Get amazing AI audio voiceovers made for long-form content such as podcasts, presentations and social media. (Get started for free)
Voice Cloning Meets Operations Research Optimizing Audio Production Workflows
Voice Cloning Meets Operations Research Optimizing Audio Production Workflows - Real-time Voice Cloning Revolutionizes Audiobook Production
Real-time voice cloning is rapidly altering how audiobooks are produced, primarily by boosting efficiency and offering a more personalized listening experience. Companies like Amazon are embracing this technology, allowing audiobook narrators to digitally replicate their voices. This allows for faster production of new titles, potentially leading to a surge in audiobook availability. The ability for authors to have their books narrated in their own voice adds a unique and intimate element to the storytelling, further enhancing the listener's engagement.
Furthermore, the ongoing refinements in voice cloning algorithms are contributing to a more polished and refined audio experience. Noise reduction and other improvements are resulting in a higher level of audio quality. The future implications of this technology extend far beyond audiobooks, likely to influence how a wide range of audio content is both created and enjoyed in the coming years. While the benefits are apparent, it's also important to consider potential implications of this technology on the roles of human narrators and the overall artistic integrity of audio storytelling.
The emergence of real-time voice cloning is significantly impacting audiobook production. These systems, powered by intricate algorithms, can now replicate a speaker's voice with remarkable fidelity, often within a mere 100 milliseconds. This breakthrough stems from leaps in deep learning, allowing voice models to learn the nuances of a speaker's voice from comparatively short audio samples – sometimes as little as 10 minutes. This reduction in the required audio data is a game-changer, streamlining the process of voice creation for audiobook production.
Beyond simply mimicking a voice, these systems are becoming increasingly adept at modulating the emotional tone of the synthetic voice. By adjusting pitch, tone, and cadence, they can now portray a wider range of emotions, bringing more depth and character to audiobook narrations. This ability contrasts sharply with the often robotic sound of older text-to-speech technologies. Today's voice cloning can produce synthetic speech that is incredibly difficult to differentiate from a human narrator, blurring the line between human and artificial narration.
The potential for personalization is another exciting aspect. Audiences may choose narrators based on their preferences, fostering a more tailored listening experience. Additionally, the voice could be modified to match a story's mood or theme. This adaptability extends beyond audiobooks to fields like podcasting, where cloned voices can create synthetic co-hosts or guest speakers, enriching the creative process while keeping the podcast's overall aesthetic consistent.
The ability to quickly clone voices and adapt them to multiple languages is another remarkable advantage. It potentially unlocks access to audiobooks for a global audience without the need for extensive re-recording. Furthermore, it is becoming possible to replicate the acoustics of different environments. Imagine being able to create audiobooks that mimic the sound of a grand hall or a cozy room. Such features create an added layer of realism and immersion within the listening experience.
However, challenges remain. While the technology has progressed dramatically, achieving perfectly consistent and natural voice modulation is still a work in progress. The potential for glitches or irregularities in the synthetic voice necessitates ongoing research into refining algorithms and improving the naturalness and reliability of cloned voices. This area is crucial to ensure the high quality and trustworthiness of this emerging technology.
Voice Cloning Meets Operations Research Optimizing Audio Production Workflows - AI-driven Noise Reduction Enhances Podcast Audio Quality
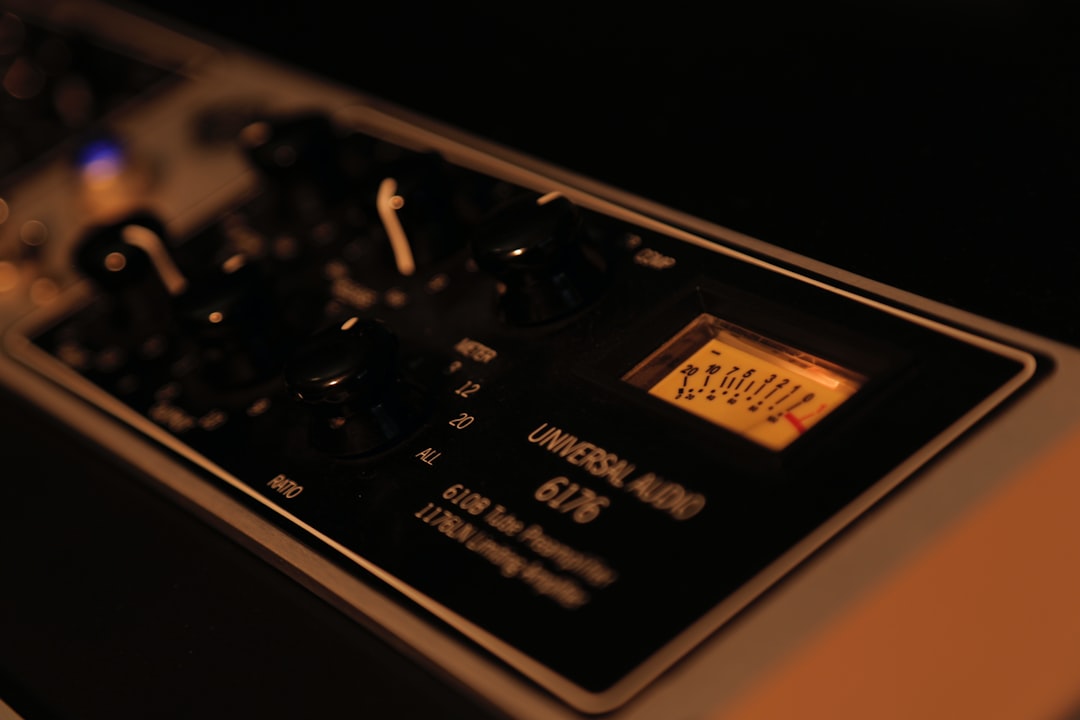
AI-powered noise reduction is transforming podcast audio quality, simplifying the production process and improving the listener's experience. These tools use sophisticated algorithms to identify and eliminate unwanted background sounds like traffic noise or room hum, ensuring the speaker's voice is the central focus. This technology not only enhances audio clarity but also streamlines the post-production workflow, allowing podcasters to spend more time on the creative aspects of content creation. As these AI systems advance, we can anticipate further improvements in audio fidelity, potentially leading to a new era of podcast audio production. However, alongside these benefits, questions arise about the impact of these innovations on the established standards and artistic integrity within the field.
The perception of audio quality is subjective, with individuals exhibiting varying sensitivities to different sound frequencies. This means that a noise reduction technique effective for one listener might not be ideal for another, highlighting the need for adaptable algorithms. Understanding how our ears perceive sound is crucial, and it turns out, louder sounds can mask quieter ones. This "masking" effect underscores the importance of effective noise reduction, where AI tools analyze audio and isolate unwanted noise without impacting the desired sounds, improving the overall clarity of podcast audio.
Research shows that listeners subconsciously react to audio quality—better audio can improve engagement and retention rates in podcasts. This makes noise reduction a critical aspect of audio production, especially in formats where audience retention is key. Some AI techniques use spectral subtraction, analyzing audio spectrograms to separate vocal elements from noise. This can improve the overall production process, and also has applications in the refinement of voice cloning efforts.
Higher frequency noises are particularly detrimental to vocal clarity. Interestingly, AI-driven noise reduction methods can learn to dampen these specific frequencies while keeping the lower, richer parts of a voice intact. This ensures the naturalness of a voice, which is especially important when using voice cloning technology. Advanced noise reduction algorithms are quite capable of reducing noise levels by up to 30 decibels without affecting the audio quality. This is very useful in noisy environments or when podcasting in challenging recording conditions.
In some tests, listeners found audio processed by AI noticeably more enjoyable and less tiring over extended periods. This shows that noise reduction isn't just a technical benefit, but can enhance the entire listening experience. Maintaining the natural tone of a speaker's voice is important, especially when cloning that voice. Luckily, AI-powered noise reduction methods can preserve this unique timbre, ensuring that the synthesized voice retains the qualities that make it distinctive.
Another technique is multi-channel noise reduction, which allows for the separate processing of each microphone input. This is particularly helpful in podcast recordings with multiple microphones or where there are overlapping sound sources. The need for noise reduction has evolved hand-in-hand with improvements in recording technologies. Microphones have gotten incredibly sensitive, making the necessity for powerful noise reduction techniques more apparent, propelling innovation in both AI and audio engineering practices.
Voice Cloning Meets Operations Research Optimizing Audio Production Workflows - Multilingual Voice Synthesis Expands Global Content Reach
Multilingual voice synthesis is dramatically expanding the global reach of audio content by allowing creators to connect with a wider range of listeners. New technologies, such as voice cloning and multi-language text-to-speech systems, now produce high-quality, natural-sounding speech across many languages without the need for extensive language-specific training. This development has broadened the reach of audiobooks, making them more accessible to people whose first language isn't the language of the original recording. Similarly, in podcast production, synthetic voices can now be easily incorporated, while retaining the speaker's original tone and emotions.
While advancements in multilingual voice synthesis are quite remarkable, there are still hurdles to overcome in creating completely consistent and authentic vocal modulation. Ongoing efforts are needed to refine these technologies and ensure that they reach their full potential. The impact of these evolving technologies on audio production will likely be far-reaching, encouraging greater linguistic diversity and connecting with a more expansive audience. This trend has the potential to foster a more inclusive audio experience overall, benefiting both content creators and listeners.
Multilingual voice synthesis is rapidly expanding the reach of audio content across the globe. The ability to quickly adapt a cloned voice to different languages is remarkable, leveraging neural networks to analyze linguistic patterns and phonetic similarities. This approach accelerates the process of making content accessible to a broader audience compared to traditional translation and re-recording methods.
However, the increased sensitivity of modern microphones presents a new challenge for audio production. Capturing the subtleties of human voice modulation becomes easier, but simultaneously requires more robust noise reduction techniques. This highlights the crucial role of AI in maintaining the quality of audio produced in a variety of settings.
Interestingly, even capturing the emotional nuances of a voice in a cloned model is becoming a focus of research. While initially, these models focused on replicating vocal sounds, the complexity of capturing emotional context through pitch and speech patterns is now a major area of study. This pursuit of capturing the full range of human expression in synthetic speech is pushing the boundaries of what we can achieve with this technology.
It's surprising how little audio data is needed to create a high-quality voice clone. Deep learning models can extract significant vocal features from just a 10-minute sample. This efficiency democratizes the technology, enabling a wider range of applications—from audiobook creation to crafting personalized messages.
AI noise reduction techniques often utilize spectrogram analysis, visually representing the audio signal. This approach facilitates the separation of vocal elements from noise, leading to clearer audio. This is beneficial across a wide range of audio formats, including podcasts and applications in voice cloning.
Studies indicate that listeners are strongly influenced by the audio quality of a piece of content. Higher quality audio enhances engagement and audience retention, highlighting the crucial role of sound engineering. This is especially pertinent in competitive mediums like podcasting, where audio quality can significantly impact listener experience.
Synthetically recreating acoustic environments is also within reach of current voice synthesis technologies. By manipulating voice characteristics, producers can create the illusion of narrations taking place in a concert hall, a library, or even a whispering forest. This adds a layer of immersion and realism that was previously unavailable.
AI-driven noise reduction technologies are becoming more adaptable, learning from listener feedback to optimize noise gate thresholds and cater to individual sound preferences. This ability to personalize the listening experience is crucial for broader user acceptance.
Furthermore, AI algorithms can effectively reduce unwanted noise while preserving the unique timbre of a voice. This is vital for voice cloning, where maintaining the authenticity of the cloned voice is paramount.
The increasing availability of computing power has enabled many voice cloning systems to operate in real-time. This feature not only speeds up audio production but also paves the way for live applications such as interactive storytelling and real-time voice-over work, promising to further blend personalized interactions with a wider reach of global content.
Voice Cloning Meets Operations Research Optimizing Audio Production Workflows - Adaptive Learning Algorithms Improve Voice Style Control
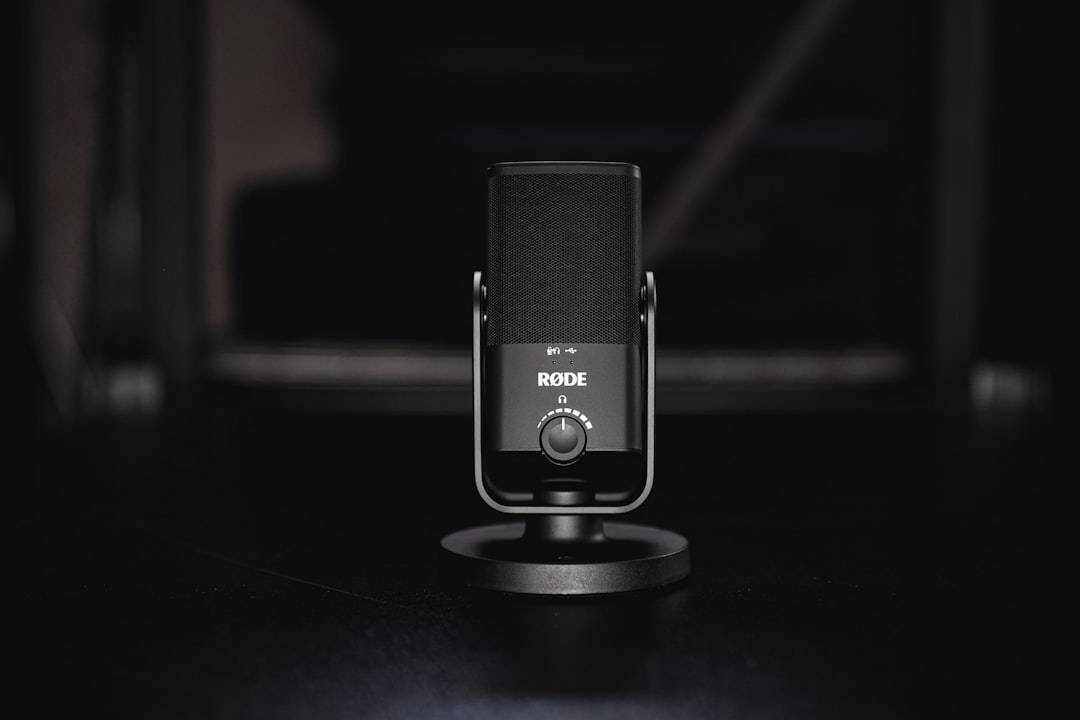
Voice cloning technology is becoming more sophisticated, thanks in part to adaptive learning algorithms that are improving our ability to control the style of synthetic voices. These algorithms allow for finer adjustments to aspects like emotion, accents, speech rhythm, and intonation, leading to more natural and expressive synthetic speech. This is especially valuable for applications like audiobook production and podcasting where the voice's character and emotional impact are central to the listener's experience.
However, achieving a seamless and natural transition of voice styles remains a complex challenge. This is particularly true when dealing with limited data, known as few-shot style transfer, where the objective is to shift the stylistic qualities of one voice onto another. Machine learning models struggle with separating speaker identity from prosody (the rhythm and intonation of speech), making it a difficult task to fine-tune voice characteristics without impacting the core sound of the voice.
Despite these challenges, ongoing development in adaptive learning algorithms holds the potential to refine voice control even further. The pursuit of highly realistic and adaptable synthetic voices must be balanced with maintaining the artistic integrity of audio content. As the technology advances, it will be interesting to see how this delicate interplay between innovation and authenticity evolves in shaping the future of audio experiences.
Adaptive learning algorithms are increasingly being used to refine control over voice styles in voice cloning systems. This dynamic approach allows the system to learn and adapt to user preferences in real time, resulting in more nuanced and expressive synthetic voices. One exciting development is the ability of these algorithms to detect subtle emotional cues within a human voice, which can then be replicated in the cloned voice. This leads to a more human-like and engaging listening experience, as the synthetic voice can express a wider range of emotions.
Surprisingly, these advanced algorithms can generate highly accurate voice clones from relatively small audio samples, sometimes as little as 10 minutes. This significant reduction in data requirements has democratized the technology, making it more accessible and adaptable to a broader range of applications. Furthermore, adaptive learning shines in multilingual voice synthesis, efficiently learning phonetic and tonal variations across languages, leading to high-quality voice clones in multiple languages.
These systems can also adapt the voice style to the context of the audio content, for example, adjusting the tone of an audiobook narrator based on the narrative's emotional trajectory. This contextual customization adds a new layer of sophistication to storytelling through audio, effectively enriching the listener's experience. The algorithms are also designed to continuously learn and improve, adjusting voice models based on new data and user interactions. This ensures that the synthetic voice remains relevant and in line with evolving preferences.
However, the quest for the perfect balance between preserving a speaker's personality and achieving accurate voice modulation remains a challenge. Adaptive learning algorithms strive to address this delicate interplay, seeking to faithfully recreate the nuances of a person's voice while ensuring the overall quality of the synthetic speech. Real-time applications, such as live podcasts or interactive storytelling, require the algorithms to modify the voice in response to live inputs or changing environments.
Interestingly, the quality of the recording equipment significantly impacts the effectiveness of adaptive algorithms. Even the most advanced software struggles to produce high-quality synthetic voices when the input audio is of poor quality, illustrating the symbiotic relationship between hardware and software in audio production. Ultimately, the goal of adaptive voice style control is to enhance the listener experience by allowing individual preferences to shape the characteristics of the cloned voice. This results in a more personalized audio experience, which is likely to be crucial for the widespread adoption of this technology.
Voice Cloning Meets Operations Research Optimizing Audio Production Workflows - Short Audio Samples Enable Rapid Voice Replication
Recent advancements in voice cloning have demonstrated the ability to rapidly replicate voices using remarkably short audio samples. Utilizing snippets as brief as 10 seconds, sophisticated systems can generate incredibly accurate digital copies of a person's voice, capturing distinctive features like their accent, unique vocal tone, and even the subtle emotional nuances they convey. This breakthrough in speed and efficiency is transforming audio production workflows in diverse areas, including audiobooks and podcasts. It permits the creation of personalized and tailored audio experiences that can resonate more strongly with listeners.
While this rapid development presents exciting possibilities, concerns related to potential misuse in the creation of deepfakes and the impact on the perceived authenticity of synthetic voices cannot be ignored. As the field progresses, it will be crucial to carefully navigate the ethical implications and ensure that the technology is utilized responsibly. The path forward necessitates a mindful balance between fostering innovation and mitigating the potential risks that accompany this powerful technology.
The efficiency of voice cloning is quite remarkable, with some systems requiring as little as 10 seconds of audio to create a convincing replica of a person's voice. This drastically reduces the time and resources needed to create voice-overs for a variety of applications, making voice cloning a much faster process than previously possible. However, this efficiency also raises some interesting questions about the potential for misuse of this technology in creating deepfakes or manipulating audio content.
The speed at which voice models can produce a replicated voice is also impressive, often completing the process in just 100 milliseconds. This rapid output allows for real-time applications, such as having a synthetic voice narrate live events or creating interactive stories where the voice adapts to user choices in a natural manner. It’s exciting to see how this speed can open up new areas of creativity.
What's truly fascinating is the increasing sophistication of these voice models. They can now replicate not only the sounds of a voice, but also the emotional tone and cadence. They are able to manipulate pitch and speech patterns to convey feelings like happiness or sadness, providing a depth that was previously unavailable with traditional text-to-speech systems. This represents a real leap forward in capturing the essence of human expression with artificial voices.
Furthermore, these systems are becoming increasingly adept at generating different languages. They can smoothly shift between languages while preserving the original speaker's voice quality and emotional delivery. This opens up new possibilities for reaching global audiences without the need to re-record content in multiple languages. However, the accuracy of the synthesized speech across all languages is still a challenge that researchers are actively working to address.
Another interesting aspect is the capacity of some systems to simulate different environments. They can add artificial acoustic elements to the voice, making it sound like it’s coming from a concert hall or a quiet library, effectively creating a more immersive audio experience. While this feature is still in its early stages, it suggests future audio production might include not only vocal sounds but also customized acoustic settings tailored to the story or genre.
The synergy between noise reduction and voice cloning is another intriguing element. Modern noise reduction techniques are increasingly integrated into voice cloning processes to clean up the audio and separate the desired speech from background noise. This collaborative approach significantly enhances the clarity and overall quality of the synthesized voice. However, finding the right balance between noise reduction and maintaining the voice's natural characteristics is a balancing act that needs to be carefully addressed.
Research continually underscores the importance of audio quality in engaging the audience. Studies show that high-quality audio leads to better engagement and improved retention in listeners, whether it’s a podcast or an audiobook. This emphasizes the importance of investing in the technology that helps produce high-fidelity sound for these media formats. The improvements to both audio quality and noise reduction are becoming paramount to enhance the audience experience.
Adaptive learning algorithms are playing a key role in enhancing the control we have over the style of a voice. These algorithms adjust in real-time based on the context of the story or the audience’s feedback. This allows us to refine and manipulate the emotions conveyed by a synthetic voice, providing better control over its expression and responsiveness. Yet, balancing that control with the preservation of the unique characteristics of the speaker's voice remains an ongoing challenge.
The quality of the original audio recording is another key factor that impacts the overall performance of voice cloning. Even with the most advanced algorithms, a poor-quality recording will limit the quality of the synthetic voice. This relationship highlights the essential link between hardware (recording equipment) and software (voice cloning algorithms), both needing to be of a certain level to achieve optimal results.
Finally, there's a creative potential unlocked by these systems. They can provide an adaptable resource for podcasters, acting as a readily available co-host or guest speaker. This ability adds a new dimension to podcasting and storytelling, allowing producers to experiment with creative concepts without logistical constraints. However, some worry this capability may reduce the demand for human voice talent and introduce concerns about originality and artistic integrity in a field already experiencing changes.
While there are challenges to overcome, voice cloning technology has undeniably progressed to an impressive level of sophistication. It continues to evolve at a rapid pace, unlocking new ways to interact with and experience audio content, raising interesting questions about how this technology will be integrated into our audio futures.
Voice Cloning Meets Operations Research Optimizing Audio Production Workflows - Integration of Operations Research Optimizes Studio Workflows
The incorporation of operations research methods is transforming audio production, particularly in areas like voice cloning, podcast creation, and audiobook production. These methods, including optimization algorithms and machine learning, help studios handle the intricate aspects of sound design and voice generation more effectively. Through data analysis and process refinement, studios can optimize resource allocation, streamline their workflows, and make better-informed decisions that contribute to higher production quality. This convergence of fields promotes efficiency and faster iteration cycles, providing a more agile and adaptable approach to content creation. However, the increasing use of such technologies raises important questions about the artistic integrity of audio content and the impact on roles traditionally held by human audio professionals. As operations research continues to intersect with the ever-evolving landscape of audio technologies, studios will need to carefully weigh the benefits and the potential drawbacks of this convergence.
Voice cloning's ability to accurately recreate a voice from mere seconds of audio is quite astonishing. This feat, driven by improvements in deep learning, is revolutionizing podcast and audiobook production, offering streamlined workflows and exciting creative possibilities. It's remarkable how quickly these systems can now replicate a voice—often within 100 milliseconds—opening the door for real-time applications like live narrations or interactive storytelling, where a synthetic voice responds seamlessly to user input. Beyond mimicking vocal sounds, the technology is gaining ground in capturing human-like emotions. These advanced voice models can now be adjusted to convey feelings like happiness or sadness, enriching the listening experience by moving beyond the often robotic sound of older text-to-speech.
The capacity to simulate various acoustic settings is also intriguing. Producers can create an illusion of specific environments—think a concert hall or a bustling café— by manipulating the audio characteristics of the voice. This adds a layer of immersion previously unheard of in audio content. It's important that these new abilities are coupled with AI-driven noise reduction techniques integrated into voice cloning, further refining audio quality. They filter out background sounds, enhancing the listener experience and ensuring the voice’s distinct qualities remain intact.
Furthermore, this technology has enabled a major leap forward in providing a more global reach to audio content. Voices can be quickly adapted to multiple languages without losing the original emotional tone and nuances, making audiobooks and podcasts more accessible to worldwide audiences. Interestingly, adaptive learning algorithms allow for the creation of even more customized audio experiences. The algorithms can refine and alter a voice's characteristics based on listener preferences or the context of a story, leading to a truly personalized listening experience. AI systems also learn from user interactions, adapting things like noise levels based on individual preferences. This constant learning and refinement ensures that the audio quality remains high and enjoyable for listeners.
However, the pursuit of perfectly replicating a voice while minimizing background noise presents a complex balance. Striking the right chord between eliminating unwanted sounds and maintaining the voice's uniqueness is crucial in voice cloning. The rapid development of voice cloning technologies also brings forth questions about potential ethical misuse. Creating convincingly realistic deepfakes is a concerning possibility. As this field develops at an impressive pace, it's imperative that alongside innovation comes a framework that ensures the technology is used responsibly to avoid undermining trust in the authenticity of audio content. This responsible usage is essential to guarantee that the incredible potential of voice cloning serves a positive purpose and adds to the breadth and depth of audio experiences, rather than undermining them.
Get amazing AI audio voiceovers made for long-form content such as podcasts, presentations and social media. (Get started for free)
More Posts from clonemyvoice.io: