Voice Cloning Meets Array Algorithms Enhancing Audio Production Efficiency
Voice Cloning Meets Array Algorithms Enhancing Audio Production Efficiency - Array Algorithms Boost Voice Synthesis Quality
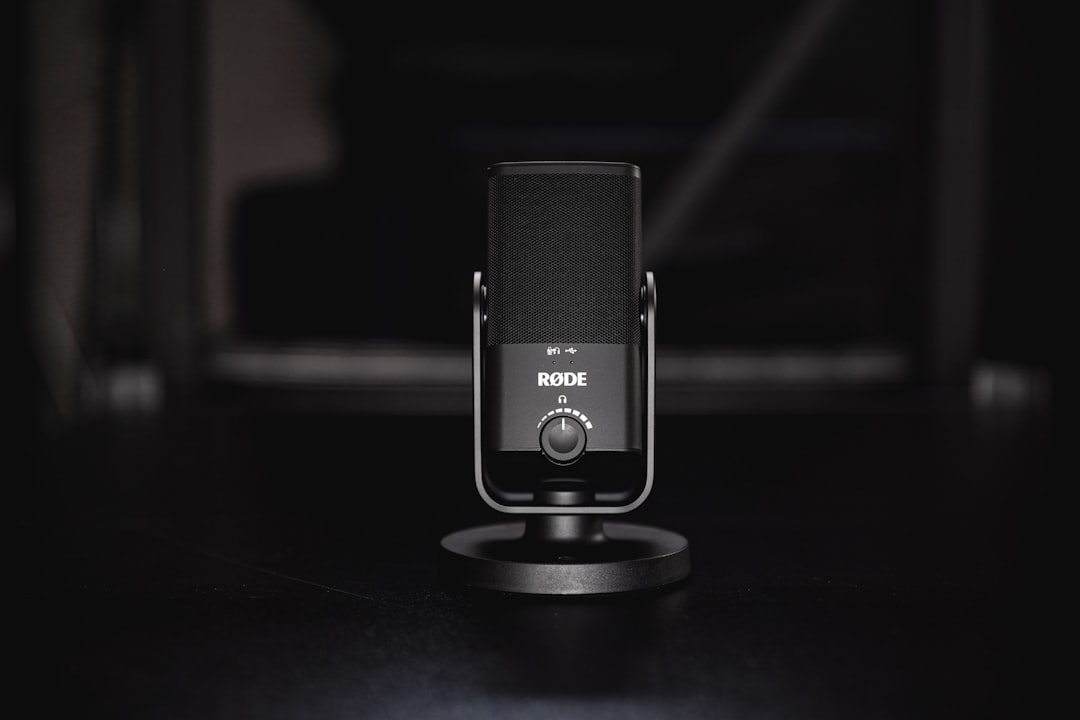
The application of array algorithms is emerging as a key factor in refining the quality of synthesized speech. These algorithms are specifically designed to optimize the processing of audio signals, leading to a reduction in unwanted noise and other sonic imperfections. This translates to a cleaner, more realistic sound output, which is highly beneficial for various applications including voice cloning for audiobooks and podcast creation.
The quest for improved voice cloning fidelity has spurred interest in incorporating more advanced algorithms. This approach not only improves the listening experience but also contributes to the development of more robust and capable voice cloning systems. Further research into these algorithms and their applications promises to bridge the divide between artificial voices and human vocal expressions, pushing the boundaries of what's achievable in audio synthesis. The ultimate goal is to create synthesized speech that is nearly indistinguishable from a genuine human voice, further enhancing the potential of these technologies.
Array algorithms are increasingly being explored in voice synthesis, particularly due to their potential to fine-tune the spectral makeup of synthesized audio. By meticulously manipulating the frequency components of sound, these algorithms can recreate subtle variations in vocal tones and textures, potentially leading to a more natural and lifelike synthetic voice.
The application of adaptive filtering within array algorithms offers a powerful method for noise suppression in voice recordings. This capability is especially beneficial in less-than-ideal recording environments common in podcasting or audiobook production, where ambient noise can degrade the clarity of the spoken word.
Interestingly, research using multi-channel audio systems has shown that array algorithms can effectively simulate the spatial distribution of voices within an audio landscape. This spatial manipulation allows for a more immersive experience when listening to podcasts or audiobooks with multiple speakers, as listeners can more easily differentiate between various voices in the scene.
Applying array algorithms to voice cloning allows for detailed analysis and synthesis of accents and dialects. This means a voice actor or synthesizing tool can potentially create diverse and nuanced character voices by processing different voice samples through array techniques, potentially broadening the scope of storytelling and voice customization.
Temporal analysis using array methods can provide a way to understand the dynamic evolution of voices over time. This is especially valuable for synthesizing voices in a way that maintains smooth dialogue flow in complex narratives with many characters, a common characteristic of audiobook productions.
The marriage of machine learning with array algorithms seems to hold promise for advancing how we control vocal pitch and intonation. This could unlock opportunities for synthesized voices to express a wider range of emotions, adding a degree of authenticity to storytelling through mediums like audiobooks and podcasts.
Integrating array algorithms into real-time voice synthesizers could be a valuable approach to minimize latency during live interactions. This feature could play a significant role in improving the responsiveness of podcasting formats, where spontaneous discussions with guests are common.
While the promise of better compression through array algorithms exists, this area still needs further refinement. The ability to significantly reduce audio file size without sacrificing audio quality could be a big boost to the distribution of podcasts and audiobooks, potentially improving user accessibility and content delivery.
One area where array algorithms can show their strength is handling the inherent variability of vocal tract characteristics and the acoustic environments in which recordings are made. By being able to compensate for variations, it could mean greater consistency in voice quality across a variety of settings.
Finally, array algorithms in advanced simulation settings can potentially enhance our understanding of how listeners perceive and react to synthesized voices. By using these models, audio producers might be able to tailor recordings more precisely to specific demographic preferences, which could optimize audience engagement with different forms of audio content.
Voice Cloning Meets Array Algorithms Enhancing Audio Production Efficiency - Real-time Multilingual Audio Output Advancements
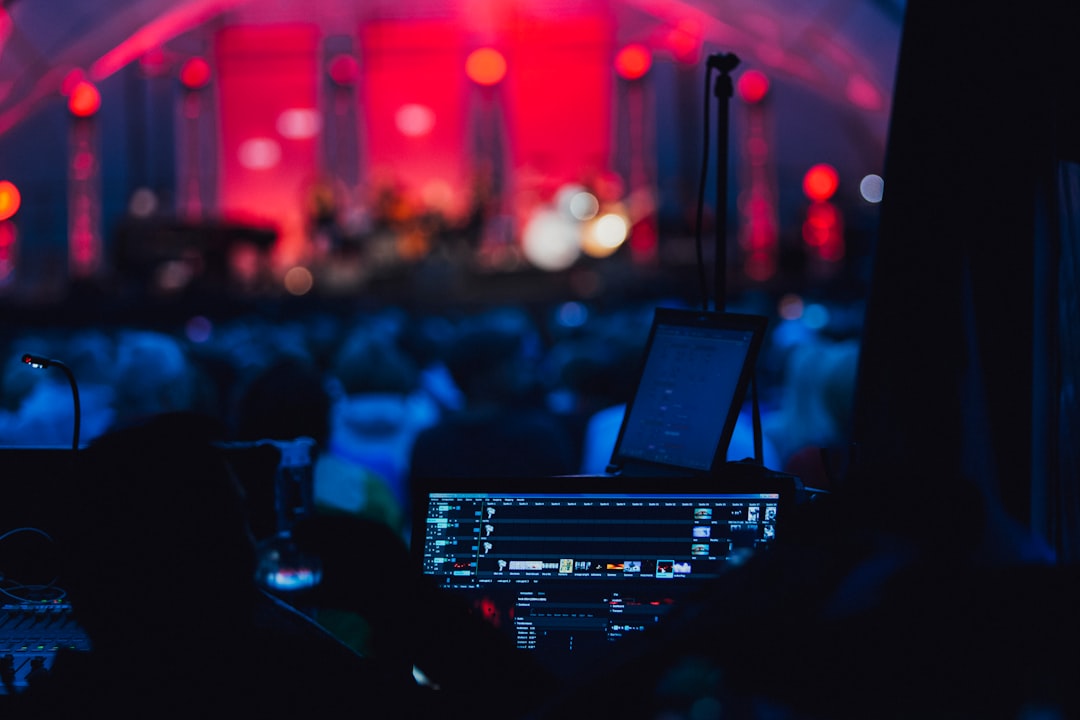
The field of real-time multilingual audio output is experiencing a surge in advancements, making significant strides in creating more natural and fluid synthesized speech across various languages. These developments are fueled by sophisticated deep learning approaches within voice cloning technologies, enabling the generation of remarkably realistic speech in a wider array of languages. This is particularly crucial as the demand for audio content expands globally.
Improvements in noise reduction algorithms play a critical role, enhancing the overall quality of the synthesized audio. This increased clarity and precision benefit a broad range of applications, including audiobook production and podcast creation. Furthermore, innovations in low-latency voice conversion are leading to a more seamless and engaging experience, particularly in scenarios demanding immediate interaction.
While these advancements are promising, they also bring to light the need for careful consideration regarding the authenticity of synthesized speech. Striking a balance between artificial and genuine human expression remains a key challenge that continues to drive research within this domain. The goal is to harness these technologies to create increasingly compelling audio content, without compromising the perceived naturalness of the voice.
Real-time multilingual audio output is becoming increasingly sophisticated, with the ability to dynamically adapt accents based on context or user feedback. Imagine a voice synthesis system that smoothly adjusts its accent to better connect with listeners in diverse settings—a feature that could significantly improve the relatability of conversations in multilingual environments.
Convolutional neural networks have shown promise in generating synthetic speech that can seamlessly transition not only between languages, but also between regional dialects. This capability could potentially lead to a new era of hyper-personalized audio experiences, allowing users to tailor content to their specific preferences within multilingual landscapes.
Exciting new techniques in spectral audio processing offer the potential to isolate individual phonemes from a speaker's voice and translate them in real-time, while still preserving the speaker's unique vocal characteristics and pronunciation. This is especially compelling for podcasting scenarios with multilingual conversations, where maintaining the authenticity of individual speakers' voices is crucial.
Voice cloning technology is now leveraging recurrent neural networks to analyze and replicate emotional cues within speech. Synthesized voices are becoming capable of not just speaking different languages, but also conveying emotions like urgency, joy, or sadness, which can significantly enhance the depth and impact of storytelling in multilingual audiobooks.
Researchers are actively working on algorithms that can seamlessly synchronize changes in pitch and rhythm with linguistic features in real time. The goal is to generate audio outputs that feel more natural and engaging in dynamic multilingual conversations or podcasts with multiple speakers. If successful, these advances could make synthesized speech much more human-like.
The integration of neural acoustic models into voice synthesis pipelines is leading to significant improvements in the predictive accuracy of synthesized voices across various languages. These models can now account for nuanced phonetic variations within dialects, even with relatively limited training data.
Real-time processing with array algorithms is now enabling instant multilingual output, seamlessly translating between languages without any perceptible delays. This breakthrough could be especially valuable for audiences who frequently switch between languages, providing a smooth and continuous listening experience.
Machine learning techniques are being employed to achieve a better balance in multilingual output. Producers can now adjust the proportion of different languages within a piece of audio, allowing for customized listening experiences in podcasts and educational content.
Advanced phase vocoders are being utilized to fine-tune the temporal characteristics of synthesized speech, significantly improving the realism of multilingual audio in real-time. This technology can help create voices that not only sound authentic but also reflect the nuances of a given context.
The emerging field of psychoacoustic modeling is shedding light on how listeners perceive and process multilingual synthesized voices. A deeper understanding of these processes could lead to the development of more engaging audio content specifically designed for diverse audience demographics within audio production workflows.
Voice Cloning Meets Array Algorithms Enhancing Audio Production Efficiency - Two-step Approach for Efficient Voice Cloning
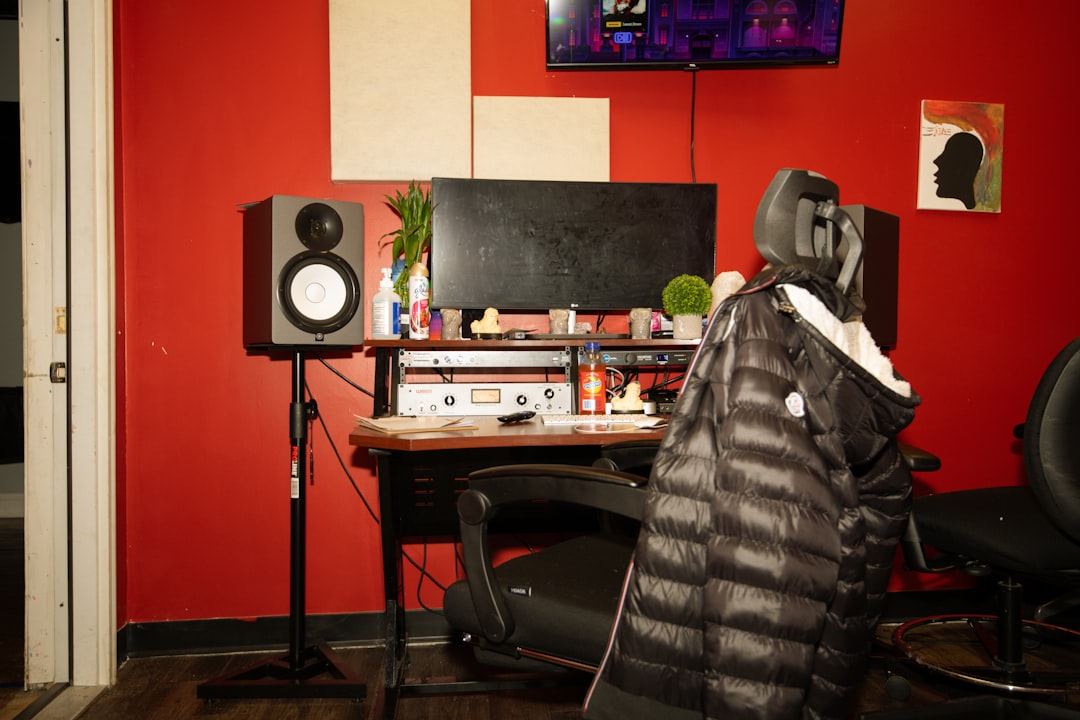
The "Two-step Approach for Efficient Voice Cloning" represents a significant advancement in creating realistic synthetic voices. This approach centers on a two-phase process aimed at enhancing the quality of synthesized audio. The primary focus is on noise reduction and improving the clarity of the voice, crucial for applications like generating audiobooks and podcasts with high fidelity. Through carefully selected algorithms, the approach strives to create a synthesized voice that more closely mirrors its source, capturing a wider range of pronunciation and vocal tones. As voice cloning technology develops, the key challenge is to find a balance between the goal of incredibly lifelike audio and the practical limitations of resources and processing power. This means there are still hurdles to overcome regarding the perceived quality and authenticity of cloned voices. Despite these challenges, the two-step approach offers a promising pathway to creating more natural and captivating synthetic voices, pushing the boundaries of what's possible in audio synthesis.
A promising avenue in voice cloning involves a two-stage process that leverages the power of microphone arrays. These arrays, by capturing audio from numerous directions, greatly enhance the quality of recorded voices, especially in situations where noise and echoes are prevalent – a boon for audio book productions, podcasts, and any application needing high-fidelity voice recordings. This multi-directional capture becomes especially crucial when the recording environment isn't ideal, which is often the case in podcasting, where background noise and variability are commonplace.
Interestingly, this two-step technique shows potential for creating synthesized voices with a consistent identity across different platforms. Whether it's an audiobook, podcast, or even a video game, the voice should sound the same, contributing to a unified listening experience.
Furthermore, the intricate algorithms used in the two-stage process give us an unprecedented level of precision in analyzing voice patterns. We can meticulously capture subtle nuances like breathing and pauses, elements that contribute significantly to the perceived naturalness of synthesized speech. These insights are valuable for improving the flow and emotional delivery in audio narratives, like audiobooks, where maintaining a listener's attention hinges on a seamless and expressive voice.
One of the intriguing aspects of this two-stage cloning method is the ability to incorporate emotion recognition technologies. This means that synthesized voices can be designed to adapt their intonation and speed depending on the intended emotion, significantly increasing the human-like qualities of synthetic voices. However, it remains a challenge to truly replicate the range and complexity of human emotions convincingly, and it is an area ripe for further investigation.
These methods also significantly enhance the robustness of voice cloning in noisy settings. Advanced noise-filtering techniques can dynamically suppress unwanted sounds in real-time, beneficial for diverse applications like live podcast recordings or outdoor voice-over productions. Achieving a truly 'clean' audio output in these cases is a constant engineering pursuit, pushing the boundaries of what's currently possible.
Another fascinating feature is the capability to carefully shape the frequency spectrum of a voice. This allows for recreating specific vocal tones with incredible precision. For instance, this could be used in audiobook productions to create diverse character voices by subtly manipulating the tone and timbre of the base voice, making them sound uniquely distinct.
Interestingly, this two-step process provides a pathway for creating voices that seamlessly blend dialectal features from different languages. This capability opens doors to producing audio content where characters effortlessly transition between languages while retaining their unique accents. Imagine audiobooks where characters' voices can shift between English, Spanish, and French while still sounding natural, a fascinating prospect with implications for storytelling.
Furthermore, it could improve accessibility for those with hearing impairments. By accurately isolating and separating different speakers in multi-speaker content, such as podcasts or audiobook scenes, audio production becomes more accessible for individuals relying on hearing aids or assistive listening devices.
Another impressive aspect is the ability to generate character voices in real-time. This opens new opportunities for interactive storytelling and live audio productions, like podcasts, where characters' voices can be adapted spontaneously during a session. This has exciting potential for interactive content and innovative forms of storytelling.
However, a key area of focus going forward is for the system to effectively learn from ongoing interactions with listeners. It could potentially adapt to audience preferences, adjusting things like accents and speech patterns over time. This capacity for personalized adaptation can elevate the listener experience, making synthesized voices feel more like genuine human expressions. The development of these dynamic learning capabilities continues to be a key area of research, attempting to bridge the gap between human and artificial voice.
Voice Cloning Meets Array Algorithms Enhancing Audio Production Efficiency - Noise Reduction Models in Voice Replication
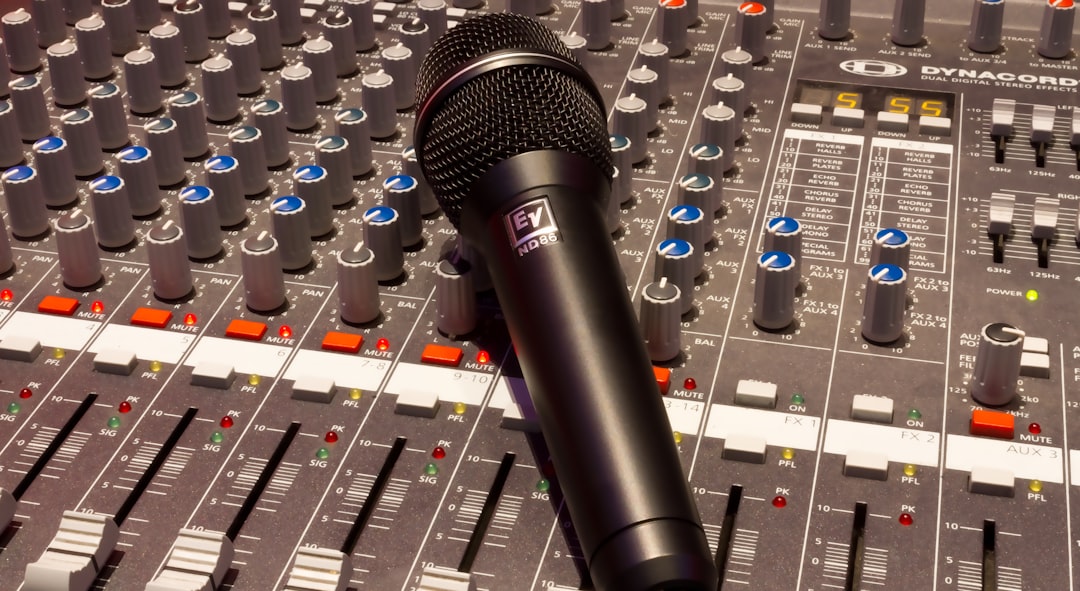
Within the field of voice replication, noise reduction models are gaining prominence as a means to enhance the quality of synthesized speech. These models employ sophisticated algorithms to eliminate unwanted background noises and other sonic imperfections, leading to clearer and more natural-sounding voice outputs. This is especially beneficial for applications such as podcast and audiobook production where a high degree of audio fidelity is essential for a positive listening experience. The development of these models is focused on effectively replicating human vocal characteristics while maintaining a high level of audio quality, aiming to bridge the gap between artificial and natural voices. However, the successful implementation of noise reduction techniques within voice cloning technology remains a complex challenge. Future research will need to address this complexity to find a balance between noise reduction efficiency and maintaining the perceived authenticity of the cloned voice.
In the realm of voice replication, noise reduction models are playing an increasingly crucial role in refining the quality of synthesized audio. One common technique is adaptive equalization, which automatically adjusts the audio's frequency response to minimize interference and enhance the clarity of the target voice. This helps to isolate and emphasize the desired vocal components, making them stand out from distracting background noise.
A powerful approach to noise reduction is spectral subtraction, where the noise characteristics are estimated and digitally removed from the audio's spectrum. This method focuses on cleaning up the frequency content, effectively isolating and boosting the frequencies that contribute to the vocal component of the audio. This is particularly important for creating synthesized speech that remains intelligible even in environments with noticeable background sounds.
Many sophisticated models also utilize waveform analysis, constantly monitoring and adapting to shifts and variations in the audio signal over time. This is essential for maintaining noise reduction effectiveness in dynamic environments. This continuous analysis is especially useful in scenarios like podcast recordings, where background noise can vary significantly over the course of a session.
The integration of microphone arrays brings another dimension to noise reduction. By analyzing the spatial distribution of sound captured from multiple microphones, these models can use multi-dimensional filtering to pinpoint and suppress unwanted sounds. This approach improves the precision of noise removal, leading to a more effective noise reduction in intricate acoustic settings, a common scenario in many audio production tasks.
The importance of phase coherence in noise reduction is also worth noting. These techniques strive to maintain the audio's natural temporal structure while filtering out extraneous noise. By preserving the timing and phasing relationships within the audio, it's possible to maintain a natural sounding rhythm and intonation of the synthesized voice. This approach directly contributes to a more immersive and engaging listening experience.
Machine learning has started to make a significant contribution to the field of noise reduction, equipping models with the ability to learn from a wide range of acoustic environments. This adaptive learning allows noise reduction systems to generalize and improve their performance in real-world situations, making them more effective in a variety of contexts, such as recording audiobooks in a variety of settings or creating podcast content in diverse locations.
Temporal masking techniques build upon the characteristics of human hearing to enhance noise reduction. They leverage the phenomenon where our auditory system tends to miss quieter sounds if they occur immediately after or before louder sounds. This property can be used to strategically suppress background noises while retaining vocal clarity. It's another tool in the noise reduction toolbox for cleaning up synthesized voices and making them sound clearer.
Despite significant advancements in this domain, existing noise reduction models still face challenges when confronted with non-stationary noise. This refers to noise patterns that change their intensity or frequency over time, creating a dynamic interference that's harder to filter out. Addressing this type of noise remains a key challenge in this field, highlighting the ongoing research and development needed to make voice cloning and other audio synthesis tasks more robust.
It's important to acknowledge that sometimes the pursuit of vocal clarity can inadvertently diminish the perceived human-like characteristics of the synthesized voice. Engineers face a delicate balance: striving for crystal-clear audio while ensuring the voice still retains a natural quality. This pursuit of a harmonious balance underscores the complexity of audio synthesis.
Furthermore, while the improvements in noise reduction are valuable, it's also important to consider the computational costs of real-time processing. Noise reduction algorithms, especially those that utilize machine learning, can be computationally intensive. This can pose challenges when implementing these models in real-time audio applications. The challenge is to maintain the advantages of these methods without sacrificing efficiency and scalability, highlighting the need for ongoing optimization efforts.
Voice Cloning Meets Array Algorithms Enhancing Audio Production Efficiency - Audiobook Production Transformed by AI Voices
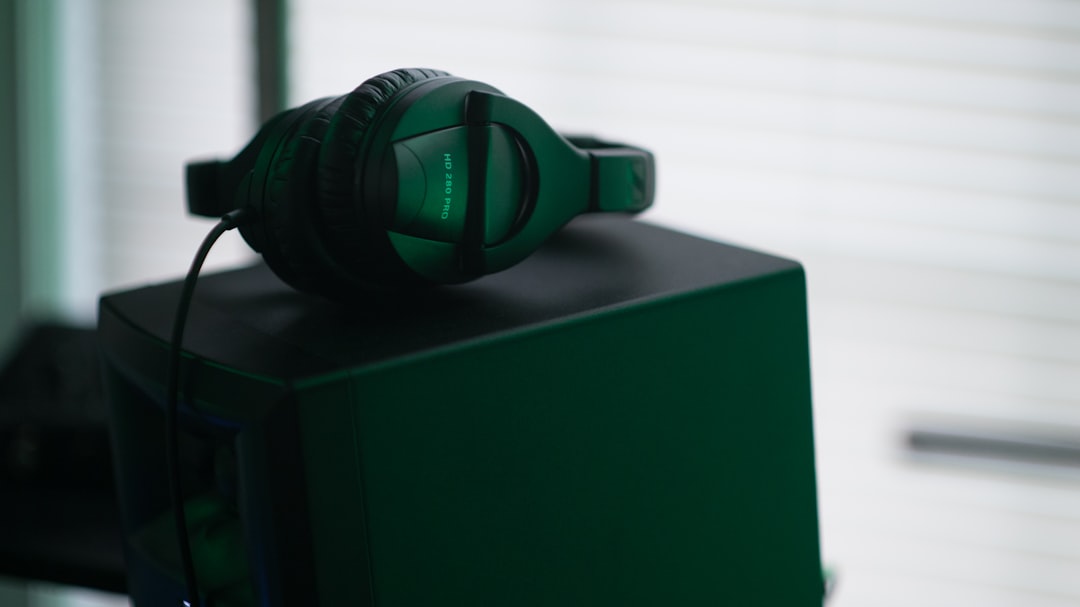
AI-powered voices are revolutionizing audiobook production, offering new avenues for customization and efficiency. The use of synthetic voices and generative AI allows for a wider range of narration styles and speeds, making it easier to create audiobooks that cater to diverse audiences. Platforms offering a multitude of AI voices, some employing voice cloning techniques, have emerged, enabling authors and producers to select voices that match the tone and style of their books. This shift, while promising, presents some challenges. Maintaining a natural and emotionally nuanced listening experience with synthesized voices remains a hurdle, as does the potential for the technology to alter the inherent dynamics of storytelling in audio formats. The continuous evolution of AI voices will likely further blur the lines between synthetic and human narration, shaping the future of audiobooks and audiobook production in unexpected ways.
AI voice technologies are significantly transforming audiobook production, moving beyond basic text-to-speech to capture a much wider range of human vocal nuances. The ability to replicate subtle aspects like vocal rhythm, inflection, and even the subtle sounds of breathing adds a layer of realism and emotional depth to synthesized voices, making audiobooks sound more lifelike. This advancement is driven by improvements in machine learning, which allows algorithms to learn the intricate characteristics of individual speakers, including variations in vocal timbre. This capability extends beyond simple voice replication, enabling dynamic adaptation of voice profiles, making it easier to create believable and varied character voices for stories.
Convolutional neural networks (CNNs) are also impacting audio production, allowing for real-time manipulation of vocal elements like pitch and tone. This control over the sound of the synthesized voice is particularly helpful when generating audiobooks that include multiple characters or require emotional expression. Furthermore, researchers are employing psychoacoustic techniques to better understand how listeners perceive synthesized speech. Insights gathered from these studies can help tailor voice cloning outputs to maximize engagement by considering listeners' auditory preferences.
The development of sophisticated noise-canceling methods like digital beamforming has also become crucial for improving audio quality in audiobooks and podcasting. By focusing on capturing audio from specific directions, producers can significantly minimize unwanted background noise, resulting in cleaner and higher-fidelity recordings. In addition, voice cloning algorithms are incorporating unique biometric features like vocal tract dimensions into their models, allowing for more accurate reproduction of regional accents and dialects. This enhances the authenticity of audiobook characters, especially those originating from diverse backgrounds.
Recent work in this area has demonstrated the ability to create "dynamic" voices that evolve during playback, adjusting the spectral characteristics of the synthetic audio to reflect changes in context or emotion. This brings a new level of naturalism to synthesized speech, mimicking the way human voices shift in response to their emotional state. Also, the implementation of array algorithms has improved the speed at which audio inputs are processed, significantly decreasing latency. This is particularly important in interactive audio formats like podcasts, where prompt responses and engaged conversations are vital.
The increased sophistication of multi-frequency noise reduction strategies has allowed for a better separation between desired vocal frequencies and extraneous noises. This enhancement leads to clearer audio outputs, particularly in recordings made in acoustically challenging environments, resulting in much better quality. Additionally, engineers are investigating the potential for interactive dialogue systems within audiobooks. This could enable the voice synthesis to adapt to user interactions, such as audience engagement metrics or direct input, creating a more personalized and engaging listening experience. This research is pushing the boundaries of audiobook experiences by exploring new dimensions of dynamic storytelling and adaptive audio content.
While these advancements are exciting, the field still faces challenges like balancing noise reduction with the preservation of the natural qualities of a voice. Maintaining a balance between high-fidelity audio and the authentic sound of a human voice continues to be a focus for researchers. Nevertheless, the field of AI voice technology shows immense promise for pushing the boundaries of how we create, distribute, and experience audio content across a wide variety of mediums.
Voice Cloning Meets Array Algorithms Enhancing Audio Production Efficiency - Balancing Technological Progress with Ethical Concerns
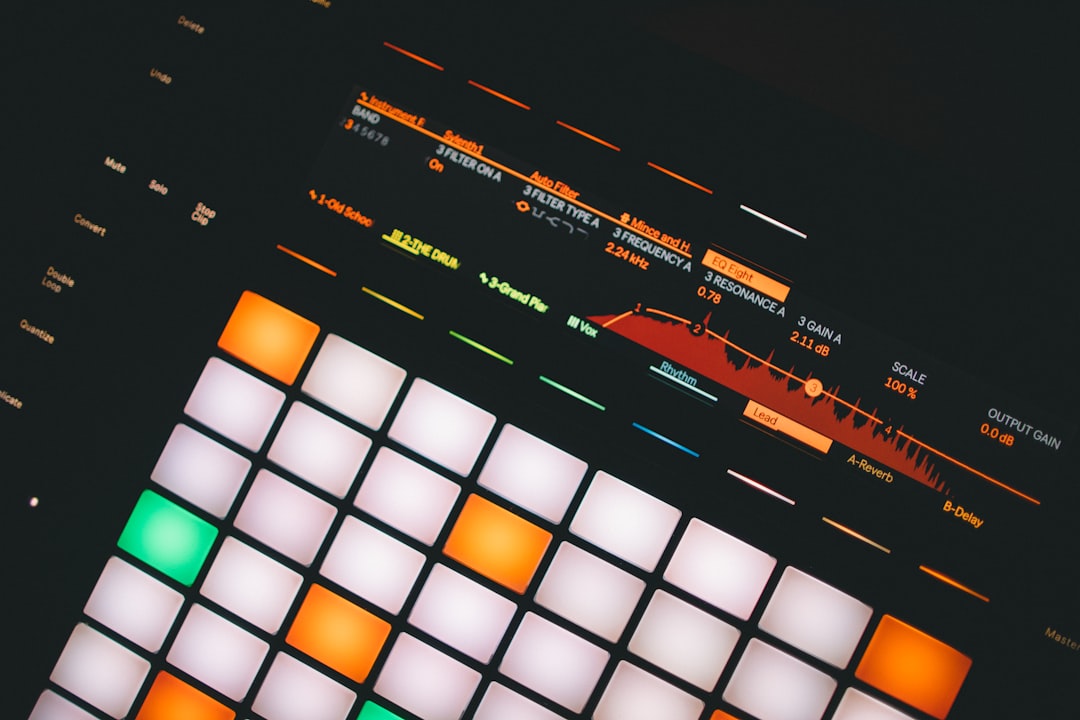
The rapid progress in voice cloning technology has brought with it a growing awareness of the ethical implications associated with its use. The ability to create incredibly realistic synthetic voices raises concerns about potential misuse, particularly the risk of individuals being impersonated or their identities manipulated without their knowledge or consent. While voice cloning can undoubtedly enhance audio production efficiency in fields like audiobook creation and podcasting, ensuring responsible development and deployment is crucial. Finding the right balance between fostering innovation and upholding ethical standards is paramount. Open and continuous discussions regarding regulations, obtaining proper consent from individuals whose voices are being cloned, and respecting individual rights are essential. This ongoing effort to manage the potential risks while maximizing the benefits will define the future landscape of voice cloning applications.
The evolution of voice cloning technology is fascinating, with recent advancements extending beyond simple voice replication. For instance, we're seeing a focus on capturing individual vocal characteristics, like the unique shape of a person's vocal tract, within the cloning process. This detail helps synthesized voices better mimic regional accents and individual speech patterns, making them sound more authentic. Furthermore, the integration of emotion recognition algorithms into voice synthesis is quite promising. These algorithms attempt to analyze natural speech and replicate the subtle emotional cues present within it, such as urgency or sadness. This capability has the potential to significantly deepen the emotional impact of character voices in audiobooks and other narrative formats.
Array algorithms are playing a more prominent role in not only cleaning up synthesized voices but also in manipulating the way sound is perceived. They can create the sensation of sound coming from specific directions, which is especially important when creating engaging audio experiences with multiple characters in an audiobook or podcast scenario. It's intriguing how we can manipulate audio in such a way to enhance the listening experience. Another noteworthy advancement is the development of dynamic voice adaptation techniques. This involves manipulating a voice in real time to match the context of a story or its emotional tone. This concept allows audiobook characters' voices to adapt and evolve as the narrative progresses, much like the way human voices change in response to their emotions.
The pursuit of low-latency voice synthesis through the application of array algorithms is particularly interesting, especially within the context of live podcast production. Lower latency means a more seamless and interactive experience, allowing for more natural-sounding conversations, an area ripe with potential for more immersive storytelling. Analyzing the temporal aspects of speech, such as the rhythm and flow of dialogues, is also critical for creating engaging audiobooks. By studying the timing of speech within complex narratives, we can engineer smoother transitions between characters and maintain the listener's engagement throughout intricate storylines.
Noise reduction is, of course, essential for high-quality audio production. Using microphone arrays allows us to implement multi-dimensional noise filters that can more effectively eliminate background interference in recording environments. This is especially useful when working in spaces where ambient noise can be problematic, such as during podcast recording or audiobook creation. The incorporation of machine learning techniques within these systems is interesting, as we are finding that voice cloning systems can now learn from listener preferences. This could potentially lead to the development of voices that adapt over time based on the responses and behaviors of listeners, resulting in a more personalized listening experience.
However, challenges persist. One major hurdle is dealing with non-stationary noise – that is, sounds that change their intensity or frequency over time. Effectively filtering these kinds of noises in real-world recording conditions remains a significant challenge. Another area needing more focus is the application of bioacoustics to enhance voice cloning fidelity. Bioacoustics involves studying how voices change based on factors like stress or fatigue. The ability to replicate these nuances in synthesized speech would greatly increase the believability of cloned voices in audio storytelling. This field continues to evolve, and the potential impact of AI voices on audio production and storytelling is still only beginning to be understood.
More Posts from clonemyvoice.io: