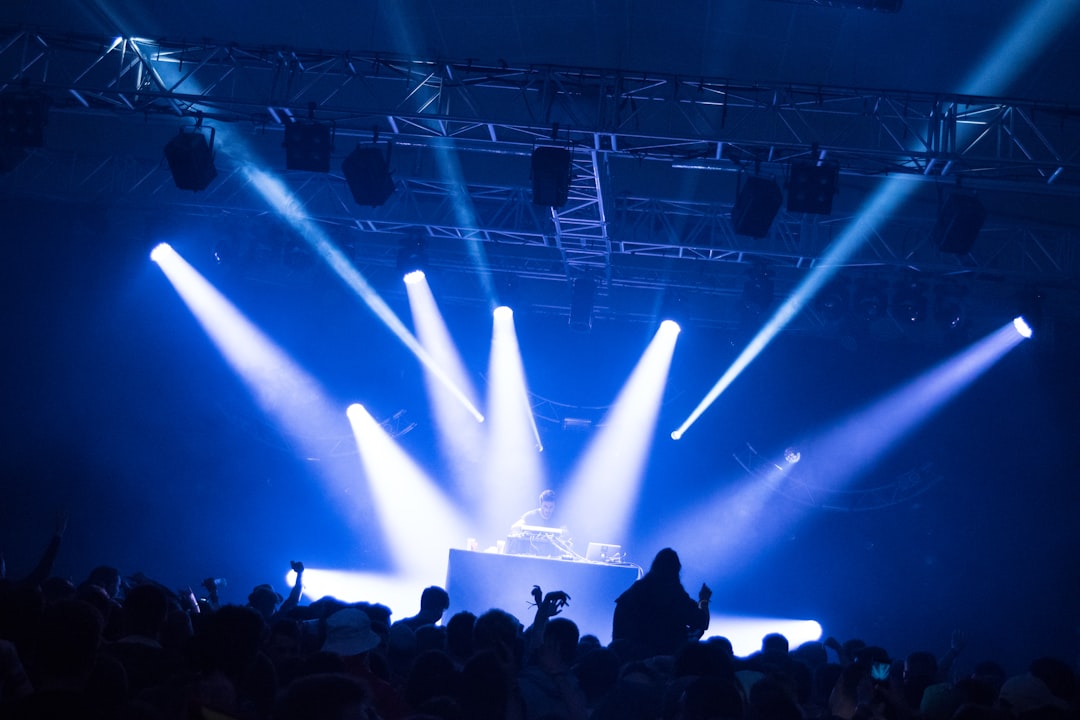
Separating vocal tracks from commercially released music has become increasingly achievable due to recent advancements in artificial intelligence and machine learning. Techniques like convolutional neural networks and specialized audio separation models have made it possible to isolate vocal elements with a high degree of precision, allowing producers to experiment with sound design in novel ways without sacrificing audio quality. Several tools have emerged, such as Spleeter and the Stem Splitter, which provide user-friendly interfaces for separating vocals from music, making remixing and sound manipulation simpler. This increased control over individual audio elements offers electronic music producers more creative freedom to shape distinct sonic landscapes. However, these capabilities also raise important considerations regarding authenticity, originality, and potential copyright implications. As these technologies continue to mature, they are expected to profoundly reshape the landscape of music production, providing both remarkable opportunities and complex challenges for creative individuals in the field.
Extracting vocal tracks from commercially released music often relies on advanced methods like phase cancellation. This technique involves inverting specific frequencies of other instruments to lower their relative volume compared to the vocal track, resulting in cleaner isolations that can inform voice cloning efforts. However, the harmonic nature of a voice frequently leads to overlapping frequencies with instrumentation in a mix, making it difficult to perfectly isolate vocals without losing clarity or creating sonic artifacts. This highlights the significance of spectral analysis in audio engineering, especially when aiming for a high-quality voice clone.
Modern vocal isolation tools increasingly leverage machine learning algorithms. These algorithms analyze the frequency patterns present in vast collections of vocal samples, enabling them to differentiate between vocal and instrumental components within a mix more accurately. This ability is crucial for high-quality voice cloning, as it allows for a more authentic representation of the original vocal performance. Advanced separation techniques, such as source separation using neural networks, have drastically improved vocal extraction from mixed audio. This flexibility is valuable for voice cloning projects where accurately replicating the original voice is paramount.
Interestingly, vocal effects like reverb and delay can pose challenges during isolation. These effects produce "ghost" echoes that complicate the extraction process. To counteract this, precise equalization and meticulous time-domain editing are often required to refine the extracted vocal track. CNNs, used in vocal isolation, learn to recognize and separate different audio sources based on their unique physical characteristics. This approach offers a level of accuracy often difficult to attain with traditional methods.
In the context of podcast production, isolating voice tracks significantly enhances audio clarity, which in turn improves listener engagement. Clear speech facilitates a more effective delivery of emotions and ensures that the intended message is not overshadowed by background noise. Furthermore, DJs and producers are increasingly leveraging isolated vocal tracks to craft remixes. This use case illustrates how vocal processing tools contribute to entirely new compositions. It emphasizes the ability to accentuate the original vocal's essence while simultaneously incorporating cutting-edge sound design techniques.
Additionally, vocal isolation procedures in commercial settings occasionally yield unexpected discoveries, such as alternate vocal takes that were not meant for public release. These discoveries can stimulate new creative avenues or inspire retrospective projects in music production. The continuous advancements in AI-driven vocal isolation technologies hold promise for future capabilities. There's a possibility that real-time processing in live performances might become a reality. This development could allow artists to manipulate and clone vocal performances instantly, fostering an experimental and innovative approach to sound design in electronic music, in particular.
Voice Cloning Techniques in Electronic Music Production Analyzing Jon Gurd's Glass from Space Between - Transfer Learning Applications in Voice Synthesis
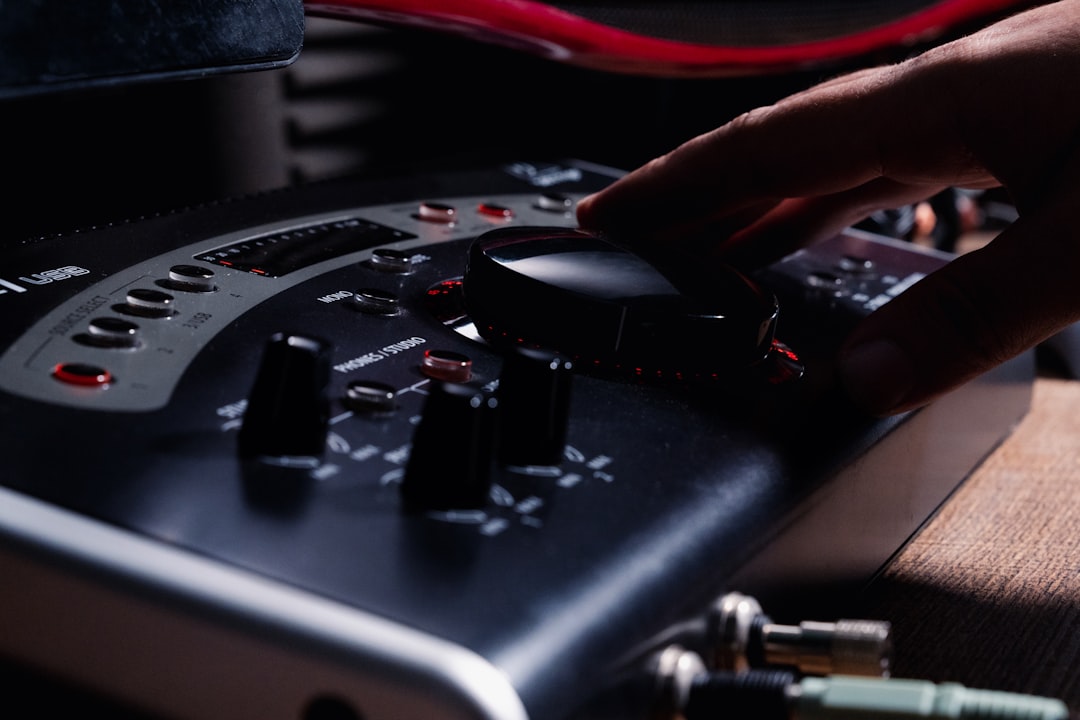
Transfer learning is a game-changer in the world of voice synthesis, impacting how we create and manipulate artificial voices. This technique allows us to take models already trained on a wide variety of speakers and adapt them to create new, specific voices. This is especially valuable in audio production, including electronic music, audiobooks, and podcasts. By adapting existing models, we can effectively synthesize high-quality vocal performances with impressive accuracy, streamlining the whole voice cloning process and reducing the need for massive amounts of new audio data. While this is quite impressive, there's still room for improvement. Current methods can struggle to perfectly capture the subtleties of human expression, such as the subtle changes in tone and emotion that make voices sound truly natural. Overcoming these hurdles will be key to making sure that synthesized voices can truly engage listeners in an authentic way. This ensures these tools meet the evolving expectations of our modern audio experience.
Transfer learning has emerged as a powerful tool in voice synthesis, significantly reducing the need for extensive training data. This efficiency is particularly beneficial when cloning voices for specific applications, as gathering large, diverse datasets can be impractical. By leveraging models trained on a broad range of voices, we can adapt them to synthesize new voices using only a handful of recordings, paving the way for quicker implementation in projects like podcast production or electronic music.
Recent developments in hybrid voice synthesis models, which combine both spectral and waveform generation, have successfully integrated transfer learning techniques. This approach has yielded a notable improvement in the quality and emotional range of synthesized voices. This aspect is crucial in electronic music production where expressive vocal lines are frequently incorporated to evoke specific moods and emotions.
Moreover, transfer learning enables the adaptation of voice models to different accents or dialects. This capability empowers producers who aim to explore a wider range of vocal styles, incorporating diverse vocal presentations in their tracks. This can be used to create unique or niche sonic characteristics within their productions.
Transfer learning also plays a key role in ensuring consistent voice quality across different audio environments. Be it a studio recording or a live performance, cloned voices maintain their characteristic timbre and expressiveness, resulting in an elevated listening experience. This holds substantial implications for voice-related projects across diverse media, from audiobooks to podcasts.
In the realm of audiobook production, transfer learning can assist in adapting vocal models to replicate specific narrative styles and intonation. This helps in creating synthesized voices that sound more natural and engaging, enhancing the overall listening experience. This is of particular importance in certain genres where narrative pacing and emotional arc are critical to conveying a story effectively.
Podcast production is another area where transfer learning offers advantages, enabling the cloning of a host's voice for creating personalized greetings or annotations. This enhances listener engagement without the necessity of additional recording sessions, making podcast production more efficient and cost-effective. However, it remains important to ensure authenticity and transparency around such uses.
Within the realm of electronic music sound design, transfer learning provides a valuable approach to incorporate elements of famous or recognizable voices into compositions while carefully circumventing copyright issues. However, this raises the ongoing questions around copyright and the ethical considerations of such practices.
Further, transfer learning can be utilized to generate dynamic vocal performances that respond to real-time musical elements like tempo and key changes during production. This facilitates a more intuitive connection between synthesized voices and the evolving soundscape. In this context, it's worth exploring whether such practices contribute to a more dynamic and immersive musical experience, although this is yet to be clearly established.
The growing sophistication of transfer learning models allows them to capture and replicate vocal variations based on emotional cues. This enables producers to craft vocal lines that effectively convey different moods and emotions, drastically enhancing the expressiveness of electronic music compositions. However, researchers must continually examine the accuracy of emotional interpretations when applying this technology.
As the technology continues to progress, researchers are actively exploring transfer learning strategies for incorporating multilingual capabilities into voice synthesis. This advancement holds the potential for seamless iteration between multiple languages, significantly expanding the global reach of audio content. While it offers a lot of potential, further investigation into how effectively this can be applied and at what quality levels will ultimately be the defining factor in its usefulness.
Voice Cloning Techniques in Electronic Music Production Analyzing Jon Gurd's Glass from Space Between - Zero-Shot Multi-Speaker Text-to-Speech Technology
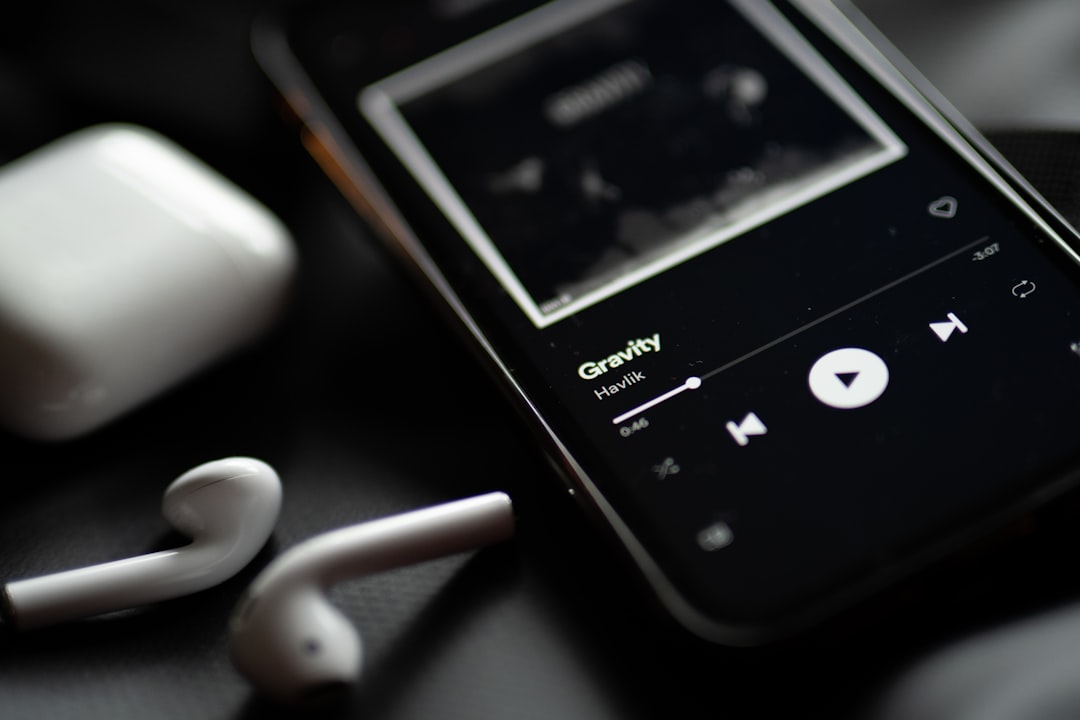
Zero-Shot Multi-Speaker Text-to-Speech (TTS) represents a notable leap forward in voice synthesis, particularly relevant to audio creation like electronic music, podcasting, or audiobook production. It allows for the generation of new voices, even if those speakers were not part of the initial training data, just by using a short audio snippet. This capability opens doors to crafting unique vocal performances with minimal input, adding a new dimension to creative audio workflows. Recent innovations in TTS, such as XTTS, have expanded the language scope, overcoming earlier limitations where models struggled to support more than a few languages.
Despite this progress, challenges remain in perfectly replicating the subtleties of human voices, such as emotional nuances and individual vocal characteristics. These aspects are crucial for crafting truly engaging audio experiences. As this technology matures, its potential for unlocking creativity in voice-based projects will be tremendous. However, it's essential to engage with the ethical questions surrounding voice authenticity and artistic expression that these technologies present. The ongoing development of these technologies will undoubtedly reshape how we produce and interact with audio.
Zero-shot multi-speaker text-to-speech (TTS) technology is fascinating because it can generate synthetic speech from speakers it hasn't been specifically trained on. Essentially, you can give it just a few seconds of audio from a new voice, and it can learn enough to produce relatively convincing speech in that voice. This expands the potential applications of synthetic voices in things like music production and podcasting considerably.
The quality of these zero-shot systems often depends on how effectively they can capture and represent a speaker's unique characteristics through what are known as embeddings. These embeddings act like a sort of digital fingerprint of a voice, allowing the system to synthesize speech that retains emotional nuances and style, even when generating new content.
One of the more appealing aspects of zero-shot methods is that they can produce decent results with minimal input audio, unlike traditional cloning which requires large datasets. This speed and efficiency are particularly beneficial for projects with time constraints, like quickly putting together a podcast or crafting a new section in a musical composition.
Zero-shot systems often rely on sophisticated algorithms like attention mechanisms. These algorithms allow the models to focus on the most important aspects of a speaker's voice when creating new content. This, in turn, enhances the coherence and quality of the synthetic audio which is crucial to keeping a listener engaged, especially within the context of music and storytelling.
The ability to generate synthetic voices of various people, potentially including public figures or even fictional characters, presents interesting possibilities for music creation and collaboration. It can reshape traditional music collaborations in ways that were previously not possible. We might see entirely new sonic palettes and creative pathways within electronic music, soundtracks, and even other genres.
The ongoing progress in zero-shot TTS has created systems that are capable of handling multiple languages and accents, further expanding the global reach of audio production for music, podcasting, and audiobook narratives. It's likely that we will see increasingly widespread use of these techniques as they mature.
Zero-shot approaches can streamline musical production workflows. Sound designers and producers can quickly prototype vocal parts within a track, experimenting with different sonic textures without having to rely on recordings from live singers or specific sound libraries. This experimentation fosters innovative audio landscapes for music productions.
In audiobook production, zero-shot synthesis offers the possibility of generating narratives with synthetic voices that portray distinct characters, enriching the listener's experience. Ideally, these voices are seamlessly woven into the story, creating a consistent auditory experience throughout the book.
However, there are limitations to zero-shot methods. One recurring issue is that they can struggle to fully capture the subtle complexities of human expression, sometimes leading to audio that feels somewhat robotic or lacking in authentic emotionality. This can detract from the overall experience, particularly in the context of electronic music where nuanced vocal expression often plays a significant role in setting the emotional tone of a piece.
Moving forward, one exciting prospect is the development of zero-shot TTS systems that allow for real-time adjustments. Imagine, for instance, being able to dynamically alter a synthesized vocal performance in response to changes in the musical accompaniment. This would lead to a more interactive and responsive music-making experience. It remains to be seen how useful this capability ultimately becomes.
Voice Cloning Techniques in Electronic Music Production Analyzing Jon Gurd's Glass from Space Between - Voice Conversion Techniques for Speaker Transformation
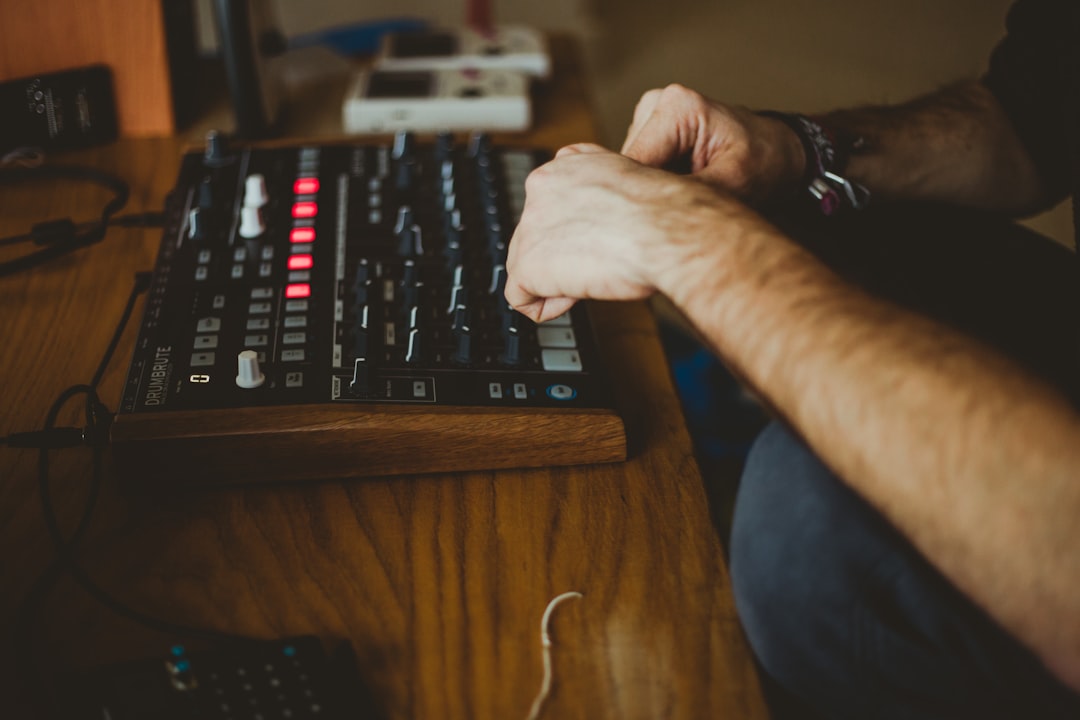
Voice conversion techniques, specifically those focused on speaker transformation, offer the ability to shift the characteristics of a speaker's voice onto another while preserving the original spoken content. These methods utilize a sophisticated blend of algorithms, encompassing speech analysis, speaker classification, and vocoding, to achieve this transformation. Deep learning's recent integration has dramatically enhanced the fidelity of voice conversion, generating more human-like voice outputs with remarkable similarity to the target speaker. This is proving invaluable in areas such as electronic music production, audiobook creation, and podcasting.
However, even with these improvements, there's an ongoing need for refinement in voice conversion techniques. Accurately preserving the emotional nuances present in human speech and maintaining consistently high audio quality throughout the conversion process are persistent obstacles. These challenges are particularly important in domains like voice cloning and podcasting where engagement and believability are crucial. As research and development in this space continue, we can expect further improvements, pushing the boundaries of what's possible with synthesized voices. This will undoubtedly have significant ramifications across audio production, stimulating further conversation about how we view authenticity and creativity within the context of digital sound design.
Voice conversion methods frequently rely on mel-spectrograms, which represent audio frequencies over time, allowing for refined adjustments of vocal characteristics during the conversion process. This visual approach helps achieve more accurate conversions because it highlights the unique spectral patterns of various speakers.
The effectiveness of voice conversion hinges on how well phonetic traits are aligned between the source and target voices. Discrepancies in phonetic characteristics can lead to unnatural-sounding outputs, emphasizing the need for meticulous model training and careful feature extraction during voice cloning efforts.
Many voice conversion systems use adversarial training, inspired by Generative Adversarial Networks (GANs). This approach challenges the model to create outputs indistinguishable from real audio, substantially improving the quality of converted voices by minimizing noticeable differences.
Interestingly, voice conversion can be used for enhancing as well as transforming a voice. By incorporating emotional cues and altering speech rates, producers can enrich the original voice with specific emotional content, turning static vocal performances into dynamic and expressive tracks.
A surprising use of voice conversion is in personalizing audiobooks and podcasts. Creators can employ it to tailor synthesized narrations to fit specific characters, creating an engaging listening experience by capturing the essence of various personas without requiring multiple voice actors.
Research suggests deep learning architectures, such as Variational Autoencoders (VAEs), can enhance the stability of voice conversion systems. These models help learn a hidden representation of vocal characteristics, enabling smoother transitions and more cohesive outputs between distinct voices.
Voice conversion systems are not limited to speech; they are also effective with singing. By mapping vocal tracts from one singer to another, producers can create new performances that retain the emotional expressiveness of the original singer, enabling novel approaches to music production.
One of the more captivating facets of voice conversion is its possible use in live performances. Using real-time voice transformation, artists could dynamically adjust their vocal delivery during a performance, allowing for spontaneous creativity that reacts to the audience or music in real-time.
Some advanced voice conversion models are trained on multilingual datasets, allowing them to adapt a speaker's qualities across languages. This capacity not only enhances accessibility in global music production but could facilitate cross-cultural collaborations between artists.
Although voice conversion offers new opportunities for creative expression, it also carries the risk of misrepresentation, especially in sensitive contexts like podcasting and media production. The capacity to convincingly imitate someone's voice can lead to ethical issues and potential misuse, highlighting the importance of using it responsibly in audio production.
Voice Cloning Techniques in Electronic Music Production Analyzing Jon Gurd's Glass from Space Between - HiFiGAN Model Enhancements for Realistic Voice Synthesis
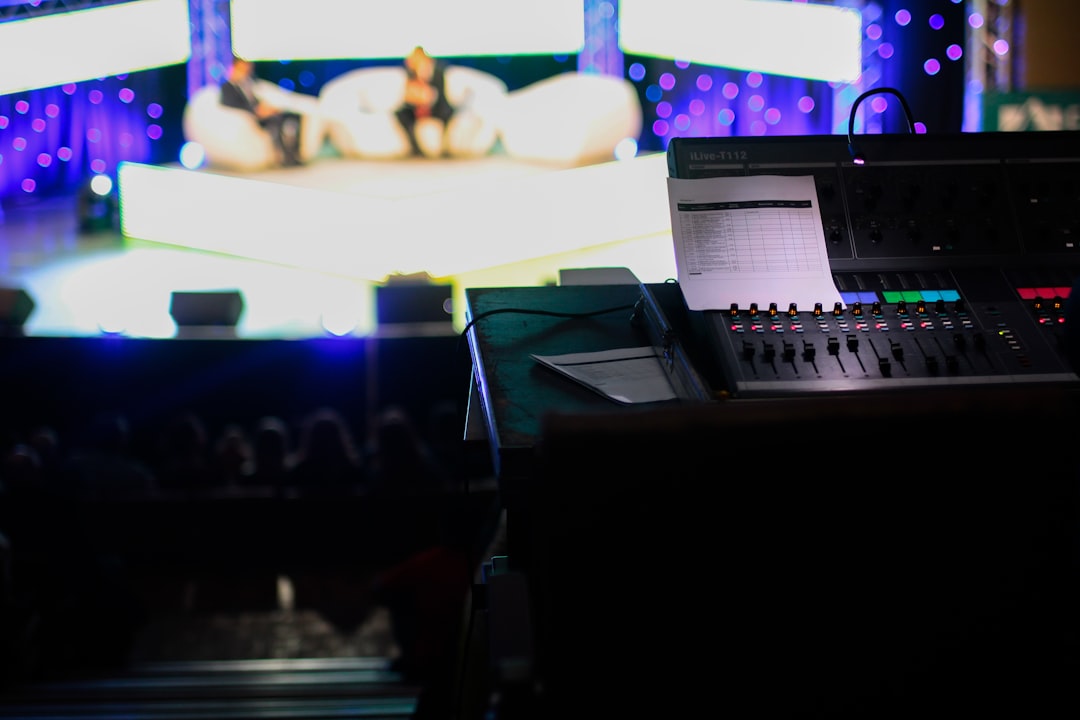
HiFiGAN's advancements have significantly improved the realism of synthesized voices, especially impacting how voice cloning is utilized within electronic music. By using an x-vector as the embedding, the model excels at capturing a speaker's distinctive vocal qualities, ultimately producing more accurate synthetic voices. Further refinements have significantly reduced the number of parameters required, resulting in faster processing times without sacrificing audio quality.
However, challenges remain in truly mimicking the emotional complexity and subtle variations found in natural human speech. These nuances are critical for creating audio that truly connects with listeners. The future development of HiFiGAN and its applications seem promising, with potential uses in composing music, generating podcast content, and other voice-centric creations. This advancement is poised to revolutionize personalized audio experiences, but it's crucial to consider the complexities this technology presents, especially as it relates to authenticity and the creative process.
HiFiGAN, a model primarily used for generating high-quality audio waveforms, has been refined to significantly improve its capabilities for realistic voice synthesis. This is particularly interesting because it enhances the representation of a speaker's voice through the use of an x-vector embedding. Essentially, the x-vector acts as a more detailed description of the speaker, which leads to a more accurate reproduction of their voice. This approach is a step forward compared to other methods that rely on d-vectors which seem to be less accurate in capturing a person's vocal traits. A key development is that the improved HiFiGAN model significantly reduces the size of the vocoder component. This not only speeds up the process of generating voice, but it also allows for its potential use on hardware that might not have been capable before. In testing, this refined model produced superior outcomes across different evaluation metrics compared to other voice synthesis techniques.
One of the critical aspects of HiFiGAN is its ability to take mel-spectrograms and convert them into raw waveforms, effectively overcoming a significant challenge in speech synthesis. This is achieved by leveraging a Generative Adversarial Network (GAN) architecture which is increasingly common in many audio and image generation projects. These GANs consist of smaller, specialized components (sub-discriminators), allowing HiFiGAN to be both computationally efficient and generate high-fidelity output.
The application of HiFiGAN in voice cloning has enabled the development of efficient Text-to-Speech (TTS) systems. These systems have shown that they can generate highly realistic synthetic voices using relatively small amounts of training data. This is quite significant because it reduces the barrier to entry to generating realistic cloned voices and expands the possibilities for its use in projects such as electronic music creation, podcasting, audiobook productions, and possibly even voice-activated interactions in smart devices. However, I do think the area of emotional tone representation, while improving, is still a hurdle that must be overcome before the general public readily accepts them. Many individuals are becoming more critical about how they are perceived, especially in light of AI being able to create synthetic voices. It will be interesting to see how this plays out as we progress.
While the promise of HiFiGAN is enormous, as with any new audio technology, we must still be mindful of its potential misuse and the implications this has on the content that is generated with it. It would be prudent to continue to explore ways to create markers for content that has been created using these technologies so that listeners can make informed choices about what they consume.
Get amazing AI audio voiceovers made for long-form content such as podcasts, presentations and social media. (Get started for free)
More Posts from clonemyvoice.io: