Get amazing AI audio voiceovers made for long-form content such as podcasts, presentations and social media. (Get started for free)
Voice Cloning in Healthcare 7 Key Security Protocols for Patient Data Protection in AI Voice Systems
Voice Cloning in Healthcare 7 Key Security Protocols for Patient Data Protection in AI Voice Systems - Biometric Voice Authentication Layers for Patient Record Access
Biometric voice authentication, using the unique patterns in a person's voice, is transforming how we secure access to patient records. This technology acts as a crucial safeguard against unauthorized access to sensitive medical data. It not only makes patient identification more efficient but also provides a necessary shield against the escalating danger of voice cloning, a threat that could compromise the integrity of patient information. Voice biometrics is becoming integrated with electronic health record (EHR) systems, demonstrating its potential to enhance security and streamline the management of healthcare information. This integration facilitates secure access to medical records while reducing the risk of data breaches. While offering considerable benefits, the development and application of these systems must always consider emerging threats. Vigilance regarding potential vulnerabilities is essential to maintain the integrity of these systems and instill patient confidence in the security of their data.
The field of voice biometrics is rapidly evolving, utilizing the intricate tapestry of human speech to authenticate individuals. Research suggests that voice characteristics, encompassing a wide range of attributes like pitch, rhythm, and timbre, can be leveraged for robust patient record access control. This approach offers a potentially powerful alternative to traditional password-based systems, achieving remarkably low false acceptance rates, thereby minimizing unauthorized access risks. While impressive, the advancements in voice cloning technology are raising new challenges. The ability to synthesize near-perfect voice replicas poses a significant concern regarding the vulnerability of voice authentication systems, highlighting the need for robust defense mechanisms.
One of the fascinating aspects of voice recognition is its ability to adapt to the dynamic nature of the human voice. Voice authentication systems employ sophisticated machine learning techniques to accommodate natural vocal shifts that occur over time, owing to aging or fluctuating health. This capacity allows for a more user-friendly experience, as the system learns and adjusts to individual vocal patterns. However, this very flexibility also presents a challenge. External factors, like environmental noise or a temporary change in vocal condition, can impact the accuracy of the system, necessitating the development of algorithms that can effectively calibrate in real-time, considering these unpredictable variables.
Moreover, voice authentication is not solely about identity verification; it also has the potential to reveal aspects of a patient's well-being. Voice analysis can detect subtle emotional changes reflected in vocal tone, which could prove beneficial in remote healthcare scenarios, potentially enabling clinicians to assess a patient's emotional state remotely. Implementing this technology in existing electronic medical record systems could potentially create a more seamless and intuitive patient experience. The use of plugins like SMARTonFHIR integrates voice authentication into the existing healthcare infrastructure, offering a path towards greater access security and potentially streamlining clinician workflows.
The development of such systems requires careful consideration of both security and practicality. The future of secure patient record access may very well hinge on the seamless integration of robust biometric voice authentication systems that can adapt to a range of conditions and offer a secure and user-friendly experience. Ultimately, the success of this endeavor will depend on the ongoing innovation and careful implementation of security protocols to ensure patient data remains confidential and accessible only to authorized personnel.
Voice Cloning in Healthcare 7 Key Security Protocols for Patient Data Protection in AI Voice Systems - Real Time Voice Data Encryption During Medical Consultations
Real-time encryption of voice data is crucial during medical consultations, especially as healthcare increasingly utilizes AI-powered voice systems. This encryption acts as a shield, protecting the sensitive information exchanged during telehealth visits and other consultations. The growing sophistication of voice cloning technology highlights the need for strong encryption measures. This is essential to ensure that only authorized individuals can access the voice data and to prevent malicious actors from potentially manipulating or misusing synthetic voices. The rise of telehealth, particularly after the pandemic, has emphasized the critical importance of maintaining the integrity of medical conversations and ensuring that interactions are authentic. Therefore, establishing strong encryption protocols is fundamental for building trust in these advanced voice technologies while prioritizing patient confidentiality and data security. Failing to implement these measures from the start could erode confidence in these valuable advancements in healthcare.
Real-time voice data encryption during medical consultations employs sophisticated techniques to safeguard the sensitive information exchanged. It often relies on robust encryption standards like Advanced Encryption Standard (AES) with 256-bit keys, the same level used for highly classified government data, highlighting its potential for securing sensitive medical discussions. However, this robust protection presents challenges. The encryption and decryption processes can introduce latency, or delays, into the conversation. Carefully designed algorithms are essential to minimize these delays and maintain the natural flow of a medical consultation – even the slightest lag can impact the quality of care.
Modern systems are increasingly incorporating adaptive encryption techniques that react in real-time to fluctuations in network conditions. This dynamic approach ensures efficient encryption, even with inconsistent internet bandwidth, which is particularly relevant in healthcare where reliable connectivity is paramount. Additionally, some encryption systems are exploring "voice fingerprinting" methods which analyze unique characteristics within an individual's voice. Beyond simple authentication, these techniques could differentiate between authorized personnel and potential imposters, adding a layer of security to the communication channel.
To streamline voice encryption, healthcare applications typically utilize audio compression technologies like Opus or G.722. These methods reduce data size without sacrificing the quality of the audio, ensuring the consultation remains clear while using less bandwidth. It's important to remember that real-time voice encryption safeguards data during transmission, unlike traditional encryption which focuses on data at rest. This continuous protection offers an effective defense against eavesdropping threats, particularly crucial as cyberattacks on healthcare data become more prevalent.
As telemedicine services expand, scaling up secure voice encryption solutions presents a considerable hurdle. Systems must be flexible enough to serve a variety of settings, from small clinics to large hospital networks, maintaining consistent security across the board. Integrating artificial intelligence (AI) into the process offers interesting possibilities. AI algorithms could potentially monitor encrypted voice data in real-time, identifying unusual patterns that may indicate a security breach. This proactive approach could provide an extra layer of protection for sensitive patient data.
Further enhancing security, multi-factor authentication techniques could be integrated with voice encryption. This might involve combining voice encryption with methods like facial recognition or fingerprint scanning, creating a more fortified barrier against unauthorized access. It's also important that these solutions comply with rigorous regulations like the Health Insurance Portability and Accountability Act (HIPAA) in the U.S. These regulations, focused on patient confidentiality, carry significant penalties for non-compliance, underscoring the need for robust encryption in all healthcare settings.
The continuing evolution of AI in healthcare is leading to exciting opportunities for improvement in the field. While the benefits are evident, careful consideration of the potential risks and thorough implementation of security protocols will be paramount to safeguarding sensitive patient information in this new era of digital healthcare.
Voice Cloning in Healthcare 7 Key Security Protocols for Patient Data Protection in AI Voice Systems - Multi Factor Voice Recognition for Healthcare Staff Login Protocols
"Multi-Factor Voice Recognition for Healthcare Staff Login Protocols" represents a crucial development in bolstering security within the increasingly digital landscape of healthcare. This approach utilizes the distinctive characteristics of an individual's voice for initial authentication, but it doesn't stop there. It layers on extra verification steps to create a more robust security barrier for accessing critical systems. The rise of voice cloning technology has highlighted a significant vulnerability in relying solely on voice for authentication. Therefore, integrating multiple verification factors becomes essential to protect sensitive patient data from unauthorized access. By combining voice recognition with other methods, such as unique security questions or one-time passcodes, healthcare organizations can significantly enhance data security. This, in turn, can streamline workflows and improve overall system efficiency, while ensuring only authorized personnel can access sensitive patient information. In essence, multi-factor voice recognition is a proactive response to evolving threats and a pathway towards establishing more secure and reliable healthcare protocols.
Multi-factor voice recognition presents a promising avenue for securing healthcare staff login protocols. Each individual's voice is unique, with characteristics like frequency and harmonics that can be harnessed to create a distinct vocal fingerprint. This principle allows systems to differentiate even between individuals with highly similar voices, highlighting the potential for robust authentication.
However, a person's voice is dynamic, changing over time due to factors like aging or temporary illnesses. The effectiveness of voice recognition relies on algorithms capable of adapting to these natural vocal shifts. Machine learning plays a crucial role, enabling systems to continuously adjust and refine their recognition capabilities, reducing the chance of legitimate users being falsely rejected.
Moreover, these advanced systems aren't just about verifying identity; they can potentially detect subtle emotional cues embedded within a caregiver's speech. This offers an intriguing possibility for remote healthcare settings, providing a way to assess a caregiver's emotional state and potentially aid in mitigating stress or burnout. This is one of the fascinating intersections of voice analysis and healthcare, showing the potential for technology to impact not only security but also well-being.
To achieve robust security, multi-factor voice recognition often integrates other biometric modalities, such as facial recognition or PIN entry, into the authentication process. This layered approach significantly complicates attempts at unauthorized access, as intruders would need to overcome multiple security hurdles. This is especially important in the healthcare sector, where access to patient data is highly sensitive and breaches can have severe consequences.
Healthcare settings can be acoustically challenging, filled with various noises and background conversations. The need for clear and reliable audio input necessitates the implementation of echo cancellation technologies. These systems effectively filter out interfering sounds, ensuring that the voice authentication process isn't impacted by the environment. In addition, the urgency of clinical workflows requires immediate processing and authentication. Consequently, these systems are built on high-performance processors and architectures that can deliver real-time results, a crucial requirement for efficient healthcare operations.
Protecting sensitive data is paramount in healthcare. Voice data collected during the authentication process is typically encrypted with robust methods like AES-256, a standard used for highly confidential government communications. This emphasis on encryption reflects the critical role security plays in this context. While voice characteristics are unique, they can also be susceptible to short-term changes during periods of stress or illness. System engineers face the challenge of developing algorithms that can accurately account for these transient vocal variations, ensuring healthcare professionals maintain access regardless of temporary voice modifications.
During the initial enrollment process, systems generate a unique voice template based on a collection of vocal features. This template serves as the foundation for future authentication attempts, ensuring a personalized and consistent experience for users. However, this also highlights the importance of complying with relevant regulations such as HIPAA. These regulations mandate strong data protection measures and non-compliance can lead to severe penalties. Developers need to consider these regulations from the beginning, embedding them into the system's design to minimize future risks and ensure patient data is appropriately protected.
While multi-factor voice recognition offers a promising path to enhanced security in healthcare, its continued development and implementation must carefully consider the dynamic nature of human speech, alongside regulatory compliance and data security concerns. These challenges highlight the ongoing work needed to truly refine and mature voice-based authentication into a reliable and secure authentication system in healthcare.
Voice Cloning in Healthcare 7 Key Security Protocols for Patient Data Protection in AI Voice Systems - Secure Voice Pattern Storage Through Blockchain Integration
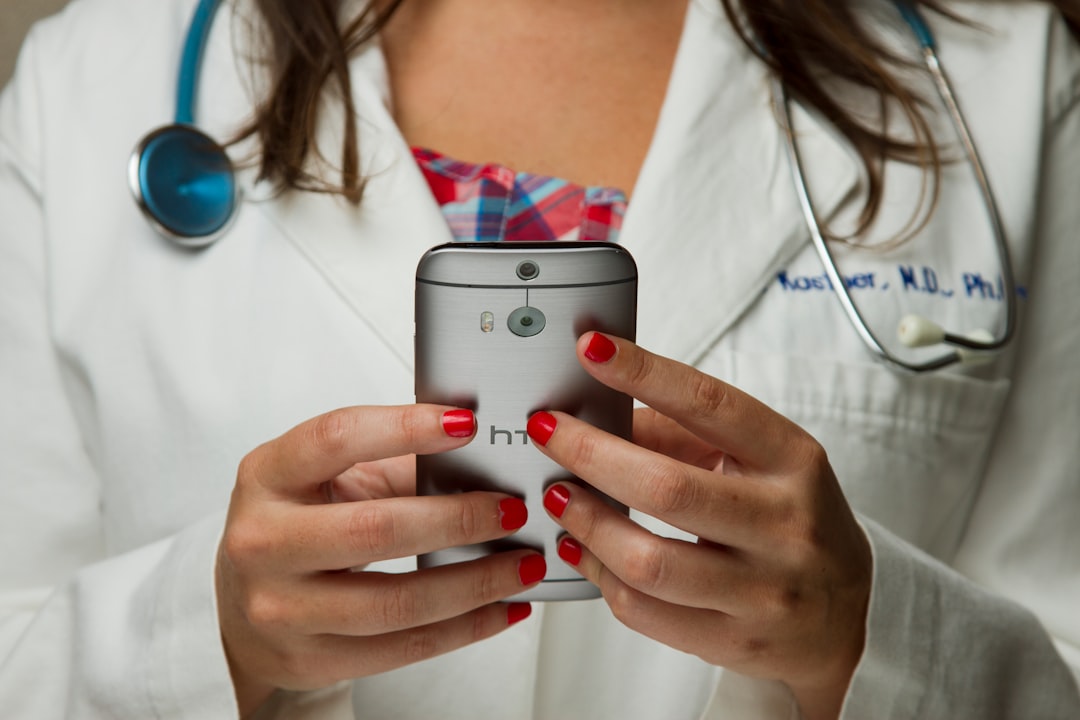
Storing voice patterns using blockchain technology offers a promising way to enhance the security of sensitive voice data, particularly in healthcare. Blockchain's decentralized and tamper-proof nature creates a robust system for storing voice biometrics, reducing the risk of unauthorized access or manipulation. This approach is vital for maintaining the confidentiality of patient interactions and other sensitive audio information. Moreover, it supports smoother data sharing across different healthcare systems while adhering to regulatory guidelines. However, the application of blockchain in healthcare is not without its obstacles. Issues like making sure different systems can easily work together and verifying user identities require solutions to fully realize its benefits. As both artificial intelligence in voice systems and blockchain technology progress, their combined potential could significantly change how we safeguard patient data in the evolving digital healthcare environment. There's still work to be done, but the prospect of a more secure future for voice-based interactions in healthcare is encouraging.
Secure voice pattern storage using blockchain integration holds considerable promise for enhancing the security of voice cloning in healthcare and beyond, including fields like audiobook production and podcast creation. Just as a fingerprint is a unique identifier, voiceprints derived from analyzing aspects of a person's voice like pitch and rhythm can serve as a digital key for accessing sensitive data. Storing these voiceprints on a blockchain creates an immutable and tamper-proof record, making it exceptionally challenging for unauthorized individuals to duplicate or alter a voice pattern.
Blockchain integration also allows for an unchangeable record of every instance a patient's voice data was accessed, including who accessed it and when. This not only fosters transparency but also boosts confidence in voice cloning applications within healthcare, demonstrating a clear path for accountability. A significant advantage of utilizing a blockchain is that it inherently offers a distributed and decentralized storage approach, mitigating the risk of a single point of failure often seen in traditional data systems. Should a cyberattack occur, the challenge of manipulating or copying a voice pattern across multiple nodes on the blockchain becomes far more complex, reinforcing security.
Voice authentication systems, leveraging the power of machine learning, are constantly evolving. They can adapt to changes in a person's voice over time, adjusting to the natural variations caused by aging or health fluctuations. This ongoing learning capability translates to smoother and more reliable access to patient data while maintaining security, lessening the chance of incorrectly rejecting legitimate users. This adaptability is crucial, as it makes the system resilient to normal changes in voice over time.
Additionally, by embedding blockchain technology, we can ensure strict compliance with data privacy and security regulations like HIPAA, creating auditable and timestamped records of each access request to a patient's voice data. This can provide valuable evidence during audits, helping healthcare organizations maintain compliance and avoid penalties. Although voice cloning is remarkably accurate, there's always a risk of malicious actors trying to impersonate a person. Blockchain-enabled voice authentication can be combined with "liveness detection," which dynamically checks that the voice it's receiving is truly real-time, a safeguard against pre-recorded voices being used to gain unauthorized access.
Beyond security, storing voice data within a blockchain framework facilitates dynamic encryption that can quickly adapt to changes in network conditions. This is crucial for telehealth consultations, which can experience unstable internet connectivity, ensuring audio quality is maintained without compromising data security during transmission. This ability to adjust to network changes helps make these systems usable in a broader range of settings.
It's also becoming clear that integrating other biometric factors like facial recognition or fingerprint scans alongside voice authentication on a blockchain offers an additional layer of protection. While offering many advantages, a significant challenge exists in achieving interoperability between different healthcare systems and platforms. Given the variety of existing health data systems, a collaborative effort is needed to establish consistent standards for securely and efficiently sharing voice data between them.
Voice pattern analysis extends beyond simple identification to potentially provide crucial health insights. By analyzing subtle changes in a patient's voice, we could detect anomalies that might signal shifts in their health or emotional state. Coupling this with blockchain storage for security means these observations could enhance remote patient monitoring and increase the ability of healthcare providers to respond in a timely and comprehensive way. While integrating blockchain and voice technologies into healthcare has a large potential to improve patient care, the question of how to standardize the exchange of voice data among diverse platforms is a significant obstacle that the research community will need to address in the coming years.
Voice Cloning in Healthcare 7 Key Security Protocols for Patient Data Protection in AI Voice Systems - Automated Voice Tampering Detection in Medical Voice Systems
The rise of voice cloning technology poses a significant threat to the integrity of medical voice systems, demanding the implementation of automated systems capable of detecting voice tampering. These systems can utilize advanced machine learning techniques, particularly neural networks, to discern subtle differences between genuine human speech and synthesized voices. By analyzing audio features and patterns, these systems can provide real-time alerts if a voice has been manipulated, preventing fraudulent activities that could compromise patient privacy or security. Such systems are crucial in safeguarding patient data and building trust in AI-driven healthcare interactions.
Real-time detection is paramount as the sophistication of voice cloning techniques continues to advance. The ability to rapidly identify manipulated voice data in real-time becomes a critical layer of defense against increasingly sophisticated attacks. This continuous monitoring also helps in integrating voice tampering detection with other existing security protocols, creating a holistic approach to safeguarding patient information.
While the development and implementation of these automated detection systems face ongoing challenges, the increasing potential for realistic voice cloning necessitates their continued refinement. Addressing the evolving threats posed by advanced voice synthesis techniques is paramount to protecting the integrity of the medical voice systems we rely on. The ongoing work in developing these detection capabilities is crucial to mitigating risks and ensuring the security of AI-driven healthcare interactions.
Automated voice tampering detection in medical voice systems is becoming increasingly important as voice cloning technology advances. These systems are designed to analyze various aspects of the audio signal to identify if a voice has been artificially altered or manipulated. By examining things like pitch variations, cadence, and other acoustic features, they can distinguish between authentic speech and synthetic alterations, helping ensure the integrity of medical communications.
One of the key aspects of this technology is the ability to perform frequency analysis on voice samples. This helps reveal spectral differences that might indicate digital manipulation. The advancements in digital signal processing make this analysis more precise, enabling quicker and more accurate detection of possible tampering.
Many of the current voice tampering detection systems rely on machine learning algorithms trained on substantial datasets containing both authentic and manipulated voices. These algorithms are constantly learning and improving, becoming better at recognizing and adapting to new methods of voice alteration. The potential for continuous improvement is crucial as voice cloning technology itself keeps evolving.
The ability to perform real-time detection during telehealth consultations is a particularly exciting development. This capability is vital for protecting the authenticity of patient-physician interactions. By spotting potential threats immediately, clinicians can take swift action to address any security issues.
Some systems even go beyond solely analyzing audio characteristics and incorporate multimodal analysis. By looking at visual cues such as lip movements and facial expressions during video calls, it's possible to improve the accuracy of voice tampering detection. This multi-layered approach increases confidence that an interaction is genuine.
Another method being investigated is the inclusion of behavioral biometrics. By establishing a baseline of a person's normal speech patterns and emotional tone, systems can flag unusual changes that may indicate tampering. This offers a more robust approach to identifying manipulation, particularly in critical healthcare environments.
The methods used for medical voice systems can be adapted for other applications like podcast production and audiobooks. This ability to detect manipulated audio helps to maintain the credibility and trust associated with these media formats, vital in maintaining audience engagement.
There are also important ethical considerations that must be addressed with these emerging technologies. Ensuring patients are aware that their voices are subject to tampering detection raises questions about informed consent and privacy. These issues require careful discussion and consideration when designing and implementing such systems.
Despite their potential, a significant challenge facing this field is the lack of universal standardization across various systems. Establishing common protocols and benchmarks would greatly improve the effectiveness of these systems and increase interoperability between different platforms.
While primarily focused on healthcare, voice tampering detection technology also has broader implications. It could have a role in other domains, including law enforcement, where recordings can be verified, education where the integrity of student work can be assessed, or even in corporate settings to protect meetings from intrusion attempts. These technologies will continue to evolve, with implications in various sectors.
Voice Cloning in Healthcare 7 Key Security Protocols for Patient Data Protection in AI Voice Systems - Voice Sample Isolation and Segmentation for Patient Privacy
In the context of voice cloning for healthcare, the ability to isolate and segment individual voice samples is crucial for protecting patient privacy. As AI-powered voice systems become more integrated into healthcare, the potential for misuse of sensitive voice data becomes a significant concern. By implementing advanced audio processing techniques, we can isolate and segment specific voice snippets from broader audio recordings. This careful extraction helps prevent the accidental leakage of identifiable patient information during the process of creating voice models. This practice not only enhances the security of voice-based interactions in telehealth but also fosters greater trust in the technology.
Ultimately, the goal is to strengthen the overall system for patient data protection in the growing digital healthcare environment. However, the field of voice cloning and AI is rapidly changing, presenting new and evolving challenges. Therefore, consistent innovation in sound isolation and segmentation methods is essential to ensure that these powerful technologies are employed responsibly and that patient confidentiality is never compromised.
Voice sample isolation and segmentation are becoming increasingly important in healthcare, particularly with the rise of voice cloning technology. These techniques allow systems to isolate individual voices from complex audio environments, like a busy hospital ward or a noisy telehealth consultation, enhancing the clarity and accuracy of voice recognition. Furthermore, these methods allow us to adapt to the natural changes in a person's voice over time, like those caused by aging or illness. This adaptive capability is crucial for maintaining the accuracy of voice-based authentication systems and ensuring that legitimate users are not incorrectly denied access to their data.
The way these technologies work is fascinating. Advanced algorithms try to mimic the human ear, parsing out individual voices and sounds even in the midst of significant background noise. This is crucial for preserving the integrity and privacy of patient data during telehealth consultations, where confidentiality is paramount. The ability to effectively separate voices allows clinicians to focus on relevant audio segments, improving the quality of remote interactions.
Interestingly, each individual's voice has a unique pattern or "voiceprint" much like a fingerprint. We can analyze features of a person's voice, such as their pitch and cadence, to create a kind of digital key for accessing sensitive data. This digital key concept is particularly important in voice cloning applications. Because each voiceprint is unique and difficult to replicate, it enhances the security protocols that protect patient information and can make it harder for malicious actors to gain unauthorized access.
Machine learning algorithms are central to the security aspects of voice sample isolation. These algorithms constantly monitor conversations in real-time, actively scanning for evidence of voice manipulation or synthetic voices. If a voice sounds unnatural or altered in any way, the system can send an immediate alert to the clinician, helping protect patient privacy and security. This approach is a crucial defense against increasingly sophisticated voice cloning attacks, ensuring that the integrity of healthcare interactions isn't compromised.
It's also remarkable that automated systems can analyze a person's voice and assess their emotional state based on subtleties like vocal tone and pace. This means that, during a telehealth visit, a doctor can gain valuable insight into how a patient is feeling, just by listening to their voice. This has great potential to improve the quality of healthcare, particularly when it comes to remote monitoring.
In order to establish a stronger security barrier, voice recognition is often paired with other biometric techniques, such as facial recognition. This multi-layered security approach adds an additional layer of protection against unauthorized access through voice cloning. It also holds promise in the realm of remote patient monitoring, where subtle changes in a patient's voice could serve as an early warning sign of a potential health issue or emotional distress, prompting faster intervention.
Blockchain technology, in its decentralized and immutable nature, can add a powerful layer of security to voice data storage. Integrating blockchain with voice analysis allows for tamper-proof records of voice data access and alterations, guaranteeing transparency and traceability. This approach enhances trust in voice-based systems and also helps streamline compliance with healthcare regulations like HIPAA. These systems would make audits easier and reduce the administrative burden on healthcare providers.
The benefits of voice sample isolation aren't restricted to healthcare. These methods are being explored in audiobook production and podcasting to maintain the integrity of audio content. Authenticity is critical in these domains, and the ability to detect manipulated audio using the same techniques that protect patients' medical information can help build trust and ensure content is free of alteration.
While the future of voice-based interactions in healthcare is filled with exciting possibilities, the challenges of ensuring security, privacy, and regulatory compliance remain. Continued research and innovation will be vital for developing and refining voice sample isolation and segmentation techniques, particularly in safeguarding sensitive information in an increasingly digital environment.
Voice Cloning in Healthcare 7 Key Security Protocols for Patient Data Protection in AI Voice Systems - End to End Voice Data Encryption for Remote Health Sessions
End-to-end voice data encryption is crucial for protecting the privacy of conversations during remote healthcare visits, a practice that has seen a substantial increase in popularity, especially since the pandemic. This type of encryption safeguards the voice data from the moment it leaves a patient's device until it reaches its destination, preventing any unauthorized access during its journey across the internet. Considering the sensitive nature of medical conversations and the increasing ability to clone voices, strong encryption is now more important than ever to ensure that interactions remain confidential and authentic. While the advantages of real-time encryption are clear, healthcare providers should also be aware of potential drawbacks, such as delays introduced into a conversation and the need for flexible encryption systems that can accommodate variable internet speeds. As telemedicine continues to grow in popularity, it's critical to prioritize security protocols like end-to-end encryption to foster a relationship built on trust between patients and healthcare systems and protect patients' sensitive information.
1. **Sound Wave Scrutiny**: Automated systems are increasingly using sophisticated analysis of sound wave patterns to identify if voice data has been manipulated. By examining the unique spectral fingerprints within voice samples, these systems can uncover evidence of digital alteration. This approach is vital for detecting synthesized or altered voices in medical applications.
2. **Adaptive Encryption**: Voice data encryption is becoming more sophisticated, employing machine learning to dynamically adjust to changes in voice quality and network conditions. This dynamic adaptation allows for seamless communication during remote health sessions, ensuring a good user experience while providing robust encryption. Although this adaptability is useful, engineers need to continuously monitor performance to make sure latency doesn't interfere with the delivery of medical care.
3. **Voice Uniqueness**: Innovative voice recognition technologies are exploring methods of "voice fingerprinting" which leverages unique features of a person's voice to create a distinctive identifier. This approach enhances security for accessing sensitive data because it makes it more challenging for unauthorized people to replicate an individual's voice. However, these methods need to account for the natural variations in a person's voice throughout their lifetime and across different speaking conditions.
4. **Emotional Tone Insights**: The analysis of subtle changes in a person's voice, specifically their tone, is becoming a method to monitor a patient's emotional state during remote consultations. This could give healthcare providers new tools to assess the well-being of a patient and adapt their care to potentially address subtle issues revealed through tone variations. It is fascinating, and while very promising, we need more work to ensure clinicians can properly interpret these signals.
5. **Real-Time Security**: By combining voice pattern analysis with biometrics, security systems can establish a baseline for an individual's usual voice characteristics. Then, any significant deviation from this normal pattern can trigger an alert, enabling a rapid response to potential security threats. It is essential to fine-tune these methods because human voices are dynamic and subtle changes can be caused by several things outside of a security threat.
6. **Broadening Impact**: Techniques developed to protect voice data in healthcare have the potential to expand to other fields like audiobook and podcast production. By ensuring the authenticity of audio content, these applications can protect the integrity of these forms of media and help maintain listener trust. However, it is crucial to consider the ethical and social implications of utilizing this technology in different settings.
7. **Streamlined Compliance**: The use of blockchain for voice data storage not only enhances security but also helps ensure compliance with regulations like HIPAA. The auditable record of every time a person accesses or modifies a person's voice data helps minimize compliance issues and reduce the likelihood of issues with audits. We still need to overcome several technological obstacles to ensure this vision of streamlining compliance becomes a reality.
8. **Multi-Sensory Analysis**: Some voice authentication systems are exploring the use of multi-modal analysis by incorporating visual data from video consultations to further improve accuracy. Comparing the movement of a person's lips in a video with the audio input can add an extra layer to help differentiate between natural and synthetic voices. However, further research is needed to determine the limitations and possibilities of this technology.
9. **Balancing Speed and Security**: Strong encryption methods like AES-256 are important for secure voice data transmission. However, these methods can introduce latency in the conversation. This tradeoff between speed and security must be carefully considered by engineers to ensure that even slight delays do not impede the quality of medical consultations. This will require research into efficient algorithms and adaptable protocols.
10. **Looking Ahead**: The integration of artificial intelligence (AI) with voice encryption offers future possibilities to identify unusual variations in a person's voice, which could possibly be indications of health changes. This intersection of security and health monitoring could significantly change how we approach remote care and potentially allow for proactive identification of patient needs. However, the successful integration of AI into healthcare will need rigorous study and testing to determine validity and reliability.
Get amazing AI audio voiceovers made for long-form content such as podcasts, presentations and social media. (Get started for free)
More Posts from clonemyvoice.io: